diff --git a/.circleci/test.yml b/.circleci/test.yml
index 5c57cd74b9..169bba2778 100644
--- a/.circleci/test.yml
+++ b/.circleci/test.yml
@@ -66,12 +66,17 @@ jobs:
mim install 'mmcv >= 2.0.0'
pip install git+https://git@github.com/open-mmlab/mmdetection.git@dev-3.x
pip install git+https://github.com/open-mmlab/mmclassification.git@dev-1.x
+ pip install git+https://github.com/open-mmlab/mmpretrain.git@dev
pip install git+https://github.com/open-mmlab/mmpose.git@dev-1.x
pip install -r requirements.txt
- run:
name: Install timm
command: |
pip install timm
+ - run:
+ name: Install transformers
+ command: |
+ pip install transformers
- when:
condition:
equal: [ "0.10.0", << parameters.torchvision >> ]
@@ -117,6 +122,10 @@ jobs:
command: |
docker exec mmaction pip install timm
docker exec mmaction python -m pip install pytorchvideo
+ - run:
+ name: Install transformers
+ command: |
+ docker exec mmaction pip install transformers
- run:
name: Install mmaction dependencies
command: |
@@ -126,6 +135,7 @@ jobs:
docker exec mmaction pip install git+https://git@github.com/open-mmlab/mmdetection.git@dev-3.x
docker exec mmaction pip install git+https://git@github.com/open-mmlab/mmpose.git@dev-1.x
docker exec mmaction pip install git+https://github.com/open-mmlab/mmclassification.git@dev-1.x
+ docker exec mmaction pip install git+https://github.com/open-mmlab/mmpretrain.git@dev
docker exec mmaction pip install -r requirements.txt
- run:
name: Build and install
diff --git a/.github/workflows/merge_stage_test.yml b/.github/workflows/merge_stage_test.yml
index 0b83911506..0a0222903a 100644
--- a/.github/workflows/merge_stage_test.yml
+++ b/.github/workflows/merge_stage_test.yml
@@ -60,6 +60,8 @@ jobs:
run: pip install git+https://github.com/open-mmlab/mmdetection.git@dev-3.x
- name: Install MMCls
run: pip install git+https://github.com/open-mmlab/mmclassification.git@dev-1.x
+ - name: Install MMPretrain
+ run: pip install git+https://github.com/open-mmlab/mmpretrain.git@dev
- name: Install MMPose
run: pip install git+https://github.com/open-mmlab/mmpose.git@dev-1.x
- name: Install PytorchVideo
@@ -67,6 +69,8 @@ jobs:
if: ${{matrix.torchvision == '0.10.0'}}
- name: Install timm
run: pip install timm
+ - name: Install transformers
+ run: pip install transformers
- name: Build and install
run: rm -rf .eggs && pip install -e .
- name: Run unittests and generate coverage report
@@ -108,6 +112,8 @@ jobs:
run: pip install lmdb
- name: Install timm
run: pip install timm
+ - name: Install transformers
+ run: pip install transformers
- name: Install TurboJpeg lib
run: sudo apt-get install -y libturbojpeg
- name: Install PyTorch
@@ -122,6 +128,8 @@ jobs:
run: pip install git+https://github.com/open-mmlab/mmdetection.git@dev-3.x
- name: Install MMCls
run: pip install git+https://github.com/open-mmlab/mmclassification.git@dev-1.x
+ - name: Install MMPretrain
+ run: pip install git+https://github.com/open-mmlab/mmpretrain.git@dev
- name: Install MMPose
run: pip install git+https://github.com/open-mmlab/mmpose.git@dev-1.x
- name: Install unittest dependencies
@@ -179,6 +187,8 @@ jobs:
run: pip install librosa soundfile
- name: Install lmdb
run: pip install lmdb
+ - name: Install transformers
+ run: pip install transformers
- name: Install mmaction dependencies
run: |
pip install git+https://github.com/open-mmlab/mmengine.git@main
@@ -186,6 +196,7 @@ jobs:
mim install 'mmcv >= 2.0.0'
pip install git+https://github.com/open-mmlab/mmdetection.git@dev-3.x
pip install git+https://github.com/open-mmlab/mmclassification.git@dev-1.x
+ pip install git+https://github.com/open-mmlab/mmpretrain.git@dev
pip install git+https://github.com/open-mmlab/mmpose.git@dev-1.x
pip install -r requirements.txt
- name: Install PytorchVideo
@@ -228,12 +239,15 @@ jobs:
mim install 'mmcv >= 2.0.0'
pip install git+https://github.com/open-mmlab/mmdetection.git@dev-3.x
pip install git+https://github.com/open-mmlab/mmclassification.git@dev-1.x
+ pip install git+https://github.com/open-mmlab/mmpretrain.git@dev
pip install git+https://github.com/open-mmlab/mmpose.git@dev-1.x
pip install -r requirements.txt
- name: Install PytorchVideo
run: python -m pip install pytorchvideo
- name: Install timm
run: python -m pip install timm
+ - name: Install transformers
+ run: python -m pip install transformers
- name: Build and install
run: |
pip install -e . -v
diff --git a/.github/workflows/pr_stage_test.yml b/.github/workflows/pr_stage_test.yml
index 2513d38596..63b9558e4b 100644
--- a/.github/workflows/pr_stage_test.yml
+++ b/.github/workflows/pr_stage_test.yml
@@ -51,6 +51,8 @@ jobs:
run: pip install git+https://github.com/open-mmlab/mmdetection.git@dev-3.x
- name: Install MMCls
run: pip install git+https://github.com/open-mmlab/mmclassification.git@dev-1.x
+ - name: Install MMPretrain
+ run: pip install git+https://github.com/open-mmlab/mmpretrain.git@dev
- name: Install MMPose
run: pip install git+https://github.com/open-mmlab/mmpose.git@dev-1.x
- name: Install unittest dependencies
@@ -119,6 +121,7 @@ jobs:
mim install 'mmcv >= 2.0.0'
pip install git+https://github.com/open-mmlab/mmdetection.git@dev-3.x
pip install git+https://github.com/open-mmlab/mmclassification.git@dev-1.x
+ pip install git+https://github.com/open-mmlab/mmpretrain.git@dev
pip install git+https://github.com/open-mmlab/mmpose.git@dev-1.x
pip install -r requirements.txt
- name: Install PytorchVideo
@@ -168,6 +171,7 @@ jobs:
mim install 'mmcv >= 2.0.0'
pip install git+https://github.com/open-mmlab/mmdetection.git@dev-3.x
pip install git+https://github.com/open-mmlab/mmclassification.git@dev-1.x
+ pip install git+https://github.com/open-mmlab/mmpretrain.git@dev
pip install git+https://github.com/open-mmlab/mmpose.git@dev-1.x
pip install -r requirements.txt
- name: Install PytorchVideo
diff --git a/README.md b/README.md
index b46a9dd41d..abf8fe59b1 100644
--- a/README.md
+++ b/README.md
@@ -75,14 +75,13 @@ English | [็ฎไฝไธญๆ](/README_zh-CN.md)
**The default branch has been switched to `main`(previous `1.x`) from `master`(current `0.x`), and we encourage users to migrate to the latest version with more supported models, stronger pre-training checkpoints and simpler coding. Please refer to [Migration Guide](https://mmaction2.readthedocs.io/en/latest/migration.html) for more details.**
-**Release (2023.07.04)**: v1.1.0 with the following new features:
-
-- Support CLIP-based multi-modality models: ActionCLIP(Arxiv'2021) and CLIP4clip(ArXiv'2022)
-- Support rich projects: gesture recognition, spatio-temporal action detection tutorial, and knowledge distillation
-- Support HACS-segments dataset(ICCV'2019), MultiSports dataset(ICCV'2021), Kinetics-710 dataset(Arxiv'2022)
-- Support VideoMAE V2(CVPR'2023), and VideoMAE(NeurIPS'2022) on action detection
-- Support TCANet(CVPR'2021)
-- Support [Pure Python style Configuration File](https://mmengine.readthedocs.io/en/latest/advanced_tutorials/config.html#a-pure-python-style-configuration-file-beta) and downloading datasets by MIM with one command
+**Release (2023.10.12)**: v1.2.0 with the following new features:
+
+- Support VindLU multi-modality algorithm and the Training of ActionClip
+- Support lightweight model MobileOne TSN/TSM
+- Support video retrieval dataset MSVD
+- Support SlowOnly K700 feature to train localization models
+- Support Video and Audio Demos
## ๐ Introduction [๐](#-table-of-contents)
diff --git a/README_zh-CN.md b/README_zh-CN.md
index 7cdea2c165..5e2b2ab241 100644
--- a/README_zh-CN.md
+++ b/README_zh-CN.md
@@ -26,7 +26,7 @@
[](https://github.com/open-mmlab/mmaction2/issues)
[๐ไธญๆๆๆกฃ](https://mmaction2.readthedocs.io/zh_CN/latest/index.html) |
-[๐ ๏ธๅฎ่ฃ
ๆๅ](https://mmaction2.readthedocs.io/zh_CN/get_started/installation.html) |
+[๐ ๏ธๅฎ่ฃ
ๆๅ](https://mmaction2.readthedocs.io/zh_CN/latest/get_started/installation.html) |
[๐ๆจกๅๅบ](https://mmaction2.readthedocs.io/zh_CN/latest/modelzoo_statistics.html) |
[๐ๆดๆฐๆฅๅฟ](https://mmaction2.readthedocs.io/en/latest/notes/changelog.html) |
[๐่ฟ่กไธญ้กน็ฎ](https://github.com/open-mmlab/mmaction2/projects) |
@@ -380,10 +380,10 @@ MMAction2 ๆฏไธๆฌพ็ฑๆฅ่ชไธๅ้ซๆ กๅไผไธ็็ ๅไบบๅๅ
ฑๅๅไธ่ดก
## โค๏ธ ๆฌข่ฟๅ ๅ
ฅ OpenMMLab ็คพๅบ [๐](#-table-of-contents)
-ๆซๆไธๆน็ไบ็ปด็ ๅฏๅ
ณๆณจ OpenMMLab ๅข้็ [็ฅไนๅฎๆน่ดฆๅท](https://www.zhihu.com/people/openmmlab)๏ผๅ ๅ
ฅ OpenMMLab ๅข้็ [ๅฎๆนไบคๆต QQ ็พค](https://jq.qq.com/?_wv=1027&k=aCvMxdr3) ๆ่็ป OpenMMLab ๅฎๆนๅพฎไฟกๅฐๅฉๆ
+ๆซๆไธๆน็ไบ็ปด็ ๅฏๅ
ณๆณจ OpenMMLab ๅข้็ [็ฅไนๅฎๆน่ดฆๅท](https://www.zhihu.com/people/openmmlab)๏ผๆซๆไธๆนๅพฎไฟกไบ็ปด็ ๆทปๅ ๅตๅตๅฅฝๅ๏ผ่ฟๅ
ฅ MMAction2 ๅพฎไฟกไบคๆต็คพ็พคใใๅ ๅฅฝๅ็ณ่ฏทๆ ผๅผ๏ผ็ ็ฉถๆนๅ+ๅฐๅบ+ๅญฆๆ ก/ๅ
ฌๅธ+ๅงๅใ
ๆไปฌไผๅจ OpenMMLab ็คพๅบไธบๅคงๅฎถ
diff --git a/configs/_base_/models/tsm_mobileone_s4.py b/configs/_base_/models/tsm_mobileone_s4.py
new file mode 100644
index 0000000000..df0c8f8c3c
--- /dev/null
+++ b/configs/_base_/models/tsm_mobileone_s4.py
@@ -0,0 +1,31 @@
+# model settings
+preprocess_cfg = dict(
+ mean=[123.675, 116.28, 103.53], std=[58.395, 57.12, 57.375])
+
+checkpoint = ('https://download.openmmlab.com/mmclassification/'
+ 'v0/mobileone/mobileone-s4_8xb32_in1k_20221110-28d888cb.pth')
+model = dict(
+ type='Recognizer2D',
+ backbone=dict(
+ type='MobileOneTSM',
+ arch='s4',
+ shift_div=8,
+ num_segments=8,
+ is_shift=True,
+ init_cfg=dict(
+ type='Pretrained', checkpoint=checkpoint, prefix='backbone')),
+ cls_head=dict(
+ type='TSMHead',
+ num_segments=8,
+ num_classes=400,
+ in_channels=2048,
+ spatial_type='avg',
+ consensus=dict(type='AvgConsensus', dim=1),
+ dropout_ratio=0.5,
+ init_std=0.001,
+ is_shift=True,
+ average_clips='prob'),
+ # model training and testing settings
+ data_preprocessor=dict(type='ActionDataPreprocessor', **preprocess_cfg),
+ train_cfg=None,
+ test_cfg=None)
diff --git a/configs/_base_/models/tsn_mobileone_s0.py b/configs/_base_/models/tsn_mobileone_s0.py
new file mode 100644
index 0000000000..83a070f143
--- /dev/null
+++ b/configs/_base_/models/tsn_mobileone_s0.py
@@ -0,0 +1,26 @@
+checkpoint = ('https://download.openmmlab.com/mmclassification/'
+ 'v0/mobileone/mobileone-s0_8xb32_in1k_20221110-0bc94952.pth')
+model = dict(
+ type='Recognizer2D',
+ backbone=dict(
+ type='mmpretrain.MobileOne',
+ arch='s0',
+ init_cfg=dict(
+ type='Pretrained', checkpoint=checkpoint, prefix='backbone'),
+ norm_eval=False),
+ cls_head=dict(
+ type='TSNHead',
+ num_classes=400,
+ in_channels=1024,
+ spatial_type='avg',
+ consensus=dict(type='AvgConsensus', dim=1),
+ dropout_ratio=0.4,
+ init_std=0.01,
+ average_clips='prob'),
+ data_preprocessor=dict(
+ type='ActionDataPreprocessor',
+ mean=[123.675, 116.28, 103.53],
+ std=[58.395, 57.12, 57.375],
+ format_shape='NCHW'),
+ train_cfg=None,
+ test_cfg=None)
diff --git a/configs/_base_/models/tsn_r18_audio.py b/configs/_base_/models/tsn_r18_audio.py
deleted file mode 100644
index be21b44c0b..0000000000
--- a/configs/_base_/models/tsn_r18_audio.py
+++ /dev/null
@@ -1,11 +0,0 @@
-# model settings
-model = dict(
- type='RecognizerAudio',
- backbone=dict(type='ResNet', depth=18, in_channels=1, norm_eval=False),
- cls_head=dict(
- type='TSNAudioHead',
- num_classes=400,
- in_channels=512,
- dropout_ratio=0.5,
- init_std=0.01,
- average_clips='prob'))
diff --git a/configs/detection/acrn/slowfast-acrn_kinetics400-pretrained-r50_8xb8-8x8x1-cosine-10e_ava21-rgb.py b/configs/detection/acrn/slowfast-acrn_kinetics400-pretrained-r50_8xb8-8x8x1-cosine-10e_ava21-rgb.py
index 10928a96ee..0b183ae812 100644
--- a/configs/detection/acrn/slowfast-acrn_kinetics400-pretrained-r50_8xb8-8x8x1-cosine-10e_ava21-rgb.py
+++ b/configs/detection/acrn/slowfast-acrn_kinetics400-pretrained-r50_8xb8-8x8x1-cosine-10e_ava21-rgb.py
@@ -46,6 +46,7 @@
shared_head=dict(type='ACRNHead', in_channels=4608, out_channels=2304),
bbox_head=dict(
type='BBoxHeadAVA',
+ background_class=True,
in_channels=2304,
num_classes=81,
multilabel=True,
@@ -88,9 +89,6 @@
proposal_file_val = f'{anno_root}/ava_dense_proposals_val.FAIR.recall_93.9.pkl'
file_client_args = dict(io_backend='disk')
-file_client_args = dict(
- io_backend='petrel',
- path_mapping=dict({'data/ava': 's254:s3://openmmlab/datasets/action/ava'}))
train_pipeline = [
dict(type='SampleAVAFrames', clip_len=32, frame_interval=2),
dict(type='RawFrameDecode', **file_client_args),
diff --git a/configs/detection/acrn/slowfast-acrn_kinetics400-pretrained-r50_8xb8-8x8x1-cosine-10e_ava22-rgb.py b/configs/detection/acrn/slowfast-acrn_kinetics400-pretrained-r50_8xb8-8x8x1-cosine-10e_ava22-rgb.py
index 4537d25cc7..3357d9c3ca 100644
--- a/configs/detection/acrn/slowfast-acrn_kinetics400-pretrained-r50_8xb8-8x8x1-cosine-10e_ava22-rgb.py
+++ b/configs/detection/acrn/slowfast-acrn_kinetics400-pretrained-r50_8xb8-8x8x1-cosine-10e_ava22-rgb.py
@@ -46,6 +46,7 @@
shared_head=dict(type='ACRNHead', in_channels=4608, out_channels=2304),
bbox_head=dict(
type='BBoxHeadAVA',
+ background_class=True,
in_channels=2304,
num_classes=81,
multilabel=True,
diff --git a/configs/detection/lfb/slowonly-lfb-infer_r50_ava21-rgb.py b/configs/detection/lfb/slowonly-lfb-infer_r50_ava21-rgb.py
index 278d87c1e1..6c1c60d7e4 100644
--- a/configs/detection/lfb/slowonly-lfb-infer_r50_ava21-rgb.py
+++ b/configs/detection/lfb/slowonly-lfb-infer_r50_ava21-rgb.py
@@ -34,6 +34,7 @@
with_temporal_pool=True),
bbox_head=dict(
type='BBoxHeadAVA',
+ background_class=True,
in_channels=2048,
num_classes=81,
multilabel=True,
diff --git a/configs/detection/lfb/slowonly-lfb-nl_kinetics400-pretrained-r50_8xb12-4x16x1-20e_ava21-rgb.py b/configs/detection/lfb/slowonly-lfb-nl_kinetics400-pretrained-r50_8xb12-4x16x1-20e_ava21-rgb.py
index 9d323ad0e4..5b1a837864 100644
--- a/configs/detection/lfb/slowonly-lfb-nl_kinetics400-pretrained-r50_8xb12-4x16x1-20e_ava21-rgb.py
+++ b/configs/detection/lfb/slowonly-lfb-nl_kinetics400-pretrained-r50_8xb12-4x16x1-20e_ava21-rgb.py
@@ -37,6 +37,7 @@
with_temporal_pool=True),
bbox_head=dict(
type='BBoxHeadAVA',
+ background_class=True,
in_channels=2560,
num_classes=81,
multilabel=True,
diff --git a/configs/detection/lfb/slowonly-lfb_ava-pretrained-r50_infer-4x16x1_ava21-rgb.py b/configs/detection/lfb/slowonly-lfb_ava-pretrained-r50_infer-4x16x1_ava21-rgb.py
index 2ba637545c..377da48f07 100644
--- a/configs/detection/lfb/slowonly-lfb_ava-pretrained-r50_infer-4x16x1_ava21-rgb.py
+++ b/configs/detection/lfb/slowonly-lfb_ava-pretrained-r50_infer-4x16x1_ava21-rgb.py
@@ -34,6 +34,7 @@
with_temporal_pool=True),
bbox_head=dict(
type='BBoxHeadAVA',
+ background_class=True,
in_channels=2048,
num_classes=81,
multilabel=True,
diff --git a/configs/detection/slowfast/slowfast_kinetics400-pretrained-r50_8xb16-4x16x1-20e_ava21-rgb.py b/configs/detection/slowfast/slowfast_kinetics400-pretrained-r50_8xb16-4x16x1-20e_ava21-rgb.py
index 0eb0e501e3..89cc9078ef 100644
--- a/configs/detection/slowfast/slowfast_kinetics400-pretrained-r50_8xb16-4x16x1-20e_ava21-rgb.py
+++ b/configs/detection/slowfast/slowfast_kinetics400-pretrained-r50_8xb16-4x16x1-20e_ava21-rgb.py
@@ -44,6 +44,7 @@
with_temporal_pool=True),
bbox_head=dict(
type='BBoxHeadAVA',
+ background_class=True,
in_channels=2304,
num_classes=81,
multilabel=True,
diff --git a/configs/detection/slowfast/slowfast_kinetics400-pretrained-r50_8xb6-8x8x1-cosine-10e_ava22-rgb.py b/configs/detection/slowfast/slowfast_kinetics400-pretrained-r50_8xb6-8x8x1-cosine-10e_ava22-rgb.py
index debeb5c7fd..a34af4fb62 100644
--- a/configs/detection/slowfast/slowfast_kinetics400-pretrained-r50_8xb6-8x8x1-cosine-10e_ava22-rgb.py
+++ b/configs/detection/slowfast/slowfast_kinetics400-pretrained-r50_8xb6-8x8x1-cosine-10e_ava22-rgb.py
@@ -45,6 +45,7 @@
with_temporal_pool=True),
bbox_head=dict(
type='BBoxHeadAVA',
+ background_class=True,
in_channels=2304,
num_classes=81,
multilabel=True,
diff --git a/configs/detection/slowfast/slowfast_kinetics400-pretrained-r50_8xb8-8x8x1-20e_ava21-rgb.py b/configs/detection/slowfast/slowfast_kinetics400-pretrained-r50_8xb8-8x8x1-20e_ava21-rgb.py
index 1e94a10960..00f3e491a8 100644
--- a/configs/detection/slowfast/slowfast_kinetics400-pretrained-r50_8xb8-8x8x1-20e_ava21-rgb.py
+++ b/configs/detection/slowfast/slowfast_kinetics400-pretrained-r50_8xb8-8x8x1-20e_ava21-rgb.py
@@ -45,6 +45,7 @@
with_temporal_pool=True),
bbox_head=dict(
type='BBoxHeadAVA',
+ background_class=True,
in_channels=2304,
num_classes=81,
multilabel=True,
diff --git a/configs/detection/slowonly/slowonly_k400-pre-r50_8xb8-4x16x1-10e_ava-kinetics-rgb.py b/configs/detection/slowonly/slowonly_k400-pre-r50_8xb8-4x16x1-10e_ava-kinetics-rgb.py
index fd44f336ac..d35cf5331a 100644
--- a/configs/detection/slowonly/slowonly_k400-pre-r50_8xb8-4x16x1-10e_ava-kinetics-rgb.py
+++ b/configs/detection/slowonly/slowonly_k400-pre-r50_8xb8-4x16x1-10e_ava-kinetics-rgb.py
@@ -29,6 +29,7 @@
with_temporal_pool=True),
bbox_head=dict(
type='BBoxHeadAVA',
+ background_class=True,
in_channels=2048,
num_classes=81,
multilabel=True,
diff --git a/configs/detection/slowonly/slowonly_k400-pre-r50_8xb8-8x8x1-10e_ava-kinetics-rgb.py b/configs/detection/slowonly/slowonly_k400-pre-r50_8xb8-8x8x1-10e_ava-kinetics-rgb.py
index 4af750e8ad..1e59cd3494 100644
--- a/configs/detection/slowonly/slowonly_k400-pre-r50_8xb8-8x8x1-10e_ava-kinetics-rgb.py
+++ b/configs/detection/slowonly/slowonly_k400-pre-r50_8xb8-8x8x1-10e_ava-kinetics-rgb.py
@@ -29,6 +29,7 @@
with_temporal_pool=True),
bbox_head=dict(
type='BBoxHeadAVA',
+ background_class=True,
in_channels=2048,
num_classes=81,
multilabel=True,
diff --git a/configs/detection/slowonly/slowonly_kinetics400-pretrained-r101_8xb16-8x8x1-20e_ava21-rgb.py b/configs/detection/slowonly/slowonly_kinetics400-pretrained-r101_8xb16-8x8x1-20e_ava21-rgb.py
index 9bee13a25c..fc83f9e34b 100644
--- a/configs/detection/slowonly/slowonly_kinetics400-pretrained-r101_8xb16-8x8x1-20e_ava21-rgb.py
+++ b/configs/detection/slowonly/slowonly_kinetics400-pretrained-r101_8xb16-8x8x1-20e_ava21-rgb.py
@@ -28,6 +28,7 @@
with_temporal_pool=True),
bbox_head=dict(
type='BBoxHeadAVA',
+ background_class=True,
in_channels=2048,
num_classes=81,
multilabel=True,
diff --git a/configs/detection/slowonly/slowonly_kinetics400-pretrained-r50-nl_8xb16-4x16x1-20e_ava21-rgb.py b/configs/detection/slowonly/slowonly_kinetics400-pretrained-r50-nl_8xb16-4x16x1-20e_ava21-rgb.py
index cdc8ea8d98..38b1e7605e 100644
--- a/configs/detection/slowonly/slowonly_kinetics400-pretrained-r50-nl_8xb16-4x16x1-20e_ava21-rgb.py
+++ b/configs/detection/slowonly/slowonly_kinetics400-pretrained-r50-nl_8xb16-4x16x1-20e_ava21-rgb.py
@@ -36,6 +36,7 @@
with_temporal_pool=True),
bbox_head=dict(
type='BBoxHeadAVA',
+ background_class=True,
in_channels=2048,
num_classes=81,
multilabel=True,
diff --git a/configs/detection/slowonly/slowonly_kinetics400-pretrained-r50-nl_8xb16-8x8x1-20e_ava21-rgb.py b/configs/detection/slowonly/slowonly_kinetics400-pretrained-r50-nl_8xb16-8x8x1-20e_ava21-rgb.py
index 9b6dd00fdb..ee6335ecac 100644
--- a/configs/detection/slowonly/slowonly_kinetics400-pretrained-r50-nl_8xb16-8x8x1-20e_ava21-rgb.py
+++ b/configs/detection/slowonly/slowonly_kinetics400-pretrained-r50-nl_8xb16-8x8x1-20e_ava21-rgb.py
@@ -36,6 +36,7 @@
with_temporal_pool=True),
bbox_head=dict(
type='BBoxHeadAVA',
+ background_class=True,
in_channels=2048,
num_classes=81,
multilabel=True,
diff --git a/configs/detection/slowonly/slowonly_kinetics400-pretrained-r50_8xb16-4x16x1-20e_ava21-rgb.py b/configs/detection/slowonly/slowonly_kinetics400-pretrained-r50_8xb16-4x16x1-20e_ava21-rgb.py
index a83408c84a..ddb5f34cb4 100644
--- a/configs/detection/slowonly/slowonly_kinetics400-pretrained-r50_8xb16-4x16x1-20e_ava21-rgb.py
+++ b/configs/detection/slowonly/slowonly_kinetics400-pretrained-r50_8xb16-4x16x1-20e_ava21-rgb.py
@@ -29,6 +29,7 @@
with_temporal_pool=True),
bbox_head=dict(
type='BBoxHeadAVA',
+ background_class=True,
in_channels=2048,
num_classes=81,
multilabel=True,
diff --git a/configs/detection/slowonly/slowonly_kinetics400-pretrained-r50_8xb16-4x16x1-8e_multisports-rgb.py b/configs/detection/slowonly/slowonly_kinetics400-pretrained-r50_8xb16-4x16x1-8e_multisports-rgb.py
index 0d83ca0d48..8b1887eac1 100644
--- a/configs/detection/slowonly/slowonly_kinetics400-pretrained-r50_8xb16-4x16x1-8e_multisports-rgb.py
+++ b/configs/detection/slowonly/slowonly_kinetics400-pretrained-r50_8xb16-4x16x1-8e_multisports-rgb.py
@@ -30,6 +30,7 @@
with_temporal_pool=True),
bbox_head=dict(
type='BBoxHeadAVA',
+ background_class=False,
in_channels=2048,
num_classes=num_classes,
multilabel=False,
diff --git a/configs/detection/slowonly/slowonly_kinetics700-pretrained-r50_8xb16-4x16x1-20e_ava21-rgb.py b/configs/detection/slowonly/slowonly_kinetics700-pretrained-r50_8xb16-4x16x1-20e_ava21-rgb.py
index a68893a015..3df1b248f7 100644
--- a/configs/detection/slowonly/slowonly_kinetics700-pretrained-r50_8xb16-4x16x1-20e_ava21-rgb.py
+++ b/configs/detection/slowonly/slowonly_kinetics700-pretrained-r50_8xb16-4x16x1-20e_ava21-rgb.py
@@ -29,6 +29,7 @@
with_temporal_pool=True),
bbox_head=dict(
type='BBoxHeadAVA',
+ background_class=True,
in_channels=2048,
num_classes=81,
multilabel=True,
diff --git a/configs/detection/videomae/vit-base-p16_videomae-k400-pre_8xb8-16x4x1-20e-adamw_ava-kinetics-rgb.py b/configs/detection/videomae/vit-base-p16_videomae-k400-pre_8xb8-16x4x1-20e-adamw_ava-kinetics-rgb.py
index 6e5950b847..8ba9c7a22a 100644
--- a/configs/detection/videomae/vit-base-p16_videomae-k400-pre_8xb8-16x4x1-20e-adamw_ava-kinetics-rgb.py
+++ b/configs/detection/videomae/vit-base-p16_videomae-k400-pre_8xb8-16x4x1-20e-adamw_ava-kinetics-rgb.py
@@ -31,6 +31,7 @@
with_temporal_pool=True),
bbox_head=dict(
type='BBoxHeadAVA',
+ background_class=True,
in_channels=768,
num_classes=81,
multilabel=True,
diff --git a/configs/detection/videomae/vit-large-p16_videomae-k400-pre_8xb8-16x4x1-20e-adamw_ava-kinetics-rgb.py b/configs/detection/videomae/vit-large-p16_videomae-k400-pre_8xb8-16x4x1-20e-adamw_ava-kinetics-rgb.py
index 229f3ae013..a97eebf898 100644
--- a/configs/detection/videomae/vit-large-p16_videomae-k400-pre_8xb8-16x4x1-20e-adamw_ava-kinetics-rgb.py
+++ b/configs/detection/videomae/vit-large-p16_videomae-k400-pre_8xb8-16x4x1-20e-adamw_ava-kinetics-rgb.py
@@ -32,6 +32,7 @@
with_temporal_pool=True),
bbox_head=dict(
type='BBoxHeadAVA',
+ background_class=True,
in_channels=1024,
num_classes=81,
multilabel=True,
diff --git a/configs/localization/bmn/README.md b/configs/localization/bmn/README.md
index ec2f625a95..f30b3a5d40 100644
--- a/configs/localization/bmn/README.md
+++ b/configs/localization/bmn/README.md
@@ -23,11 +23,12 @@ Temporal action proposal generation is an challenging and promising task which a
| feature | gpus | pretrain | AUC | AR@1 | AR@5 | AR@10 | AR@100 | gpu_mem(M) | iter time(s) | config | ckpt | log |
| :-----------: | :--: | :------: | :---: | :---: | :---: | :---: | :----: | :--------: | :----------: | :------------------------------------------: | :----------------------------------------: | :---------------------------------------: |
| cuhk_mean_100 | 2 | None | 67.25 | 32.89 | 49.43 | 56.64 | 75.29 | 5412 | - | [config](/configs/localization/bmn/bmn_2xb8-400x100-9e_activitynet-feature.py) | [ckpt](https://download.openmmlab.com/mmaction/v1.0/localization/bmn/bmn_2xb8-400x100-9e_activitynet-feature_20220908-79f92857.pth) | [log](https://download.openmmlab.com/mmaction/v1.0/localization/bmn/bmn_2xb8-400x100-9e_activitynet-feature.log) |
+| slowonly-k700 | 2 | None | 68.04 | 33.44 | 50.53 | 57.65 | 75.77 | - | - | [config](/configs/localization/bmn/bmn_2xb8-2048x100-9e_activitynet-slowonly-k700-feature.py) | [ckpt](https://download.openmmlab.com/mmaction/v1.0/localization/bmn/bmn_2xb8-2048x100-9e_activitynet-slowonly-k700-feature_20230907-50b939b2.pth) | [log](https://download.openmmlab.com/mmaction/v1.0/localization/bmn/bmn_2xb8-2048x100-9e_activitynet-slowonly-k700-feature.log) |
1. The **gpus** indicates the number of gpu we used to get the checkpoint.
According to the [Linear Scaling Rule](https://arxiv.org/abs/1706.02677), you may set the learning rate proportional to the batch size if you use different GPUs or videos per GPU,
e.g., lr=0.01 for 4 GPUs x 2 video/gpu and lr=0.08 for 16 GPUs x 4 video/gpu.
-2. For feature column, cuhk_mean_100 denotes the widely used cuhk activitynet feature extracted by [anet2016-cuhk](https://github.com/yjxiong/anet2016-cuhk).
+2. For feature column, cuhk_mean_100 denotes the widely used cuhk activitynet feature extracted by [anet2016-cuhk](https://github.com/yjxiong/anet2016-cuhk). The slowonly-k700 denotes the feature extracted using MMAction2's [SlowOnly model trained on Kinetics 700](/configs/recognition/slowonly/slowonly_imagenet-pretrained-r50_16xb16-8x8x1-steplr-150e_kinetics700-rgb.py). You can download this feature from [ActivityNet Data Preparation](/tools/data/activitynet/README.md).
3. We evaluate the action detection performance of BMN, using [anet_cuhk_2017](https://download.openmmlab.com/mmaction/localization/cuhk_anet17_pred.json) submission for ActivityNet2017 Untrimmed Video Classification Track to assign label for each action proposal.
\*We train BMN with the [official repo](https://github.com/JJBOY/BMN-Boundary-Matching-Network), evaluate its proposal generation and action detection performance with [anet_cuhk_2017](https://download.openmmlab.com/mmaction/localization/cuhk_anet17_pred.json) for label assigning.
@@ -42,6 +43,12 @@ Train BMN model on ActivityNet features dataset.
bash tools/dist_train.sh configs/localization/bmn/bmn_2xb8-400x100-9e_activitynet-feature.py 2
```
+Train BMN model on ActivityNet SlowOnly-K700 features dataset.
+
+```shell
+bash tools/dist_train.sh configs/localization/bmn/bmn_2xb8-2048x100-9e_activitynet-slowonly-k700-feature.py 2
+```
+
For more details, you can refer to the **Training** part in the [Training and Test Tutorial](/docs/en/user_guides/train_test.md).
## Test
diff --git a/configs/localization/bmn/bmn_2xb8-2048x100-9e_activitynet-slowonly-k700-feature.py b/configs/localization/bmn/bmn_2xb8-2048x100-9e_activitynet-slowonly-k700-feature.py
new file mode 100644
index 0000000000..9230578a86
--- /dev/null
+++ b/configs/localization/bmn/bmn_2xb8-2048x100-9e_activitynet-slowonly-k700-feature.py
@@ -0,0 +1,110 @@
+_base_ = [
+ '../../_base_/models/bmn_400x100.py', '../../_base_/default_runtime.py'
+]
+
+model = dict(feat_dim=2048)
+
+# dataset settings
+dataset_type = 'ActivityNetDataset'
+data_root = 'data/ActivityNet/k700slowonly'
+data_root_val = 'data/ActivityNet/k700slowonly'
+ann_file_train = 'data/ActivityNet/anet_anno_train.json'
+ann_file_val = 'data/ActivityNet/anet_anno_val.json'
+ann_file_test = 'data/ActivityNet/anet_anno_val.json'
+
+train_pipeline = [
+ dict(type='LoadLocalizationFeature'),
+ dict(type='GenerateLocalizationLabels'),
+ dict(
+ type='PackLocalizationInputs',
+ keys=('gt_bbox', ),
+ meta_keys=('video_name', ))
+]
+
+val_pipeline = [
+ dict(type='LoadLocalizationFeature'),
+ dict(type='GenerateLocalizationLabels'),
+ dict(
+ type='PackLocalizationInputs',
+ keys=('gt_bbox', ),
+ meta_keys=('video_name', 'duration_second', 'duration_frame',
+ 'annotations', 'feature_frame'))
+]
+
+test_pipeline = [
+ dict(type='LoadLocalizationFeature'),
+ dict(
+ type='PackLocalizationInputs',
+ keys=('gt_bbox', ),
+ meta_keys=('video_name', 'duration_second', 'duration_frame',
+ 'annotations', 'feature_frame'))
+]
+
+train_dataloader = dict(
+ batch_size=8,
+ num_workers=8,
+ persistent_workers=True,
+ sampler=dict(type='DefaultSampler', shuffle=True),
+ drop_last=True,
+ dataset=dict(
+ type=dataset_type,
+ ann_file=ann_file_train,
+ data_prefix=dict(video=data_root),
+ pipeline=train_pipeline))
+
+val_dataloader = dict(
+ batch_size=1,
+ num_workers=8,
+ persistent_workers=True,
+ sampler=dict(type='DefaultSampler', shuffle=False),
+ dataset=dict(
+ type=dataset_type,
+ ann_file=ann_file_val,
+ data_prefix=dict(video=data_root_val),
+ pipeline=val_pipeline,
+ test_mode=True))
+
+test_dataloader = dict(
+ batch_size=1,
+ num_workers=8,
+ persistent_workers=True,
+ sampler=dict(type='DefaultSampler', shuffle=False),
+ dataset=dict(
+ type=dataset_type,
+ ann_file=ann_file_test,
+ data_prefix=dict(video=data_root_val),
+ pipeline=test_pipeline,
+ test_mode=True))
+
+max_epochs = 9
+train_cfg = dict(
+ type='EpochBasedTrainLoop',
+ max_epochs=max_epochs,
+ val_begin=1,
+ val_interval=1)
+
+val_cfg = dict(type='ValLoop')
+test_cfg = dict(type='TestLoop')
+
+optim_wrapper = dict(
+ optimizer=dict(type='Adam', lr=0.001, weight_decay=0.0001),
+ clip_grad=dict(max_norm=40, norm_type=2))
+
+param_scheduler = [
+ dict(
+ type='MultiStepLR',
+ begin=0,
+ end=max_epochs,
+ by_epoch=True,
+ milestones=[
+ 7,
+ ],
+ gamma=0.1)
+]
+
+work_dir = './work_dirs/bmn_400x100_2x8_9e_activitynet_feature/'
+test_evaluator = dict(
+ type='ANetMetric',
+ metric_type='AR@AN',
+ dump_config=dict(out=f'{work_dir}/results.json', output_format='json'))
+val_evaluator = test_evaluator
diff --git a/configs/localization/bsn/README.md b/configs/localization/bsn/README.md
index efd2d2c0d0..da52d1375d 100644
--- a/configs/localization/bsn/README.md
+++ b/configs/localization/bsn/README.md
@@ -23,17 +23,20 @@ Temporal action proposal generation is an important yet challenging problem, sin
| feature | gpus | pretrain | AUC | AR@1 | AR@5 | AR@10 | AR@100 | gpu_mem(M) | iter time(s) | config | ckpt | log |
| :-----------: | :--: | :------: | :---: | :---: | :---: | :---: | :----: | :-------------: | :----------: | :----------------------------------------: | :--------------------------------------: | :--------------------------------------: |
| cuhk_mean_100 | 1 | None | 66.26 | 32.71 | 48.43 | 55.28 | 74.27 | 43(TEM)+25(PEM) | - | [config_TEM](/configs/localization/bsn/bsn_tem_1xb16-400x100-20e_activitynet-feature.py) [config_PGM](/configs/localization/bsn/bsn_pgm_400x100_activitynet-feature.py) [config_PEM](/configs/localization/bsn/bsn_pem_1xb16-400x100-20e_activitynet-feature.py) | [ckpt_TEM](https://download.openmmlab.com/mmaction/v1.0/localization/bsn/bsn_tem_1xb16-400x100-20e_activitynet-feature_20220908-9da79951.pth) [ckpt_PEM](https://download.openmmlab.com/mmaction/v1.0/localization/bsn/bsn_pem_1xb16-400x100-20e_activitynet-feature_20220908-ec2eb21d.pth) | [log_tem](https://download.openmmlab.com/mmaction/v1.0/localization/bsn/bsn_tem_1xb16-400x100-20e_activitynet-feature.log) [log_pem](https://download.openmmlab.com/mmaction/v1.0/localization/bsn/bsn_pem_1xb16-400x100-20e_activitynet-feature.log) |
+| slowonly-k700 | 1 | None | 67.63 | 33.04 | 48.79 | 56.01 | 75.74 | - | - | [config_TEM](/configs/localization/bsn/bsn_tem_1xb16-2048x100-20e_activitynet-k700-feature.py) [config_PGM](/configs/localization/bsn/bsn_pgm_2048x100_activitynet-slowonly-k700-feature.py) [config_PEM](/configs/localization/bsn/bsn_pem_1xb16-2048x100-20e_activitynet-slowonly-k700-feature.py) | [ckpt_TEM](https://download.openmmlab.com/mmaction/v1.0/localization/bsn/bsn_tem_1xb16-2048x100-20e_activitynet-k700-feature_20230907-76069fda.pth) [ckpt_PEM](https://download.openmmlab.com/mmaction/v1.0/localization/bsn/bsn_pem_1xb16-2048x100-20e_activitynet-slowonly-k700-feature_20230907-44158b6d.pth) | [log_tem](https://download.openmmlab.com/mmaction/v1.0/localization/bsn/bsn_tem_1xb16-400x100-20e_activitynet-feature.log) [log_pem](https://download.openmmlab.com/mmaction/v1.0/localization/bsn/bsn_pem_1xb16-400x100-20e_activitynet-feature.log) |
1. The **gpus** indicates the number of gpu we used to get the checkpoint.
According to the [Linear Scaling Rule](https://arxiv.org/abs/1706.02677), you may set the learning rate proportional to the batch size if you use different GPUs or videos per GPU,
e.g., lr=0.01 for 4 GPUs x 2 video/gpu and lr=0.08 for 16 GPUs x 4 video/gpu.
-2. For feature column, cuhk_mean_100 denotes the widely used cuhk activitynet feature extracted by [anet2016-cuhk](https://github.com/yjxiong/anet2016-cuhk).
+2. For feature column, cuhk_mean_100 denotes the widely used cuhk activitynet feature extracted by [anet2016-cuhk](https://github.com/yjxiong/anet2016-cuhk). The slowonly-k700 denotes the feature extracted using MMAction2's [SlowOnly model trained on Kinetics 700](/configs/recognition/slowonly/slowonly_imagenet-pretrained-r50_16xb16-8x8x1-steplr-150e_kinetics700-rgb.py). You can download this feature from [ActivityNet Data Preparation](/tools/data/activitynet/README.md).
For more details on data preparation, you can refer to [ActivityNet Data Preparation](/tools/data/activitynet/README.md).
## Training and Test
-The traing of the BSN model is three-stages. Firstly train the Temporal evaluation module (TEM):
+The traing of the BSN model is three-stages. We take the `cuhk_mean_100` feature as an example. For `slowonly-k700` feature, just need to replace the config file with the corresponding config file with `slowonly-k700` in the file name.
+
+Firstly train the Temporal evaluation module (TEM):
```shell
python3 tools/train.py configs/localization/bsn/bsn_tem_1xb16-400x100-20e_activitynet-feature.py
diff --git a/configs/localization/bsn/bsn_pem_1xb16-2048x100-20e_activitynet-slowonly-k700-feature.py b/configs/localization/bsn/bsn_pem_1xb16-2048x100-20e_activitynet-slowonly-k700-feature.py
new file mode 100644
index 0000000000..25bb7df698
--- /dev/null
+++ b/configs/localization/bsn/bsn_pem_1xb16-2048x100-20e_activitynet-slowonly-k700-feature.py
@@ -0,0 +1,84 @@
+_base_ = [
+ '../../_base_/models/bsn_pem.py', '../../_base_/schedules/adam_20e.py',
+ '../../_base_/default_runtime.py'
+]
+
+# dataset settings
+dataset_type = 'ActivityNetDataset'
+data_root = 'data/ActivityNet/k700slowonly'
+data_root_val = 'data/ActivityNet/k700slowonly'
+ann_file_train = 'data/ActivityNet/anet_anno_train.json'
+ann_file_val = 'data/ActivityNet/anet_anno_val.json'
+ann_file_test = 'data/ActivityNet/anet_anno_val.json'
+
+work_dir = 'work_dirs/bsn_400x100_20e_1x16_activitynet_feature/'
+pgm_proposals_dir = f'{work_dir}/pgm_proposals/'
+pgm_features_dir = f'{work_dir}/pgm_features/'
+
+train_pipeline = [
+ dict(
+ type='LoadProposals',
+ top_k=500,
+ pgm_proposals_dir=pgm_proposals_dir,
+ pgm_features_dir=pgm_features_dir),
+ dict(
+ type='PackLocalizationInputs',
+ keys=('reference_temporal_iou', 'bsp_feature'),
+ meta_keys=())
+]
+val_pipeline = [
+ dict(
+ type='LoadProposals',
+ top_k=1000,
+ pgm_proposals_dir=pgm_proposals_dir,
+ pgm_features_dir=pgm_features_dir),
+ dict(
+ type='PackLocalizationInputs',
+ keys=('tmin', 'tmax', 'tmin_score', 'tmax_score', 'bsp_feature'),
+ meta_keys=('video_name', 'duration_second', 'duration_frame',
+ 'annotations', 'feature_frame')),
+]
+test_pipeline = val_pipeline
+
+train_dataloader = dict(
+ batch_size=16,
+ num_workers=8,
+ persistent_workers=True,
+ sampler=dict(type='DefaultSampler', shuffle=True),
+ dataset=dict(
+ type=dataset_type,
+ ann_file=ann_file_train,
+ data_prefix=dict(video=data_root),
+ pipeline=train_pipeline))
+
+val_dataloader = dict(
+ batch_size=1,
+ num_workers=8,
+ persistent_workers=True,
+ sampler=dict(type='DefaultSampler', shuffle=False),
+ dataset=dict(
+ type=dataset_type,
+ ann_file=ann_file_val,
+ data_prefix=dict(video=data_root_val),
+ pipeline=val_pipeline,
+ test_mode=True))
+
+test_dataloader = dict(
+ batch_size=1,
+ num_workers=8,
+ persistent_workers=True,
+ sampler=dict(type='DefaultSampler', shuffle=False),
+ dataset=dict(
+ type=dataset_type,
+ ann_file=ann_file_test,
+ data_prefix=dict(video=data_root_val),
+ pipeline=test_pipeline,
+ test_mode=True))
+
+train_cfg = dict(val_interval=20)
+
+test_evaluator = dict(
+ type='ANetMetric',
+ metric_type='AR@AN',
+ dump_config=dict(out=f'{work_dir}/results.json', output_format='json'))
+val_evaluator = test_evaluator
diff --git a/configs/localization/bsn/bsn_pgm_2048x100_activitynet-slowonly-k700-feature.py b/configs/localization/bsn/bsn_pgm_2048x100_activitynet-slowonly-k700-feature.py
new file mode 100644
index 0000000000..544bc12a2e
--- /dev/null
+++ b/configs/localization/bsn/bsn_pgm_2048x100_activitynet-slowonly-k700-feature.py
@@ -0,0 +1,32 @@
+# dataset settings
+dataset_type = 'ActivityNetDataset'
+data_root = 'data/ActivityNet/k700slowonly'
+data_root_val = 'data/ActivityNet/k700slowonly'
+ann_file_train = 'data/ActivityNet/anet_anno_train.json'
+ann_file_val = 'data/ActivityNet/anet_anno_val.json'
+ann_file_test = 'data/ActivityNet/anet_anno_test.json'
+
+work_dir = 'work_dirs/bsn_400x100_20e_1x16_activitynet_feature/'
+tem_results_dir = f'{work_dir}/tem_results/'
+pgm_proposals_dir = f'{work_dir}/pgm_proposals/'
+pgm_features_dir = f'{work_dir}/pgm_features/'
+
+temporal_scale = 100
+pgm_proposals_cfg = dict(
+ pgm_proposals_thread=8, temporal_scale=temporal_scale, peak_threshold=0.5)
+pgm_features_test_cfg = dict(
+ pgm_features_thread=32,
+ top_k=1000,
+ num_sample_start=8,
+ num_sample_end=8,
+ num_sample_action=16,
+ num_sample_interp=3,
+ bsp_boundary_ratio=0.2)
+pgm_features_train_cfg = dict(
+ pgm_features_thread=32,
+ top_k=500,
+ num_sample_start=8,
+ num_sample_end=8,
+ num_sample_action=16,
+ num_sample_interp=3,
+ bsp_boundary_ratio=0.2)
diff --git a/configs/localization/bsn/bsn_tem_1xb16-2048x100-20e_activitynet-k700-feature.py b/configs/localization/bsn/bsn_tem_1xb16-2048x100-20e_activitynet-k700-feature.py
new file mode 100644
index 0000000000..c4e5821e81
--- /dev/null
+++ b/configs/localization/bsn/bsn_tem_1xb16-2048x100-20e_activitynet-k700-feature.py
@@ -0,0 +1,95 @@
+_base_ = ['../../_base_/models/bsn_tem.py', '../../_base_/default_runtime.py']
+
+model = dict(tem_feat_dim=2048)
+
+# dataset settings
+dataset_type = 'ActivityNetDataset'
+data_root = 'data/ActivityNet/k700slowonly'
+data_root_val = 'data/ActivityNet/k700slowonly'
+ann_file_train = 'data/ActivityNet/anet_anno_train.json'
+ann_file_val = 'data/ActivityNet/anet_anno_val.json'
+ann_file_test = 'data/ActivityNet/anet_anno_trainval.json'
+
+train_pipeline = [
+ dict(type='LoadLocalizationFeature'),
+ dict(type='GenerateLocalizationLabels'),
+ dict(
+ type='PackLocalizationInputs',
+ keys=('gt_bbox', ),
+ meta_keys=('video_name', ))
+]
+val_pipeline = [
+ dict(type='LoadLocalizationFeature'),
+ dict(type='GenerateLocalizationLabels'),
+ dict(
+ type='PackLocalizationInputs',
+ keys=('gt_bbox', ),
+ meta_keys=('video_name', ))
+]
+test_pipeline = [
+ dict(type='LoadLocalizationFeature'),
+ dict(type='PackLocalizationInputs', meta_keys=('video_name', ))
+]
+
+train_dataloader = dict(
+ batch_size=16,
+ num_workers=8,
+ persistent_workers=True,
+ sampler=dict(type='DefaultSampler', shuffle=True),
+ dataset=dict(
+ type=dataset_type,
+ ann_file=ann_file_train,
+ data_prefix=dict(video=data_root),
+ pipeline=train_pipeline))
+val_dataloader = dict(
+ batch_size=16,
+ num_workers=8,
+ persistent_workers=True,
+ sampler=dict(type='DefaultSampler', shuffle=False),
+ dataset=dict(
+ type=dataset_type,
+ ann_file=ann_file_val,
+ data_prefix=dict(video=data_root_val),
+ pipeline=val_pipeline,
+ test_mode=True))
+test_dataloader = dict(
+ batch_size=1,
+ num_workers=8,
+ persistent_workers=True,
+ sampler=dict(type='DefaultSampler', shuffle=False),
+ dataset=dict(
+ type=dataset_type,
+ ann_file=ann_file_test,
+ data_prefix=dict(video=data_root_val),
+ pipeline=test_pipeline,
+ test_mode=True))
+
+train_cfg = dict(
+ type='EpochBasedTrainLoop', max_epochs=20, val_begin=1, val_interval=20)
+val_cfg = dict(type='ValLoop')
+test_cfg = dict(type='TestLoop')
+
+optim_wrapper = dict(
+ optimizer=dict(type='Adam', lr=0.001, weight_decay=0.0001),
+ clip_grad=dict(max_norm=40, norm_type=2))
+
+param_scheduler = [
+ dict(
+ type='MultiStepLR',
+ begin=0,
+ end=20,
+ by_epoch=True,
+ milestones=[7, 14],
+ gamma=0.1)
+]
+
+work_dir = 'work_dirs/bsn_400x100_20e_1x16_activitynet_feature/'
+tem_results_dir = f'{work_dir}/tem_results/'
+
+test_evaluator = dict(
+ type='ANetMetric',
+ metric_type='TEM',
+ dump_config=dict(out=tem_results_dir, output_format='csv'))
+val_evaluator = test_evaluator
+
+default_hooks = dict(checkpoint=dict(filename_tmpl='tem_epoch_{}.pth'))
diff --git a/configs/localization/drn/README.md b/configs/localization/drn/README.md
new file mode 100644
index 0000000000..7eb5b3edda
--- /dev/null
+++ b/configs/localization/drn/README.md
@@ -0,0 +1,84 @@
+# DRN
+
+[Dense Regression Network for Video Grounding](https://openaccess.thecvf.com/content_CVPR_2020/papers/Zeng_Dense_Regression_Network_for_Video_Grounding_CVPR_2020_paper.pdf)
+
+
+
+## Abstract
+
+
+
+We address the problem of video grounding from natural language queries. The key challenge in this task is that one training video might only contain a few annotated starting/ending frames that can be used as positive examples for model training. Most conventional approaches directly train a binary classifier using such imbalance data, thus achieving inferior results. The key idea of this paper is to use the distances between the frame within the ground truth and the starting (ending) frame as dense supervisions to improve the video grounding accuracy. Specifically, we design a novel dense regression network (DRN) to regress the distances from each frame to the starting (ending) frame of the video segment described by the query. We also propose a simple but effective IoU regression head module to explicitly consider the localization quality of the grounding results (i.e., the IoU between the predicted location and the ground truth). Experimental results show that our approach significantly outperforms state-of-the-arts on three datasets (i.e., Charades-STA, ActivityNet-Captions, and TACoS).
+
+
+
+
+

+
+
+## Results and Models
+
+### Charades STA C3D feature
+
+| feature | gpus | pretrain | Recall@Top1(IoU=0.5) | Recall@Top5(IoU=0.5) | config | ckpt | log |
+| :-----: | :--: | :------: | :------------------: | :------------------: | :----------------------------------------------: | :---------------------------------------------: | :--------------------------------------------: |
+| C3D | 2 | None | 47.04 | 84.57 | [config](configs/localization/drn/drn_2xb16-4096-10e_c3d-feature_third.py) | [ckpt](https://download.openmmlab.com/mmaction/v1.0/localization/drn/drn_2xb16-4096-10e_c3d-feature_20230809-ec0429a6.pth) | [log](https://download.openmmlab.com/mmaction/v1.0/drn_2xb16-4096-10e_c3d-feature.log) |
+
+For more details on data preparation, you can refer to [Charades STA Data Preparation](/tools/data/charades-sta/README.md).
+
+## Train
+
+The training of DRN has three stages. Following the official paper, the second and the third stage loads the best checkpoint from previous stage.
+
+The first stage training:
+
+```shell
+bash tools/dist_train.sh configs/localization/drn/drn_2xb16-4096-10e_c3d-feature_first.py 2
+```
+
+The second stage training:
+
+```shell
+BEST_CKPT=work_dirs/drn_2xb16-4096-10e_c3d-feature_first/SOME.PTH
+bash tools/dist_train.sh configs/localization/drn/drn_2xb16-4096-10e_c3d-feature_second.py 2 --cfg-options load_from=${BEST_CKPT}
+```
+
+The third stage training:
+
+```shell
+BEST_CKPT=work_dirs/drn_2xb16-4096-10e_c3d-feature_second/SOME.PTH
+bash tools/dist_train.sh configs/localization/drn/drn_2xb16-4096-10e_c3d-feature_third.py 2 --cfg-options load_from=${BEST_CKPT}
+```
+
+## Test
+
+Test DRN on Charades STA C3D feature:
+
+```shell
+python3 tools/test.py configs/localization/drn/drn_2xb16-4096-10e_c3d-feature_third.py CHECKPOINT.PTH
+```
+
+For more details, you can refer to the **Testing** part in the [Training and Test Tutorial](/docs/en/user_guides/train_test.md).
+
+## Citation
+
+```BibTeX
+@inproceedings{DRN2020CVPR,
+ author = {Runhao, Zeng and Haoming, Xu and Wenbing, Huang and Peihao, Chen and Mingkui, Tan and Chuang Gan},
+ title = {Dense Regression Network for Video Grounding},
+ booktitle = {CVPR},
+ year = {2020},
+}
+```
+
+
+
+```BibTeX
+@inproceedings{gao2017tall,
+ title={Tall: Temporal activity localization via language query},
+ author={Gao, Jiyang and Sun, Chen and Yang, Zhenheng and Nevatia, Ram},
+ booktitle={Proceedings of the IEEE international conference on computer vision},
+ pages={5267--5275},
+ year={2017}
+}
+```
diff --git a/configs/localization/drn/drn_2xb16-4096-10e_c3d-feature_first.py b/configs/localization/drn/drn_2xb16-4096-10e_c3d-feature_first.py
new file mode 100644
index 0000000000..e66076e962
--- /dev/null
+++ b/configs/localization/drn/drn_2xb16-4096-10e_c3d-feature_first.py
@@ -0,0 +1,115 @@
+_base_ = ['../../_base_/default_runtime.py']
+
+# model settings
+model = dict(
+ type='DRN',
+ vocab_size=1301,
+ feature_dim=4096,
+ embed_dim=300,
+ hidden_dim=512,
+ bidirection=True,
+ first_output_dim=256,
+ fpn_feature_dim=512,
+ lstm_layers=1,
+ graph_node_features=1024,
+ fcos_pre_nms_top_n=32,
+ fcos_inference_thr=0.05,
+ fcos_prior_prob=0.01,
+ focal_alpha=0.25,
+ focal_gamma=2.0,
+ fpn_stride=[1, 2, 4],
+ fcos_nms_thr=0.6,
+ fcos_conv_layers=1,
+ fcos_num_class=2,
+ is_first_stage=True,
+ is_second_stage=False)
+
+# dataset settings
+dataset_type = 'CharadesSTADataset'
+root = 'data/CharadesSTA'
+data_root = f'{root}/C3D_unit16_overlap0.5_merged/'
+data_root_val = f'{root}/C3D_unit16_overlap0.5_merged/'
+ann_file_train = f'{root}/Charades_sta_train.txt'
+ann_file_val = f'{root}/Charades_sta_test.txt'
+ann_file_test = f'{root}/Charades_sta_test.txt'
+
+word2id_file = f'{root}/Charades_word2id.json'
+fps_file = f'{root}/Charades_fps_dict.json'
+duration_file = f'{root}/Charades_duration.json'
+num_frames_file = f'{root}/Charades_frames_info.json'
+window_size = 16
+ft_overlap = 0.5
+
+train_pipeline = [
+ dict(
+ type='PackLocalizationInputs',
+ keys=('gt_bbox', 'proposals'),
+ meta_keys=('vid_name', 'query_tokens', 'query_length', 'num_proposals',
+ 'num_frames'))
+]
+
+val_pipeline = train_pipeline
+test_pipeline = val_pipeline
+
+train_dataloader = dict(
+ batch_size=16,
+ num_workers=8,
+ persistent_workers=True,
+ sampler=dict(type='DefaultSampler', shuffle=True),
+ drop_last=True,
+ dataset=dict(
+ type=dataset_type,
+ ann_file=ann_file_train,
+ data_prefix=dict(video=data_root),
+ pipeline=train_pipeline,
+ word2id_file=word2id_file,
+ fps_file=fps_file,
+ duration_file=duration_file,
+ num_frames_file=num_frames_file,
+ window_size=window_size,
+ ft_overlap=ft_overlap),
+)
+
+val_dataloader = dict(
+ batch_size=1,
+ num_workers=4,
+ persistent_workers=True,
+ sampler=dict(type='DefaultSampler', shuffle=True),
+ drop_last=True,
+ dataset=dict(
+ type=dataset_type,
+ ann_file=ann_file_val,
+ data_prefix=dict(video=data_root),
+ pipeline=val_pipeline,
+ word2id_file=word2id_file,
+ fps_file=fps_file,
+ duration_file=duration_file,
+ num_frames_file=num_frames_file,
+ window_size=window_size,
+ ft_overlap=ft_overlap),
+)
+test_dataloader = val_dataloader
+
+max_epochs = 10
+train_cfg = dict(
+ type='EpochBasedTrainLoop',
+ max_epochs=max_epochs,
+ val_begin=1,
+ val_interval=1)
+
+val_cfg = dict(type='ValLoop')
+test_cfg = dict(type='TestLoop')
+
+val_evaluator = dict(type='RecallatTopK', topK_list=(1, 5), threshold=0.5)
+test_evaluator = val_evaluator
+
+optim_wrapper = dict(
+ optimizer=dict(type='Adam', lr=1e-3),
+ clip_grad=dict(max_norm=5, norm_type=2),
+)
+
+param_scheduler = [
+ dict(type='LinearLR', start_factor=0.1, by_epoch=True, begin=0, end=5),
+]
+
+find_unused_parameters = True
diff --git a/configs/localization/drn/drn_2xb16-4096-10e_c3d-feature_second.py b/configs/localization/drn/drn_2xb16-4096-10e_c3d-feature_second.py
new file mode 100644
index 0000000000..46a671db4c
--- /dev/null
+++ b/configs/localization/drn/drn_2xb16-4096-10e_c3d-feature_second.py
@@ -0,0 +1,110 @@
+_base_ = ['../../_base_/default_runtime.py']
+
+# model settings
+model = dict(
+ type='DRN',
+ vocab_size=1301,
+ feature_dim=4096,
+ embed_dim=300,
+ hidden_dim=512,
+ bidirection=True,
+ first_output_dim=256,
+ fpn_feature_dim=512,
+ lstm_layers=1,
+ graph_node_features=1024,
+ fcos_pre_nms_top_n=32,
+ fcos_inference_thr=0.05,
+ fcos_prior_prob=0.01,
+ focal_alpha=0.25,
+ focal_gamma=2.0,
+ fpn_stride=[1, 2, 4],
+ fcos_nms_thr=0.6,
+ fcos_conv_layers=1,
+ fcos_num_class=2,
+ is_first_stage=False,
+ is_second_stage=True)
+
+# dataset settings
+dataset_type = 'CharadesSTADataset'
+root = 'data/CharadesSTA'
+data_root = f'{root}/C3D_unit16_overlap0.5_merged/'
+data_root_val = f'{root}/C3D_unit16_overlap0.5_merged/'
+ann_file_train = f'{root}/Charades_sta_train.txt'
+ann_file_val = f'{root}/Charades_sta_test.txt'
+ann_file_test = f'{root}/Charades_sta_test.txt'
+
+word2id_file = f'{root}/Charades_word2id.json'
+fps_file = f'{root}/Charades_fps_dict.json'
+duration_file = f'{root}/Charades_duration.json'
+num_frames_file = f'{root}/Charades_frames_info.json'
+window_size = 16
+ft_overlap = 0.5
+
+train_pipeline = [
+ dict(
+ type='PackLocalizationInputs',
+ keys=('gt_bbox', 'proposals'),
+ meta_keys=('vid_name', 'query_tokens', 'query_length', 'num_proposals',
+ 'num_frames'))
+]
+
+val_pipeline = train_pipeline
+test_pipeline = val_pipeline
+
+train_dataloader = dict(
+ batch_size=16,
+ num_workers=8,
+ persistent_workers=True,
+ sampler=dict(type='DefaultSampler', shuffle=True),
+ drop_last=True,
+ dataset=dict(
+ type=dataset_type,
+ ann_file=ann_file_train,
+ data_prefix=dict(video=data_root),
+ pipeline=train_pipeline,
+ word2id_file=word2id_file,
+ fps_file=fps_file,
+ duration_file=duration_file,
+ num_frames_file=num_frames_file,
+ window_size=window_size,
+ ft_overlap=ft_overlap),
+)
+
+val_dataloader = dict(
+ batch_size=1,
+ num_workers=4,
+ persistent_workers=True,
+ sampler=dict(type='DefaultSampler', shuffle=True),
+ drop_last=True,
+ dataset=dict(
+ type=dataset_type,
+ ann_file=ann_file_val,
+ data_prefix=dict(video=data_root),
+ pipeline=val_pipeline,
+ word2id_file=word2id_file,
+ fps_file=fps_file,
+ duration_file=duration_file,
+ num_frames_file=num_frames_file,
+ window_size=window_size,
+ ft_overlap=ft_overlap),
+)
+test_dataloader = val_dataloader
+
+max_epochs = 10
+train_cfg = dict(
+ type='EpochBasedTrainLoop',
+ max_epochs=max_epochs,
+ val_begin=1,
+ val_interval=1)
+
+val_cfg = dict(type='ValLoop')
+test_cfg = dict(type='TestLoop')
+
+val_evaluator = dict(type='RecallatTopK', topK_list=(1, 5), threshold=0.5)
+test_evaluator = val_evaluator
+
+optim_wrapper = dict(
+ optimizer=dict(type='Adam', lr=1e-5),
+ clip_grad=dict(max_norm=5, norm_type=2))
+
+find_unused_parameters = True
diff --git a/configs/localization/drn/drn_2xb16-4096-10e_c3d-feature_third.py b/configs/localization/drn/drn_2xb16-4096-10e_c3d-feature_third.py
new file mode 100644
index 0000000000..2a286415bc
--- /dev/null
+++ b/configs/localization/drn/drn_2xb16-4096-10e_c3d-feature_third.py
@@ -0,0 +1,110 @@
+_base_ = ['../../_base_/default_runtime.py']
+
+# model settings
+model = dict(
+ type='DRN',
+ vocab_size=1301,
+ feature_dim=4096,
+ embed_dim=300,
+ hidden_dim=512,
+ bidirection=True,
+ first_output_dim=256,
+ fpn_feature_dim=512,
+ lstm_layers=1,
+ graph_node_features=1024,
+ fcos_pre_nms_top_n=32,
+ fcos_inference_thr=0.05,
+ fcos_prior_prob=0.01,
+ focal_alpha=0.25,
+ focal_gamma=2.0,
+ fpn_stride=[1, 2, 4],
+ fcos_nms_thr=0.6,
+ fcos_conv_layers=1,
+ fcos_num_class=2,
+ is_first_stage=False,
+ is_second_stage=False)
+
+# dataset settings
+dataset_type = 'CharadesSTADataset'
+root = 'data/CharadesSTA'
+data_root = f'{root}/C3D_unit16_overlap0.5_merged/'
+data_root_val = f'{root}/C3D_unit16_overlap0.5_merged/'
+ann_file_train = f'{root}/Charades_sta_train.txt'
+ann_file_val = f'{root}/Charades_sta_test.txt'
+ann_file_test = f'{root}/Charades_sta_test.txt'
+
+word2id_file = f'{root}/Charades_word2id.json'
+fps_file = f'{root}/Charades_fps_dict.json'
+duration_file = f'{root}/Charades_duration.json'
+num_frames_file = f'{root}/Charades_frames_info.json'
+window_size = 16
+ft_overlap = 0.5
+
+train_pipeline = [
+ dict(
+ type='PackLocalizationInputs',
+ keys=('gt_bbox', 'proposals'),
+ meta_keys=('vid_name', 'query_tokens', 'query_length', 'num_proposals',
+ 'num_frames'))
+]
+
+val_pipeline = train_pipeline
+test_pipeline = val_pipeline
+
+train_dataloader = dict(
+ batch_size=16,
+ num_workers=8,
+ persistent_workers=True,
+ sampler=dict(type='DefaultSampler', shuffle=True),
+ drop_last=True,
+ dataset=dict(
+ type=dataset_type,
+ ann_file=ann_file_train,
+ data_prefix=dict(video=data_root),
+ pipeline=train_pipeline,
+ word2id_file=word2id_file,
+ fps_file=fps_file,
+ duration_file=duration_file,
+ num_frames_file=num_frames_file,
+ window_size=window_size,
+ ft_overlap=ft_overlap),
+)
+
+val_dataloader = dict(
+ batch_size=1,
+ num_workers=4,
+ persistent_workers=True,
+ sampler=dict(type='DefaultSampler', shuffle=True),
+ drop_last=True,
+ dataset=dict(
+ type=dataset_type,
+ ann_file=ann_file_val,
+ data_prefix=dict(video=data_root),
+ pipeline=val_pipeline,
+ word2id_file=word2id_file,
+ fps_file=fps_file,
+ duration_file=duration_file,
+ num_frames_file=num_frames_file,
+ window_size=window_size,
+ ft_overlap=ft_overlap),
+)
+test_dataloader = val_dataloader
+
+max_epochs = 10
+train_cfg = dict(
+ type='EpochBasedTrainLoop',
+ max_epochs=max_epochs,
+ val_begin=1,
+ val_interval=1)
+
+val_cfg = dict(type='ValLoop')
+test_cfg = dict(type='TestLoop')
+
+val_evaluator = dict(type='RecallatTopK', topK_list=(1, 5), threshold=0.5)
+test_evaluator = val_evaluator
+
+optim_wrapper = dict(
+ optimizer=dict(type='Adam', lr=1e-6),
+ clip_grad=dict(max_norm=5, norm_type=2))
+
+find_unused_parameters = True
diff --git a/configs/localization/drn/metafile.yml b/configs/localization/drn/metafile.yml
new file mode 100644
index 0000000000..d092668b1e
--- /dev/null
+++ b/configs/localization/drn/metafile.yml
@@ -0,0 +1,26 @@
+Collections:
+- Name: DRN
+ README: configs/localization/drn/README.md
+ Paper:
+ URL: https://openaccess.thecvf.com/content_CVPR_2020/papers/Zeng_Dense_Regression_Network_for_Video_Grounding_CVPR_2020_paper.pdf
+ Title: "Dense Regression Network for Video Grounding"
+
+Models:
+ - Name: drn_2xb16-4096-10e_c3d-feature_third
+ Config: configs/localization/drn/drn_2xb16-4096-10e_c3d-feature_third.py
+ In Collection: DRN
+ Metadata:
+ Batch Size: 16
+ Epochs: 10
+ Training Data: Charades STA
+ Training Resources: 2 GPUs
+ feature: C3D
+ Modality: RGB
+ Results:
+ - Dataset: Charades STA
+ Task: Video Grounding
+ Metrics:
+ Recall@Top1(IoU=0.5): 47.04
+ Recall@Top5(IoU=0.5): 84.57
+ Training Log: https://download.openmmlab.com/mmaction/v1.0/drn_2xb16-4096-10e_c3d-feature.log
+ Weights: https://download.openmmlab.com/mmaction/v1.0/localization/drn/drn_2xb16-4096-10e_c3d-feature_20230809-ec0429a6.pth
diff --git a/configs/multimodal/vindlu/README.md b/configs/multimodal/vindlu/README.md
new file mode 100644
index 0000000000..c49fed61fa
--- /dev/null
+++ b/configs/multimodal/vindlu/README.md
@@ -0,0 +1,87 @@
+# VindLU
+
+[VindLU: A Recipe for Effective Video-and-Language Pretraining](https://arxiv.org/abs/2212.05051)
+
+
+
+## Abstract
+
+
+
+The last several years have witnessed remarkable progress in video-and-language (VidL) understanding. However, most modern VidL approaches use complex and specialized model architectures and sophisticated pretraining protocols, making the reproducibility, analysis and comparisons of these frameworks difficult. Hence, instead of proposing yet another new VidL model, this paper conducts a thorough empirical study demystifying the most important factors in the VidL model design. Among the factors that we investigate are (i) the spatiotemporal architecture design, (ii) the multimodal fusion schemes, (iii) the pretraining objectives, (iv) the choice of pretraining data, (v) pretraining and finetuning protocols, and (vi) dataset and model scaling. Our empirical study reveals that the most important design factors include: temporal modeling, video-to-text multimodal fusion, masked modeling objectives, and joint training on images and videos. Using these empirical insights, we then develop a step-by-step recipe, dubbed VindLU, for effective VidL pretraining. Our final model trained using our recipe achieves comparable or better than state-of-the-art results on several VidL tasks without relying on external CLIP pretraining. In particular, on the text-to-video retrieval task, our approach obtains 61.2% on DiDeMo, and 55.0% on ActivityNet, outperforming current SOTA by 7.8% and 6.1% respectively. Furthermore, our model also obtains state-of-the-art video question-answering results on ActivityNet-QA, MSRVTT-QA, MSRVTT-MC and TVQA. Our code and pretrained models are publicly available at: https://github.com/klauscc/VindLU.
+
+
+
+
+

+
+
+## Results and Models
+
+### Video Retrieval on MSRVTT-9k
+
+| frame sampling strategy | resolution | gpus | vision encoder | text encoder | pretraining | Recall@1 | config | ckpt | log |
+| :---------------------: | :--------: | :--: | :------------: | :----------: | :--------------------: | :------: | :-----------------------------------: | :---------------------------------: | :---------------------------------: |
+| uniform 12 | 224x224 | 8 | BEiT-Base | Bert-Base | C5M (WebVid-2M + CC3M) | 44.0 | [config](/configs/multimodal/vindlu/vindlu_beit-base_8x16_retrieval_msrvtt-9k.py) | [ckpt](https://download.openmmlab.com/mmaction/v1.0/multimodal/vindlu/vindlu_beit-base_8x16_retrieval_msrvtt-9k/vindlu_beit-base_8x16_retrieval_msrvtt-9k_20230905-fc36231e.pth) | [log](https://download.openmmlab.com/mmaction/v1.0/multimodal/vindlu/vindlu_beit-base_8x16_retrieval_msrvtt-9k/vindlu_beit-base_8x16_retrieval_msrvtt-9k.log) |
+
+### Video Question-Answering on MSRVTT-QA
+
+| frame sampling strategy | resolution | gpus | vision encoder | text encoder | pretraining | top1 acc | config | ckpt | log |
+| :---------------------: | :--------: | :--: | :------------: | :----------: | :--------------------: | :------: | :-----------------------------------: | :---------------------------------: | :---------------------------------: |
+| uniform 12 | 224x224 | 8 | BEiT-Base | Bert-Base | C5M (WebVid-2M + CC3M) | 43.6 | [config](/configs/multimodal/vindlu/vindlu_beit-base_8x8_vqa_msrvtt-qa.py) | [ckpt](https://download.openmmlab.com/mmaction/v1.0/multimodal/vindlu/vindlu_beit-base_8x8_vqa_msrvtt-qa/vindlu_beit-base_8x8_vqa_msrvtt-qa_20230906-6e693e64.pth) | [log](https://download.openmmlab.com/mmaction/v1.0/multimodal/vindlu/vindlu_beit-base_8x8_vqa_msrvtt-qa/vindlu_beit-base_8x8_vqa_msrvtt-qa.log) |
+
+### Multiple-Choice Question-Answering on MSRVTT-MC (Inference)
+
+| frame sampling strategy | resolution | gpus | vision encoder | text encoder | pretraining | top1 acc | config | ckpt |
+| :---------------------: | :--------: | :--: | :------------: | :----------: | :--------------------: | :------: | :----------------------------------------------------: | :---------------------------------------------------: |
+| uniform 12 | 224x224 | 8 | BEiT-Base | Bert-Base | C5M (WebVid-2M + CC3M) | 97.6 | [config](/configs/multimodal/vindlu/vindlu_beit-base_vqa-mc_msrvtt-mc.py) | [ckpt](https://download.openmmlab.com/mmaction/v1.0/multimodal/vindlu/vindlu_beit-base_8x16_retrieval_msrvtt-9k/vindlu_beit-base_8x16_retrieval_msrvtt-9k_20230905-fc36231e.pth) |
+
+1. Currently, we only support the fine-tuning stage of VindLU models based on the pretrained checkpoint provided by the [original repo](https://github.com/klauscc/VindLU).
+
+For more details on data preparation, you can refer to [prepare msrvtt](/tools/data/msrvtt/README.md).
+
+## Train
+
+You can use the following command to train a model.
+
+```shell
+python tools/train.py ${CONFIG_FILE} [optional arguments]
+```
+
+Example: train VindLU model on MSRVTT-9k dataset in a deterministic option with periodic validation.
+
+```shell
+python tools/train.py configs/multimodal/vindlu/vindlu_beit-base_8x16_retrieval_msrvtt-9k.py \
+ --seed 0 --deterministic
+```
+
+For more details, you can refer to the **Training** part in the [Training and Test Tutorial](/docs/en/user_guides/train_test.md).
+
+## Test
+
+You can use the following command to test a model.
+
+```shell
+python tools/test.py ${CONFIG_FILE} ${CHECKPOINT_FILE} [optional arguments]
+```
+
+Example: test CLIP4Clip model on MSRVTT-9k dataset and dump the result to a pkl file.
+
+```shell
+python tools/test.py cconfigs/multimodal/vindlu/vindlu_beit-base_8x16_retrieval_msrvtt-9k.py \
+ checkpoints/SOME_CHECKPOINT.pth --dump result.pkl
+```
+
+For more details, you can refer to the **Test** part in the [Training and Test Tutorial](/docs/en/user_guides/train_test.md).
+
+## Citation
+
+```BibTeX
+@inproceedings{cheng2023vindlu,
+ title={Vindlu: A recipe for effective video-and-language pretraining},
+ author={Cheng, Feng and Wang, Xizi and Lei, Jie and Crandall, David and Bansal, Mohit and Bertasius, Gedas},
+ booktitle={Proceedings of the IEEE/CVF Conference on Computer Vision and Pattern Recognition},
+ pages={10739--10750},
+ year={2023}
+}
+```
diff --git a/configs/multimodal/vindlu/metafile.yml b/configs/multimodal/vindlu/metafile.yml
new file mode 100644
index 0000000000..d7fdf7fe24
--- /dev/null
+++ b/configs/multimodal/vindlu/metafile.yml
@@ -0,0 +1,55 @@
+Collections:
+ - Name: VindLU
+ README: configs/multimodal/vindlu/README.md
+ Paper:
+ URL: https://arxiv.org/abs/2212.05051
+ Title: 'VindLU: A Recipe for Effective Video-and-Language Pretraining'
+
+Models:
+ - Name: vindlu_beit-base_8x16_retrieval_msrvtt-9k
+ Config: configs/multimodal/vindlu/vindlu_beit-base_8x16_retrieval_msrvtt-9k.py
+ In Collection: VindLU
+ Metadata:
+ Architecture: BEiT-Base
+ Batch Size: 16
+ Epochs: 5
+ Training Data: MSRVTT-9k
+ Training Resources: 8 GPUs
+ Results:
+ Dataset: MSRVTT
+ Task: Video Retrieval
+ Metrics:
+ Recall@1: 44.0
+ Recall@5: 70.6
+ Recall@10: 80.0
+ Training Log: https://download.openmmlab.com/mmaction/v1.0/multimodal/vindlu/vindlu_beit-base_8x16_retrieval_msrvtt-9k/vindlu_beit-base_8x16_retrieval_msrvtt-9k.log
+ Weights: https://download.openmmlab.com/mmaction/v1.0/multimodal/vindlu/vindlu_beit-base_8x16_retrieval_msrvtt-9k/vindlu_beit-base_8x16_retrieval_msrvtt-9k_20230905-fc36231e.pth
+
+ - Name: vindlu_beit-base_8x8_vqa_msrvtt-qa
+ Config: configs/multimodal/vindlu/vindlu_beit-base_8x8_vqa_msrvtt-qa.py
+ In Collection: VindLU
+ Metadata:
+ Architecture: BEiT-Base
+ Batch Size: 8
+ Epochs: 10
+ Training Data: MSRVTT-qa
+ Training Resources: 8 GPUs
+ Results:
+ Dataset: MSRVTT
+ Task: Video Question-Answering
+ Metrics:
+ Top 1 Accuracy: 43.6
+ Training Log: https://download.openmmlab.com/mmaction/v1.0/multimodal/vindlu/vindlu_beit-base_8x8_vqa_msrvtt-qa/vindlu_beit-base_8x8_vqa_msrvtt-qa.log
+ Weights: https://download.openmmlab.com/mmaction/v1.0/multimodal/vindlu/vindlu_beit-base_8x8_vqa_msrvtt-qa/vindlu_beit-base_8x8_vqa_msrvtt-qa_20230906-6e693e64.pth
+
+ - Name: vindlu_beit-base_vqa-mc_msrvtt-mc
+ Config: configs/multimodal/vindlu/vindlu_beit-base_vqa-mc_msrvtt-mc.py
+ In Collection: VindLU
+ Metadata:
+ Architecture: BEiT-Base
+ Results:
+ Dataset: MSRVTT-MC
+ Task: Multiple-Choice Question-Answering
+ Metrics:
+ Top 1 Accuracy: 97.6
+ Weights: https://download.openmmlab.com/mmaction/v1.0/multimodal/vindlu/vindlu_beit-base_8x16_retrieval_msrvtt-9k/vindlu_beit-base_8x16_retrieval_msrvtt-9k_20230905-fc36231e.pth
diff --git a/configs/multimodal/vindlu/vindlu_beit-base_8x16_retrieval_msrvtt-9k.py b/configs/multimodal/vindlu/vindlu_beit-base_8x16_retrieval_msrvtt-9k.py
new file mode 100644
index 0000000000..fd20acbc24
--- /dev/null
+++ b/configs/multimodal/vindlu/vindlu_beit-base_8x16_retrieval_msrvtt-9k.py
@@ -0,0 +1,200 @@
+_base_ = ['../../_base_/default_runtime.py']
+
+video_root = 'data/msrvtt/videos_2fps_224'
+anno_file_train = 'data/msrvtt/annotations/msrvtt_ret_train9k.json'
+anno_file_test = 'data/msrvtt/annotations/msrvtt_ret_test1k.json'
+pretrained_ckpt_url = 'https://download.openmmlab.com/mmaction/v1.0/multimodal/vindlu/vindlu_c5m_pretrain.pth' # noqa: E501
+
+# model settings
+model = dict(
+ type='VindLURetrieval',
+ gradient_checkpointing=True,
+ init_cfg=dict(type='Pretrained', checkpoint=pretrained_ckpt_url),
+ data_preprocessor=dict(
+ type='ActionDataPreprocessor',
+ mean=[128],
+ std=[128],
+ format_shape='NCTHW'),
+ tokenizer=dict(
+ type='VindLUTokenizer',
+ pretrained_model_name_or_path='bert-base-uncased'),
+ vision_encoder=dict(
+ type='BeitModel3D',
+ config='microsoft/beit-base-patch16-224-pt22k-ft22k',
+ tem_config=dict(
+ num_frames=12,
+ temporal_model_block='timesformer',
+ temporal_model_position='last',
+ temporal_model_config=dict(input_dim=768),
+ use_temporal_position_embedding=True),
+ encoder_width=768,
+ add_ln=True),
+ text_encoder=dict(
+ type='XBertModel',
+ pretrained_model_name_or_path='bert-base-uncased',
+ encoder_width=768,
+ fusion_layer=9,
+ add_pooling_layer=False),
+ proj_dim=256,
+ temperature=0.07,
+ max_txt_len=32,
+ topk=128)
+
+file_client_args = dict(io_backend='disk')
+train_pipeline = [
+ dict(type='DecordInit', **file_client_args),
+ dict(
+ type='SampleFrames',
+ clip_len=1,
+ frame_interval=1,
+ num_clips=12,
+ out_of_bound_opt='repeat_last',
+ ),
+ dict(type='DecordDecode'),
+ dict(type='RandomResizedCrop', area_range=(0.5, 1.0)),
+ dict(
+ type='Resize',
+ scale=(224, 224),
+ keep_ratio=False,
+ interpolation='bicubic'),
+ dict(type='Flip', flip_ratio=0.5),
+ dict(type='FormatShape', input_format='NCHW'),
+ dict(
+ type='PackActionInputs',
+ algorithm_keys=(
+ 'text',
+ 'gt_video_id',
+ 'gt_text_id',
+ ))
+]
+
+val_pipeline = [
+ dict(type='DecordInit', **file_client_args),
+ dict(
+ type='SampleFrames',
+ clip_len=1,
+ frame_interval=1,
+ num_clips=12,
+ test_mode=True,
+ out_of_bound_opt='repeat_last'),
+ dict(type='DecordDecode'),
+ dict(
+ type='Resize',
+ scale=(224, 224),
+ keep_ratio=False,
+ interpolation='bicubic'),
+ dict(type='FormatShape', input_format='NCHW'),
+ dict(
+ type='PackActionInputs',
+ algorithm_keys=(
+ 'text',
+ 'gt_video_id',
+ 'gt_text_id',
+ ))
+]
+
+test_pipeline = [
+ dict(type='DecordInit', **file_client_args),
+ dict(
+ type='SampleFrames',
+ clip_len=1,
+ frame_interval=1,
+ num_clips=12,
+ test_mode=True,
+ out_of_bound_opt='repeat_last'),
+ dict(type='DecordDecode'),
+ dict(
+ type='Resize',
+ scale=(224, 224),
+ keep_ratio=False,
+ interpolation='bicubic'),
+ dict(type='FormatShape', input_format='NCHW'),
+ dict(
+ type='PackActionInputs',
+ algorithm_keys=(
+ 'text',
+ 'gt_video_id',
+ 'gt_text_id',
+ ))
+]
+
+dataset_type = 'MSRVTTRetrieval'
+
+train_dataloader = dict(
+ batch_size=32,
+ num_workers=8,
+ persistent_workers=True,
+ sampler=dict(type='DefaultSampler', shuffle=True),
+ dataset=dict(
+ type=dataset_type,
+ ann_file=anno_file_train,
+ pipeline=train_pipeline,
+ data_prefix=dict(video=video_root),
+ ))
+
+val_dataloader = dict(
+ batch_size=8,
+ num_workers=8,
+ persistent_workers=True,
+ sampler=dict(type='DefaultSampler', shuffle=False),
+ dataset=dict(
+ type=dataset_type,
+ ann_file=anno_file_test,
+ pipeline=test_pipeline,
+ data_prefix=dict(video=video_root),
+ ))
+
+test_dataloader = dict(
+ batch_size=8,
+ num_workers=8,
+ persistent_workers=True,
+ sampler=dict(type='DefaultSampler', shuffle=False),
+ dataset=dict(
+ type=dataset_type,
+ ann_file=anno_file_test,
+ pipeline=test_pipeline,
+ data_prefix=dict(video=video_root),
+ ))
+
+train_cfg = dict(
+ type='EpochBasedTrainLoop', max_epochs=5, val_begin=1, val_interval=1)
+val_cfg = dict(type='RetrievalValLoop')
+test_cfg = dict(type='RetrievalTestLoop')
+
+val_evaluator = dict(type='RetrievalRecall', topk=(1, 5, 10))
+test_evaluator = dict(type='RetrievalRecall', topk=(1, 5, 10))
+
+param_scheduler = [
+ dict(
+ type='CosineAnnealingLR',
+ T_max=5,
+ eta_min_ratio=0.01,
+ by_epoch=True,
+ begin=0,
+ end=5,
+ convert_to_iter_based=True)
+]
+
+optim_wrapper = dict(
+ type='AmpOptimWrapper',
+ optimizer=dict(type='AdamW', lr=1e-5, weight_decay=0.02),
+ paramwise_cfg=dict(
+ bypass_duplicate=True, norm_decay_mult=0.0, bias_decay_mult=0.0),
+ clip_grad=dict(max_norm=50, norm_type=2),
+)
+
+model_wrapper_cfg = dict(type='MMDistributedDataParallel', static_graph=True)
+
+default_hooks = dict(
+ checkpoint=dict(
+ type='CheckpointHook',
+ interval=1,
+ save_best='t2i/retrieval/Recall@1',
+ rule='greater'),
+ logger=dict(type='LoggerHook', interval=20, ignore_last=False))
+
+auto_scale_lr = dict(enable=True, base_batch_size=128)
+
+find_unused_parameters = True
+
+custom_hooks = [dict(type='EmptyCacheHook', after_epoch=True)]
diff --git a/configs/multimodal/vindlu/vindlu_beit-base_8x8_vqa_msrvtt-qa.py b/configs/multimodal/vindlu/vindlu_beit-base_8x8_vqa_msrvtt-qa.py
new file mode 100644
index 0000000000..461b045cdb
--- /dev/null
+++ b/configs/multimodal/vindlu/vindlu_beit-base_8x8_vqa_msrvtt-qa.py
@@ -0,0 +1,190 @@
+_base_ = ['../../_base_/default_runtime.py']
+
+video_root = 'data/msrvtt/videos_2fps_224'
+anno_file_train = 'data/msrvtt/annotations/msrvtt_qa_train.json'
+anno_file_val = 'data/msrvtt/annotations/msrvtt_qa_val.json'
+anno_file_test = 'data/msrvtt/annotations/msrvtt_qa_test.json'
+answer_list_file = 'data/msrvtt/annotations/msrvtt_qa_answer_list.json'
+pretrained_ckpt_url = 'https://download.openmmlab.com/mmaction/v1.0/multimodal/vindlu/vindlu_c5m_pretrain.pth' # noqa: E501
+
+# model settings
+model = dict(
+ type='VindLUVQA',
+ init_cfg=dict(type='Pretrained', checkpoint=pretrained_ckpt_url),
+ data_preprocessor=dict(
+ type='ActionDataPreprocessor',
+ mean=[128],
+ std=[128],
+ format_shape='NCTHW'),
+ tokenizer=dict(
+ type='VindLUTokenizer',
+ pretrained_model_name_or_path='bert-base-uncased',
+ ),
+ vision_encoder=dict(
+ type='BeitModel3D',
+ config='microsoft/beit-base-patch16-224-pt22k-ft22k',
+ tem_config=dict(
+ num_frames=12,
+ temporal_model_block='timesformer',
+ temporal_model_position='last',
+ temporal_model_config=dict(input_dim=768),
+ use_temporal_position_embedding=True),
+ encoder_width=768,
+ add_ln=True),
+ text_encoder=dict(
+ type='XBertModel',
+ pretrained_model_name_or_path='bert-base-uncased',
+ encoder_width=768,
+ fusion_layer=9,
+ add_pooling_layer=False),
+ text_decoder=dict(
+ type='BertDecoder',
+ pretrained_model_name_or_path='bert-base-uncased',
+ encoder_width=768,
+ fusion_layer=0,
+ num_hidden_layers=3,
+ add_pooling_layer=True),
+ proj_dim=256,
+ temperature=0.07,
+ max_question_len=25,
+ max_answer_len=5,
+ num_ans_candidates=128,
+ gradient_checkpointing=True,
+ answer_list_path=answer_list_file)
+
+file_client_args = dict(io_backend='disk')
+
+train_pipeline = [
+ dict(type='DecordInit', **file_client_args),
+ dict(
+ type='SampleFrames',
+ clip_len=1,
+ frame_interval=1,
+ num_clips=12,
+ out_of_bound_opt='repeat_last'),
+ dict(type='DecordDecode'),
+ dict(type='RandomResizedCrop', area_range=(0.5, 1.0)),
+ dict(
+ type='Resize',
+ scale=(224, 224),
+ keep_ratio=False,
+ interpolation='bicubic'),
+ dict(type='Flip', flip_ratio=0.5),
+ dict(type='FormatShape', input_format='NCHW'),
+ dict(
+ type='PackActionInputs',
+ algorithm_keys=(
+ 'question',
+ 'question_id',
+ 'gt_answer',
+ 'gt_answer_weight',
+ ))
+]
+
+val_pipeline = [
+ dict(type='DecordInit', **file_client_args),
+ dict(
+ type='SampleFrames',
+ clip_len=1,
+ frame_interval=1,
+ num_clips=12,
+ test_mode=True,
+ out_of_bound_opt='repeat_last'),
+ dict(type='DecordDecode'),
+ dict(
+ type='Resize',
+ scale=(224, 224),
+ keep_ratio=False,
+ interpolation='bicubic'),
+ dict(type='FormatShape', input_format='NCHW'),
+ dict(
+ type='PackActionInputs',
+ algorithm_keys=(
+ 'question',
+ 'gt_answer',
+ 'question_id',
+ ))
+]
+
+test_pipeline = val_pipeline
+
+dataset_type = 'MSRVTTVQA'
+
+train_dataloader = dict(
+ batch_size=8,
+ num_workers=8,
+ persistent_workers=True,
+ sampler=dict(type='DefaultSampler', shuffle=True),
+ dataset=dict(
+ type=dataset_type,
+ ann_file=anno_file_train,
+ pipeline=train_pipeline,
+ data_prefix=dict(video=video_root),
+ ))
+
+val_dataloader = dict(
+ batch_size=16,
+ num_workers=4,
+ persistent_workers=True,
+ sampler=dict(type='DefaultSampler', shuffle=False),
+ dataset=dict(
+ type=dataset_type,
+ ann_file=anno_file_val,
+ pipeline=val_pipeline,
+ data_prefix=dict(video=video_root),
+ ))
+
+test_dataloader = dict(
+ batch_size=16,
+ num_workers=4,
+ persistent_workers=True,
+ sampler=dict(type='DefaultSampler', shuffle=False),
+ dataset=dict(
+ type=dataset_type,
+ ann_file=anno_file_test,
+ pipeline=test_pipeline,
+ data_prefix=dict(video=video_root),
+ ))
+
+val_evaluator = dict(type='VQAAcc')
+test_evaluator = dict(type='VQAAcc')
+
+train_cfg = dict(
+ type='EpochBasedTrainLoop', max_epochs=10, val_begin=1, val_interval=1)
+val_cfg = dict(type='ValLoop')
+test_cfg = dict(type='TestLoop')
+
+param_scheduler = [
+ dict(
+ type='LinearLR',
+ start_factor=0.01,
+ by_epoch=True,
+ begin=0,
+ end=1,
+ convert_to_iter_based=True),
+ dict(
+ type='CosineAnnealingLR',
+ T_max=10,
+ eta_min_ratio=0.01,
+ by_epoch=True,
+ begin=1,
+ end=10,
+ convert_to_iter_based=True)
+]
+
+optim_wrapper = dict(
+ type='AmpOptimWrapper',
+ optimizer=dict(type='AdamW', lr=1e-5, weight_decay=0.02),
+ paramwise_cfg=dict(
+ bypass_duplicate=True, norm_decay_mult=0.0, bias_decay_mult=0.0),
+ clip_grad=dict(max_norm=50, norm_type=2),
+)
+
+model_wrapper_cfg = dict(type='MMDistributedDataParallel', static_graph=True)
+
+default_hooks = dict(
+ logger=dict(type='LoggerHook', interval=20, ignore_last=False))
+
+auto_scale_lr = dict(enable=True, base_batch_size=32)
+
+find_unused_parameters = True
diff --git a/configs/multimodal/vindlu/vindlu_beit-base_vqa-mc_msrvtt-mc.py b/configs/multimodal/vindlu/vindlu_beit-base_vqa-mc_msrvtt-mc.py
new file mode 100644
index 0000000000..7ec0271928
--- /dev/null
+++ b/configs/multimodal/vindlu/vindlu_beit-base_vqa-mc_msrvtt-mc.py
@@ -0,0 +1,80 @@
+_base_ = ['../../_base_/default_runtime.py']
+
+video_root = 'data/msrvtt/videos_2fps_224'
+anno_file_test = 'data/msrvtt/annotations/msrvtt_mc_test.json'
+
+# model settings
+model = dict(
+ type='VindLURetrievalMC',
+ data_preprocessor=dict(
+ type='ActionDataPreprocessor',
+ mean=[128],
+ std=[128],
+ format_shape='NCTHW'),
+ tokenizer=dict(
+ type='VindLUTokenizer',
+ pretrained_model_name_or_path='bert-base-uncased'),
+ vision_encoder=dict(
+ type='BeitModel3D',
+ config='microsoft/beit-base-patch16-224-pt22k-ft22k',
+ tem_config=dict(
+ num_frames=12,
+ temporal_model_block='timesformer',
+ temporal_model_position='last',
+ temporal_model_config=dict(input_dim=768),
+ use_temporal_position_embedding=True),
+ encoder_width=768,
+ add_ln=True),
+ text_encoder=dict(
+ type='XBertModel',
+ pretrained_model_name_or_path='bert-base-uncased',
+ encoder_width=768,
+ fusion_layer=9,
+ add_pooling_layer=False),
+ text_decoder=dict(
+ type='BertDecoder',
+ pretrained_model_name_or_path='bert-base-uncased',
+ encoder_width=768,
+ fusion_layer=0,
+ num_hidden_layers=3,
+ add_pooling_layer=True),
+ proj_dim=256,
+ temperature=0.07,
+ max_txt_len=32,
+ gradient_checkpointing=True)
+
+file_client_args = dict(io_backend='disk')
+
+test_pipeline = [
+ dict(type='DecordInit', **file_client_args),
+ dict(
+ type='SampleFrames',
+ clip_len=1,
+ frame_interval=1,
+ num_clips=12,
+ test_mode=True),
+ dict(type='DecordDecode'),
+ dict(type='Resize', scale=(224, 224), keep_ratio=False),
+ dict(type='FormatShape', input_format='NCHW'),
+ dict(type='PackActionInputs', algorithm_keys=('caption_options', ))
+]
+
+dataset_type = 'MSRVTTVQAMC'
+
+test_dataloader = dict(
+ batch_size=32,
+ num_workers=16,
+ persistent_workers=True,
+ sampler=dict(type='DefaultSampler', shuffle=False),
+ dataset=dict(
+ type=dataset_type,
+ ann_file=anno_file_test,
+ pipeline=test_pipeline,
+ data_prefix=dict(video=video_root),
+ ))
+
+test_evaluator = dict(type='VQAMCACC')
+test_cfg = dict(type='TestLoop')
+
+default_hooks = dict(
+ logger=dict(type='LoggerHook', interval=20, ignore_last=False), )
diff --git a/configs/recognition/slowonly/slowonly_r50_8xb16-16x4x1-256e_kinetics400-flow.py b/configs/recognition/slowonly/slowonly_r50_8xb16-16x4x1-256e_kinetics400-flow.py
new file mode 100644
index 0000000000..92221d9e97
--- /dev/null
+++ b/configs/recognition/slowonly/slowonly_r50_8xb16-16x4x1-256e_kinetics400-flow.py
@@ -0,0 +1,146 @@
+_base_ = '../../_base_/default_runtime.py'
+
+model = dict(
+ type='Recognizer3D',
+ backbone=dict(
+ type='ResNet3dSlowOnly',
+ depth=50,
+ pretrained=None,
+ lateral=False,
+ in_channels=2,
+ conv1_kernel=(1, 7, 7),
+ conv1_stride_t=1,
+ pool1_stride_t=1,
+ inflate=(0, 0, 1, 1),
+ norm_eval=False),
+ cls_head=dict(
+ type='I3DHead',
+ in_channels=2048,
+ num_classes=400,
+ spatial_type='avg',
+ dropout_ratio=0.5,
+ average_clips='prob'),
+ data_preprocessor=dict(
+ type='ActionDataPreprocessor',
+ mean=[128, 128],
+ std=[128, 128],
+ format_shape='NCTHW'))
+
+# dataset settings
+dataset_type = 'RawframeDataset'
+data_root = 'data/kinetics400/rawframes_train'
+data_root_val = 'data/kinetics400/rawframes_val'
+ann_file_train = 'data/kinetics400/kinetics400_train_list_flow.txt'
+ann_file_val = 'data/kinetics400/kinetics400_val_list_flow.txt'
+ann_file_test = 'data/kinetics400/kinetics400_val_list_flow.txt'
+file_client_args = dict(io_backend='disk')
+train_pipeline = [
+ dict(type='SampleFrames', clip_len=16, frame_interval=4, num_clips=1),
+ dict(type='RawFrameDecode', **file_client_args),
+ dict(type='Resize', scale=(-1, 256)),
+ dict(type='RandomResizedCrop'),
+ dict(type='Resize', scale=(224, 224), keep_ratio=False),
+ dict(type='Flip', flip_ratio=0.5),
+ dict(type='FormatShape', input_format='NCTHW'),
+ dict(type='PackActionInputs')
+]
+
+val_pipeline = [
+ dict(
+ type='SampleFrames',
+ clip_len=16,
+ frame_interval=4,
+ num_clips=2,
+ test_mode=True),
+ dict(type='RawFrameDecode', **file_client_args),
+ dict(type='Resize', scale=(-1, 256)),
+ dict(type='CenterCrop', crop_size=224),
+ dict(type='FormatShape', input_format='NCTHW'),
+ dict(type='PackActionInputs')
+]
+
+test_pipeline = [
+ dict(
+ type='SampleFrames',
+ clip_len=16,
+ frame_interval=4,
+ num_clips=10,
+ test_mode=True),
+ dict(type='RawFrameDecode', **file_client_args),
+ dict(type='Resize', scale=(-1, 256)),
+ dict(type='ThreeCrop', crop_size=256),
+ dict(type='FormatShape', input_format='NCTHW'),
+ dict(type='PackActionInputs')
+]
+
+train_dataloader = dict(
+ batch_size=16,
+ num_workers=8,
+ persistent_workers=True,
+ sampler=dict(type='DefaultSampler', shuffle=True),
+ dataset=dict(
+ type=dataset_type,
+ ann_file=ann_file_train,
+ filename_tmpl='{}_{:05d}.jpg',
+ modality='Flow',
+ data_prefix=dict(img=data_root),
+ pipeline=train_pipeline))
+val_dataloader = dict(
+ batch_size=16,
+ num_workers=8,
+ persistent_workers=True,
+ sampler=dict(type='DefaultSampler', shuffle=False),
+ dataset=dict(
+ type=dataset_type,
+ ann_file=ann_file_val,
+ filename_tmpl='{}_{:05d}.jpg',
+ modality='Flow',
+ data_prefix=dict(img=data_root_val),
+ pipeline=val_pipeline,
+ test_mode=True))
+test_dataloader = dict(
+ batch_size=1,
+ num_workers=8,
+ persistent_workers=True,
+ sampler=dict(type='DefaultSampler', shuffle=False),
+ dataset=dict(
+ type=dataset_type,
+ ann_file=ann_file_val,
+ filename_tmpl='{}_{:05d}.jpg',
+ modality='Flow',
+ data_prefix=dict(img=data_root_val),
+ pipeline=test_pipeline,
+ test_mode=True))
+
+val_evaluator = dict(type='AccMetric')
+test_evaluator = val_evaluator
+
+train_cfg = dict(
+ type='EpochBasedTrainLoop', max_epochs=256, val_begin=1, val_interval=8)
+val_cfg = dict(type='ValLoop')
+test_cfg = dict(type='TestLoop')
+
+# learning policy
+param_scheduler = [
+ dict(type='LinearLR', start_factor=0.1, by_epoch=True, begin=0, end=34),
+ dict(
+ type='CosineAnnealingLR',
+ T_max=222,
+ eta_min=0,
+ by_epoch=True,
+ begin=34,
+ end=256)
+]
+
+optim_wrapper = dict(
+ optimizer=dict(type='SGD', lr=0.2, momentum=0.9, weight_decay=1e-4),
+ clip_grad=dict(max_norm=40, norm_type=2))
+
+# runtime settings
+default_hooks = dict(checkpoint=dict(interval=8, max_keep_ckpts=3))
+
+# Default setting for scaling LR automatically
+# - `enable` means enable scaling LR automatically
+# or not by default.
+# - `base_batch_size` = (8 GPUs) x (16 samples per GPU).
+auto_scale_lr = dict(enable=False, base_batch_size=128)
diff --git a/configs/recognition/tsm/README.md b/configs/recognition/tsm/README.md
index 3014d0e26b..0a02e14cf6 100644
--- a/configs/recognition/tsm/README.md
+++ b/configs/recognition/tsm/README.md
@@ -30,6 +30,7 @@ The explosive growth in video streaming gives rise to challenges on performing v
| 1x1x8 | 224x224 | 8 | ResNet50 (NonLocalGauss) | ImageNet | 73.66 | 90.99 | 8 clips x 10 crop | 59.06G | 28.00M | [config](/configs/recognition/tsm/tsm_imagenet-pretrained-r50-nl-gaussian_8xb16-1x1x8-50e_kinetics400-rgb.py) | [ckpt](https://download.openmmlab.com/mmaction/v1.0/recognition/tsm/tsm_imagenet-pretrained-r50-nl-gaussian_8xb16-1x1x8-50e_kinetics400-rgb/tsm_imagenet-pretrained-r50-nl-gaussian_8xb16-1x1x8-50e_kinetics400-rgb_20220831-7e54dacf.pth) | [log](https://download.openmmlab.com/mmaction/v1.0/recognition/tsm/tsm_imagenet-pretrained-r50-nl-gaussian_8xb16-1x1x8-50e_kinetics400-rgb/tsm_imagenet-pretrained-r50-nl-gaussian_8xb16-1x1x8-50e_kinetics400-rgb.log) |
| 1x1x8 | 224x224 | 8 | ResNet50 (NonLocalEmbedGauss) | ImageNet | 74.34 | 91.23 | 8 clips x 10 crop | 61.30G | 31.68M | [config](/configs/recognition/tsm/tsm_imagenet-pretrained-r50-nl-embedded-gaussian_8xb16-1x1x8-50e_kinetics400-rgb.py) | [ckpt](https://download.openmmlab.com/mmaction/v1.0/recognition/tsm/tsm_imagenet-pretrained-r50-nl-embedded-gaussian_8xb16-1x1x8-50e_kinetics400-rgb/tsm_imagenet-pretrained-r50-nl-embedded-gaussian_8xb16-1x1x8-50e_kinetics400-rgb_20220831-35eddb57.pth) | [log](https://download.openmmlab.com/mmaction/v1.0/recognition/tsm/tsm_imagenet-pretrained-r50-nl-embedded-gaussian_8xb16-1x1x8-50e_kinetics400-rgb/tsm_imagenet-pretrained-r50-nl-embedded-gaussian_8xb16-1x1x8-50e_kinetics400-rgb.log) |
| 1x1x8 | 224x224 | 8 | MobileNetV2 | ImageNet | 68.71 | 88.32 | 8 clips x 3 crop | 3.269G | 2.736M | [config](/configs/recognition/tsm/tsm_imagenet-pretrained-mobilenetv2_8xb16-1x1x8-100e_kinetics400-rgb.py) | [ckpt](https://download.openmmlab.com/mmaction/v1.0/recognition/tsm/tsm_imagenet-pretrained-mobilenetv2_8xb16-1x1x8-100e_kinetics400-rgb/tsm_imagenet-pretrained-mobilenetv2_8xb16-1x1x8-100e_kinetics400-rgb_20230414-401127fd.pth) | [log](https://download.openmmlab.com/mmaction/v1.0/recognition/tsm/tsm_imagenet-pretrained-mobilenetv2_8xb16-1x1x8-100e_kinetics400-rgb/tsm_imagenet-pretrained-mobilenetv2_8xb16-1x1x8-100e_kinetics400-rgb.log) |
+| 1x1x16 | 224x224 | 8 | MobileOne-S4 | ImageNet | 74.38 | 91.71 | 16 clips x 10 crop | 48.65G | 13.72M | [config](/configs/recognition/tsm/tsm_imagenet-pretrained-mobileone-s4_8xb16-1x1x16-50e_kinetics400-rgb.py) | [ckpt](https://download.openmmlab.com/mmaction/v1.0/recognition/tsm/tsm_imagenet-pretrained-mobileone-s4_8xb16-1x1x16-50e_kinetics400-rgb/tsm_imagenet-pretrained-mobileone-s4_8xb16-1x1x16-50e_kinetics400-rgb_20230825-a7f8876b.pth) | [log](https://download.openmmlab.com/mmaction/v1.0/recognition/tsm/tsm_imagenet-pretrained-mobileone-s4_8xb16-1x1x16-50e_kinetics400-rgb/tsm_imagenet-pretrained-mobileone-s4_8xb16-1x1x16-50e_kinetics400-rgb.log) |
### Something-something V2
@@ -41,6 +42,7 @@ The explosive growth in video streaming gives rise to challenges on performing v
1. The **gpus** indicates the number of gpus we used to get the checkpoint. If you want to use a different number of gpus or videos per gpu, the best way is to set `--auto-scale-lr` when calling `tools/train.py`, this parameter will auto-scale the learning rate according to the actual batch size and the original batch size.
2. The validation set of Kinetics400 we used consists of 19796 videos. These videos are available at [Kinetics400-Validation](https://mycuhk-my.sharepoint.com/:u:/g/personal/1155136485_link_cuhk_edu_hk/EbXw2WX94J1Hunyt3MWNDJUBz-nHvQYhO9pvKqm6g39PMA?e=a9QldB). The corresponding [data list](https://download.openmmlab.com/mmaction/dataset/k400_val/kinetics_val_list.txt) (each line is of the format 'video_id, num_frames, label_index') and the [label map](https://download.openmmlab.com/mmaction/dataset/k400_val/kinetics_class2ind.txt) are also available.
+3. MoibleOne backbone supports reparameterization during inference. You can use the provided [reparameterize tool](/tools/convert/reparameterize_model.py) to convert the checkpoint and switch to the [deploy config file](/configs/recognition/tsm/tsm_imagenet-pretrained-mobileone-s4_deploy_8xb16-1x1x16-50e_kinetics400-rgb.py).
For more details on data preparation, you can refer to [Kinetics400](/tools/data/kinetics/README.md).
diff --git a/configs/recognition/tsm/metafile.yml b/configs/recognition/tsm/metafile.yml
index 409f5a95df..0360c16758 100644
--- a/configs/recognition/tsm/metafile.yml
+++ b/configs/recognition/tsm/metafile.yml
@@ -167,6 +167,30 @@ Models:
Training Log: https://download.openmmlab.com/mmaction/v1.0/recognition/tsm/tsm_imagenet-pretrained-r50-nl-gaussian_8xb16-1x1x8-50e_kinetics400-rgb/tsm_imagenet-pretrained-r50-nl-gaussian_8xb16-1x1x8-50e_kinetics400-rgb.log
Weights: https://download.openmmlab.com/mmaction/v1.0/recognition/tsm/tsm_imagenet-pretrained-r50-nl-gaussian_8xb16-1x1x8-50e_kinetics400-rgb/tsm_imagenet-pretrained-r50-nl-gaussian_8xb16-1x1x8-50e_kinetics400-rgb_20220831-7e54dacf.pth
+ - Name: tsm_imagenet-pretrained-mobileone-s4_8xb16-1x1x16-50e_kinetics400-rgb
+ Config: configs/recognition/tsm/tsm_imagenet-pretrained-mobileone-s4_8xb16-1x1x16-50e_kinetics400-rgb.py
+ In Collection: TSM
+ Metadata:
+ Architecture: MobileOne-S4
+ Batch Size: 16
+ Epochs: 100
+ FLOPs: 48.65G
+ Parameters: 13.72M
+ Pretrained: ImageNet
+ Resolution: 224x224
+ Training Data: Kinetics-400
+ Training Resources: 8 GPUs
+ Modality: RGB
+ Results:
+ - Dataset: Kinetics-400
+ Task: Action Recognition
+ Metrics:
+ Top 1 Accuracy: 74.38
+ Top 5 Accuracy: 91.71
+ Training Log: https://download.openmmlab.com/mmaction/v1.0/recognition/tsm/tsm_imagenet-pretrained-mobileone-s4_8xb16-1x1x16-50e_kinetics400-rgb/tsm_imagenet-pretrained-mobileone-s4_8xb16-1x1x16-50e_kinetics400-rgb.log
+ Weights: https://download.openmmlab.com/mmaction/v1.0/recognition/tsm/tsm_imagenet-pretrained-mobileone-s4_8xb16-1x1x16-50e_kinetics400-rgb/tsm_imagenet-pretrained-mobileone-s4_8xb16-1x1x16-50e_kinetics400-rgb_20230825-a7f8876b.pth
+
+
- Name: tsm_imagenet-pretrained-r101_8xb16-1x1x8-50e_sthv2-rgb
Config: configs/recognition/tsm/tsm_imagenet-pretrained-r101_8xb16-1x1x8-50e_sthv2-rgb.py
In Collection: TSM
diff --git a/configs/recognition/tsm/tsm_imagenet-pretrained-mobileone-s4_8xb16-1x1x16-50e_kinetics400-rgb.py b/configs/recognition/tsm/tsm_imagenet-pretrained-mobileone-s4_8xb16-1x1x16-50e_kinetics400-rgb.py
new file mode 100644
index 0000000000..e4fac52656
--- /dev/null
+++ b/configs/recognition/tsm/tsm_imagenet-pretrained-mobileone-s4_8xb16-1x1x16-50e_kinetics400-rgb.py
@@ -0,0 +1,126 @@
+_base_ = [
+ '../../_base_/models/tsm_mobileone_s4.py',
+ '../../_base_/default_runtime.py'
+]
+
+model = dict(cls_head=dict(num_segments=16))
+# dataset settings
+dataset_type = 'VideoDataset'
+data_root = 'data/kinetics400/videos_train'
+data_root_val = 'data/kinetics400/videos_val'
+ann_file_train = 'data/kinetics400/kinetics400_train_list_videos.txt'
+ann_file_val = 'data/kinetics400/kinetics400_val_list_videos.txt'
+
+file_client_args = dict(io_backend='disk')
+
+train_pipeline = [
+ dict(type='DecordInit', **file_client_args),
+ dict(type='SampleFrames', clip_len=1, frame_interval=1, num_clips=16),
+ dict(type='DecordDecode'),
+ dict(type='Resize', scale=(-1, 256)),
+ dict(
+ type='MultiScaleCrop',
+ input_size=224,
+ scales=(1, 0.875, 0.75, 0.66),
+ random_crop=False,
+ max_wh_scale_gap=1,
+ num_fixed_crops=13),
+ dict(type='Resize', scale=(224, 224), keep_ratio=False),
+ dict(type='Flip', flip_ratio=0.5),
+ dict(type='FormatShape', input_format='NCHW'),
+ dict(type='PackActionInputs')
+]
+val_pipeline = [
+ dict(type='DecordInit', **file_client_args),
+ dict(
+ type='SampleFrames',
+ clip_len=1,
+ frame_interval=1,
+ num_clips=16,
+ test_mode=True),
+ dict(type='DecordDecode'),
+ dict(type='Resize', scale=(-1, 256)),
+ dict(type='CenterCrop', crop_size=224),
+ dict(type='FormatShape', input_format='NCHW'),
+ dict(type='PackActionInputs')
+]
+test_pipeline = [
+ dict(type='DecordInit', **file_client_args),
+ dict(
+ type='SampleFrames',
+ clip_len=1,
+ frame_interval=1,
+ num_clips=16,
+ test_mode=True),
+ dict(type='DecordDecode'),
+ dict(type='Resize', scale=(-1, 256)),
+ dict(type='ThreeCrop', crop_size=256),
+ dict(type='FormatShape', input_format='NCHW'),
+ dict(type='PackActionInputs')
+]
+
+train_dataloader = dict(
+ batch_size=8,
+ num_workers=8,
+ persistent_workers=True,
+ sampler=dict(type='DefaultSampler', shuffle=True),
+ dataset=dict(
+ type=dataset_type,
+ ann_file=ann_file_train,
+ data_prefix=dict(video=data_root),
+ pipeline=train_pipeline))
+val_dataloader = dict(
+ batch_size=8,
+ num_workers=8,
+ persistent_workers=True,
+ sampler=dict(type='DefaultSampler', shuffle=False),
+ dataset=dict(
+ type=dataset_type,
+ ann_file=ann_file_val,
+ data_prefix=dict(video=data_root_val),
+ pipeline=val_pipeline,
+ test_mode=True))
+test_dataloader = dict(
+ batch_size=1,
+ num_workers=8,
+ persistent_workers=True,
+ sampler=dict(type='DefaultSampler', shuffle=False),
+ dataset=dict(
+ type=dataset_type,
+ ann_file=ann_file_val,
+ data_prefix=dict(video=data_root_val),
+ pipeline=test_pipeline,
+ test_mode=True))
+
+val_evaluator = dict(type='AccMetric')
+test_evaluator = val_evaluator
+
+default_hooks = dict(checkpoint=dict(interval=3, max_keep_ckpts=3))
+
+train_cfg = dict(
+ type='EpochBasedTrainLoop', max_epochs=50, val_begin=1, val_interval=1)
+val_cfg = dict(type='ValLoop')
+test_cfg = dict(type='TestLoop')
+
+param_scheduler = [
+ dict(type='LinearLR', start_factor=0.1, by_epoch=True, begin=0, end=5),
+ dict(
+ type='MultiStepLR',
+ begin=0,
+ end=50,
+ by_epoch=True,
+ milestones=[25, 45],
+ gamma=0.1)
+]
+
+optim_wrapper = dict(
+ constructor='TSMOptimWrapperConstructor',
+ paramwise_cfg=dict(fc_lr5=True),
+ optimizer=dict(type='SGD', lr=0.02, momentum=0.9, weight_decay=0.00002),
+ clip_grad=dict(max_norm=20, norm_type=2))
+
+# Default setting for scaling LR automatically
+# - `enable` means enable scaling LR automatically
+# or not by default.
+# - `base_batch_size` = (8 GPUs) x (16 samples per GPU).
+auto_scale_lr = dict(enable=True, base_batch_size=128)
diff --git a/configs/recognition/tsm/tsm_imagenet-pretrained-mobileone-s4_deploy_8xb16-1x1x16-50e_kinetics400-rgb.py b/configs/recognition/tsm/tsm_imagenet-pretrained-mobileone-s4_deploy_8xb16-1x1x16-50e_kinetics400-rgb.py
new file mode 100644
index 0000000000..ecd0ed32e0
--- /dev/null
+++ b/configs/recognition/tsm/tsm_imagenet-pretrained-mobileone-s4_deploy_8xb16-1x1x16-50e_kinetics400-rgb.py
@@ -0,0 +1,5 @@
+_base_ = [
+ './tsm_imagenet-pretrained-mobileone-s4_8xb16-1x1x16-50e_kinetics400-rgb.py', # noqa: E501
+]
+
+model = dict(backbone=dict(deploy=True))
diff --git a/configs/recognition/tsn/README.md b/configs/recognition/tsn/README.md
index 8ff8222649..ca21386ce2 100644
--- a/configs/recognition/tsn/README.md
+++ b/configs/recognition/tsn/README.md
@@ -40,6 +40,7 @@ Deep convolutional networks have achieved great success for visual recognition i
It's possible and convenient to use a 3rd-party backbone for TSN under the framework of MMAction2, here we provide some examples for:
- [x] Backbones from [MMClassification](https://github.com/open-mmlab/mmclassification/)
+- [x] Backbones from [MMPretrain](https://github.com/open-mmlab/mmpretrain)
- [x] Backbones from [TorchVision](https://github.com/pytorch/vision/)
- [x] Backbones from [TIMM (pytorch-image-models)](https://github.com/rwightman/pytorch-image-models)
@@ -49,10 +50,12 @@ It's possible and convenient to use a 3rd-party backbone for TSN under the frame
| 1x1x3 | MultiStep | 224x224 | 8 | DenseNet161 | ImageNet | 72.07 | 90.15 | 25 clips x 10 crop | 194.6G | 27.36M | [config](/configs/recognition/tsn/custom_backbones/tsn_imagenet-pretrained-dense161_8xb32-1x1x3-100e_kinetics400-rgb.py) | [ckpt](https://download.openmmlab.com/mmaction/v1.0/recognition/tsn/tsn_imagenet-pretrained-dense161_8xb32-1x1x3-100e_kinetics400-rgb/tsn_imagenet-pretrained-dense161_8xb32-1x1x3-100e_kinetics400-rgb_20220906-5f4c0daf.pth) | [log](https://download.openmmlab.com/mmaction/v1.0/recognition/tsn/tsn_imagenet-pretrained-dense161_8xb32-1x1x3-100e_kinetics400-rgb/tsn_imagenet-pretrained-dense161_8xb32-1x1x3-100e_kinetics400-rgb.log) |
| 1x1x3 | MultiStep | 224x224 | 8 | Swin Transformer | ImageNet | 77.03 | 92.61 | 25 clips x 10 crop | 386.7G | 87.15M | [config](/configs/recognition/tsn/custom_backbones/tsn_imagenet-pretrained-swin-transformer_8xb32-1x1x3-100e_kinetics400-rgb.py) | [ckpt](https://download.openmmlab.com/mmaction/v1.0/recognition/tsn/tsn_imagenet-pretrained-swin-transformer_8xb32-1x1x3-100e_kinetics400-rgb/tsn_imagenet-pretrained-swin-transformer_8xb32-1x1x3-100e_kinetics400-rgb_20220906-65ed814e.pth) | [log](https://download.openmmlab.com/mmaction/v1.0/recognition/tsn/tsn_imagenet-pretrained-swin-transformer_8xb32-1x1x3-100e_kinetics400-rgb/tsn_imagenet-pretrained-swin-transformer_8xb32-1x1x3-100e_kinetics400-rgb.log) |
| 1x1x8 | MultiStep | 224x224 | 8 | Swin Transformer | ImageNet | 79.22 | 94.20 | 25 clips x 10 crop | 386.7G | 87.15M | [config](/configs/recognition/tsn/custom_backbones/tsn_imagenet-pretrained-swin-transformer_32xb8-1x1x8-50e_kinetics400-rgb.py) | [ckpt](https://download.openmmlab.com/mmaction/v1.0/recognition/tsn/tsn_imagenet-pretrained-swin-transformer_32xb8-1x1x8-50e_kinetics400-rgb/tsn_imagenet-pretrained-swin-transformer_32xb8-1x1x8-50e_kinetics400-rgb_20230530-428f0064.pth) | [log](https://download.openmmlab.com/mmaction/v1.0/recognition/tsn/tsn_imagenet-pretrained-swin-transformer_32xb8-1x1x8-50e_kinetics400-rgb/tsn_imagenet-pretrained-swin-transformer_32xb8-1x1x8-50e_kinetics400-rgb.log) |
+| 1x1x8 | MultiStep | 224x224 | 8 | MobileOne-S4 | ImageNet | 73.65 | 91.32 | 25 clips x 10 crop | 76G | 13.72M | [config](/configs/recognition/tsn/custom_backbones/tsn_imagenet-pretrained-mobileone-s4_8xb32-1x1x8-100e_kinetics400-rgb.py) | [ckpt](https://download.openmmlab.com/mmaction/v1.0/recognition/tsn/tsn_imagenet-pretrained-mobileone-s4_8xb32-1x1x8-100e_kinetics400-rgb/tsn_imagenet-pretrained-mobileone-s4_8xb32-1x1x8-100e_kinetics400-rgb_20230825-2da3c1f7.pth) | [log](https://download.openmmlab.com/mmaction/v1.0/recognition/tsn/tsn_imagenet-pretrained-mobileone-s4_8xb32-1x1x8-100e_kinetics400-rgb/tsn_imagenet-pretrained-mobileone-s4_8xb32-1x1x8-100e_kinetics400-rgb.log) |
1. Note that some backbones in TIMM are not supported due to multiple reasons. Please refer to [PR #880](https://github.com/open-mmlab/mmaction2/pull/880) for details.
2. The **gpus** indicates the number of gpus we used to get the checkpoint. If you want to use a different number of gpus or videos per gpu, the best way is to set `--auto-scale-lr` when calling `tools/train.py`, this parameter will auto-scale the learning rate according to the actual batch size and the original batch size.
3. The validation set of Kinetics400 we used consists of 19796 videos. These videos are available at [Kinetics400-Validation](https://mycuhk-my.sharepoint.com/:u:/g/personal/1155136485_link_cuhk_edu_hk/EbXw2WX94J1Hunyt3MWNDJUBz-nHvQYhO9pvKqm6g39PMA?e=a9QldB). The corresponding [data list](https://download.openmmlab.com/mmaction/dataset/k400_val/kinetics_val_list.txt) (each line is of the format 'video_id, num_frames, label_index') and the [label map](https://download.openmmlab.com/mmaction/dataset/k400_val/kinetics_class2ind.txt) are also available.
+4. MoibleOne backbone supports reparameterization during inference. You can use the provided [reparameterize tool](/tools/convert/reparameterize_model.py) to convert the checkpoint and switch to the [deploy config file](/configs/recognition/tsn/custom_backbones/tsn_imagenet-pretrained-mobileone-s4_deploy_8xb32-1x1x8-100e_kinetics400-rgb.py).
For more details on data preparation, you can refer to
diff --git a/configs/recognition/tsn/custom_backbones/tsn_imagenet-pretrained-mobileone-s4_8xb32-1x1x8-100e_kinetics400-rgb.py b/configs/recognition/tsn/custom_backbones/tsn_imagenet-pretrained-mobileone-s4_8xb32-1x1x8-100e_kinetics400-rgb.py
new file mode 100644
index 0000000000..5f07bf40ab
--- /dev/null
+++ b/configs/recognition/tsn/custom_backbones/tsn_imagenet-pretrained-mobileone-s4_8xb32-1x1x8-100e_kinetics400-rgb.py
@@ -0,0 +1,75 @@
+_base_ = ['../tsn_imagenet-pretrained-r50_8xb32-1x1x3-100e_kinetics400-rgb.py']
+
+# dataset settings
+checkpoint = ('https://download.openmmlab.com/mmclassification/'
+ 'v0/mobileone/mobileone-s4_8xb32_in1k_20221110-28d888cb.pth')
+model = dict(
+ backbone=dict(
+ type='mmpretrain.MobileOne',
+ arch='s4',
+ out_indices=(3, ),
+ init_cfg=dict(
+ type='Pretrained', checkpoint=checkpoint, prefix='backbone'),
+ _delete_=True),
+ cls_head=dict(in_channels=2048))
+
+dataset_type = 'VideoDataset'
+data_root = 'data/kinetics400/videos_train'
+data_root_val = 'data/kinetics400/videos_val'
+ann_file_train = 'data/kinetics400/kinetics400_train_list_videos.txt'
+ann_file_val = 'data/kinetics400/kinetics400_val_list_videos.txt'
+
+file_client_args = dict(io_backend='disk')
+
+train_pipeline = [
+ dict(type='DecordInit', **file_client_args),
+ dict(type='SampleFrames', clip_len=1, frame_interval=1, num_clips=8),
+ dict(type='DecordDecode'),
+ dict(type='Resize', scale=(-1, 256)),
+ dict(
+ type='MultiScaleCrop',
+ input_size=224,
+ scales=(1, 0.875, 0.75, 0.66),
+ random_crop=False,
+ max_wh_scale_gap=1),
+ dict(type='Resize', scale=(224, 224), keep_ratio=False),
+ dict(type='Flip', flip_ratio=0.5),
+ dict(type='FormatShape', input_format='NCHW'),
+ dict(type='PackActionInputs')
+]
+val_pipeline = [
+ dict(type='DecordInit', **file_client_args),
+ dict(
+ type='SampleFrames',
+ clip_len=1,
+ frame_interval=1,
+ num_clips=8,
+ test_mode=True),
+ dict(type='DecordDecode'),
+ dict(type='Resize', scale=(-1, 256)),
+ dict(type='CenterCrop', crop_size=224),
+ dict(type='FormatShape', input_format='NCHW'),
+ dict(type='PackActionInputs')
+]
+
+train_dataloader = dict(
+ batch_size=16,
+ num_workers=8,
+ persistent_workers=True,
+ sampler=dict(type='DefaultSampler', shuffle=True),
+ dataset=dict(
+ type=dataset_type,
+ ann_file=ann_file_train,
+ data_prefix=dict(video=data_root),
+ pipeline=train_pipeline))
+val_dataloader = dict(
+ batch_size=16,
+ num_workers=8,
+ persistent_workers=True,
+ sampler=dict(type='DefaultSampler', shuffle=False),
+ dataset=dict(
+ type=dataset_type,
+ ann_file=ann_file_val,
+ data_prefix=dict(video=data_root_val),
+ pipeline=val_pipeline,
+ test_mode=True))
diff --git a/configs/recognition/tsn/custom_backbones/tsn_imagenet-pretrained-mobileone-s4_deploy_8xb32-1x1x8-100e_kinetics400-rgb.py b/configs/recognition/tsn/custom_backbones/tsn_imagenet-pretrained-mobileone-s4_deploy_8xb32-1x1x8-100e_kinetics400-rgb.py
new file mode 100644
index 0000000000..38ab106a3f
--- /dev/null
+++ b/configs/recognition/tsn/custom_backbones/tsn_imagenet-pretrained-mobileone-s4_deploy_8xb32-1x1x8-100e_kinetics400-rgb.py
@@ -0,0 +1,5 @@
+_base_ = [
+ './tsn_imagenet-pretrained-mobileone-s4_8xb32-1x1x8-100e_kinetics400-rgb.py' # noqa: E501
+]
+
+model = dict(backbone=dict(deploy=True))
diff --git a/configs/recognition/tsn/metafile.yml b/configs/recognition/tsn/metafile.yml
index 378040098c..06822d633c 100644
--- a/configs/recognition/tsn/metafile.yml
+++ b/configs/recognition/tsn/metafile.yml
@@ -215,6 +215,29 @@ Models:
Training Log: https://download.openmmlab.com/mmaction/v1.0/recognition/tsn/tsn_imagenet-pretrained-swin-transformer_32xb8-1x1x8-50e_kinetics400-rgb/tsn_imagenet-pretrained-swin-transformer_32xb8-1x1x8-50e_kinetics400-rgb.log
Weights: https://download.openmmlab.com/mmaction/v1.0/recognition/tsn/tsn_imagenet-pretrained-swin-transformer_8xb32-1x1x3-100e_kinetics400-rgb/tsn_imagenet-pretrained-swin-transformer_8xb32-1x1x3-100e_kinetics400-rgb_20220906-65ed814e.pth
+ - Name: tsn_imagenet-pretrained-mobileone-s4_8xb32-1x1x8-100e_kinetics400-rgb
+ Config: configs/recognition/tsn/custom_backbones/tsn_imagenet-pretrained-mobileone-s4_8xb32-1x1x8-100e_kinetics400-rgb.py
+ In Collection: TSN
+ Metadata:
+ Architecture: MobileOne-S4
+ Batch Size: 32
+ Epochs: 100
+ FLOPs: 76G
+ Parameters: 13.72M
+ Pretrained: ImageNet
+ Resolution: 224x224
+ Training Data: Kinetics-400
+ Training Resources: 8 GPUs
+ Modality: RGB
+ Results:
+ - Dataset: Kinetics-400
+ Task: Action Recognition
+ Metrics:
+ Top 1 Accuracy: 73.65
+ Top 5 Accuracy: 91.32
+ Training Log: https://download.openmmlab.com/mmaction/v1.0/recognition/tsn/tsn_imagenet-pretrained-mobileone-s4_8xb32-1x1x8-100e_kinetics400-rgb/tsn_imagenet-pretrained-mobileone-s4_8xb32-1x1x8-100e_kinetics400-rgb.log
+ Weights: https://download.openmmlab.com/mmaction/v1.0/recognition/tsn/tsn_imagenet-pretrained-mobileone-s4_8xb32-1x1x8-100e_kinetics400-rgb/tsn_imagenet-pretrained-mobileone-s4_8xb32-1x1x8-100e_kinetics400-rgb_20230825-2da3c1f7.pth
+
- Name: tsn_imagenet-pretrained-r50_8xb32-1x1x8-50e_sthv2-rgb
Config: configs/recognition/tsn/tsn_imagenet-pretrained-r50_8xb32-1x1x8-50e_sthv2-rgb.py
In Collection: TSN
diff --git a/configs/recognition/tsn/tsn_imagenet-pretrained-r50_8xb32_5x1x3-110e_kinetics400-flow.py b/configs/recognition/tsn/tsn_imagenet-pretrained-r50_8xb32_5x1x3-110e_kinetics400-flow.py
new file mode 100644
index 0000000000..a25eb31334
--- /dev/null
+++ b/configs/recognition/tsn/tsn_imagenet-pretrained-r50_8xb32_5x1x3-110e_kinetics400-flow.py
@@ -0,0 +1,141 @@
+_base_ = '../../_base_/default_runtime.py'
+
+clip_len = 5
+
+model = dict(
+ type='Recognizer2D',
+ backbone=dict(
+ type='ResNet',
+ pretrained='https://download.pytorch.org/models/resnet50-11ad3fa6.pth',
+ depth=50,
+ in_channels=2 * clip_len, # ``in_channels`` should be 2 * clip_len
+ norm_eval=False),
+ cls_head=dict(
+ type='TSNHead',
+ num_classes=400,
+ in_channels=2048,
+ spatial_type='avg',
+ consensus=dict(type='AvgConsensus', dim=1),
+ dropout_ratio=0.4,
+ init_std=0.01,
+ average_clips='prob'),
+ data_preprocessor=dict(
+ type='ActionDataPreprocessor',
+ mean=[128, 128] * clip_len, # ``in_channels`` should be 2 * clip_len
+ std=[128, 128] * clip_len, # ``in_channels`` should be 2 * clip_len
+ format_shape='NCHW'))
+
+# dataset settings
+dataset_type = 'RawframeDataset'
+data_root = 'data/kinetics400/rawframes_train'
+data_root_val = 'data/kinetics400/rawframes_val'
+ann_file_train = 'data/kinetics400/kinetics400_train_list_flow.txt'
+ann_file_val = 'data/kinetics400/kinetics400_val_list_flow.txt'
+ann_file_test = 'data/kinetics400/kinetics400_val_list_flow.txt'
+file_client_args = dict(io_backend='disk')
+train_pipeline = [
+ dict(
+ type='SampleFrames', clip_len=clip_len, frame_interval=1, num_clips=3),
+ dict(type='RawFrameDecode', **file_client_args),
+ dict(type='Resize', scale=(-1, 256)),
+ dict(type='RandomResizedCrop'),
+ dict(type='Resize', scale=(224, 224), keep_ratio=False),
+ dict(type='Flip', flip_ratio=0.5),
+ dict(type='FormatShape', input_format='NCHW'),
+ dict(type='PackActionInputs')
+]
+val_pipeline = [
+ dict(
+ type='SampleFrames',
+ clip_len=clip_len,
+ frame_interval=1,
+ num_clips=3,
+ test_mode=True),
+ dict(type='RawFrameDecode', **file_client_args),
+ dict(type='Resize', scale=(-1, 256)),
+ dict(type='CenterCrop', crop_size=224),
+ dict(type='FormatShape', input_format='NCHW'),
+ dict(type='PackActionInputs')
+]
+test_pipeline = [
+ dict(
+ type='SampleFrames',
+ clip_len=clip_len,
+ frame_interval=1,
+ num_clips=25,
+ test_mode=True),
+ dict(type='RawFrameDecode'),
+ dict(type='Resize', scale=(-1, 256)),
+ dict(type='TenCrop', crop_size=224),
+ dict(type='FormatShape', input_format='NCHW'),
+ dict(type='PackActionInputs')
+]
+
+train_dataloader = dict(
+ batch_size=32,
+ num_workers=8,
+ persistent_workers=True,
+ sampler=dict(type='DefaultSampler', shuffle=True),
+ dataset=dict(
+ type=dataset_type,
+ ann_file=ann_file_train,
+ filename_tmpl='{}_{:05d}.jpg',
+ modality='Flow',
+ data_prefix=dict(img=data_root),
+ pipeline=train_pipeline))
+val_dataloader = dict(
+ batch_size=32,
+ num_workers=8,
+ persistent_workers=True,
+ sampler=dict(type='DefaultSampler', shuffle=False),
+ dataset=dict(
+ type=dataset_type,
+ ann_file=ann_file_val,
+ filename_tmpl='{}_{:05d}.jpg',
+ modality='Flow',
+ data_prefix=dict(img=data_root_val),
+ pipeline=val_pipeline,
+ test_mode=True))
+test_dataloader = dict(
+ batch_size=1,
+ num_workers=8,
+ persistent_workers=True,
+ sampler=dict(type='DefaultSampler', shuffle=False),
+ dataset=dict(
+ type=dataset_type,
+ ann_file=ann_file_val,
+ filename_tmpl='{}_{:05d}.jpg',
+ modality='Flow',
+ data_prefix=dict(img=data_root_val),
+ pipeline=test_pipeline,
+ test_mode=True))
+
+val_evaluator = dict(type='AccMetric')
+test_evaluator = val_evaluator
+
+train_cfg = dict(
+ type='EpochBasedTrainLoop', max_epochs=110, val_begin=1, val_interval=5)
+val_cfg = dict(type='ValLoop')
+test_cfg = dict(type='TestLoop')
+
+optim_wrapper = dict(
+ optimizer=dict(type='SGD', lr=0.005, momentum=0.9, weight_decay=0.0001),
+ clip_grad=dict(max_norm=40, norm_type=2))
+
+param_scheduler = [
+ dict(
+ type='MultiStepLR',
+ begin=0,
+ end=110,
+ by_epoch=True,
+ milestones=[70, 100],
+ gamma=0.1)
+]
+
+default_hooks = dict(checkpoint=dict(interval=5, max_keep_ckpts=3))
+
+# Default setting for scaling LR automatically
+# - `enable` means enable scaling LR automatically
+# or not by default.
+# - `base_batch_size` = (8 GPUs) x (32 samples per GPU).
+auto_scale_lr = dict(enable=False, base_batch_size=256)
diff --git a/configs/recognition/uniformer/README.md b/configs/recognition/uniformer/README.md
index 6d04b7920e..4cbbba0e8a 100644
--- a/configs/recognition/uniformer/README.md
+++ b/configs/recognition/uniformer/README.md
@@ -32,7 +32,7 @@ The models are ported from the repo [UniFormer](https://github.com/Sense-X/UniFo
2. The values in `top1/5 acc` is tested on the same data list as the original repo, and the label map is provided by [UniFormer](https://drive.google.com/drive/folders/17VB-XdF3Kfr9ORmnGyXCxTMs86n0L4QL). The total videos are available at [Kinetics400](https://pan.baidu.com/s/1t5K0FRz3PGAT-37-3FwAfg) (BaiduYun password: g5kp), which consists of 19787 videos.
3. The values in columns named after "mm-Kinetics" are the testing results on the Kinetics dataset held by MMAction2, which is also used by other models in MMAction2. Due to the differences between various versions of Kinetics dataset, there is a little gap between `top1/5 acc` and `mm-Kinetics top1/5 acc`. For a fair comparison with other models, we report both results here. Note that we simply report the inference results, since the training set is different between UniFormer and other models, the results are lower than that tested on the author's version.
4. Since the original models for Kinetics-400/600/700 adopt different [label file](https://drive.google.com/drive/folders/17VB-XdF3Kfr9ORmnGyXCxTMs86n0L4QL), we simply map the weight according to the label name. New label map for Kinetics-400/600/700 can be found [here](https://github.com/open-mmlab/mmaction2/tree/main/tools/data/kinetics).
-5. Due to some difference between [SlowFast](https://github.com/facebookresearch/SlowFast) and MMAction, there are some gaps between their performances.
+5. Due to some difference between [SlowFast](https://github.com/facebookresearch/SlowFast) and MMAction2, there are some gaps between their performances.
For more details on data preparation, you can refer to [preparing_kinetics](/tools/data/kinetics/README.md).
diff --git a/configs/recognition/uniformer/README_zh-CN.md b/configs/recognition/uniformer/README_zh-CN.md
new file mode 100644
index 0000000000..b5bda7f039
--- /dev/null
+++ b/configs/recognition/uniformer/README_zh-CN.md
@@ -0,0 +1,55 @@
+# UniFormer
+
+[UniFormer: Unified Transformer for Efficient Spatiotemporal Representation Learning](https://arxiv.org/abs/2201.04676)
+
+
+
+## ็ฎไป
+
+```BibTeX
+@inproceedings{
+ li2022uniformer,
+ title={UniFormer: Unified Transformer for Efficient Spatial-Temporal Representation Learning},
+ author={Kunchang Li and Yali Wang and Gao Peng and Guanglu Song and Yu Liu and Hongsheng Li and Yu Qiao},
+ booktitle={International Conference on Learning Representations},
+ year={2022},
+ url={https://openreview.net/forum?id=nBU_u6DLvoK}
+}
+```
+
+## ๆจกๅๅบ
+
+### Kinetics-400
+
+| ๅธง้ๆ ท็ญ็ฅ | ๅ่พจ็ | ไธปๅนฒ็ฝ็ป | top1 ๅ็กฎ็ | top5 ๅ็กฎ็ | [ๅ่ๆ็ฎ](https://github.com/Sense-X/UniFormer/blob/main/video_classification/README.md) top1 ๅ็กฎ็ | [ๅ่ๆ็ฎ](https://github.com/Sense-X/UniFormer/blob/main/video_classification/README.md) top5 ๅ็กฎ็ | mm-Kinetics top1 ๅ็กฎ็ | mm-Kinetics top5 ๅ็กฎ็ | ๆต่ฏๆนๆก | FLOPs | ๅๆฐ้ | ้
็ฝฎๆไปถ | ckpt |
+| :--------: | :------------: | :---------: | :---------: | :---------: | :---------------------------------------------------------------------------------------------------: | :---------------------------------------------------------------------------------------------------: | :---------------------: | :---------------------: | :--------------: | :---: | :----: | :-----------------------------------------------------------------------------------------------: | :------------------------------------------------------------------------------------------------------------------------------------------------------: |
+| 16x4x1 | short-side 320 | UniFormer-S | 80.9 | 94.6 | 80.8 | 94.7 | 80.9 | 94.6 | 4 clips x 1 crop | 41.8G | 21.4M | [config](/configs/recognition/uniformer/uniformer-small_imagenet1k-pre_16x4x1_kinetics400-rgb.py) | [ckpt](https://download.openmmlab.com/mmaction/v1.0/recognition/uniformerv1/uniformer-small_imagenet1k-pre_16x4x1_kinetics400-rgb_20221219-c630a037.pth) |
+| 16x4x1 | short-side 320 | UniFormer-B | 82.0 | 95.0 | 82.0 | 95.1 | 82.0 | 95.0 | 4 clips x 1 crop | 96.7G | 49.8M | [config](/configs/recognition/uniformer/uniformer-base_imagenet1k-pre_16x4x1_kinetics400-rgb.py) | [ckpt](https://download.openmmlab.com/mmaction/v1.0/recognition/uniformerv1/uniformer-base_imagenet1k-pre_16x4x1_kinetics400-rgb_20221219-157c2e66.pth) |
+| 32x4x1 | short-side 320 | UniFormer-B | 83.1 | 95.3 | 82.9 | 95.4 | 83.0 | 95.3 | 4 clips x 1 crop | 59G | 49.8M | [config](/configs/recognition/uniformer/uniformer-base_imagenet1k-pre_32x4x1_kinetics400-rgb.py) | [ckpt](https://download.openmmlab.com/mmaction/v1.0/recognition/uniformerv1/uniformer-base_imagenet1k-pre_32x4x1_kinetics400-rgb_20221219-b776322c.pth) |
+
+่ฟไบๆจกๅ่ฟ็งป่ช [UniFormer](https://github.com/Sense-X/UniFormer/blob/main/video_classification/README.md)ไปๅบ๏ผๅนถๅจๆไปฌ็ๆฐๆฎไธ่ฟ่กไบๆต่ฏใ็ฎๅ๏ผๆไปฌไป
ๆฏๆๅฏน UniFormer ๆจกๅ็ๆต่ฏ๏ผ่ฎญ็ปๅ่ฝๅฐๅพๅฟซๆไพใ
+
+1. ๅ็งฐไธบ"ๅ่ๆ็ฎ"็ๅไธญ็ๅผๆฏๅๅงไปๅบ็็ปๆใ
+2. `top1/5 ๅ็กฎ็`ไธญ็ๅผๆฏๆจกๅๅจไธๅๅงไปๅบ็ธๅ็ๆฐๆฎ้ไธ็ๆต่ฏ็ปๆ๏ผๅ็ฑปๅจ็ปๆ-ๆ ็ญพๆ ๅฐไธ[UniFormer](https://drive.google.com/drive/folders/17VB-XdF3Kfr9ORmnGyXCxTMs86n0L4QL)ไธ่ดใๆฐๆฎ้ๆปๅ
ฑๆ19787ไธช่ง้ข๏ผๅฏไปฅๅจ[Kinetics400](https://pan.baidu.com/s/1t5K0FRz3PGAT-37-3FwAfg)๏ผ็พๅบฆไบๅฏ็ ๏ผg5kp๏ผไธญ่ทๅใ
+3. ๅ็งฐไธบ "mm-Kinetics" ็ๅไธญ็ๅผๆฏๆจกๅๅจ MMAction2 ๆๆ็ Kinetics ๆฐๆฎ้ไธ็ๆต่ฏ็ปๆ๏ผๅ
ถไป MMAction2 ๆจกๅไนไฝฟ็จไบ่ฏฅๆฐๆฎ้ใ็ฑไบ Kinetics ๆฐๆฎ้็ๅไธช็ๆฌไน้ดๅญๅจๅทฎๅผ๏ผๅ ๆญค `top1/5 ๅ็กฎ็` ๅ `mm-Kinetics top1/5 ๅ็กฎ็` ไน้ดๅญๅจไธไบๅทฎ่ทใไธบไบไธๅ
ถไปๆจกๅ่ฟ่กๅ
ฌๅนณๆฏ่พ๏ผๆไปฌๅจ่ฟ้ๆฅๅไบไธคไธช็ปๆใ่ฏทๆณจๆ๏ผๆไปฌๅชๆฅๅไบๆจ็็ปๆ๏ผ็ฑไบ UniFormer ๅๅ
ถไปๆจกๅไน้ด็่ฎญ็ป้ไธๅ๏ผ่ฏฅ็ปๆไฝไบๅจไฝ่
็ๆฌไธๆต่ฏ็็ปๆใ
+4. ็ฑไบ Kinetics-400/600/700 ็ๅๅงๆจกๅ้็จไบไธๅ็[ๆ ็ญพๆไปถ](https://drive.google.com/drive/folders/17VB-XdF3Kfr9ORmnGyXCxTMs86n0L4QL)๏ผๆไปฌๆ นๆฎๆ ็ญพๅ็งฐ็ฎๅๅฐๆ ๅฐไบๆ้ใKinetics-400/600/700 ็ๆฐๆ ็ญพๆ ๅฐๅฏไปฅๅจ[่ฟ้](https://github.com/open-mmlab/mmaction2/tree/main/tools/data/kinetics)ๆพๅฐใ
+5. ็ฑไบ \[SlowFast\] (https://github.com/facebookresearch/SlowFast)ๅ MMAction2 ไน้ดๅญๅจไธไบๅทฎๅผ๏ผๅฎไปฌ็ๆง่ฝๅญๅจไธไบๅทฎ่ทใ
+
+ๆๅ
ณๆฐๆฎๅๅค็ๆดๅค่ฏฆ็ปไฟกๆฏ๏ผๆจๅฏไปฅๅ่[ๅๅค_kinetics](/tools/data/kinetics/README_zh-CN.md)ใ
+
+## ๅฆไฝๆต่ฏ
+
+ๆจๅฏไปฅไฝฟ็จไปฅไธๅฝไปคๆฅๆต่ฏๆจกๅ๏ผ
+
+```shell
+python tools/test.py ${CONFIG_FILE} ${CHECKPOINT_FILE} [optional arguments]
+```
+
+็คบไพ๏ผๅจKinetics-400ๆฐๆฎ้ไธๆต่ฏ UniFormer-S ๆจกๅ๏ผๅนถๅฐ็ปๆ่ฝฌๅจๅฐไธไธช pkl ๆไปถไธญใ
+
+```shell
+python tools/test.py configs/recognition/uniformer/uniformer-small_imagenet1k-pre_16x4x1_kinetics400-rgb.py \
+ checkpoints/SOME_CHECKPOINT.pth --dump result.pkl
+```
+
+ๆๅ
ณๆดๅค่ฏฆ็ปไฟกๆฏ๏ผ่ฏทๅ่[่ฎญ็ปๅๆต่ฏๆ็จ](/docs/zh_cn/user_guides/train_test.md)ไธญ็**ๆต่ฏ**้จๅใ
diff --git a/configs/recognition/uniformerv2/README_zh-CN.md b/configs/recognition/uniformerv2/README_zh-CN.md
new file mode 100644
index 0000000000..a8e43760b0
--- /dev/null
+++ b/configs/recognition/uniformerv2/README_zh-CN.md
@@ -0,0 +1,98 @@
+# UniFormerV2
+
+[UniFormerV2: Spatiotemporal Learning by Arming Image ViTs with Video UniFormer](https://arxiv.org/abs/2211.09552)
+
+
+
+## ็ฎไป
+
+```BibTeX
+@article{Li2022UniFormerV2SL,
+ title={UniFormerV2: Spatiotemporal Learning by Arming Image ViTs with Video UniFormer},
+ author={Kunchang Li and Yali Wang and Yinan He and Yizhuo Li and Yi Wang and Limin Wang and Y. Qiao},
+ journal={ArXiv},
+ year={2022},
+ volume={abs/2211.09552}
+}
+```
+
+## ๆจกๅๅบ
+
+### Kinetics-400
+
+| ๅๅ้ๆ ทๅธงๆฐ | ๅ่พจ็ | ไธปๅนฒ็ฝ็ป | ไธ่ฎญ็ป | top1 ๅ็กฎ็ | top5 ๅ็กฎ็ | [ๅ่ๆ็ฎ](https://github.com/OpenGVLab/UniFormerV2/blob/main/MODEL_ZOO.md) top1 ๅ็กฎ็ | [ๅ่ๆ็ฎ](https://github.com/OpenGVLab/UniFormerV2/blob/main/MODEL_ZOO.md) top5 ๅ็กฎ็ | mm-Kinetics top1 ๅ็กฎ็ | mm-Kinetics top5 ๅ็กฎ็ | ๆต่ฏๆนๆก | FLOPs | ๅๆฐ้ | ้
็ฝฎๆไปถ | ckpt | log |
+| :----------: | :------------: | :--------------------: | :--------------: | :---------: | :---------: | :-------------------------------------------------------------------------------------: | :-------------------------------------------------------------------------------------: | :---------------------: | :---------------------: | :--------------: | :---: | :----: | :---------------------------------------------------------------------------------------------------------------------: | :----------------------------------------------------------------------------------------------------------------------------------------------------------------------------------------------------------------------------------------------------: | :---------------------------------------------------------------------------------------------------------------------------------------------------------------------------------------------------------------------------------: |
+| 8 | short-side 320 | UniFormerV2-B/16 | clip | - | - | 84.3 | 96.4 | 84.4 | 96.3 | 4 clips x 3 crop | 0.1T | 115M | [config](/configs/recognition/uniformerv2/uniformerv2-base-p16-res224_clip_8xb32-u8_kinetics400-rgb.py) | [ckpt](https://download.openmmlab.com/mmaction/v1.0/recognition/uniformerv2/uniformerv2-base-p16-res224_clip_8xb32-u8_kinetics400-rgb/uniformerv2-base-p16-res224_clip_8xb32-u8_kinetics400-rgb_20230313-e29fc968.pth) | [log](https://download.openmmlab.com/mmaction/v1.0/recognition/uniformerv2/uniformerv2-base-p16-res224_clip_8xb32-u8_kinetics400-rgb/uniformerv2-base-p16-res224_clip_8xb32-u8_kinetics400-rgb.log) |
+| 8 | short-side 320 | UniFormerV2-B/16 | clip-kinetics710 | - | - | 85.6 | 97.0 | 85.8 | 97.1 | 4 clips x 3 crop | 0.1T | 115M | [config](/configs/recognition/uniformerv2/uniformerv2-base-p16-res224_clip-kinetics710-pre_8xb32-u8_kinetics400-rgb.py) | [ckpt](https://download.openmmlab.com/mmaction/v1.0/recognition/uniformerv2/uniformerv2-base-p16-res224_clip-kinetics710-pre_8xb32-u8_kinetics400-rgb/uniformerv2-base-p16-res224_clip-kinetics710-pre_8xb32-u8_kinetics400-rgb_20230313-75be0806.pth) | [log](https://download.openmmlab.com/mmaction/v1.0/recognition/uniformerv2/uniformerv2-base-p16-res224_clip-kinetics710-pre_8xb32-u8_kinetics400-rgb/uniformerv2-base-p16-res224_clip-kinetics710-pre_8xb32-u8_kinetics400-rgb.log) |
+| 8 | short-side 320 | UniFormerV2-L/14\* | clip-kinetics710 | 88.7 | 98.1 | 88.8 | 98.1 | 88.7 | 98.1 | 4 clips x 3 crop | 0.7T | 354M | [config](/configs/recognition/uniformerv2/uniformerv2-large-p14-res224_clip-kinetics710-pre_u8_kinetics400-rgb.py) | [ckpt](https://download.openmmlab.com/mmaction/v1.0/recognition/uniformerv2/kinetics400/uniformerv2-large-p14-res224_clip-kinetics710-pre_u8_kinetics400-rgb_20221219-972ea063.pth) | - |
+| 16 | short-side 320 | UniFormerV2-L/14\* | clip-kinetics710 | 89.0 | 98.2 | 89.1 | 98.2 | 89.0 | 98.2 | 4 clips x 3 crop | 1.3T | 354M | [config](/configs/recognition/uniformerv2/uniformerv2-large-p14-res224_clip-kinetics710-pre_u16_kinetics400-rgb.py) | [ckpt](https://download.openmmlab.com/mmaction/v1.0/recognition/uniformerv2/kinetics400/uniformerv2-large-p14-res224_clip-kinetics710-pre_u16_kinetics400-rgb_20221219-6dc86d05.pth) | - |
+| 32 | short-side 320 | UniFormerV2-L/14\* | clip-kinetics710 | 89.3 | 98.2 | 89.3 | 98.2 | 89.4 | 98.2 | 2 clips x 3 crop | 2.7T | 354M | [config](/configs/recognition/uniformerv2/uniformerv2-large-p14-res224_clip-kinetics710-pre_u32_kinetics400-rgb.py) | [ckpt](https://download.openmmlab.com/mmaction/v1.0/recognition/uniformerv2/kinetics400/uniformerv2-large-p14-res224_clip-kinetics710-pre_u32_kinetics400-rgb_20221219-56a46f64.pth) | - |
+| 32 | short-side 320 | UniFormerV2-L/14@336\* | clip-kinetics710 | 89.5 | 98.4 | 89.7 | 98.3 | 89.5 | 98.4 | 2 clips x 3 crop | 6.3T | 354M | [config](/configs/recognition/uniformerv2/uniformerv2-large-p14-res336_clip-kinetics710-pre_u32_kinetics400-rgb.py) | [ckpt](https://download.openmmlab.com/mmaction/v1.0/recognition/uniformerv2/kinetics400/uniformerv2-large-p14-res336_clip-kinetics710-pre_u32_kinetics400-rgb_20221219-1dd7650f.pth) | - |
+
+### Kinetics-600
+
+| ๅๅ้ๆ ทๅธงๆฐ | ๅ่พจ็ | ไธปๅนฒ็ฝ็ป | ้ข่ฎญ็ป | top1 ๅ็กฎ็ | top5 ๅ็กฎ็ | [ๅ่ๆ็ฎ](https://github.com/OpenGVLab/UniFormerV2/blob/main/MODEL_ZOO.md) top1 ๅ็กฎ็ | [ๅ่ๆ็ฎ](https://github.com/OpenGVLab/UniFormerV2/blob/main/MODEL_ZOO.md) top5 ๅ็กฎ็ | mm-Kinetics top1 ๅ็กฎ็ | mm-Kinetics top5 ๅ็กฎ็ | ๆต่ฏๆนๆก | FLOPs | ๅๆฐ้ | ้
็ฝฎๆไปถ | ckpt | log |
+| :----------: | :----: | :--------------------: | :--------------: | :---------: | :---------: | :-------------------------------------------------------------------------------------: | :-------------------------------------------------------------------------------------: | :---------------------: | :---------------------: | :--------------: | :---: | :----: | :---------------------------------------------------------------------------------------------------------------------: | :----------------------------------------------------------------------------------------------------------------------------------------------------------------------------------------------------------------------------------------------------: | :---------------------------------------------------------------------------------------------------------------------------------------------------------------------------------------------------------------------------------: |
+| 8 | Raw | UniFormerV2-B/16 | clip-kinetics710 | - | - | 86.1 | 97.2 | 86.4 | 97.3 | 4 clips x 3 crop | 0.1T | 115M | [config](/configs/recognition/uniformerv2/uniformerv2-base-p16-res224_clip-kinetics710-pre_8xb32-u8_kinetics600-rgb.py) | [ckpt](https://download.openmmlab.com/mmaction/v1.0/recognition/uniformerv2/uniformerv2-base-p16-res224_clip-kinetics710-pre_8xb32-u8_kinetics600-rgb/uniformerv2-base-p16-res224_clip-kinetics710-pre_8xb32-u8_kinetics600-rgb_20230313-544f06f0.pth) | [log](https://download.openmmlab.com/mmaction/v1.0/recognition/uniformerv2/uniformerv2-base-p16-res224_clip-kinetics710-pre_8xb32-u8_kinetics600-rgb/uniformerv2-base-p16-res224_clip-kinetics710-pre_8xb32-u8_kinetics600-rgb.log) |
+| 8 | Raw | UniFormerV2-L/14\* | clip-kinetics710 | 89.0 | 98.3 | 89.0 | 98.2 | 87.5 | 98.0 | 4 clips x 3 crop | 0.7T | 354M | [config](/configs/recognition/uniformerv2/uniformerv2-large-p14-res224_clip-kinetics710-pre_u8_kinetics600-rgb.py) | [ckpt](https://download.openmmlab.com/mmaction/v1.0/recognition/uniformerv2/kinetics600/uniformerv2-large-p14-res224_clip-kinetics710-pre_u8_kinetics600-rgb_20221219-cf88e4c2.pth) | - |
+| 16 | Raw | UniFormerV2-L/14\* | clip-kinetics710 | 89.4 | 98.3 | 89.4 | 98.3 | 87.8 | 98.0 | 4 clips x 3 crop | 1.3T | 354M | [config](/configs/recognition/uniformerv2/uniformerv2-large-p14-res224_clip-kinetics710-pre_u16_kinetics600-rgb.py) | [ckpt](https://download.openmmlab.com/mmaction/v1.0/recognition/uniformerv2/kinetics600/uniformerv2-large-p14-res224_clip-kinetics710-pre_u16_kinetics600-rgb_20221219-38ff0e3e.pth) | - |
+| 32 | Raw | UniFormerV2-L/14\* | clip-kinetics710 | 89.2 | 98.3 | 89.5 | 98.3 | 87.7 | 98.1 | 2 clips x 3 crop | 2.7T | 354M | [config](/configs/recognition/uniformerv2/uniformerv2-large-p14-res224_clip-kinetics710-pre_u32_kinetics600-rgb.py) | [ckpt](https://download.openmmlab.com/mmaction/v1.0/recognition/uniformerv2/kinetics600/uniformerv2-large-p14-res224_clip-kinetics710-pre_u32_kinetics600-rgb_20221219-d450d071.pth) | - |
+| 32 | Raw | UniFormerV2-L/14@336\* | clip-kinetics710 | 89.8 | 98.5 | 89.9 | 98.5 | 88.8 | 98.3 | 2 clips x 3 crop | 6.3T | 354M | [config](/configs/recognition/uniformerv2/uniformerv2-large-p14-res336_clip-kinetics710-pre_u32_kinetics600-rgb.py) | [ckpt](https://download.openmmlab.com/mmaction/v1.0/recognition/uniformerv2/kinetics600/uniformerv2-large-p14-res336_clip-kinetics710-pre_u32_kinetics600-rgb_20221219-f984f5d2.pth) | - |
+
+### Kinetics-700
+
+| ๅๅ้ๆ ทๅธงๆฐ | ๅ่พจ็ | ไธปๅนฒ็ฝ็ป | ้ข่ฎญ็ป | top1 ๅ็กฎ็ | top5 ๅ็กฎ็ | [ๅ่ๆ็ฎ](https://github.com/OpenGVLab/UniFormerV2/blob/main/MODEL_ZOO.md) top1 ๅ็กฎ็ | [ๅ่ๆ็ฎ](https://github.com/OpenGVLab/UniFormerV2/blob/main/MODEL_ZOO.md) top5 ๅ็กฎ็ | mm-Kinetics top1 ๅ็กฎ็ | mm-Kinetics top5 ๅ็กฎ็ | ๆต่ฏๆนๆก | FLOPs | ๅๆฐ้ | ้
็ฝฎๆไปถ | ckpt | log |
+| :----------: | :----: | :--------------------: | :--------------: | :---------: | :---------: | :-------------------------------------------------------------------------------------: | :-------------------------------------------------------------------------------------: | :---------------------: | :---------------------: | :--------------: | :---: | :----: | :---------------------------------------------------------------------------------------------------------------------: | :----------------------------------------------------------------------------------------------------------------------------------------------------------------------------------------------------------------------------------------------------: | :---------------------------------------------------------------------------------------------------------------------------------------------------------------------------------------------------------------------------------: |
+| 8 | Raw | UniFormerV2-B/16 | clip | - | - | 75.8 | 92.8 | 75.9 | 92.9 | 4 clips x 3 crop | 0.1T | 115M | [config](/configs/recognition/uniformerv2/uniformerv2-base-p16-res224_clip-kinetics710-pre_8xb32-u8_kinetics700-rgb.py) | [ckpt](https://download.openmmlab.com/mmaction/v1.0/recognition/uniformerv2/uniformerv2-base-p16-res224_clip_8xb32-u8_kinetics700-rgb/uniformerv2-base-p16-res224_clip_8xb32-u8_kinetics700-rgb_20230313-f02e48ad.pth) | [log](https://download.openmmlab.com/mmaction/v1.0/recognition/uniformerv2/uniformerv2-base-p16-res224_clip_8xb32-u8_kinetics700-rgb/uniformerv2-base-p16-res224_clip_8xb32-u8_kinetics700-rgb.log) |
+| 8 | Raw | UniFormerV2-B/16 | clip-kinetics710 | - | - | 76.3 | 92.7 | 76.3 | 92.9 | 4 clips x 3 crop | 0.1T | 115M | [config](/configs/recognition/uniformerv2/uniformerv2-base-p16-res224_clip-kinetics710-pre_8xb32-u8_kinetics700-rgb.py) | [ckpt](https://download.openmmlab.com/mmaction/v1.0/recognition/uniformerv2/uniformerv2-base-p16-res224_clip-kinetics710-pre_8xb32-u8_kinetics700-rgb/uniformerv2-base-p16-res224_clip-kinetics710-pre_8xb32-u8_kinetics700-rgb_20230313-69070837.pth) | [log](https://download.openmmlab.com/mmaction/v1.0/recognition/uniformerv2/uniformerv2-base-p16-res224_clip-kinetics710-pre_8xb32-u8_kinetics700-rgb/uniformerv2-base-p16-res224_clip-kinetics710-pre_8xb32-u8_kinetics700-rgb.log) |
+| 8 | Raw | UniFormerV2-L/14\* | clip-kinetics710 | 80.8 | 95.2 | 80.8 | 95.4 | 79.4 | 94.8 | 4 clips x 3 crop | 0.7T | 354M | [config](/configs/recognition/uniformerv2/uniformerv2-large-p14-res224_clip-kinetics710-pre_u8_kinetics700-rgb.py) | [ckpt](https://download.openmmlab.com/mmaction/v1.0/recognition/uniformerv2/kinetics700/uniformerv2-large-p14-res224_clip-kinetics710-pre_u8_kinetics700-rgb_20221219-bfb9f401.pth) | - |
+| 16 | Raw | UniFormerV2-L/14\* | clip-kinetics710 | 81.2 | 95.6 | 81.2 | 95.6 | 79.2 | 95.0 | 4 clips x 3 crop | 1.3T | 354M | [config](/configs/recognition/uniformerv2/uniformerv2-large-p14-res224_clip-kinetics710-pre_u16_kinetics700-rgb.py) | [ckpt](https://download.openmmlab.com/mmaction/v1.0/recognition/uniformerv2/kinetics700/uniformerv2-large-p14-res224_clip-kinetics710-pre_u16_kinetics700-rgb_20221219-745209d2.pth) | - |
+| 32 | Raw | UniFormerV2-L/14\* | clip-kinetics710 | 81.4 | 95.7 | 81.5 | 95.7 | 79.8 | 95.3 | 2 clips x 3 crop | 2.7T | 354M | [config](/configs/recognition/uniformerv2/uniformerv2-large-p14-res224_clip-kinetics710-pre_u32_kinetics700-rgb.py) | [ckpt](https://download.openmmlab.com/mmaction/v1.0/recognition/uniformerv2/kinetics700/uniformerv2-large-p14-res224_clip-kinetics710-pre_u32_kinetics700-rgb_20221219-eebe7056.pth) | - |
+| 32 | Raw | UniFormerV2-L/14@336\* | clip-kinetics710 | 82.1 | 96.0 | 82.1 | 96.1 | 80.6 | 95.6 | 2 clips x 3 crop | 6.3T | 354M | [config](/configs/recognition/uniformerv2/uniformerv2-large-p14-res336_clip-kinetics710-pre_u32_kinetics700-rgb.py) | [ckpt](https://download.openmmlab.com/mmaction/v1.0/recognition/uniformerv2/kinetics700/uniformerv2-large-p14-res336_clip-kinetics710-pre_u32_kinetics700-rgb_20221219-95cf9046.pth) | - |
+
+### MiTv1
+
+| ๅๅ้ๆ ทๅธงๆฐ | ๅ่พจ็ | ไธปๅนฒ็ฝ็ป | ้ข่ฎญ็ป | top1 ๅ็กฎ็ | top5 ๅ็กฎ็ | [ๅ่ๆ็ฎ](https://github.com/OpenGVLab/UniFormerV2/blob/main/MODEL_ZOO.md) top1 ๅ็กฎ็ | [ๅ่ๆ็ฎ](https://github.com/OpenGVLab/UniFormerV2/blob/main/MODEL_ZOO.md) top5 ๅ็กฎ็ | ๆต่ฏๆนๆก | FLOPs | ๅๆฐ้ | config | ckpt | log |
+| :----------: | :----: | :--------------------: | :--------------------------: | :---------: | :---------: | :-------------------------------------------------------------------------------------: | :-------------------------------------------------------------------------------------: | :--------------: | :---: | :----: | :------------------------------------------------------------------------------------------------------------------------------: | :----------------------------------------------------------------------------------------------------------------------------------------------------------------------------------------------------------------------------------------------------------------------: | :---------------------------------------------------------------------------------------------------------------------------------------------------------------------------------------------------------------------------------------------------: |
+| 8 | Raw | UniFormerV2-B/16 | clip-kinetics710-kinetics400 | 42.3 | 71.5 | 42.6 | 71.7 | 4 clips x 3 crop | 0.1T | 115M | [config](/configs/recognition/uniformerv2/uniformerv2-base-p16-res224_clip-kinetics710-kinetics-k400-pre_16xb32-u8_mitv1-rgb.py) | [ckpt](https://download.openmmlab.com/mmaction/v1.0/recognition/uniformerv2/uniformerv2-base-p16-res224_clip-kinetics710-kinetics-k400-pre_16xb32-u8_mitv1-rgb/uniformerv2-base-p16-res224_clip-kinetics710-kinetics-k400-pre_16xb32-u8_mitv1-rgb_20230313-a6f4a567.pth) | [log](https://download.openmmlab.com/mmaction/v1.0/recognition/uniformerv2/uniformerv2-base-p16-res224_clip-kinetics710-kinetics-k400-pre_16xb32-u8_mitv1-rgb/uniformerv2-base-p16-res224_clip-kinetics710-kinetics-k400-pre_16xb32-u8_mitv1-rgb.log) |
+| 8 | Raw | UniFormerV2-L/14\* | clip-kinetics710-kinetics400 | 47.0 | 76.1 | 47.0 | 76.1 | 4 clips x 3 crop | 0.7T | 354M | [config](/configs/recognition/uniformerv2/uniformerv2-large-p16-res224_clip-kinetics710-kinetics-k400-pre_u8_mitv1-rgb.py) | [ckpt](https://download.openmmlab.com/mmaction/v1.0/recognition/uniformerv2/mitv1/uniformerv2-large-p16-res224_clip-kinetics710-kinetics-k400-pre_u8_mitv1-rgb_20221219-882c0598.pth) | - |
+| 8 | Raw | UniFormerV2-L/14@336\* | clip-kinetics710-kinetics400 | 47.7 | 76.8 | 47.8 | 76.0 | 4 clips x 3 crop | 1.6T | 354M | [config](/configs/recognition/uniformerv2/uniformerv2-large-p16-res336_clip-kinetics710-kinetics-k400-pre_u8_mitv1-rgb.py) | [ckpt](https://download.openmmlab.com/mmaction/v1.0/recognition/uniformerv2/mitv1/uniformerv2-large-p16-res336_clip-kinetics710-kinetics-k400-pre_u8_mitv1-rgb_20221219-9020986e.pth) | - |
+
+### Kinetics-710
+
+| ๅๅ้ๆ ทๅธงๆฐ | ๅ่พจ็ | ไธปๅนฒ็ฝ็ป | ้ข่ฎญ็ป | top1 ๅ็กฎ็ | top5 ๅ็กฎ็ | config | ckpt | log |
+| :----------: | :----: | :--------------------: | :----: | :---------: | :---------: | :-------------------------------------------: | :------------------------------------------: | :-----------------------------------------: |
+| 8 | Raw | UniFormerV2-B/16\* | clip | 78.9 | 94.2 | [config](/configs/recognition/uniformerv2/uniformerv2-base-p16-res224_clip-pre_u8_kinetics710-rgb.py) | [ckpt](https://download.openmmlab.com/mmaction/v1.0/recognition/uniformerv2/uniformerv2-base-p16-res224_clip-pre_u8_kinetics710-rgb/uniformerv2-base-p16-res224_clip-pre_u8_kinetics710-rgb_20230612-63cdbad9.pth) | [log](https://download.openmmlab.com/mmaction/v1.0/recognition/uniformerv2/uniformerv2-base-p16-res224_clip-pre_u8_kinetics710-rgb/uniformerv2-base-p16-res224_clip-pre_u8_kinetics710-rgb.log) |
+| 8 | Raw | UniFormerV2-L/14\* | clip | - | - | [config](/configs/recognition/uniformerv2/uniformerv2-large-p14-res224_clip-pre_u8_kinetics710-rgb.py) | [ckpt](https://download.openmmlab.com/mmaction/v1.0/recognition/uniformerv2/kinetics710/uniformerv2-large-p14-res224_clip-pre_u8_kinetics710-rgb_20230612-d002a407.pth) | - |
+| 8 | Raw | UniFormerV2-L/14@336\* | clip | - | - | [config](/configs/recognition/uniformerv2/uniformerv2-large-p14-res336_clip-pre_u8_kinetics710-rgb.py) | [ckpt](https://download.openmmlab.com/mmaction/v1.0/recognition/uniformerv2/kinetics710/uniformerv2-large-p14-res336_clip-pre_u8_kinetics710-rgb_20230612-d723ddc1.pth) | - |
+
+ไปฅไธๅธฆๆ * ็ๆจกๅๆฏ่ฟ็งป่ช[UniFormerV2ไปๅบ](https://github.com/OpenGVLab/UniFormerV2/blob/main/MODEL_ZOO.md)๏ผๅนถๅจๆไปฌ็ๆฐๆฎไธ่ฟ่กไบๆต่ฏใ็ฑไบ็ฎๅ้ๅถ๏ผๆไปฌไป
ๆฏๆๅบ็กๆจกๅ๏ผๅณ UniFormerV2-B/16๏ผ่ฎญ็ป้
็ฝฎ็ๅฏ้ ๆงใ
+
+1. "ๅ่ๆ็ฎ"ๅไธญ็ๆฐๅผๆฏๅๅงไปๅบ็็ปๆใ
+2. `top1/5ๅ็กฎ็` ไธญ็ๆฐๅผๆฏๅจไธๅๅงไปๅบ็ธๅ็ๆฐๆฎไธ่ฟ่กๆต่ฏๅพๅฐ็๏ผๅนถไธๅ็ฑปๅจ-ๆ ็ญพๆ ๅฐไธ [UniFormerV2](https://drive.google.com/drive/folders/17VB-XdF3Kfr9ORmnGyXCxTMs86n0L4QL)ไธ่ดใ
+3. "mm-Kinetics" ๅไธญ็ๆฐๅผๆฏๅจ MMAction2 ๆๆ็ Kinetics ๆฐๆฎ้ไธ่ฟ่ก็ๆต่ฏ็ปๆ๏ผๅ
ถไป MMAction2 ๆจกๅไนไฝฟ็จไบ่ฏฅๆฐๆฎ้ใ็ฑไบๅไธช็ๆฌ็ Kinetics ๆฐๆฎ้ไน้ดๅญๅจๅทฎๅผ๏ผ`top1/5ๅ็กฎ็` ๅ `mm-Kinetics top1/5ๅ็กฎ็` ไน้ดๅญๅจไธไบๅทฎๅผใไธบไบไธๅ
ถไปๆจกๅ่ฟ่กๅ
ฌๅนณๆฏ่พ๏ผๆไปฌๅจ่ฟ้ๆฅๅไบไธคไธช็ปๆใ่ฏทๆณจๆ๏ผๆไปฌๅชๆฅๅๆจๆญ็ปๆ๏ผๅ ไธบ UniFormer ๅๅ
ถไปๆจกๅ็่ฎญ็ป้ไธๅ๏ผๆไปฅ่ฏฅ็ปๆไฝไบๅจไฝ่
็ๆฌไธๆต่ฏ็็ปๆใ
+4. ็ฑไบ Kinetics-400/600/700 ็ๅๅงๆจกๅ้็จไบไธๅ็[ๆ ็ญพๆไปถ](https://drive.google.com/drive/folders/17VB-XdF3Kfr9ORmnGyXCxTMs86n0L4QL)๏ผๆไปฌๆ นๆฎๆ ็ญพๅ็งฐ็ฎๅๆ ๅฐๆ้ใKinetics-400/600/700็ๆฐๆ ็ญพๆ ๅฐๅฏไปฅๅจ[่ฟ้](/tools/data/kinetics)ๆพๅฐใ
+5. ็ฑไบ [SlowFast](https://github.com/facebookresearch/SlowFast)ๅ MMAction2 ไน้ดๅญๅจไธไบๅทฎๅผ๏ผๅฎไปฌ็ๆง่ฝไน้ดๅญๅจไธไบๅทฎ่ทใ
+6. ๆไปฌไฝฟ็จKinetics-710่ฟ่ก้ข่ฎญ็ป๏ผ่ฟๆๅฉไบๆ้ซๅ
ถไปๆฐๆฎ้็ๆง่ฝใไฝ ๅฏไปฅๅจ[่ฎบๆ](https://arxiv.org/abs/2211.09552)ไธญๆพๅฐๆดๅค็ป่ใๆไปฌ่ฟๆ นๆฎ Kinetics-710 ็ๆจกๅๆ้่ฟ่กไบๆ้ๆ ๅฐ๏ผไฝ ๅฏไปฅๅจ[่ฟ้](/tools/data/kinetics710/label_map_k710.txt)ๆพๅฐๆ ็ญพๆ ๅฐใ
+
+ๆๅ
ณๆฐๆฎๅๅค็ๆดๅค่ฏฆ็ปไฟกๆฏ๏ผๅฏไปฅๅ่ไปฅไธ้พๆฅ๏ผ
+
+- [ๅๅค Kinetics ๆฐๆฎ้](/tools/data/kinetics/README_zh-CN.md)
+- [ๅๅค MIT ๆฐๆฎ้](/tools/data/mit/README_zh-CN.md)
+
+## ๅฆไฝๆต่ฏ
+
+ๆจๅฏไปฅไฝฟ็จไปฅไธๅฝไปคๆฅๆต่ฏๆจกๅ๏ผ
+
+```shell
+python tools/test.py ${CONFIG_FILE} ${CHECKPOINT_FILE} [optional arguments]
+```
+
+็คบไพ๏ผๅจ Kinetics-400 ๆฐๆฎ้ไธๆต่ฏ UniFormerV2-B/16 ๆจกๅ๏ผๅนถๅฐ็ปๆ่ฝฌๅจๅฐไธไธชpklๆไปถไธญใ
+
+```shell
+python tools/test.py configs/recognition/uniformerv2/uniformerv2-base-p16-res224_clip-kinetics710-pre_u8_kinetics400-rgb.py \
+ checkpoints/SOME_CHECKPOINT.pth --dump result.pkl
+```
+
+ๆๅ
ณๆดๅค่ฏฆ็ปไฟกๆฏ๏ผ่ฏทๅ่[่ฎญ็ปๅๆต่ฏๆ็จ](/docs/zh_cn/user_guides/train_test.md)ไธญ็**ๆต่ฏ**้จๅใ
diff --git a/configs/recognition_audio/resnet/README.md b/configs/recognition_audio/resnet/README.md
index f6386e313f..3a58b201c7 100644
--- a/configs/recognition_audio/resnet/README.md
+++ b/configs/recognition_audio/resnet/README.md
@@ -8,7 +8,7 @@
-We present Audiovisual SlowFast Networks, an architecture for integrated audiovisual perception. AVSlowFast has Slow and Fast visual pathways that are deeply inte- grated with a Faster Audio pathway to model vision and sound in a unified representation. We fuse audio and vi- sual features at multiple layers, enabling audio to con- tribute to the formation of hierarchical audiovisual con- cepts. To overcome training difficulties that arise from dif- ferent learning dynamics for audio and visual modalities, we introduce DropPathway, which randomly drops the Au- dio pathway during training as an effective regularization technique. Inspired by prior studies in neuroscience, we perform hierarchical audiovisual synchronization to learn joint audiovisual features. We report state-of-the-art results on six video action classification and detection datasets, perform detailed ablation studies, and show the gener- alization of AVSlowFast to learn self-supervised audiovi- sual features. Code will be made available at: https: //github.com/facebookresearch/SlowFast.
+We present Audiovisual SlowFast Networks, an architecture for integrated audiovisual perception. AVSlowFast has Slow and Fast visual pathways that are deeply integrated with a Faster Audio pathway to model vision and sound in a unified representation. We fuse audio and visual features at multiple layers, enabling audio to contribute to the formation of hierarchical audiovisual concepts. To overcome training difficulties that arise from different learning dynamics for audio and visual modalities, we introduce DropPathway, which randomly drops the Au- dio pathway during training as an effective regularization technique. Inspired by prior studies in neuroscience, we perform hierarchical audiovisual synchronization to learn joint audiovisual features. We report state-of-the-art results on six video action classification and detection datasets, perform detailed ablation studies, and show the generalization of AVSlowFast to learn self-supervised audiovisual features.
@@ -20,16 +20,9 @@ We present Audiovisual SlowFast Networks, an architecture for integrated audiovi
### Kinetics-400
-| frame sampling strategy | n_fft | gpus | backbone | pretrain | top1 acc | top5 acc | testing protocol | gpu_mem(M) | config | ckpt | log |
-| :---------------------: | :---: | :--: | :------: | :------: | :------: | :------: | :--------------: | :--------: | :------------------------------------: | :----------------------------------: | :----------------------------------: |
-| 64x1x1 | 1024 | 8 | Resnet18 | None | 19.7 | 35.75 | 10 clips | 1897 | [config](/configs/recognition_audio/resnet/tsn_r18_8xb320-64x1x1-100e_kinetics400-audio-feature.py) | [ckpt](https://download.openmmlab.com/mmaction/v1.0/recognition_audio/resnet/tsn_r18_8xb320-64x1x1-100e_kinetics400-audio-feature/tsn_r18_8xb320-64x1x1-100e_kinetics400-audio-feature_20201012-bf34df6c.pth) | [log](https://download.openmmlab.com/mmaction/v1.0/recognition_audio/resnet/tsn_r18_8xb320-64x1x1-100e_kinetics400-audio-feature/tsn_r18_8xb320-64x1x1-100e_kinetics400-audio-feature.log) |
-
-1. The **gpus** indicates the number of gpus we used to get the checkpoint. It is noteworthy that the configs we provide are used for 8 gpus as default.
- According to the [Linear Scaling Rule](https://arxiv.org/abs/1706.02677), you may set the learning rate proportional to the batch size if you use different GPUs or videos per GPU,
- e.g., lr=0.01 for 4 GPUs x 2 video/gpu and lr=0.08 for 16 GPUs x 4 video/gpu.
-2. The validation set of Kinetics400 we used consists of 19796 videos. These videos are available at [Kinetics400-Validation](https://mycuhk-my.sharepoint.com/:u:/g/personal/1155136485_link_cuhk_edu_hk/EbXw2WX94J1Hunyt3MWNDJUBz-nHvQYhO9pvKqm6g39PMA?e=a9QldB). The corresponding [data list](https://download.openmmlab.com/mmaction/dataset/k400_val/kinetics_val_list.txt) (each line is of the format 'video_id, num_frames, label_index') and the [label map](https://download.openmmlab.com/mmaction/dataset/k400_val/kinetics_class2ind.txt) are also available.
-
-For more details on data preparation, you can refer to `Prepare audio` in [Data Preparation Tutorial](/docs/en/user_guides/prepare_dataset.md).
+| frame sampling strategy | n_fft | gpus | backbone | pretrain | top1 acc | top5 acc | testing protocol | FLOPs | params | config | ckpt | log |
+| :---------------------: | :---: | :--: | :------: | :------: | :------: | :------: | :--------------: | :---: | :----: | :------------------------------------: | :----------------------------------: | :---------------------------------: |
+| 64x1x1 | 1024 | 8 | Resnet18 | None | 13.7 | 27.3 | 1 clips | 0.37G | 11.4M | [config](/configs/recognition_audio/resnet/tsn_r18_8xb320-64x1x1-100e_kinetics400-audio-feature.py) | [ckpt](https://download.openmmlab.com/mmaction/v1.0/recognition_audio/resnet/tsn_r18_8xb320-64x1x1-100e_kinetics400-audio-feature/tsn_r18_8xb320-64x1x1-100e_kinetics400-audio-feature_20230702-e4642fb0.pth) | [log](https://download.openmmlab.com/mmaction/v1.0/recognition_audio/resnet/tsn_r18_8xb320-64x1x1-100e_kinetics400-audio-feature/tsn_r18_8xb320-64x1x1-100e_kinetics400-audio-feature.log) |
## Train
@@ -43,7 +36,7 @@ Example: train ResNet model on Kinetics-400 audio dataset in a deterministic opt
```shell
python tools/train.py configs/recognition_audio/resnet/tsn_r18_8xb320-64x1x1-100e_kinetics400-audio-feature.py \
- --cfg-options randomness.seed=0 randomness.deterministic=True
+ --seed 0 --deterministic
```
For more details, you can refer to the **Training** part in the [Training and Test Tutorial](/docs/en/user_guides/train_test.md).
diff --git a/configs/recognition_audio/resnet/metafile.yml b/configs/recognition_audio/resnet/metafile.yml
index f82d234e9a..26f495cd9e 100644
--- a/configs/recognition_audio/resnet/metafile.yml
+++ b/configs/recognition_audio/resnet/metafile.yml
@@ -11,16 +11,20 @@ Models:
In Collection: Audio
Metadata:
Architecture: ResNet18
+ Batch Size: 320
+ Epochs: 100
+ FLOPs: 0.37G
+ Parameters: 11.4M
Pretrained: None
+ n_fft: 1024
Training Data: Kinetics-400
Training Resources: 8 GPUs
- n_fft: 1024
Modality: Audio
Results:
- Dataset: Kinetics-400
Task: Action Recognition
Metrics:
- Top 1 Accuracy: 19.7
- Top 5 Accuracy: 35.75
+ Top 1 Accuracy: 13.7
+ Top 5 Accuracy: 27.3
Training Log: https://download.openmmlab.com/mmaction/v1.0/recognition_audio/resnet/tsn_r18_8xb320-64x1x1-100e_kinetics400-audio-feature/tsn_r18_8xb320-64x1x1-100e_kinetics400-audio-feature.log
- Weights: https://download.openmmlab.com/mmaction/v1.0/recognition_audio/resnet/tsn_r18_8xb320-64x1x1-100e_kinetics400-audio-feature/tsn_r18_8xb320-64x1x1-100e_kinetics400-audio-feature_20201012-bf34df6c.pth
+ Weights: https://download.openmmlab.com/mmaction/v1.0/recognition_audio/resnet/tsn_r18_8xb320-64x1x1-100e_kinetics400-audio-feature/tsn_r18_8xb320-64x1x1-100e_kinetics400-audio-feature_20230702-e4642fb0.pth
diff --git a/configs/recognition_audio/resnet/tsn_r18_8xb320-64x1x1-100e_kinetics400-audio-feature.py b/configs/recognition_audio/resnet/tsn_r18_8xb320-64x1x1-100e_kinetics400-audio-feature.py
index 8a37ab5bad..9b00c34796 100644
--- a/configs/recognition_audio/resnet/tsn_r18_8xb320-64x1x1-100e_kinetics400-audio-feature.py
+++ b/configs/recognition_audio/resnet/tsn_r18_8xb320-64x1x1-100e_kinetics400-audio-feature.py
@@ -1,14 +1,24 @@
-_base_ = [
- '../../_base_/models/tsn_r18_audio.py', '../../_base_/default_runtime.py'
-]
+_base_ = '../../_base_/default_runtime.py'
+
+# model settings
+model = dict(
+ type='RecognizerAudio',
+ backbone=dict(type='ResNet', depth=18, in_channels=1, norm_eval=False),
+ cls_head=dict(
+ type='TSNAudioHead',
+ num_classes=400,
+ in_channels=512,
+ dropout_ratio=0.5,
+ init_std=0.01,
+ average_clips='prob'))
# dataset settings
dataset_type = 'AudioDataset'
-data_root = 'data/kinetics400/audio_features_train'
-data_root_val = 'data/kinetics400/audio_features_val'
-ann_file_train = 'data/kinetics400/kinetics400_val_list_audio_features.txt'
-ann_file_val = 'data/kinetics400/kinetics400_val_list_audio_features.txt'
-ann_file_test = 'data/kinetics400/kinetics400_val_list_audio_features.txt'
+data_root = 'data/kinetics400'
+ann_file_train = 'kinetics400_train_list_audio_features.txt'
+ann_file_val = 'kinetics400_val_list_audio_features.txt'
+ann_file_test = 'kinetics400_val_list_audio_features.txt'
+
train_pipeline = [
dict(type='LoadAudioFeature'),
dict(type='SampleFrames', clip_len=64, frame_interval=1, num_clips=1),
@@ -28,53 +38,42 @@
dict(type='FormatAudioShape', input_format='NCTF'),
dict(type='PackActionInputs')
]
-test_pipeline = [
- dict(type='LoadAudioFeature'),
- dict(
- type='SampleFrames',
- clip_len=64,
- frame_interval=1,
- num_clips=10,
- test_mode=True),
- dict(type='AudioFeatureSelector'),
- dict(type='FormatAudioShape', input_format='NCTF'),
- dict(type='PackActionInputs')
-]
+test_pipeline = val_pipeline
train_dataloader = dict(
batch_size=320,
- num_workers=2,
+ num_workers=4,
persistent_workers=True,
sampler=dict(type='DefaultSampler', shuffle=True),
dataset=dict(
type=dataset_type,
ann_file=ann_file_train,
- data_prefix=dict(audio=data_root_val),
- suffix='.npy',
- pipeline=train_pipeline))
+ pipeline=train_pipeline,
+ data_root=data_root,
+ data_prefix=dict(audio='audio_features_train')))
val_dataloader = dict(
batch_size=320,
- num_workers=2,
+ num_workers=4,
persistent_workers=True,
sampler=dict(type='DefaultSampler', shuffle=False),
dataset=dict(
type=dataset_type,
ann_file=ann_file_val,
pipeline=val_pipeline,
- data_prefix=dict(audio=data_root_val),
- suffix='.npy',
+ data_root=data_root,
+ data_prefix=dict(audio='audio_features_val'),
test_mode=True))
test_dataloader = dict(
batch_size=1,
- num_workers=2,
+ num_workers=4,
persistent_workers=True,
sampler=dict(type='DefaultSampler', shuffle=False),
dataset=dict(
type=dataset_type,
ann_file=ann_file_test,
pipeline=test_pipeline,
- data_prefix=dict(audio=data_root_val),
- suffix='.npy',
+ data_root=data_root,
+ data_prefix=dict(audio='audio_features_val'),
test_mode=True))
val_evaluator = dict(type='AccMetric')
@@ -90,8 +89,7 @@
]
optim_wrapper = dict(
- optimizer=dict(type='SGD', lr=0.1, momentum=0.9, weight_decay=0.0001),
+ optimizer=dict(type='SGD', lr=0.2, momentum=0.9, weight_decay=0.0001),
clip_grad=dict(max_norm=40, norm_type=2))
-default_hooks = dict(
- checkpoint=dict(max_keep_ckpts=3, interval=5), logger=dict(interval=20))
+default_hooks = dict(checkpoint=dict(max_keep_ckpts=3, interval=5))
diff --git a/configs/recognition_audio/resnet/tsn_r18_8xb320-64x1x1-100e_kinetics400-audio.py b/configs/recognition_audio/resnet/tsn_r18_8xb320-64x1x1-100e_kinetics400-audio.py
deleted file mode 100644
index ccae1b251f..0000000000
--- a/configs/recognition_audio/resnet/tsn_r18_8xb320-64x1x1-100e_kinetics400-audio.py
+++ /dev/null
@@ -1,100 +0,0 @@
-_base_ = [
- '../../_base_/models/tsn_r18_audio.py', '../../_base_/default_runtime.py'
-]
-
-# dataset settings
-dataset_type = 'AudioDataset'
-data_root = 'data/kinetics400/audios_train'
-data_root_val = 'data/kinetics400/audios_val'
-ann_file_train = 'data/kinetics400/kinetics400_train_list_audios.txt'
-ann_file_val = 'data/kinetics400/kinetics400_val_list_audios.txt'
-ann_file_test = 'data/kinetics400/kinetics400_val_list_audios.txt'
-train_pipeline = [
- dict(type='AudioDecodeInit'),
- dict(type='SampleFrames', clip_len=64, frame_interval=1, num_clips=1),
- dict(type='AudioDecode'),
- dict(type='AudioAmplify', ratio=1.5),
- dict(type='MelSpectrogram'),
- dict(type='FormatAudioShape', input_format='NCTF'),
- dict(type='PackActionInputs')
-]
-val_pipeline = [
- dict(type='AudioDecodeInit'),
- dict(
- type='SampleFrames',
- clip_len=64,
- frame_interval=1,
- num_clips=1,
- test_mode=True),
- dict(type='AudioDecode'),
- dict(type='AudioAmplify', ratio=1.5),
- dict(type='MelSpectrogram'),
- dict(type='FormatAudioShape', input_format='NCTF'),
- dict(type='PackActionInputs')
-]
-test_pipeline = [
- dict(type='AudioDecodeInit'),
- dict(
- type='SampleFrames',
- clip_len=64,
- frame_interval=1,
- num_clips=10,
- test_mode=True),
- dict(type='AudioDecode'),
- dict(type='AudioAmplify', ratio=1.5),
- dict(type='MelSpectrogram'),
- dict(type='FormatAudioShape', input_format='NCTF'),
- dict(type='PackActionInputs')
-]
-
-train_dataloader = dict(
- batch_size=320,
- num_workers=2,
- persistent_workers=True,
- sampler=dict(type='DefaultSampler', shuffle=True),
- dataset=dict(
- type=dataset_type,
- ann_file=ann_file_train,
- data_prefix=dict(audio=data_root),
- pipeline=train_pipeline))
-val_dataloader = dict(
- batch_size=320,
- num_workers=2,
- persistent_workers=True,
- sampler=dict(type='DefaultSampler', shuffle=False),
- dataset=dict(
- type=dataset_type,
- ann_file=ann_file_val,
- pipeline=val_pipeline,
- data_prefix=dict(audio=data_root_val),
- test_mode=True))
-test_dataloader = dict(
- batch_size=1,
- num_workers=2,
- persistent_workers=True,
- sampler=dict(type='DefaultSampler', shuffle=False),
- dataset=dict(
- type=dataset_type,
- ann_file=ann_file_test,
- pipeline=test_pipeline,
- data_prefix=dict(audio=data_root_val),
- test_mode=True))
-
-val_evaluator = dict(type='AccMetric')
-test_evaluator = val_evaluator
-
-train_cfg = dict(
- type='EpochBasedTrainLoop', max_epochs=100, val_begin=1, val_interval=5)
-val_cfg = dict(type='ValLoop')
-test_cfg = dict(type='TestLoop')
-
-param_scheduler = [
- dict(type='CosineAnnealingLR', eta_min=0, T_max=100, by_epoch=True)
-]
-
-optim_wrapper = dict(
- optimizer=dict(type='SGD', lr=0.1, momentum=0.9, weight_decay=0.0001),
- clip_grad=dict(max_norm=40, norm_type=2))
-
-default_hooks = dict(
- checkpoint=dict(max_keep_ckpts=3, interval=5), logger=dict(interval=20))
diff --git a/configs/skeleton/posec3d/README.md b/configs/skeleton/posec3d/README.md
index 93b526e5ac..2546706e57 100644
--- a/configs/skeleton/posec3d/README.md
+++ b/configs/skeleton/posec3d/README.md
@@ -79,10 +79,13 @@ Human skeleton, as a compact representation of human action, has received increa
| :---------------------: | :------------: | :--: | :----------: | :------: | :--------------: | :---: | :----: | :-------------------------------------: | :-----------------------------------: | :----------------------------------: |
| uniform 48 | keypoint | 8 | SlowOnly-R50 | 69.6 | 10 clips | 14.6G | 3.0M | [config](/configs/skeleton/posec3d/slowonly_kinetics400-pretrained-r50_8xb16-u48-120e_hmdb51-split1-keypoint.py) | [ckpt](https://download.openmmlab.com/mmaction/v1.0/skeleton/posec3d/slowonly_kinetics400-pretrained-r50_8xb16-u48-120e_hmdb51-split1-keypoint/slowonly_kinetics400-pretrained-r50_8xb16-u48-120e_hmdb51-split1-keypoint_20220815-17eaa484.pth) | [log](https://download.openmmlab.com/mmaction/v1.0/skeleton/posec3d/slowonly_kinetics400-pretrained-r50_8xb16-u48-120e_hmdb51-split1-keypoint/slowonly_kinetics400-pretrained-r50_8xb16-u48-120e_hmdb51-split1-keypoint.log) |
-1. The **gpus** indicates the number of gpu we used to get the checkpoint. It is noteworthy that the configs we provide are used for 8 gpus as default.
- According to the [Linear Scaling Rule](https://arxiv.org/abs/1706.02677), you may set the learning rate proportional to the batch size if you use different GPUs or videos per GPU,
- e.g., lr=0.01 for 8 GPUs x 8 videos/gpu and lr=0.04 for 16 GPUs x 16 videos/gpu.
-2. You can follow the guide in [Preparing Skeleton Dataset](/tools/data/skeleton/README.md) to obtain skeleton annotations used in the above configs.
+# Kinetics400
+
+| frame sampling strategy | pseudo heatmap | gpus | backbone | top1 acc | testing protocol | FLOPs | params | config | ckpt | log |
+| :---------------------: | :------------: | :--: | :----------: | :------: | :--------------: | :---: | :----: | :-------------------------------------: | :-----------------------------------: | :----------------------------------: |
+| uniform 48 | keypoint | 8 | SlowOnly-R50 | 47.4 | 10 clips | 19.1G | 3.2M | [config](/configs/skeleton/posec3d/slowonly_r50_8xb32-u48-240e_k400-keypoint.py) | [ckpt](https://download.openmmlab.com/mmaction/v1.0/skeleton/posec3d/slowonly_r50_8xb32-u48-240e_k400-keypoint/slowonly_r50_8xb32-u48-240e_k400-keypoint_20230731-7f498b55.pth) | [log](https://download.openmmlab.com/mmaction/v1.0/skeleton/posec3d/slowonly_r50_8xb32-u48-240e_k400-keypoint/slowonly_r50_8xb32-u48-240e_k400-keypoint.log) |
+
+You can follow the guide in [Preparing Skeleton Dataset](/tools/data/skeleton/README.md) to obtain skeleton annotations used in the above configs.
## Train
@@ -96,7 +99,7 @@ Example: train PoseC3D model on FineGYM dataset in a deterministic option.
```shell
python tools/train.py configs/skeleton/posec3d/slowonly_r50_8xb16-u48-240e_gym-keypoint.py \
- --cfg-options randomness.seed=0 randomness.deterministic=True
+ --seed=0 --deterministic
```
For training with your custom dataset, you can refer to [Custom Dataset Training](/configs/skeleton/posec3d/custom_dataset_training.md).
diff --git a/configs/skeleton/posec3d/metafile.yml b/configs/skeleton/posec3d/metafile.yml
index b949a23d47..8a5b58bf76 100644
--- a/configs/skeleton/posec3d/metafile.yml
+++ b/configs/skeleton/posec3d/metafile.yml
@@ -125,3 +125,23 @@ Models:
Top 1 Accuracy: 86.8
Training Log: https://download.openmmlab.com/mmaction/v1.0/skeleton/posec3d/slowonly_kinetics400-pretrained-r50_8xb16-u48-120e_ucf101-split1-keypoint/slowonly_kinetics400-pretrained-r50_8xb16-u48-120e_ucf101-split1-keypoint.log
Weights: https://download.openmmlab.com/mmaction/v1.0/skeleton/posec3d/slowonly_kinetics400-pretrained-r50_8xb16-u48-120e_ucf101-split1-keypoint/slowonly_kinetics400-pretrained-r50_8xb16-u48-120e_ucf101-split1-keypoint_20220815-9972260d.pth
+
+ - Name: slowonly_r50_8xb32-u48-240e_k400-keypoint
+ Config: configs/skeleton/posec3d/slowonly_r50_8xb32-u48-240e_k400-keypoint.py
+ In Collection: PoseC3D
+ Metadata:
+ Architecture: SlowOnly-R50
+ Batch Size: 32
+ Epochs: 240
+ FLOPs: 19.1G
+ Parameters: 3.2M
+ Training Data: Kinetic400
+ Training Resources: 8 GPUs
+ pseudo heatmap: keypoint
+ Results:
+ - Dataset: Kinetic400
+ Task: Skeleton-based Action Recognition
+ Metrics:
+ Top 1 Accuracy: 47.4
+ Training Log: https://download.openmmlab.com/mmaction/v1.0/skeleton/posec3d/slowonly_r50_8xb32-u48-240e_k400-keypoint/slowonly_r50_8xb32-u48-240e_k400-keypoint.log
+ Weights: https://download.openmmlab.com/mmaction/v1.0/skeleton/posec3d/slowonly_r50_8xb32-u48-240e_k400-keypoint/slowonly_r50_8xb32-u48-240e_k400-keypoint_20230731-7f498b55.pth
diff --git a/configs/skeleton/posec3d/slowonly_r50_8xb32-u48-240e_k400-keypoint.py b/configs/skeleton/posec3d/slowonly_r50_8xb32-u48-240e_k400-keypoint.py
new file mode 100644
index 0000000000..320d37898a
--- /dev/null
+++ b/configs/skeleton/posec3d/slowonly_r50_8xb32-u48-240e_k400-keypoint.py
@@ -0,0 +1,146 @@
+_base_ = '../../_base_/default_runtime.py'
+
+model = dict(
+ type='Recognizer3D',
+ backbone=dict(
+ type='ResNet3dSlowOnly',
+ depth=50,
+ pretrained=None,
+ in_channels=17,
+ base_channels=32,
+ num_stages=3,
+ out_indices=(2, ),
+ stage_blocks=(3, 4, 6),
+ conv1_stride_s=1,
+ pool1_stride_s=1,
+ inflate=(0, 1, 1),
+ spatial_strides=(2, 2, 2),
+ temporal_strides=(1, 1, 2),
+ dilations=(1, 1, 1)),
+ cls_head=dict(
+ type='I3DHead',
+ in_channels=512,
+ num_classes=400,
+ spatial_type='avg',
+ dropout_ratio=0.5,
+ average_clips='prob'))
+
+dataset_type = 'PoseDataset'
+data_root = 'data/skeleton/kpfiles'
+ann_file = 'data/skeleton/k400_2d.pkl'
+left_kp = [1, 3, 5, 7, 9, 11, 13, 15]
+right_kp = [2, 4, 6, 8, 10, 12, 14, 16]
+box_thr = 0.5
+valid_ratio = 0.0
+
+train_pipeline = [
+ dict(type='DecompressPose', squeeze=True),
+ dict(type='UniformSampleFrames', clip_len=48),
+ dict(type='PoseDecode'),
+ dict(type='PoseCompact', hw_ratio=1., allow_imgpad=True),
+ dict(type='Resize', scale=(-1, 64)),
+ dict(type='RandomResizedCrop', area_range=(0.56, 1.0)),
+ dict(type='Resize', scale=(56, 56), keep_ratio=False),
+ dict(type='Flip', flip_ratio=0.5, left_kp=left_kp, right_kp=right_kp),
+ dict(type='GeneratePoseTarget', with_kp=True, with_limb=False),
+ dict(type='FormatShape', input_format='NCTHW_Heatmap'),
+ dict(type='PackActionInputs')
+]
+val_pipeline = [
+ dict(type='DecompressPose', squeeze=True),
+ dict(type='UniformSampleFrames', clip_len=48, num_clips=1, test_mode=True),
+ dict(type='PoseDecode'),
+ dict(type='PoseCompact', hw_ratio=1., allow_imgpad=True),
+ dict(type='Resize', scale=(64, 64), keep_ratio=False),
+ dict(type='GeneratePoseTarget', with_kp=True, with_limb=False),
+ dict(type='FormatShape', input_format='NCTHW_Heatmap'),
+ dict(type='PackActionInputs')
+]
+test_pipeline = [
+ dict(type='DecompressPose', squeeze=True),
+ dict(
+ type='UniformSampleFrames', clip_len=48, num_clips=10, test_mode=True),
+ dict(type='PoseDecode'),
+ dict(type='PoseCompact', hw_ratio=1., allow_imgpad=True),
+ dict(type='Resize', scale=(64, 64), keep_ratio=False),
+ dict(
+ type='GeneratePoseTarget',
+ with_kp=True,
+ with_limb=False,
+ double=True,
+ left_kp=left_kp,
+ right_kp=right_kp),
+ dict(type='FormatShape', input_format='NCTHW_Heatmap'),
+ dict(type='PackActionInputs')
+]
+
+train_dataloader = dict(
+ batch_size=32,
+ num_workers=8,
+ persistent_workers=True,
+ sampler=dict(type='DefaultSampler', shuffle=True),
+ dataset=dict(
+ type='RepeatDataset',
+ times=10,
+ dataset=dict(
+ type=dataset_type,
+ ann_file=ann_file,
+ split='train',
+ pipeline=train_pipeline,
+ box_thr=box_thr,
+ data_prefix=dict(skeleton=data_root))))
+val_dataloader = dict(
+ batch_size=32,
+ num_workers=8,
+ persistent_workers=True,
+ sampler=dict(type='DefaultSampler', shuffle=False),
+ dataset=dict(
+ type=dataset_type,
+ ann_file=ann_file,
+ split='val',
+ pipeline=val_pipeline,
+ box_thr=box_thr,
+ data_prefix=dict(skeleton=data_root),
+ test_mode=True))
+test_dataloader = dict(
+ batch_size=1,
+ num_workers=8,
+ persistent_workers=True,
+ sampler=dict(type='DefaultSampler', shuffle=False),
+ dataset=dict(
+ type=dataset_type,
+ ann_file=ann_file,
+ split='val',
+ pipeline=test_pipeline,
+ box_thr=box_thr,
+ data_prefix=dict(skeleton=data_root),
+ test_mode=True))
+
+val_evaluator = dict(type='AccMetric')
+test_evaluator = val_evaluator
+
+train_cfg = dict(
+ type='EpochBasedTrainLoop', max_epochs=24, val_begin=1, val_interval=1)
+val_cfg = dict(type='ValLoop')
+test_cfg = dict(type='TestLoop')
+
+param_scheduler = [
+ dict(
+ type='CosineAnnealingLR',
+ eta_min=0,
+ T_max=24,
+ by_epoch=True,
+ convert_to_iter_based=True)
+]
+
+optim_wrapper = dict(
+ optimizer=dict(type='SGD', lr=0.4, momentum=0.9, weight_decay=0.0001),
+ clip_grad=dict(max_norm=40, norm_type=2))
+
+default_hooks = dict(checkpoint=dict(max_keep_ckpts=3))
+
+# Default setting for scaling LR automatically
+# - `enable` means enable scaling LR automatically
+# or not by default.
+# - `base_batch_size` = (8 GPUs) x (32 samples per GPU).
+auto_scale_lr = dict(enable=False, base_batch_size=256)
diff --git a/demo/README.md b/demo/README.md
index 5b36c4a0d0..40ad34315f 100644
--- a/demo/README.md
+++ b/demo/README.md
@@ -12,6 +12,7 @@
- [SpatioTemporal Action Detection Video Demo](#spatiotemporal-action-detection-video-demo): A demo script to predict the spatiotemporal action detection result using a single video.
- [SpatioTemporal Action Detection ONNX Video Demo](#spatiotemporal-action-detection-onnx-video-demo): A demo script to predict the SpatioTemporal Action Detection result using the onnx file instead of building the PyTorch models.
- [Inferencer Demo](#inferencer): A demo script to implement fast predict for video analysis tasks based on unified inferencer interface.
+- [Audio Demo](#audio-demo): A demo script to predict the recognition result using a single audio file.
- [Video Structuralize Demo](#video-structuralize-demo): A demo script to predict the skeleton-based and rgb-based action recognition and spatio-temporal action detection result using a single video.
## Modify configs through script arguments
@@ -588,6 +589,34 @@ Assume that you are located at `$MMACTION2`.
--label-file tools/data/kinetics/label_map_k400.txt
```
+## Audio Demo
+
+Demo script to predict the audio-based action recognition using a single audio feature.
+
+The script [`extract_audio.py`](/tools/data/extract_audio.py) can be used to extract audios from videos and the script [`build_audio_features.py`](/tools/data/build_audio_features.py) can be used to extract the audio features.
+
+```shell
+python demo/demo_audio.py ${CONFIG_FILE} ${CHECKPOINT_FILE} ${AUDIO_FILE} {LABEL_FILE} [--device ${DEVICE}]
+```
+
+Optional arguments:
+
+- `DEVICE`: Type of device to run the demo. Allowed values are cuda devices like `cuda:0` or `cpu`. If not specified, it will be set to `cuda:0`.
+
+Examples:
+
+Assume that you are located at `$MMACTION2` and have already downloaded the checkpoints to the directory `checkpoints/`,
+or use checkpoint url from `configs/` to directly load the corresponding checkpoint, which will be automatically saved in `$HOME/.cache/torch/checkpoints`.
+
+1. Recognize an audio file as input by using a tsn model on cuda by default.
+
+ ```shell
+ python demo/demo_audio.py \
+ configs/recognition_audio/resnet/tsn_r18_8xb320-64x1x1-100e_kinetics400-audio-feature.py \
+ https://download.openmmlab.com/mmaction/v1.0/recognition_audio/resnet/tsn_r18_8xb320-64x1x1-100e_kinetics400-audio-feature/tsn_r18_8xb320-64x1x1-100e_kinetics400-audio-feature_20230702-e4642fb0.pth \
+ audio_feature.npy tools/data/kinetics/label_map_k400.txt
+ ```
+
## Video Structuralize Demo
We provide a demo script to predict the skeleton-based and rgb-based action recognition and spatio-temporal action detection result using a single video.
diff --git a/demo/demo.ipynb b/demo/demo.ipynb
index ebcf2ff538..9d5e958864 100644
--- a/demo/demo.ipynb
+++ b/demo/demo.ipynb
@@ -70,7 +70,7 @@
"label = '../tools/data/kinetics/label_map_k400.txt'\n",
"results = inference_recognizer(model, video)\n",
"\n",
- "pred_scores = results.pred_scores.item.tolist()\n",
+ "pred_scores = results.pred_score.tolist()\n",
"score_tuples = tuple(zip(range(len(pred_scores)), pred_scores))\n",
"score_sorted = sorted(score_tuples, key=itemgetter(1), reverse=True)\n",
"top5_label = score_sorted[:5]\n",
diff --git a/demo/demo.py b/demo/demo.py
index 6c9b5db5a5..d2ec044a04 100644
--- a/demo/demo.py
+++ b/demo/demo.py
@@ -119,7 +119,7 @@ def main():
model = init_recognizer(cfg, args.checkpoint, device=args.device)
pred_result = inference_recognizer(model, args.video)
- pred_scores = pred_result.pred_scores.item.tolist()
+ pred_scores = pred_result.pred_score.tolist()
score_tuples = tuple(zip(range(len(pred_scores)), pred_scores))
score_sorted = sorted(score_tuples, key=itemgetter(1), reverse=True)
top5_label = score_sorted[:5]
diff --git a/demo/demo_audio.py b/demo/demo_audio.py
new file mode 100644
index 0000000000..c874813f1f
--- /dev/null
+++ b/demo/demo_audio.py
@@ -0,0 +1,57 @@
+# Copyright (c) OpenMMLab. All rights reserved.
+import argparse
+from operator import itemgetter
+
+import torch
+from mmengine import Config, DictAction
+
+from mmaction.apis import inference_recognizer, init_recognizer
+
+
+def parse_args():
+ parser = argparse.ArgumentParser(description='MMAction2 demo')
+ parser.add_argument('config', help='test config file path')
+ parser.add_argument('checkpoint', help='checkpoint file/url')
+ parser.add_argument('audio', help='audio file')
+ parser.add_argument('label', help='label file')
+ parser.add_argument(
+ '--cfg-options',
+ nargs='+',
+ action=DictAction,
+ default={},
+ help='override some settings in the used config, the key-value pair '
+ 'in xxx=yyy format will be merged into config file. For example, '
+ "'--cfg-options model.backbone.depth=18 model.backbone.with_cp=True'")
+ parser.add_argument(
+ '--device', type=str, default='cuda:0', help='CPU/CUDA device option')
+ args = parser.parse_args()
+ return args
+
+
+def main():
+ args = parse_args()
+ device = torch.device(args.device)
+ cfg = Config.fromfile(args.config)
+ cfg.merge_from_dict(args.cfg_options)
+ model = init_recognizer(cfg, args.checkpoint, device=device)
+
+ if not args.audio.endswith('.npy'):
+ raise NotImplementedError('Demo works on extracted audio features')
+ pred_result = inference_recognizer(model, args.audio)
+
+ pred_scores = pred_result.pred_score.tolist()
+ score_tuples = tuple(zip(range(len(pred_scores)), pred_scores))
+ score_sorted = sorted(score_tuples, key=itemgetter(1), reverse=True)
+ top5_label = score_sorted[:5]
+
+ labels = open(args.label).readlines()
+ labels = [x.strip() for x in labels]
+ results = [(labels[k[0]], k[1]) for k in top5_label]
+
+ print('The top-5 labels with corresponding scores are:')
+ for result in results:
+ print(f'{result[0]}: ', result[1])
+
+
+if __name__ == '__main__':
+ main()
diff --git a/demo/demo_skeleton.py b/demo/demo_skeleton.py
index 7a162ef468..19245b6540 100644
--- a/demo/demo_skeleton.py
+++ b/demo/demo_skeleton.py
@@ -152,7 +152,7 @@ def main():
model = init_recognizer(config, args.checkpoint, args.device)
result = inference_skeleton(model, pose_results, (h, w))
- max_pred_index = result.pred_scores.item.argmax().item()
+ max_pred_index = result.pred_score.argmax().item()
label_map = [x.strip() for x in open(args.label_map).readlines()]
action_label = label_map[max_pred_index]
diff --git a/demo/demo_video_structuralize.py b/demo/demo_video_structuralize.py
index 805dda7e14..85784efbf5 100644
--- a/demo/demo_video_structuralize.py
+++ b/demo/demo_video_structuralize.py
@@ -373,7 +373,7 @@ def skeleton_based_action_recognition(args, pose_results, h, w):
skeleton_model = init_recognizer(
skeleton_config, args.skeleton_checkpoint, device=args.device)
result = inference_skeleton(skeleton_model, pose_results, (h, w))
- action_idx = result.pred_scores.item.argmax().item()
+ action_idx = result.pred_score.argmax().item()
return label_map[action_idx]
@@ -382,7 +382,7 @@ def rgb_based_action_recognition(args):
rgb_config.model.backbone.pretrained = None
rgb_model = init_recognizer(rgb_config, args.rgb_checkpoint, args.device)
action_results = inference_recognizer(rgb_model, args.video)
- rgb_action_result = action_results.pred_scores.item.argmax().item()
+ rgb_action_result = action_results.pred_score.argmax().item()
label_map = [x.strip() for x in open(args.label_map).readlines()]
return label_map[rgb_action_result]
@@ -460,7 +460,7 @@ def skeleton_based_stdet(args, label_map, human_detections, pose_results,
output = inference_recognizer(skeleton_stdet_model, fake_anno)
# for multi-label recognition
- score = output.pred_scores.item.tolist()
+ score = output.pred_score.tolist()
for k in range(len(score)): # 81
if k not in label_map:
continue
diff --git a/demo/fuse/bone.pkl b/demo/fuse/bone.pkl
index a5cc72b3a1..21d311924c 100644
Binary files a/demo/fuse/bone.pkl and b/demo/fuse/bone.pkl differ
diff --git a/demo/fuse/joint.pkl b/demo/fuse/joint.pkl
index 1259a508ce..96d023b336 100644
Binary files a/demo/fuse/joint.pkl and b/demo/fuse/joint.pkl differ
diff --git a/demo/long_video_demo.py b/demo/long_video_demo.py
index bb7e51a234..eea03348ff 100644
--- a/demo/long_video_demo.py
+++ b/demo/long_video_demo.py
@@ -216,7 +216,7 @@ def inference(model, data, args, frame_queue):
result = inference_recognizer(
model, cur_data, test_pipeline=args.test_pipeline)
- scores = result.pred_scores.item.tolist()
+ scores = result.pred_score.tolist()
if args.stride > 0:
pred_stride = int(args.sample_length * args.stride)
diff --git a/demo/mmaction2_tutorial.ipynb b/demo/mmaction2_tutorial.ipynb
index 1a9d6ec70e..4d24a04d5e 100644
--- a/demo/mmaction2_tutorial.ipynb
+++ b/demo/mmaction2_tutorial.ipynb
@@ -1,1936 +1,1936 @@
{
- "cells": [
- {
- "cell_type": "markdown",
- "metadata": {
- "id": "VcjSRFELVbNk"
- },
- "source": [
- "# MMAction2 Tutorial\n",
- "\n",
- "Welcome to MMAction2! This is the official colab tutorial for using MMAction2. In this tutorial, you will learn\n",
- "- Perform inference with a MMAction2 recognizer.\n",
- "- Train a new recognizer with a new dataset.\n",
- "\n",
- "\n",
- "Let's start!"
- ]
- },
- {
- "cell_type": "markdown",
- "metadata": {
- "id": "7LqHGkGEVqpm"
- },
- "source": [
- "## Install MMAction2"
- ]
- },
- {
- "cell_type": "code",
- "execution_count": 2,
- "metadata": {
- "colab": {
- "base_uri": "https://localhost:8080/"
- },
- "id": "Bf8PpPXtVvmg",
- "outputId": "9d3f4594-f151-4ee9-a19b-09f8a439ac04"
- },
- "outputs": [
- {
- "output_type": "stream",
- "name": "stdout",
- "text": [
- "nvcc: NVIDIA (R) Cuda compiler driver\n",
- "Copyright (c) 2005-2022 NVIDIA Corporation\n",
- "Built on Wed_Sep_21_10:33:58_PDT_2022\n",
- "Cuda compilation tools, release 11.8, V11.8.89\n",
- "Build cuda_11.8.r11.8/compiler.31833905_0\n",
- "gcc (Ubuntu 9.4.0-1ubuntu1~20.04.1) 9.4.0\n",
- "Copyright (C) 2019 Free Software Foundation, Inc.\n",
- "This is free software; see the source for copying conditions. There is NO\n",
- "warranty; not even for MERCHANTABILITY or FITNESS FOR A PARTICULAR PURPOSE.\n",
- "\n"
- ]
- }
- ],
- "source": [
- "# Check nvcc version\n",
- "!nvcc -V\n",
- "# Check GCC version\n",
- "!gcc --version"
- ]
- },
- {
- "cell_type": "code",
- "execution_count": 3,
- "metadata": {
- "id": "ZPwKGzqydnb2",
- "outputId": "27506fa7-48a2-4fe0-d377-56f940dafec4",
- "colab": {
- "base_uri": "https://localhost:8080/"
- }
- },
- "outputs": [
- {
- "output_type": "stream",
- "name": "stdout",
- "text": [
- "Looking in indexes: https://download.pytorch.org/whl/cu118, https://us-python.pkg.dev/colab-wheels/public/simple/\n",
- "Requirement already satisfied: torch in /usr/local/lib/python3.10/dist-packages (2.0.0+cu118)\n",
- "Requirement already satisfied: torchvision in /usr/local/lib/python3.10/dist-packages (0.15.1+cu118)\n",
- "Requirement already satisfied: torchaudio in /usr/local/lib/python3.10/dist-packages (2.0.1+cu118)\n",
- "Requirement already satisfied: filelock in /usr/local/lib/python3.10/dist-packages (from torch) (3.12.0)\n",
- "Requirement already satisfied: typing-extensions in /usr/local/lib/python3.10/dist-packages (from torch) (4.5.0)\n",
- "Requirement already satisfied: sympy in /usr/local/lib/python3.10/dist-packages (from torch) (1.11.1)\n",
- "Requirement already satisfied: networkx in /usr/local/lib/python3.10/dist-packages (from torch) (3.1)\n",
- "Requirement already satisfied: jinja2 in /usr/local/lib/python3.10/dist-packages (from torch) (3.1.2)\n",
- "Requirement already satisfied: triton==2.0.0 in /usr/local/lib/python3.10/dist-packages (from torch) (2.0.0)\n",
- "Requirement already satisfied: cmake in /usr/local/lib/python3.10/dist-packages (from triton==2.0.0->torch) (3.25.2)\n",
- "Requirement already satisfied: lit in /usr/local/lib/python3.10/dist-packages (from triton==2.0.0->torch) (16.0.3)\n",
- "Requirement already satisfied: numpy in /usr/local/lib/python3.10/dist-packages (from torchvision) (1.22.4)\n",
- "Requirement already satisfied: requests in /usr/local/lib/python3.10/dist-packages (from torchvision) (2.27.1)\n",
- "Requirement already satisfied: pillow!=8.3.*,>=5.3.0 in /usr/local/lib/python3.10/dist-packages (from torchvision) (8.4.0)\n",
- "Requirement already satisfied: MarkupSafe>=2.0 in /usr/local/lib/python3.10/dist-packages (from jinja2->torch) (2.1.2)\n",
- "Requirement already satisfied: urllib3<1.27,>=1.21.1 in /usr/local/lib/python3.10/dist-packages (from requests->torchvision) (1.26.15)\n",
- "Requirement already satisfied: certifi>=2017.4.17 in /usr/local/lib/python3.10/dist-packages (from requests->torchvision) (2022.12.7)\n",
- "Requirement already satisfied: charset-normalizer~=2.0.0 in /usr/local/lib/python3.10/dist-packages (from requests->torchvision) (2.0.12)\n",
- "Requirement already satisfied: idna<4,>=2.5 in /usr/local/lib/python3.10/dist-packages (from requests->torchvision) (3.4)\n",
- "Requirement already satisfied: mpmath>=0.19 in /usr/local/lib/python3.10/dist-packages (from sympy->torch) (1.3.0)\n"
- ]
- }
- ],
- "source": [
- "# install dependencies: (if your colab has CUDA 11.8)\n",
- "%pip install torch torchvision torchaudio --index-url https://download.pytorch.org/whl/cu118"
- ]
- },
- {
- "cell_type": "code",
- "execution_count": 4,
- "metadata": {
- "colab": {
- "base_uri": "https://localhost:8080/"
- },
- "id": "5PAJ4ArzV5Ry",
- "outputId": "eb8539a0-9524-4c48-f3e1-0b013ce0d344"
- },
- "outputs": [
- {
- "output_type": "stream",
- "name": "stdout",
- "text": [
- "Looking in indexes: https://pypi.org/simple, https://us-python.pkg.dev/colab-wheels/public/simple/\n",
- "Collecting openmim\n",
- " Downloading openmim-0.3.7-py2.py3-none-any.whl (51 kB)\n",
- "\u001b[2K \u001b[90mโโโโโโโโโโโโโโโโโโโโโโโโโโโโโโโโโโโโโโโโ\u001b[0m \u001b[32m51.3/51.3 kB\u001b[0m \u001b[31m4.0 MB/s\u001b[0m eta \u001b[36m0:00:00\u001b[0m\n",
- "\u001b[?25hRequirement already satisfied: Click in /usr/local/lib/python3.10/dist-packages (from openmim) (8.1.3)\n",
- "Collecting colorama (from openmim)\n",
- " Downloading colorama-0.4.6-py2.py3-none-any.whl (25 kB)\n",
- "Collecting model-index (from openmim)\n",
- " Downloading model_index-0.1.11-py3-none-any.whl (34 kB)\n",
- "Requirement already satisfied: pandas in /usr/local/lib/python3.10/dist-packages (from openmim) (1.5.3)\n",
- "Requirement already satisfied: pip>=19.3 in /usr/local/lib/python3.10/dist-packages (from openmim) (23.1.2)\n",
- "Requirement already satisfied: requests in /usr/local/lib/python3.10/dist-packages (from openmim) (2.27.1)\n",
- "Requirement already satisfied: rich in /usr/local/lib/python3.10/dist-packages (from openmim) (13.3.4)\n",
- "Requirement already satisfied: tabulate in /usr/local/lib/python3.10/dist-packages (from openmim) (0.8.10)\n",
- "Requirement already satisfied: pyyaml in /usr/local/lib/python3.10/dist-packages (from model-index->openmim) (6.0)\n",
- "Requirement already satisfied: markdown in /usr/local/lib/python3.10/dist-packages (from model-index->openmim) (3.4.3)\n",
- "Collecting ordered-set (from model-index->openmim)\n",
- " Downloading ordered_set-4.1.0-py3-none-any.whl (7.6 kB)\n",
- "Requirement already satisfied: python-dateutil>=2.8.1 in /usr/local/lib/python3.10/dist-packages (from pandas->openmim) (2.8.2)\n",
- "Requirement already satisfied: pytz>=2020.1 in /usr/local/lib/python3.10/dist-packages (from pandas->openmim) (2022.7.1)\n",
- "Requirement already satisfied: numpy>=1.21.0 in /usr/local/lib/python3.10/dist-packages (from pandas->openmim) (1.22.4)\n",
- "Requirement already satisfied: urllib3<1.27,>=1.21.1 in /usr/local/lib/python3.10/dist-packages (from requests->openmim) (1.26.15)\n",
- "Requirement already satisfied: certifi>=2017.4.17 in /usr/local/lib/python3.10/dist-packages (from requests->openmim) (2022.12.7)\n",
- "Requirement already satisfied: charset-normalizer~=2.0.0 in /usr/local/lib/python3.10/dist-packages (from requests->openmim) (2.0.12)\n",
- "Requirement already satisfied: idna<4,>=2.5 in /usr/local/lib/python3.10/dist-packages (from requests->openmim) (3.4)\n",
- "Requirement already satisfied: markdown-it-py<3.0.0,>=2.2.0 in /usr/local/lib/python3.10/dist-packages (from rich->openmim) (2.2.0)\n",
- "Requirement already satisfied: pygments<3.0.0,>=2.13.0 in /usr/local/lib/python3.10/dist-packages (from rich->openmim) (2.14.0)\n",
- "Requirement already satisfied: mdurl~=0.1 in /usr/local/lib/python3.10/dist-packages (from markdown-it-py<3.0.0,>=2.2.0->rich->openmim) (0.1.2)\n",
- "Requirement already satisfied: six>=1.5 in /usr/local/lib/python3.10/dist-packages (from python-dateutil>=2.8.1->pandas->openmim) (1.16.0)\n",
- "Installing collected packages: ordered-set, colorama, model-index, openmim\n",
- "Successfully installed colorama-0.4.6 model-index-0.1.11 openmim-0.3.7 ordered-set-4.1.0\n",
- "Looking in indexes: https://pypi.org/simple, https://us-python.pkg.dev/colab-wheels/public/simple/\n",
- "Looking in links: https://download.openmmlab.com/mmcv/dist/cu118/torch2.0.0/index.html\n",
- "Collecting mmengine\n",
- " Downloading mmengine-0.7.3-py3-none-any.whl (372 kB)\n",
- "\u001b[2K \u001b[90mโโโโโโโโโโโโโโโโโโโโโโโโโโโโโโโโโโโโโโ\u001b[0m \u001b[32m372.1/372.1 kB\u001b[0m \u001b[31m20.5 MB/s\u001b[0m eta \u001b[36m0:00:00\u001b[0m\n",
- "\u001b[?25hCollecting addict (from mmengine)\n",
- " Downloading addict-2.4.0-py3-none-any.whl (3.8 kB)\n",
- "Requirement already satisfied: matplotlib in /usr/local/lib/python3.10/dist-packages (from mmengine) (3.7.1)\n",
- "Requirement already satisfied: numpy in /usr/local/lib/python3.10/dist-packages (from mmengine) (1.22.4)\n",
- "Requirement already satisfied: pyyaml in /usr/local/lib/python3.10/dist-packages (from mmengine) (6.0)\n",
- "Requirement already satisfied: rich in /usr/local/lib/python3.10/dist-packages (from mmengine) (13.3.4)\n",
- "Requirement already satisfied: termcolor in /usr/local/lib/python3.10/dist-packages (from mmengine) (2.3.0)\n",
- "Collecting yapf (from mmengine)\n",
- " Downloading yapf-0.33.0-py2.py3-none-any.whl (200 kB)\n",
- "\u001b[2K \u001b[90mโโโโโโโโโโโโโโโโโโโโโโโโโโโโโโโโโโโโโโ\u001b[0m \u001b[32m200.9/200.9 kB\u001b[0m \u001b[31m21.0 MB/s\u001b[0m eta \u001b[36m0:00:00\u001b[0m\n",
- "\u001b[?25hRequirement already satisfied: opencv-python>=3 in /usr/local/lib/python3.10/dist-packages (from mmengine) (4.7.0.72)\n",
- "Requirement already satisfied: contourpy>=1.0.1 in /usr/local/lib/python3.10/dist-packages (from matplotlib->mmengine) (1.0.7)\n",
- "Requirement already satisfied: cycler>=0.10 in /usr/local/lib/python3.10/dist-packages (from matplotlib->mmengine) (0.11.0)\n",
- "Requirement already satisfied: fonttools>=4.22.0 in /usr/local/lib/python3.10/dist-packages (from matplotlib->mmengine) (4.39.3)\n",
- "Requirement already satisfied: kiwisolver>=1.0.1 in /usr/local/lib/python3.10/dist-packages (from matplotlib->mmengine) (1.4.4)\n",
- "Requirement already satisfied: packaging>=20.0 in /usr/local/lib/python3.10/dist-packages (from matplotlib->mmengine) (23.1)\n",
- "Requirement already satisfied: pillow>=6.2.0 in /usr/local/lib/python3.10/dist-packages (from matplotlib->mmengine) (8.4.0)\n",
- "Requirement already satisfied: pyparsing>=2.3.1 in /usr/local/lib/python3.10/dist-packages (from matplotlib->mmengine) (3.0.9)\n",
- "Requirement already satisfied: python-dateutil>=2.7 in /usr/local/lib/python3.10/dist-packages (from matplotlib->mmengine) (2.8.2)\n",
- "Requirement already satisfied: markdown-it-py<3.0.0,>=2.2.0 in /usr/local/lib/python3.10/dist-packages (from rich->mmengine) (2.2.0)\n",
- "Requirement already satisfied: pygments<3.0.0,>=2.13.0 in /usr/local/lib/python3.10/dist-packages (from rich->mmengine) (2.14.0)\n",
- "Requirement already satisfied: tomli>=2.0.1 in /usr/local/lib/python3.10/dist-packages (from yapf->mmengine) (2.0.1)\n",
- "Requirement already satisfied: mdurl~=0.1 in /usr/local/lib/python3.10/dist-packages (from markdown-it-py<3.0.0,>=2.2.0->rich->mmengine) (0.1.2)\n",
- "Requirement already satisfied: six>=1.5 in /usr/local/lib/python3.10/dist-packages (from python-dateutil>=2.7->matplotlib->mmengine) (1.16.0)\n",
- "Installing collected packages: addict, yapf, mmengine\n",
- "Successfully installed addict-2.4.0 mmengine-0.7.3 yapf-0.33.0\n",
- "Looking in indexes: https://pypi.org/simple, https://us-python.pkg.dev/colab-wheels/public/simple/\n",
- "Looking in links: https://download.openmmlab.com/mmcv/dist/cu118/torch2.0.0/index.html\n",
- "Collecting mmcv>=2.0.0\n",
- " Downloading https://download.openmmlab.com/mmcv/dist/cu118/torch2.0.0/mmcv-2.0.0-cp310-cp310-manylinux1_x86_64.whl (74.4 MB)\n",
- "\u001b[2K \u001b[90mโโโโโโโโโโโโโโโโโโโโโโโโโโโโโโโโโโโโโโโโ\u001b[0m \u001b[32m74.4/74.4 MB\u001b[0m \u001b[31m9.7 MB/s\u001b[0m eta \u001b[36m0:00:00\u001b[0m\n",
- "\u001b[?25hRequirement already satisfied: addict in /usr/local/lib/python3.10/dist-packages (from mmcv>=2.0.0) (2.4.0)\n",
- "Requirement already satisfied: mmengine>=0.2.0 in /usr/local/lib/python3.10/dist-packages (from mmcv>=2.0.0) (0.7.3)\n",
- "Requirement already satisfied: numpy in /usr/local/lib/python3.10/dist-packages (from mmcv>=2.0.0) (1.22.4)\n",
- "Requirement already satisfied: packaging in /usr/local/lib/python3.10/dist-packages (from mmcv>=2.0.0) (23.1)\n",
- "Requirement already satisfied: Pillow in /usr/local/lib/python3.10/dist-packages (from mmcv>=2.0.0) (8.4.0)\n",
- "Requirement already satisfied: pyyaml in /usr/local/lib/python3.10/dist-packages (from mmcv>=2.0.0) (6.0)\n",
- "Requirement already satisfied: yapf in /usr/local/lib/python3.10/dist-packages (from mmcv>=2.0.0) (0.33.0)\n",
- "Requirement already satisfied: opencv-python>=3 in /usr/local/lib/python3.10/dist-packages (from mmcv>=2.0.0) (4.7.0.72)\n",
- "Requirement already satisfied: matplotlib in /usr/local/lib/python3.10/dist-packages (from mmengine>=0.2.0->mmcv>=2.0.0) (3.7.1)\n",
- "Requirement already satisfied: rich in /usr/local/lib/python3.10/dist-packages (from mmengine>=0.2.0->mmcv>=2.0.0) (13.3.4)\n",
- "Requirement already satisfied: termcolor in /usr/local/lib/python3.10/dist-packages (from mmengine>=0.2.0->mmcv>=2.0.0) (2.3.0)\n",
- "Requirement already satisfied: tomli>=2.0.1 in /usr/local/lib/python3.10/dist-packages (from yapf->mmcv>=2.0.0) (2.0.1)\n",
- "Requirement already satisfied: contourpy>=1.0.1 in /usr/local/lib/python3.10/dist-packages (from matplotlib->mmengine>=0.2.0->mmcv>=2.0.0) (1.0.7)\n",
- "Requirement already satisfied: cycler>=0.10 in /usr/local/lib/python3.10/dist-packages (from matplotlib->mmengine>=0.2.0->mmcv>=2.0.0) (0.11.0)\n",
- "Requirement already satisfied: fonttools>=4.22.0 in /usr/local/lib/python3.10/dist-packages (from matplotlib->mmengine>=0.2.0->mmcv>=2.0.0) (4.39.3)\n",
- "Requirement already satisfied: kiwisolver>=1.0.1 in /usr/local/lib/python3.10/dist-packages (from matplotlib->mmengine>=0.2.0->mmcv>=2.0.0) (1.4.4)\n",
- "Requirement already satisfied: pyparsing>=2.3.1 in /usr/local/lib/python3.10/dist-packages (from matplotlib->mmengine>=0.2.0->mmcv>=2.0.0) (3.0.9)\n",
- "Requirement already satisfied: python-dateutil>=2.7 in /usr/local/lib/python3.10/dist-packages (from matplotlib->mmengine>=0.2.0->mmcv>=2.0.0) (2.8.2)\n",
- "Requirement already satisfied: markdown-it-py<3.0.0,>=2.2.0 in /usr/local/lib/python3.10/dist-packages (from rich->mmengine>=0.2.0->mmcv>=2.0.0) (2.2.0)\n",
- "Requirement already satisfied: pygments<3.0.0,>=2.13.0 in /usr/local/lib/python3.10/dist-packages (from rich->mmengine>=0.2.0->mmcv>=2.0.0) (2.14.0)\n",
- "Requirement already satisfied: mdurl~=0.1 in /usr/local/lib/python3.10/dist-packages (from markdown-it-py<3.0.0,>=2.2.0->rich->mmengine>=0.2.0->mmcv>=2.0.0) (0.1.2)\n",
- "Requirement already satisfied: six>=1.5 in /usr/local/lib/python3.10/dist-packages (from python-dateutil>=2.7->matplotlib->mmengine>=0.2.0->mmcv>=2.0.0) (1.16.0)\n",
- "Installing collected packages: mmcv\n",
- "Successfully installed mmcv-2.0.0\n",
- "Cloning into 'mmaction2'...\n",
- "remote: Enumerating objects: 21284, done.\u001b[K\n",
- "remote: Counting objects: 100% (394/394), done.\u001b[K\n",
- "remote: Compressing objects: 100% (287/287), done.\u001b[K\n",
- "remote: Total 21284 (delta 175), reused 248 (delta 103), pack-reused 20890\u001b[K\n",
- "Receiving objects: 100% (21284/21284), 68.63 MiB | 16.59 MiB/s, done.\n",
- "Resolving deltas: 100% (14990/14990), done.\n",
- "/content/mmaction2\n",
- "Looking in indexes: https://pypi.org/simple, https://us-python.pkg.dev/colab-wheels/public/simple/\n",
- "Obtaining file:///content/mmaction2\n",
- " Preparing metadata (setup.py) ... \u001b[?25l\u001b[?25hdone\n",
- "Collecting decord>=0.4.1 (from mmaction2==1.0.0)\n",
- " Downloading decord-0.6.0-py3-none-manylinux2010_x86_64.whl (13.6 MB)\n",
- "\u001b[2K \u001b[90mโโโโโโโโโโโโโโโโโโโโโโโโโโโโโโโโโโโโโโโโ\u001b[0m \u001b[32m13.6/13.6 MB\u001b[0m \u001b[31m76.9 MB/s\u001b[0m eta \u001b[36m0:00:00\u001b[0m\n",
- "\u001b[?25hCollecting einops (from mmaction2==1.0.0)\n",
- " Downloading einops-0.6.1-py3-none-any.whl (42 kB)\n",
- "\u001b[2K \u001b[90mโโโโโโโโโโโโโโโโโโโโโโโโโโโโโโโโโโโโโโโโ\u001b[0m \u001b[32m42.2/42.2 kB\u001b[0m \u001b[31m4.5 MB/s\u001b[0m eta \u001b[36m0:00:00\u001b[0m\n",
- "\u001b[?25hRequirement already satisfied: matplotlib in /usr/local/lib/python3.10/dist-packages (from mmaction2==1.0.0) (3.7.1)\n",
- "Requirement already satisfied: numpy in /usr/local/lib/python3.10/dist-packages (from mmaction2==1.0.0) (1.22.4)\n",
- "Requirement already satisfied: opencv-contrib-python in /usr/local/lib/python3.10/dist-packages (from mmaction2==1.0.0) (4.7.0.72)\n",
- "Requirement already satisfied: Pillow in /usr/local/lib/python3.10/dist-packages (from mmaction2==1.0.0) (8.4.0)\n",
- "Requirement already satisfied: scipy in /usr/local/lib/python3.10/dist-packages (from mmaction2==1.0.0) (1.10.1)\n",
- "Requirement already satisfied: torch>=1.3 in /usr/local/lib/python3.10/dist-packages (from mmaction2==1.0.0) (2.0.0+cu118)\n",
- "Requirement already satisfied: filelock in /usr/local/lib/python3.10/dist-packages (from torch>=1.3->mmaction2==1.0.0) (3.12.0)\n",
- "Requirement already satisfied: typing-extensions in /usr/local/lib/python3.10/dist-packages (from torch>=1.3->mmaction2==1.0.0) (4.5.0)\n",
- "Requirement already satisfied: sympy in /usr/local/lib/python3.10/dist-packages (from torch>=1.3->mmaction2==1.0.0) (1.11.1)\n",
- "Requirement already satisfied: networkx in /usr/local/lib/python3.10/dist-packages (from torch>=1.3->mmaction2==1.0.0) (3.1)\n",
- "Requirement already satisfied: jinja2 in /usr/local/lib/python3.10/dist-packages (from torch>=1.3->mmaction2==1.0.0) (3.1.2)\n",
- "Requirement already satisfied: triton==2.0.0 in /usr/local/lib/python3.10/dist-packages (from torch>=1.3->mmaction2==1.0.0) (2.0.0)\n",
- "Requirement already satisfied: cmake in /usr/local/lib/python3.10/dist-packages (from triton==2.0.0->torch>=1.3->mmaction2==1.0.0) (3.25.2)\n",
- "Requirement already satisfied: lit in /usr/local/lib/python3.10/dist-packages (from triton==2.0.0->torch>=1.3->mmaction2==1.0.0) (16.0.3)\n",
- "Requirement already satisfied: contourpy>=1.0.1 in /usr/local/lib/python3.10/dist-packages (from matplotlib->mmaction2==1.0.0) (1.0.7)\n",
- "Requirement already satisfied: cycler>=0.10 in /usr/local/lib/python3.10/dist-packages (from matplotlib->mmaction2==1.0.0) (0.11.0)\n",
- "Requirement already satisfied: fonttools>=4.22.0 in /usr/local/lib/python3.10/dist-packages (from matplotlib->mmaction2==1.0.0) (4.39.3)\n",
- "Requirement already satisfied: kiwisolver>=1.0.1 in /usr/local/lib/python3.10/dist-packages (from matplotlib->mmaction2==1.0.0) (1.4.4)\n",
- "Requirement already satisfied: packaging>=20.0 in /usr/local/lib/python3.10/dist-packages (from matplotlib->mmaction2==1.0.0) (23.1)\n",
- "Requirement already satisfied: pyparsing>=2.3.1 in /usr/local/lib/python3.10/dist-packages (from matplotlib->mmaction2==1.0.0) (3.0.9)\n",
- "Requirement already satisfied: python-dateutil>=2.7 in /usr/local/lib/python3.10/dist-packages (from matplotlib->mmaction2==1.0.0) (2.8.2)\n",
- "Requirement already satisfied: six>=1.5 in /usr/local/lib/python3.10/dist-packages (from python-dateutil>=2.7->matplotlib->mmaction2==1.0.0) (1.16.0)\n",
- "Requirement already satisfied: MarkupSafe>=2.0 in /usr/local/lib/python3.10/dist-packages (from jinja2->torch>=1.3->mmaction2==1.0.0) (2.1.2)\n",
- "Requirement already satisfied: mpmath>=0.19 in /usr/local/lib/python3.10/dist-packages (from sympy->torch>=1.3->mmaction2==1.0.0) (1.3.0)\n",
- "Installing collected packages: einops, decord, mmaction2\n",
- " Running setup.py develop for mmaction2\n",
- "Successfully installed decord-0.6.0 einops-0.6.1 mmaction2-1.0.0\n",
- "Looking in indexes: https://pypi.org/simple, https://us-python.pkg.dev/colab-wheels/public/simple/\n",
- "Collecting av>=9.0 (from -r requirements/optional.txt (line 1))\n",
- " Downloading av-10.0.0-cp310-cp310-manylinux_2_17_x86_64.manylinux2014_x86_64.whl (31.0 MB)\n",
- "\u001b[2K \u001b[90mโโโโโโโโโโโโโโโโโโโโโโโโโโโโโโโโโโโโโโโโ\u001b[0m \u001b[32m31.0/31.0 MB\u001b[0m \u001b[31m38.3 MB/s\u001b[0m eta \u001b[36m0:00:00\u001b[0m\n",
- "\u001b[?25hRequirement already satisfied: future in /usr/local/lib/python3.10/dist-packages (from -r requirements/optional.txt (line 2)) (0.18.3)\n",
- "Collecting fvcore (from -r requirements/optional.txt (line 3))\n",
- " Downloading fvcore-0.1.5.post20221221.tar.gz (50 kB)\n",
- "\u001b[2K \u001b[90mโโโโโโโโโโโโโโโโโโโโโโโโโโโโโโโโโโโโโโโโ\u001b[0m \u001b[32m50.2/50.2 kB\u001b[0m \u001b[31m6.7 MB/s\u001b[0m eta \u001b[36m0:00:00\u001b[0m\n",
- "\u001b[?25h Preparing metadata (setup.py) ... \u001b[?25l\u001b[?25hdone\n",
- "Requirement already satisfied: imgaug in /usr/local/lib/python3.10/dist-packages (from -r requirements/optional.txt (line 4)) (0.4.0)\n",
- "Requirement already satisfied: librosa in /usr/local/lib/python3.10/dist-packages (from -r requirements/optional.txt (line 5)) (0.10.0.post2)\n",
- "Collecting lmdb (from -r requirements/optional.txt (line 6))\n",
- " Downloading lmdb-1.4.1-cp310-cp310-manylinux_2_17_x86_64.manylinux2014_x86_64.whl (299 kB)\n",
- "\u001b[2K \u001b[90mโโโโโโโโโโโโโโโโโโโโโโโโโโโโโโโโโโโโโโ\u001b[0m \u001b[32m299.2/299.2 kB\u001b[0m \u001b[31m30.2 MB/s\u001b[0m eta \u001b[36m0:00:00\u001b[0m\n",
- "\u001b[?25hRequirement already satisfied: moviepy in /usr/local/lib/python3.10/dist-packages (from -r requirements/optional.txt (line 7)) (1.0.3)\n",
- "Requirement already satisfied: packaging in /usr/local/lib/python3.10/dist-packages (from -r requirements/optional.txt (line 8)) (23.1)\n",
- "Collecting pims (from -r requirements/optional.txt (line 9))\n",
- " Downloading PIMS-0.6.1.tar.gz (86 kB)\n",
- "\u001b[2K \u001b[90mโโโโโโโโโโโโโโโโโโโโโโโโโโโโโโโโโโโโโโโโ\u001b[0m \u001b[32m86.0/86.0 kB\u001b[0m \u001b[31m12.2 MB/s\u001b[0m eta \u001b[36m0:00:00\u001b[0m\n",
- "\u001b[?25h Preparing metadata (setup.py) ... \u001b[?25l\u001b[?25hdone\n",
- "Collecting PyTurboJPEG (from -r requirements/optional.txt (line 10))\n",
- " Downloading PyTurboJPEG-1.7.1.tar.gz (11 kB)\n",
- " Preparing metadata (setup.py) ... \u001b[?25l\u001b[?25hdone\n",
- "Requirement already satisfied: soundfile in /usr/local/lib/python3.10/dist-packages (from -r requirements/optional.txt (line 11)) (0.12.1)\n",
- "Requirement already satisfied: tensorboard in /usr/local/lib/python3.10/dist-packages (from -r requirements/optional.txt (line 12)) (2.12.2)\n",
- "Collecting wandb (from -r requirements/optional.txt (line 13))\n",
- " Downloading wandb-0.15.2-py3-none-any.whl (2.0 MB)\n",
- "\u001b[2K \u001b[90mโโโโโโโโโโโโโโโโโโโโโโโโโโโโโโโโโโโโโโโโ\u001b[0m \u001b[32m2.0/2.0 MB\u001b[0m \u001b[31m79.1 MB/s\u001b[0m eta \u001b[36m0:00:00\u001b[0m\n",
- "\u001b[?25hRequirement already satisfied: numpy in /usr/local/lib/python3.10/dist-packages (from fvcore->-r requirements/optional.txt (line 3)) (1.22.4)\n",
- "Collecting yacs>=0.1.6 (from fvcore->-r requirements/optional.txt (line 3))\n",
- " Downloading yacs-0.1.8-py3-none-any.whl (14 kB)\n",
- "Requirement already satisfied: pyyaml>=5.1 in /usr/local/lib/python3.10/dist-packages (from fvcore->-r requirements/optional.txt (line 3)) (6.0)\n",
- "Requirement already satisfied: tqdm in /usr/local/lib/python3.10/dist-packages (from fvcore->-r requirements/optional.txt (line 3)) (4.65.0)\n",
- "Requirement already satisfied: termcolor>=1.1 in /usr/local/lib/python3.10/dist-packages (from fvcore->-r requirements/optional.txt (line 3)) (2.3.0)\n",
- "Requirement already satisfied: Pillow in /usr/local/lib/python3.10/dist-packages (from fvcore->-r requirements/optional.txt (line 3)) (8.4.0)\n",
- "Requirement already satisfied: tabulate in /usr/local/lib/python3.10/dist-packages (from fvcore->-r requirements/optional.txt (line 3)) (0.8.10)\n",
- "Collecting iopath>=0.1.7 (from fvcore->-r requirements/optional.txt (line 3))\n",
- " Downloading iopath-0.1.10.tar.gz (42 kB)\n",
- "\u001b[2K \u001b[90mโโโโโโโโโโโโโโโโโโโโโโโโโโโโโโโโโโโโโโโโ\u001b[0m \u001b[32m42.2/42.2 kB\u001b[0m \u001b[31m4.8 MB/s\u001b[0m eta \u001b[36m0:00:00\u001b[0m\n",
- "\u001b[?25h Preparing metadata (setup.py) ... \u001b[?25l\u001b[?25hdone\n",
- "Requirement already satisfied: six in /usr/local/lib/python3.10/dist-packages (from imgaug->-r requirements/optional.txt (line 4)) (1.16.0)\n",
- "Requirement already satisfied: scipy in /usr/local/lib/python3.10/dist-packages (from imgaug->-r requirements/optional.txt (line 4)) (1.10.1)\n",
- "Requirement already satisfied: matplotlib in /usr/local/lib/python3.10/dist-packages (from imgaug->-r requirements/optional.txt (line 4)) (3.7.1)\n",
- "Requirement already satisfied: scikit-image>=0.14.2 in /usr/local/lib/python3.10/dist-packages (from imgaug->-r requirements/optional.txt (line 4)) (0.19.3)\n",
- "Requirement already satisfied: opencv-python in /usr/local/lib/python3.10/dist-packages (from imgaug->-r requirements/optional.txt (line 4)) (4.7.0.72)\n",
- "Requirement already satisfied: imageio in /usr/local/lib/python3.10/dist-packages (from imgaug->-r requirements/optional.txt (line 4)) (2.25.1)\n",
- "Requirement already satisfied: Shapely in /usr/local/lib/python3.10/dist-packages (from imgaug->-r requirements/optional.txt (line 4)) (2.0.1)\n",
- "Requirement already satisfied: audioread>=2.1.9 in /usr/local/lib/python3.10/dist-packages (from librosa->-r requirements/optional.txt (line 5)) (3.0.0)\n",
- "Requirement already satisfied: scikit-learn>=0.20.0 in /usr/local/lib/python3.10/dist-packages (from librosa->-r requirements/optional.txt (line 5)) (1.2.2)\n",
- "Requirement already satisfied: joblib>=0.14 in /usr/local/lib/python3.10/dist-packages (from librosa->-r requirements/optional.txt (line 5)) (1.2.0)\n",
- "Requirement already satisfied: decorator>=4.3.0 in /usr/local/lib/python3.10/dist-packages (from librosa->-r requirements/optional.txt (line 5)) (4.4.2)\n",
- "Requirement already satisfied: numba>=0.51.0 in /usr/local/lib/python3.10/dist-packages (from librosa->-r requirements/optional.txt (line 5)) (0.56.4)\n",
- "Requirement already satisfied: pooch<1.7,>=1.0 in /usr/local/lib/python3.10/dist-packages (from librosa->-r requirements/optional.txt (line 5)) (1.6.0)\n",
- "Requirement already satisfied: soxr>=0.3.2 in /usr/local/lib/python3.10/dist-packages (from librosa->-r requirements/optional.txt (line 5)) (0.3.5)\n",
- "Requirement already satisfied: typing-extensions>=4.1.1 in /usr/local/lib/python3.10/dist-packages (from librosa->-r requirements/optional.txt (line 5)) (4.5.0)\n",
- "Requirement already satisfied: lazy-loader>=0.1 in /usr/local/lib/python3.10/dist-packages (from librosa->-r requirements/optional.txt (line 5)) (0.2)\n",
- "Requirement already satisfied: msgpack>=1.0 in /usr/local/lib/python3.10/dist-packages (from librosa->-r requirements/optional.txt (line 5)) (1.0.5)\n",
- "Requirement already satisfied: requests<3.0,>=2.8.1 in /usr/local/lib/python3.10/dist-packages (from moviepy->-r requirements/optional.txt (line 7)) (2.27.1)\n",
- "Requirement already satisfied: proglog<=1.0.0 in /usr/local/lib/python3.10/dist-packages (from moviepy->-r requirements/optional.txt (line 7)) (0.1.10)\n",
- "Requirement already satisfied: imageio-ffmpeg>=0.2.0 in /usr/local/lib/python3.10/dist-packages (from moviepy->-r requirements/optional.txt (line 7)) (0.4.8)\n",
- "Collecting slicerator>=0.9.8 (from pims->-r requirements/optional.txt (line 9))\n",
- " Downloading slicerator-1.1.0-py3-none-any.whl (10 kB)\n",
- "Requirement already satisfied: cffi>=1.0 in /usr/local/lib/python3.10/dist-packages (from soundfile->-r requirements/optional.txt (line 11)) (1.15.1)\n",
- "Requirement already satisfied: absl-py>=0.4 in /usr/local/lib/python3.10/dist-packages (from tensorboard->-r requirements/optional.txt (line 12)) (1.4.0)\n",
- "Requirement already satisfied: grpcio>=1.48.2 in /usr/local/lib/python3.10/dist-packages (from tensorboard->-r requirements/optional.txt (line 12)) (1.54.0)\n",
- "Requirement already satisfied: google-auth<3,>=1.6.3 in /usr/local/lib/python3.10/dist-packages (from tensorboard->-r requirements/optional.txt (line 12)) (2.17.3)\n",
- "Requirement already satisfied: google-auth-oauthlib<1.1,>=0.5 in /usr/local/lib/python3.10/dist-packages (from tensorboard->-r requirements/optional.txt (line 12)) (1.0.0)\n",
- "Requirement already satisfied: markdown>=2.6.8 in /usr/local/lib/python3.10/dist-packages (from tensorboard->-r requirements/optional.txt (line 12)) (3.4.3)\n",
- "Requirement already satisfied: protobuf>=3.19.6 in /usr/local/lib/python3.10/dist-packages (from tensorboard->-r requirements/optional.txt (line 12)) (3.20.3)\n",
- "Requirement already satisfied: setuptools>=41.0.0 in /usr/local/lib/python3.10/dist-packages (from tensorboard->-r requirements/optional.txt (line 12)) (67.7.2)\n",
- "Requirement already satisfied: tensorboard-data-server<0.8.0,>=0.7.0 in /usr/local/lib/python3.10/dist-packages (from tensorboard->-r requirements/optional.txt (line 12)) (0.7.0)\n",
- "Requirement already satisfied: tensorboard-plugin-wit>=1.6.0 in /usr/local/lib/python3.10/dist-packages (from tensorboard->-r requirements/optional.txt (line 12)) (1.8.1)\n",
- "Requirement already satisfied: werkzeug>=1.0.1 in /usr/local/lib/python3.10/dist-packages (from tensorboard->-r requirements/optional.txt (line 12)) (2.3.0)\n",
- "Requirement already satisfied: wheel>=0.26 in /usr/local/lib/python3.10/dist-packages (from tensorboard->-r requirements/optional.txt (line 12)) (0.40.0)\n",
- "Requirement already satisfied: Click!=8.0.0,>=7.0 in /usr/local/lib/python3.10/dist-packages (from wandb->-r requirements/optional.txt (line 13)) (8.1.3)\n",
- "Collecting GitPython!=3.1.29,>=1.0.0 (from wandb->-r requirements/optional.txt (line 13))\n",
- " Downloading GitPython-3.1.31-py3-none-any.whl (184 kB)\n",
- "\u001b[2K \u001b[90mโโโโโโโโโโโโโโโโโโโโโโโโโโโโโโโโโโโโโโ\u001b[0m \u001b[32m184.3/184.3 kB\u001b[0m \u001b[31m22.9 MB/s\u001b[0m eta \u001b[36m0:00:00\u001b[0m\n",
- "\u001b[?25hRequirement already satisfied: psutil>=5.0.0 in /usr/local/lib/python3.10/dist-packages (from wandb->-r requirements/optional.txt (line 13)) (5.9.5)\n",
- "Collecting sentry-sdk>=1.0.0 (from wandb->-r requirements/optional.txt (line 13))\n",
- " Downloading sentry_sdk-1.22.2-py2.py3-none-any.whl (203 kB)\n",
- "\u001b[2K \u001b[90mโโโโโโโโโโโโโโโโโโโโโโโโโโโโโโโโโโโโโโ\u001b[0m \u001b[32m203.3/203.3 kB\u001b[0m \u001b[31m25.7 MB/s\u001b[0m eta \u001b[36m0:00:00\u001b[0m\n",
- "\u001b[?25hCollecting docker-pycreds>=0.4.0 (from wandb->-r requirements/optional.txt (line 13))\n",
- " Downloading docker_pycreds-0.4.0-py2.py3-none-any.whl (9.0 kB)\n",
- "Collecting pathtools (from wandb->-r requirements/optional.txt (line 13))\n",
- " Downloading pathtools-0.1.2.tar.gz (11 kB)\n",
- " Preparing metadata (setup.py) ... \u001b[?25l\u001b[?25hdone\n",
- "Collecting setproctitle (from wandb->-r requirements/optional.txt (line 13))\n",
- " Downloading setproctitle-1.3.2-cp310-cp310-manylinux_2_5_x86_64.manylinux1_x86_64.manylinux_2_17_x86_64.manylinux2014_x86_64.whl (30 kB)\n",
- "Requirement already satisfied: appdirs>=1.4.3 in /usr/local/lib/python3.10/dist-packages (from wandb->-r requirements/optional.txt (line 13)) (1.4.4)\n",
- "Requirement already satisfied: pycparser in /usr/local/lib/python3.10/dist-packages (from cffi>=1.0->soundfile->-r requirements/optional.txt (line 11)) (2.21)\n",
- "Collecting gitdb<5,>=4.0.1 (from GitPython!=3.1.29,>=1.0.0->wandb->-r requirements/optional.txt (line 13))\n",
- " Downloading gitdb-4.0.10-py3-none-any.whl (62 kB)\n",
- "\u001b[2K \u001b[90mโโโโโโโโโโโโโโโโโโโโโโโโโโโโโโโโโโโโโโโโ\u001b[0m \u001b[32m62.7/62.7 kB\u001b[0m \u001b[31m9.0 MB/s\u001b[0m eta \u001b[36m0:00:00\u001b[0m\n",
- "\u001b[?25hRequirement already satisfied: cachetools<6.0,>=2.0.0 in /usr/local/lib/python3.10/dist-packages (from google-auth<3,>=1.6.3->tensorboard->-r requirements/optional.txt (line 12)) (5.3.0)\n",
- "Requirement already satisfied: pyasn1-modules>=0.2.1 in /usr/local/lib/python3.10/dist-packages (from google-auth<3,>=1.6.3->tensorboard->-r requirements/optional.txt (line 12)) (0.3.0)\n",
- "Requirement already satisfied: rsa<5,>=3.1.4 in /usr/local/lib/python3.10/dist-packages (from google-auth<3,>=1.6.3->tensorboard->-r requirements/optional.txt (line 12)) (4.9)\n",
- "Requirement already satisfied: requests-oauthlib>=0.7.0 in /usr/local/lib/python3.10/dist-packages (from google-auth-oauthlib<1.1,>=0.5->tensorboard->-r requirements/optional.txt (line 12)) (1.3.1)\n",
- "Collecting portalocker (from iopath>=0.1.7->fvcore->-r requirements/optional.txt (line 3))\n",
- " Downloading portalocker-2.7.0-py2.py3-none-any.whl (15 kB)\n",
- "Requirement already satisfied: llvmlite<0.40,>=0.39.0dev0 in /usr/local/lib/python3.10/dist-packages (from numba>=0.51.0->librosa->-r requirements/optional.txt (line 5)) (0.39.1)\n",
- "Requirement already satisfied: urllib3<1.27,>=1.21.1 in /usr/local/lib/python3.10/dist-packages (from requests<3.0,>=2.8.1->moviepy->-r requirements/optional.txt (line 7)) (1.26.15)\n",
- "Requirement already satisfied: certifi>=2017.4.17 in /usr/local/lib/python3.10/dist-packages (from requests<3.0,>=2.8.1->moviepy->-r requirements/optional.txt (line 7)) (2022.12.7)\n",
- "Requirement already satisfied: charset-normalizer~=2.0.0 in /usr/local/lib/python3.10/dist-packages (from requests<3.0,>=2.8.1->moviepy->-r requirements/optional.txt (line 7)) (2.0.12)\n",
- "Requirement already satisfied: idna<4,>=2.5 in /usr/local/lib/python3.10/dist-packages (from requests<3.0,>=2.8.1->moviepy->-r requirements/optional.txt (line 7)) (3.4)\n",
- "Requirement already satisfied: networkx>=2.2 in /usr/local/lib/python3.10/dist-packages (from scikit-image>=0.14.2->imgaug->-r requirements/optional.txt (line 4)) (3.1)\n",
- "Requirement already satisfied: tifffile>=2019.7.26 in /usr/local/lib/python3.10/dist-packages (from scikit-image>=0.14.2->imgaug->-r requirements/optional.txt (line 4)) (2023.4.12)\n",
- "Requirement already satisfied: PyWavelets>=1.1.1 in /usr/local/lib/python3.10/dist-packages (from scikit-image>=0.14.2->imgaug->-r requirements/optional.txt (line 4)) (1.4.1)\n",
- "Requirement already satisfied: threadpoolctl>=2.0.0 in /usr/local/lib/python3.10/dist-packages (from scikit-learn>=0.20.0->librosa->-r requirements/optional.txt (line 5)) (3.1.0)\n",
- "Requirement already satisfied: MarkupSafe>=2.1.1 in /usr/local/lib/python3.10/dist-packages (from werkzeug>=1.0.1->tensorboard->-r requirements/optional.txt (line 12)) (2.1.2)\n",
- "Requirement already satisfied: contourpy>=1.0.1 in /usr/local/lib/python3.10/dist-packages (from matplotlib->imgaug->-r requirements/optional.txt (line 4)) (1.0.7)\n",
- "Requirement already satisfied: cycler>=0.10 in /usr/local/lib/python3.10/dist-packages (from matplotlib->imgaug->-r requirements/optional.txt (line 4)) (0.11.0)\n",
- "Requirement already satisfied: fonttools>=4.22.0 in /usr/local/lib/python3.10/dist-packages (from matplotlib->imgaug->-r requirements/optional.txt (line 4)) (4.39.3)\n",
- "Requirement already satisfied: kiwisolver>=1.0.1 in /usr/local/lib/python3.10/dist-packages (from matplotlib->imgaug->-r requirements/optional.txt (line 4)) (1.4.4)\n",
- "Requirement already satisfied: pyparsing>=2.3.1 in /usr/local/lib/python3.10/dist-packages (from matplotlib->imgaug->-r requirements/optional.txt (line 4)) (3.0.9)\n",
- "Requirement already satisfied: python-dateutil>=2.7 in /usr/local/lib/python3.10/dist-packages (from matplotlib->imgaug->-r requirements/optional.txt (line 4)) (2.8.2)\n",
- "Collecting smmap<6,>=3.0.1 (from gitdb<5,>=4.0.1->GitPython!=3.1.29,>=1.0.0->wandb->-r requirements/optional.txt (line 13))\n",
- " Downloading smmap-5.0.0-py3-none-any.whl (24 kB)\n",
- "Requirement already satisfied: pyasn1<0.6.0,>=0.4.6 in /usr/local/lib/python3.10/dist-packages (from pyasn1-modules>=0.2.1->google-auth<3,>=1.6.3->tensorboard->-r requirements/optional.txt (line 12)) (0.5.0)\n",
- "Requirement already satisfied: oauthlib>=3.0.0 in /usr/local/lib/python3.10/dist-packages (from requests-oauthlib>=0.7.0->google-auth-oauthlib<1.1,>=0.5->tensorboard->-r requirements/optional.txt (line 12)) (3.2.2)\n",
- "Building wheels for collected packages: fvcore, pims, PyTurboJPEG, iopath, pathtools\n",
- " Building wheel for fvcore (setup.py) ... \u001b[?25l\u001b[?25hdone\n",
- " Created wheel for fvcore: filename=fvcore-0.1.5.post20221221-py3-none-any.whl size=61405 sha256=25c1e50155c8788d00eec898793c96133a746a8bb076ffc5c01f5a4dc256751e\n",
- " Stored in directory: /root/.cache/pip/wheels/01/c0/af/77c1cf53a1be9e42a52b48e5af2169d40ec2e89f7362489dd0\n",
- " Building wheel for pims (setup.py) ... \u001b[?25l\u001b[?25hdone\n",
- " Created wheel for pims: filename=PIMS-0.6.1-py3-none-any.whl size=82619 sha256=59a328dc88a438c60cfb6e937e04c8a7dd55ad2a2905034cd41ff80cdbba6497\n",
- " Stored in directory: /root/.cache/pip/wheels/cc/bf/3e/bfa77232d942f8244145f9c713b6b38f6ef04b6fb5c021c114\n",
- " Building wheel for PyTurboJPEG (setup.py) ... \u001b[?25l\u001b[?25hdone\n",
- " Created wheel for PyTurboJPEG: filename=PyTurboJPEG-1.7.1-py3-none-any.whl size=12243 sha256=ddf6424c85ac533335abd96dd9e98b014ea1dd4f143c88cd35ecb08d6128f411\n",
- " Stored in directory: /root/.cache/pip/wheels/de/6e/b1/e7ba70c328c3395555cb92ca8820babb32950d867858b1948b\n",
- " Building wheel for iopath (setup.py) ... \u001b[?25l\u001b[?25hdone\n",
- " Created wheel for iopath: filename=iopath-0.1.10-py3-none-any.whl size=31531 sha256=db977a4344bebbdd710665e767caab4fbcf53cc6aea0707cd38d26c45718331e\n",
- " Stored in directory: /root/.cache/pip/wheels/9a/a3/b6/ac0fcd1b4ed5cfeb3db92e6a0e476cfd48ed0df92b91080c1d\n",
- " Building wheel for pathtools (setup.py) ... \u001b[?25l\u001b[?25hdone\n",
- " Created wheel for pathtools: filename=pathtools-0.1.2-py3-none-any.whl size=8791 sha256=08bb5753ce029aef01f25c3e81882d93c0e040e5932e90a02a062ad058756b52\n",
- " Stored in directory: /root/.cache/pip/wheels/e7/f3/22/152153d6eb222ee7a56ff8617d80ee5207207a8c00a7aab794\n",
- "Successfully built fvcore pims PyTurboJPEG iopath pathtools\n",
- "Installing collected packages: slicerator, pathtools, lmdb, av, yacs, smmap, setproctitle, sentry-sdk, PyTurboJPEG, portalocker, docker-pycreds, pims, iopath, gitdb, GitPython, fvcore, wandb\n",
- "Successfully installed GitPython-3.1.31 PyTurboJPEG-1.7.1 av-10.0.0 docker-pycreds-0.4.0 fvcore-0.1.5.post20221221 gitdb-4.0.10 iopath-0.1.10 lmdb-1.4.1 pathtools-0.1.2 pims-0.6.1 portalocker-2.7.0 sentry-sdk-1.22.2 setproctitle-1.3.2 slicerator-1.1.0 smmap-5.0.0 wandb-0.15.2 yacs-0.1.8\n"
- ]
- }
- ],
- "source": [
- "# install MMEngine, MMCV and MMDetection using MIM\n",
- "%pip install -U openmim\n",
- "!mim install mmengine\n",
- "!mim install \"mmcv>=2.0.0\"\n",
- "\n",
- "# Install mmaction2\n",
- "!rm -rf mmaction2\n",
- "!git clone https://github.com/open-mmlab/mmaction2.git -b main\n",
- "%cd mmaction2\n",
- "\n",
- "!pip install -e .\n",
- "\n",
- "# Install some optional requirements\n",
- "!pip install -r requirements/optional.txt"
- ]
- },
- {
- "cell_type": "code",
- "execution_count": 5,
- "metadata": {
- "colab": {
- "base_uri": "https://localhost:8080/"
- },
- "id": "No_zZAFpWC-a",
- "outputId": "9386dd81-2308-4adb-d3cb-798de11c035e"
- },
- "outputs": [
- {
- "output_type": "stream",
- "name": "stdout",
- "text": [
- "2.0.0+cu118 True\n",
- "1.0.0\n",
- "11.8\n",
- "GCC 9.3\n",
- "OrderedDict([('sys.platform', 'linux'), ('Python', '3.10.11 (main, Apr 5 2023, 14:15:10) [GCC 9.4.0]'), ('CUDA available', True), ('numpy_random_seed', 2147483648), ('GPU 0', 'Tesla T4'), ('CUDA_HOME', '/usr/local/cuda'), ('NVCC', 'Cuda compilation tools, release 11.8, V11.8.89'), ('GCC', 'x86_64-linux-gnu-gcc (Ubuntu 9.4.0-1ubuntu1~20.04.1) 9.4.0'), ('PyTorch', '2.0.0+cu118'), ('PyTorch compiling details', 'PyTorch built with:\\n - GCC 9.3\\n - C++ Version: 201703\\n - Intel(R) oneAPI Math Kernel Library Version 2022.2-Product Build 20220804 for Intel(R) 64 architecture applications\\n - Intel(R) MKL-DNN v2.7.3 (Git Hash 6dbeffbae1f23cbbeae17adb7b5b13f1f37c080e)\\n - OpenMP 201511 (a.k.a. OpenMP 4.5)\\n - LAPACK is enabled (usually provided by MKL)\\n - NNPACK is enabled\\n - CPU capability usage: AVX2\\n - CUDA Runtime 11.8\\n - NVCC architecture flags: -gencode;arch=compute_37,code=sm_37;-gencode;arch=compute_50,code=sm_50;-gencode;arch=compute_60,code=sm_60;-gencode;arch=compute_70,code=sm_70;-gencode;arch=compute_75,code=sm_75;-gencode;arch=compute_80,code=sm_80;-gencode;arch=compute_86,code=sm_86;-gencode;arch=compute_90,code=sm_90\\n - CuDNN 8.7\\n - Magma 2.6.1\\n - Build settings: BLAS_INFO=mkl, BUILD_TYPE=Release, CUDA_VERSION=11.8, CUDNN_VERSION=8.7.0, CXX_COMPILER=/opt/rh/devtoolset-9/root/usr/bin/c++, CXX_FLAGS= -D_GLIBCXX_USE_CXX11_ABI=0 -fabi-version=11 -Wno-deprecated -fvisibility-inlines-hidden -DUSE_PTHREADPOOL -DNDEBUG -DUSE_KINETO -DLIBKINETO_NOROCTRACER -DUSE_FBGEMM -DUSE_QNNPACK -DUSE_PYTORCH_QNNPACK -DUSE_XNNPACK -DSYMBOLICATE_MOBILE_DEBUG_HANDLE -O2 -fPIC -Wall -Wextra -Werror=return-type -Werror=non-virtual-dtor -Werror=bool-operation -Wnarrowing -Wno-missing-field-initializers -Wno-type-limits -Wno-array-bounds -Wno-unknown-pragmas -Wunused-local-typedefs -Wno-unused-parameter -Wno-unused-function -Wno-unused-result -Wno-strict-overflow -Wno-strict-aliasing -Wno-error=deprecated-declarations -Wno-stringop-overflow -Wno-psabi -Wno-error=pedantic -Wno-error=redundant-decls -Wno-error=old-style-cast -fdiagnostics-color=always -faligned-new -Wno-unused-but-set-variable -Wno-maybe-uninitialized -fno-math-errno -fno-trapping-math -Werror=format -Werror=cast-function-type -Wno-stringop-overflow, LAPACK_INFO=mkl, PERF_WITH_AVX=1, PERF_WITH_AVX2=1, PERF_WITH_AVX512=1, TORCH_DISABLE_GPU_ASSERTS=ON, TORCH_VERSION=2.0.0, USE_CUDA=ON, USE_CUDNN=ON, USE_EXCEPTION_PTR=1, USE_GFLAGS=OFF, USE_GLOG=OFF, USE_MKL=ON, USE_MKLDNN=ON, USE_MPI=OFF, USE_NCCL=1, USE_NNPACK=ON, USE_OPENMP=ON, USE_ROCM=OFF, \\n'), ('TorchVision', '0.15.1+cu118'), ('OpenCV', '4.7.0'), ('MMEngine', '0.7.3')])\n"
- ]
- }
- ],
- "source": [
- "# Check Pytorch installation\n",
- "import torch, torchvision\n",
- "print(torch.__version__, torch.cuda.is_available())\n",
- "\n",
- "# Check MMAction2 installation\n",
- "import mmaction\n",
- "print(mmaction.__version__)\n",
- "\n",
- "# Check MMCV installation\n",
- "from mmcv.ops import get_compiling_cuda_version, get_compiler_version\n",
- "print(get_compiling_cuda_version())\n",
- "print(get_compiler_version())\n",
- "\n",
- "# Check MMEngine installation\n",
- "from mmengine.utils.dl_utils import collect_env\n",
- "print(collect_env())"
- ]
- },
- {
- "cell_type": "markdown",
- "metadata": {
- "id": "pXf7oV5DWdab"
- },
- "source": [
- "## Perform inference with a MMAction2 recognizer\n",
- "MMAction2 already provides high level APIs to do inference and training."
- ]
- },
- {
- "cell_type": "code",
- "execution_count": 6,
- "metadata": {
- "colab": {
- "base_uri": "https://localhost:8080/"
- },
- "id": "64CW6d_AaT-Q",
- "outputId": "ea330d8c-2e20-4dbd-d046-51d7c9ec4f7a"
- },
- "outputs": [
- {
- "output_type": "stream",
- "name": "stdout",
- "text": [
- "--2023-05-15 03:33:08-- https://download.openmmlab.com/mmaction/recognition/tsn/tsn_r50_1x1x3_100e_kinetics400_rgb/tsn_r50_1x1x3_100e_kinetics400_rgb_20200614-e508be42.pth\n",
- "Resolving download.openmmlab.com (download.openmmlab.com)... 163.181.82.216, 163.181.82.218, 163.181.82.213, ...\n",
- "Connecting to download.openmmlab.com (download.openmmlab.com)|163.181.82.216|:443... connected.\n",
- "HTTP request sent, awaiting response... 200 OK\n",
- "Length: 97579339 (93M) [application/octet-stream]\n",
- "Saving to: โcheckpoints/tsn_r50_1x1x3_100e_kinetics400_rgb_20200614-e508be42.pthโ\n",
- "\n",
- "checkpoints/tsn_r50 100%[===================>] 93.06M 26.1MB/s in 3.6s \n",
- "\n",
- "2023-05-15 03:33:12 (26.2 MB/s) - โcheckpoints/tsn_r50_1x1x3_100e_kinetics400_rgb_20200614-e508be42.pthโ saved [97579339/97579339]\n",
- "\n"
- ]
- }
- ],
- "source": [
- "!mkdir checkpoints\n",
- "!wget -c https://download.openmmlab.com/mmaction/recognition/tsn/tsn_r50_1x1x3_100e_kinetics400_rgb/tsn_r50_1x1x3_100e_kinetics400_rgb_20200614-e508be42.pth \\\n",
- " -O checkpoints/tsn_r50_1x1x3_100e_kinetics400_rgb_20200614-e508be42.pth"
- ]
+ "cells": [
+ {
+ "cell_type": "markdown",
+ "metadata": {
+ "id": "VcjSRFELVbNk"
+ },
+ "source": [
+ "# MMAction2 Tutorial\n",
+ "\n",
+ "Welcome to MMAction2! This is the official colab tutorial for using MMAction2. In this tutorial, you will learn\n",
+ "- Perform inference with a MMAction2 recognizer.\n",
+ "- Train a new recognizer with a new dataset.\n",
+ "\n",
+ "\n",
+ "Let's start!"
+ ]
+ },
+ {
+ "cell_type": "markdown",
+ "metadata": {
+ "id": "7LqHGkGEVqpm"
+ },
+ "source": [
+ "## Install MMAction2"
+ ]
+ },
+ {
+ "cell_type": "code",
+ "execution_count": 2,
+ "metadata": {
+ "colab": {
+ "base_uri": "https://localhost:8080/"
},
+ "id": "Bf8PpPXtVvmg",
+ "outputId": "9d3f4594-f151-4ee9-a19b-09f8a439ac04"
+ },
+ "outputs": [
{
- "cell_type": "code",
- "execution_count": 7,
- "metadata": {
- "colab": {
- "base_uri": "https://localhost:8080/"
- },
- "id": "HNZB7NoSabzj",
- "outputId": "c0c2ba71-72ff-4cac-a5b8-65590f5a6bb0"
- },
- "outputs": [
- {
- "output_type": "stream",
- "name": "stdout",
- "text": [
- "Loads checkpoint by local backend from path: checkpoints/tsn_r50_1x1x3_100e_kinetics400_rgb_20200614-e508be42.pth\n"
- ]
- }
- ],
- "source": [
- "from mmaction.apis import inference_recognizer, init_recognizer\n",
- "from mmengine import Config\n",
- "\n",
- "\n",
- "# Choose to use a config and initialize the recognizer\n",
- "config = 'configs/recognition/tsn/tsn_imagenet-pretrained-r50_8xb32-1x1x3-100e_kinetics400-rgb.py'\n",
- "config = Config.fromfile(config)\n",
- "# Setup a checkpoint file to load\n",
- "checkpoint = 'checkpoints/tsn_r50_1x1x3_100e_kinetics400_rgb_20200614-e508be42.pth'\n",
- "# Initialize the recognizer\n",
- "model = init_recognizer(config, checkpoint, device='cuda:0')"
- ]
- },
+ "output_type": "stream",
+ "name": "stdout",
+ "text": [
+ "nvcc: NVIDIA (R) Cuda compiler driver\n",
+ "Copyright (c) 2005-2022 NVIDIA Corporation\n",
+ "Built on Wed_Sep_21_10:33:58_PDT_2022\n",
+ "Cuda compilation tools, release 11.8, V11.8.89\n",
+ "Build cuda_11.8.r11.8/compiler.31833905_0\n",
+ "gcc (Ubuntu 9.4.0-1ubuntu1~20.04.1) 9.4.0\n",
+ "Copyright (C) 2019 Free Software Foundation, Inc.\n",
+ "This is free software; see the source for copying conditions. There is NO\n",
+ "warranty; not even for MERCHANTABILITY or FITNESS FOR A PARTICULAR PURPOSE.\n",
+ "\n"
+ ]
+ }
+ ],
+ "source": [
+ "# Check nvcc version\n",
+ "!nvcc -V\n",
+ "# Check GCC version\n",
+ "!gcc --version"
+ ]
+ },
+ {
+ "cell_type": "code",
+ "execution_count": 3,
+ "metadata": {
+ "id": "ZPwKGzqydnb2",
+ "outputId": "27506fa7-48a2-4fe0-d377-56f940dafec4",
+ "colab": {
+ "base_uri": "https://localhost:8080/"
+ }
+ },
+ "outputs": [
{
- "cell_type": "code",
- "execution_count": 8,
- "metadata": {
- "id": "rEMsBnpHapAn",
- "outputId": "ec05049e-7289-4798-94fa-2b773cb23634",
- "colab": {
- "base_uri": "https://localhost:8080/"
- }
- },
- "outputs": [
- {
- "output_type": "stream",
- "name": "stdout",
- "text": [
- "05/15 03:33:18 - mmengine - WARNING - \"FileClient\" will be deprecated in future. Please use io functions in https://mmengine.readthedocs.io/en/latest/api/fileio.html#file-io\n",
- "05/15 03:33:18 - mmengine - WARNING - \"HardDiskBackend\" is the alias of \"LocalBackend\" and the former will be deprecated in future.\n"
- ]
- }
- ],
- "source": [
- "# Use the recognizer to do inference\n",
- "from operator import itemgetter\n",
- "video = 'demo/demo.mp4'\n",
- "label = 'tools/data/kinetics/label_map_k400.txt'\n",
- "results = inference_recognizer(model, video)\n",
- "\n",
- "pred_scores = results.pred_scores.item.tolist()\n",
- "score_tuples = tuple(zip(range(len(pred_scores)), pred_scores))\n",
- "score_sorted = sorted(score_tuples, key=itemgetter(1), reverse=True)\n",
- "top5_label = score_sorted[:5]\n",
- "\n",
- "labels = open(label).readlines()\n",
- "labels = [x.strip() for x in labels]\n",
- "results = [(labels[k[0]], k[1]) for k in top5_label]\n"
- ]
+ "output_type": "stream",
+ "name": "stdout",
+ "text": [
+ "Looking in indexes: https://download.pytorch.org/whl/cu118, https://us-python.pkg.dev/colab-wheels/public/simple/\n",
+ "Requirement already satisfied: torch in /usr/local/lib/python3.10/dist-packages (2.0.0+cu118)\n",
+ "Requirement already satisfied: torchvision in /usr/local/lib/python3.10/dist-packages (0.15.1+cu118)\n",
+ "Requirement already satisfied: torchaudio in /usr/local/lib/python3.10/dist-packages (2.0.1+cu118)\n",
+ "Requirement already satisfied: filelock in /usr/local/lib/python3.10/dist-packages (from torch) (3.12.0)\n",
+ "Requirement already satisfied: typing-extensions in /usr/local/lib/python3.10/dist-packages (from torch) (4.5.0)\n",
+ "Requirement already satisfied: sympy in /usr/local/lib/python3.10/dist-packages (from torch) (1.11.1)\n",
+ "Requirement already satisfied: networkx in /usr/local/lib/python3.10/dist-packages (from torch) (3.1)\n",
+ "Requirement already satisfied: jinja2 in /usr/local/lib/python3.10/dist-packages (from torch) (3.1.2)\n",
+ "Requirement already satisfied: triton==2.0.0 in /usr/local/lib/python3.10/dist-packages (from torch) (2.0.0)\n",
+ "Requirement already satisfied: cmake in /usr/local/lib/python3.10/dist-packages (from triton==2.0.0->torch) (3.25.2)\n",
+ "Requirement already satisfied: lit in /usr/local/lib/python3.10/dist-packages (from triton==2.0.0->torch) (16.0.3)\n",
+ "Requirement already satisfied: numpy in /usr/local/lib/python3.10/dist-packages (from torchvision) (1.22.4)\n",
+ "Requirement already satisfied: requests in /usr/local/lib/python3.10/dist-packages (from torchvision) (2.27.1)\n",
+ "Requirement already satisfied: pillow!=8.3.*,>=5.3.0 in /usr/local/lib/python3.10/dist-packages (from torchvision) (8.4.0)\n",
+ "Requirement already satisfied: MarkupSafe>=2.0 in /usr/local/lib/python3.10/dist-packages (from jinja2->torch) (2.1.2)\n",
+ "Requirement already satisfied: urllib3<1.27,>=1.21.1 in /usr/local/lib/python3.10/dist-packages (from requests->torchvision) (1.26.15)\n",
+ "Requirement already satisfied: certifi>=2017.4.17 in /usr/local/lib/python3.10/dist-packages (from requests->torchvision) (2022.12.7)\n",
+ "Requirement already satisfied: charset-normalizer~=2.0.0 in /usr/local/lib/python3.10/dist-packages (from requests->torchvision) (2.0.12)\n",
+ "Requirement already satisfied: idna<4,>=2.5 in /usr/local/lib/python3.10/dist-packages (from requests->torchvision) (3.4)\n",
+ "Requirement already satisfied: mpmath>=0.19 in /usr/local/lib/python3.10/dist-packages (from sympy->torch) (1.3.0)\n"
+ ]
+ }
+ ],
+ "source": [
+ "# install dependencies: (if your colab has CUDA 11.8)\n",
+ "%pip install torch torchvision torchaudio --index-url https://download.pytorch.org/whl/cu118"
+ ]
+ },
+ {
+ "cell_type": "code",
+ "execution_count": 4,
+ "metadata": {
+ "colab": {
+ "base_uri": "https://localhost:8080/"
},
+ "id": "5PAJ4ArzV5Ry",
+ "outputId": "eb8539a0-9524-4c48-f3e1-0b013ce0d344"
+ },
+ "outputs": [
{
- "cell_type": "code",
- "execution_count": 9,
- "metadata": {
- "colab": {
- "base_uri": "https://localhost:8080/"
- },
- "id": "NIyJXqfWathq",
- "outputId": "cb25aca9-e72d-4c54-f295-4c889713cb3a"
- },
- "outputs": [
- {
- "output_type": "stream",
- "name": "stdout",
- "text": [
- "The top-5 labels with corresponding scores are:\n",
- "arm wrestling: 1.0\n",
- "rock scissors paper: 6.434453414527752e-09\n",
- "shaking hands: 2.7599860175087088e-09\n",
- "clapping: 1.3454612979302283e-09\n",
- "massaging feet: 5.555100823784187e-10\n"
- ]
- }
- ],
- "source": [
- "print('The top-5 labels with corresponding scores are:')\n",
- "for result in results:\n",
- " print(f'{result[0]}: ', result[1])"
- ]
+ "output_type": "stream",
+ "name": "stdout",
+ "text": [
+ "Looking in indexes: https://pypi.org/simple, https://us-python.pkg.dev/colab-wheels/public/simple/\n",
+ "Collecting openmim\n",
+ " Downloading openmim-0.3.7-py2.py3-none-any.whl (51 kB)\n",
+ "\u001B[2K \u001B[90mโโโโโโโโโโโโโโโโโโโโโโโโโโโโโโโโโโโโโโโโ\u001B[0m \u001B[32m51.3/51.3 kB\u001B[0m \u001B[31m4.0 MB/s\u001B[0m eta \u001B[36m0:00:00\u001B[0m\n",
+ "\u001B[?25hRequirement already satisfied: Click in /usr/local/lib/python3.10/dist-packages (from openmim) (8.1.3)\n",
+ "Collecting colorama (from openmim)\n",
+ " Downloading colorama-0.4.6-py2.py3-none-any.whl (25 kB)\n",
+ "Collecting model-index (from openmim)\n",
+ " Downloading model_index-0.1.11-py3-none-any.whl (34 kB)\n",
+ "Requirement already satisfied: pandas in /usr/local/lib/python3.10/dist-packages (from openmim) (1.5.3)\n",
+ "Requirement already satisfied: pip>=19.3 in /usr/local/lib/python3.10/dist-packages (from openmim) (23.1.2)\n",
+ "Requirement already satisfied: requests in /usr/local/lib/python3.10/dist-packages (from openmim) (2.27.1)\n",
+ "Requirement already satisfied: rich in /usr/local/lib/python3.10/dist-packages (from openmim) (13.3.4)\n",
+ "Requirement already satisfied: tabulate in /usr/local/lib/python3.10/dist-packages (from openmim) (0.8.10)\n",
+ "Requirement already satisfied: pyyaml in /usr/local/lib/python3.10/dist-packages (from model-index->openmim) (6.0)\n",
+ "Requirement already satisfied: markdown in /usr/local/lib/python3.10/dist-packages (from model-index->openmim) (3.4.3)\n",
+ "Collecting ordered-set (from model-index->openmim)\n",
+ " Downloading ordered_set-4.1.0-py3-none-any.whl (7.6 kB)\n",
+ "Requirement already satisfied: python-dateutil>=2.8.1 in /usr/local/lib/python3.10/dist-packages (from pandas->openmim) (2.8.2)\n",
+ "Requirement already satisfied: pytz>=2020.1 in /usr/local/lib/python3.10/dist-packages (from pandas->openmim) (2022.7.1)\n",
+ "Requirement already satisfied: numpy>=1.21.0 in /usr/local/lib/python3.10/dist-packages (from pandas->openmim) (1.22.4)\n",
+ "Requirement already satisfied: urllib3<1.27,>=1.21.1 in /usr/local/lib/python3.10/dist-packages (from requests->openmim) (1.26.15)\n",
+ "Requirement already satisfied: certifi>=2017.4.17 in /usr/local/lib/python3.10/dist-packages (from requests->openmim) (2022.12.7)\n",
+ "Requirement already satisfied: charset-normalizer~=2.0.0 in /usr/local/lib/python3.10/dist-packages (from requests->openmim) (2.0.12)\n",
+ "Requirement already satisfied: idna<4,>=2.5 in /usr/local/lib/python3.10/dist-packages (from requests->openmim) (3.4)\n",
+ "Requirement already satisfied: markdown-it-py<3.0.0,>=2.2.0 in /usr/local/lib/python3.10/dist-packages (from rich->openmim) (2.2.0)\n",
+ "Requirement already satisfied: pygments<3.0.0,>=2.13.0 in /usr/local/lib/python3.10/dist-packages (from rich->openmim) (2.14.0)\n",
+ "Requirement already satisfied: mdurl~=0.1 in /usr/local/lib/python3.10/dist-packages (from markdown-it-py<3.0.0,>=2.2.0->rich->openmim) (0.1.2)\n",
+ "Requirement already satisfied: six>=1.5 in /usr/local/lib/python3.10/dist-packages (from python-dateutil>=2.8.1->pandas->openmim) (1.16.0)\n",
+ "Installing collected packages: ordered-set, colorama, model-index, openmim\n",
+ "Successfully installed colorama-0.4.6 model-index-0.1.11 openmim-0.3.7 ordered-set-4.1.0\n",
+ "Looking in indexes: https://pypi.org/simple, https://us-python.pkg.dev/colab-wheels/public/simple/\n",
+ "Looking in links: https://download.openmmlab.com/mmcv/dist/cu118/torch2.0.0/index.html\n",
+ "Collecting mmengine\n",
+ " Downloading mmengine-0.7.3-py3-none-any.whl (372 kB)\n",
+ "\u001B[2K \u001B[90mโโโโโโโโโโโโโโโโโโโโโโโโโโโโโโโโโโโโโโ\u001B[0m \u001B[32m372.1/372.1 kB\u001B[0m \u001B[31m20.5 MB/s\u001B[0m eta \u001B[36m0:00:00\u001B[0m\n",
+ "\u001B[?25hCollecting addict (from mmengine)\n",
+ " Downloading addict-2.4.0-py3-none-any.whl (3.8 kB)\n",
+ "Requirement already satisfied: matplotlib in /usr/local/lib/python3.10/dist-packages (from mmengine) (3.7.1)\n",
+ "Requirement already satisfied: numpy in /usr/local/lib/python3.10/dist-packages (from mmengine) (1.22.4)\n",
+ "Requirement already satisfied: pyyaml in /usr/local/lib/python3.10/dist-packages (from mmengine) (6.0)\n",
+ "Requirement already satisfied: rich in /usr/local/lib/python3.10/dist-packages (from mmengine) (13.3.4)\n",
+ "Requirement already satisfied: termcolor in /usr/local/lib/python3.10/dist-packages (from mmengine) (2.3.0)\n",
+ "Collecting yapf (from mmengine)\n",
+ " Downloading yapf-0.33.0-py2.py3-none-any.whl (200 kB)\n",
+ "\u001B[2K \u001B[90mโโโโโโโโโโโโโโโโโโโโโโโโโโโโโโโโโโโโโโ\u001B[0m \u001B[32m200.9/200.9 kB\u001B[0m \u001B[31m21.0 MB/s\u001B[0m eta \u001B[36m0:00:00\u001B[0m\n",
+ "\u001B[?25hRequirement already satisfied: opencv-python>=3 in /usr/local/lib/python3.10/dist-packages (from mmengine) (4.7.0.72)\n",
+ "Requirement already satisfied: contourpy>=1.0.1 in /usr/local/lib/python3.10/dist-packages (from matplotlib->mmengine) (1.0.7)\n",
+ "Requirement already satisfied: cycler>=0.10 in /usr/local/lib/python3.10/dist-packages (from matplotlib->mmengine) (0.11.0)\n",
+ "Requirement already satisfied: fonttools>=4.22.0 in /usr/local/lib/python3.10/dist-packages (from matplotlib->mmengine) (4.39.3)\n",
+ "Requirement already satisfied: kiwisolver>=1.0.1 in /usr/local/lib/python3.10/dist-packages (from matplotlib->mmengine) (1.4.4)\n",
+ "Requirement already satisfied: packaging>=20.0 in /usr/local/lib/python3.10/dist-packages (from matplotlib->mmengine) (23.1)\n",
+ "Requirement already satisfied: pillow>=6.2.0 in /usr/local/lib/python3.10/dist-packages (from matplotlib->mmengine) (8.4.0)\n",
+ "Requirement already satisfied: pyparsing>=2.3.1 in /usr/local/lib/python3.10/dist-packages (from matplotlib->mmengine) (3.0.9)\n",
+ "Requirement already satisfied: python-dateutil>=2.7 in /usr/local/lib/python3.10/dist-packages (from matplotlib->mmengine) (2.8.2)\n",
+ "Requirement already satisfied: markdown-it-py<3.0.0,>=2.2.0 in /usr/local/lib/python3.10/dist-packages (from rich->mmengine) (2.2.0)\n",
+ "Requirement already satisfied: pygments<3.0.0,>=2.13.0 in /usr/local/lib/python3.10/dist-packages (from rich->mmengine) (2.14.0)\n",
+ "Requirement already satisfied: tomli>=2.0.1 in /usr/local/lib/python3.10/dist-packages (from yapf->mmengine) (2.0.1)\n",
+ "Requirement already satisfied: mdurl~=0.1 in /usr/local/lib/python3.10/dist-packages (from markdown-it-py<3.0.0,>=2.2.0->rich->mmengine) (0.1.2)\n",
+ "Requirement already satisfied: six>=1.5 in /usr/local/lib/python3.10/dist-packages (from python-dateutil>=2.7->matplotlib->mmengine) (1.16.0)\n",
+ "Installing collected packages: addict, yapf, mmengine\n",
+ "Successfully installed addict-2.4.0 mmengine-0.7.3 yapf-0.33.0\n",
+ "Looking in indexes: https://pypi.org/simple, https://us-python.pkg.dev/colab-wheels/public/simple/\n",
+ "Looking in links: https://download.openmmlab.com/mmcv/dist/cu118/torch2.0.0/index.html\n",
+ "Collecting mmcv>=2.0.0\n",
+ " Downloading https://download.openmmlab.com/mmcv/dist/cu118/torch2.0.0/mmcv-2.0.0-cp310-cp310-manylinux1_x86_64.whl (74.4 MB)\n",
+ "\u001B[2K \u001B[90mโโโโโโโโโโโโโโโโโโโโโโโโโโโโโโโโโโโโโโโโ\u001B[0m \u001B[32m74.4/74.4 MB\u001B[0m \u001B[31m9.7 MB/s\u001B[0m eta \u001B[36m0:00:00\u001B[0m\n",
+ "\u001B[?25hRequirement already satisfied: addict in /usr/local/lib/python3.10/dist-packages (from mmcv>=2.0.0) (2.4.0)\n",
+ "Requirement already satisfied: mmengine>=0.2.0 in /usr/local/lib/python3.10/dist-packages (from mmcv>=2.0.0) (0.7.3)\n",
+ "Requirement already satisfied: numpy in /usr/local/lib/python3.10/dist-packages (from mmcv>=2.0.0) (1.22.4)\n",
+ "Requirement already satisfied: packaging in /usr/local/lib/python3.10/dist-packages (from mmcv>=2.0.0) (23.1)\n",
+ "Requirement already satisfied: Pillow in /usr/local/lib/python3.10/dist-packages (from mmcv>=2.0.0) (8.4.0)\n",
+ "Requirement already satisfied: pyyaml in /usr/local/lib/python3.10/dist-packages (from mmcv>=2.0.0) (6.0)\n",
+ "Requirement already satisfied: yapf in /usr/local/lib/python3.10/dist-packages (from mmcv>=2.0.0) (0.33.0)\n",
+ "Requirement already satisfied: opencv-python>=3 in /usr/local/lib/python3.10/dist-packages (from mmcv>=2.0.0) (4.7.0.72)\n",
+ "Requirement already satisfied: matplotlib in /usr/local/lib/python3.10/dist-packages (from mmengine>=0.2.0->mmcv>=2.0.0) (3.7.1)\n",
+ "Requirement already satisfied: rich in /usr/local/lib/python3.10/dist-packages (from mmengine>=0.2.0->mmcv>=2.0.0) (13.3.4)\n",
+ "Requirement already satisfied: termcolor in /usr/local/lib/python3.10/dist-packages (from mmengine>=0.2.0->mmcv>=2.0.0) (2.3.0)\n",
+ "Requirement already satisfied: tomli>=2.0.1 in /usr/local/lib/python3.10/dist-packages (from yapf->mmcv>=2.0.0) (2.0.1)\n",
+ "Requirement already satisfied: contourpy>=1.0.1 in /usr/local/lib/python3.10/dist-packages (from matplotlib->mmengine>=0.2.0->mmcv>=2.0.0) (1.0.7)\n",
+ "Requirement already satisfied: cycler>=0.10 in /usr/local/lib/python3.10/dist-packages (from matplotlib->mmengine>=0.2.0->mmcv>=2.0.0) (0.11.0)\n",
+ "Requirement already satisfied: fonttools>=4.22.0 in /usr/local/lib/python3.10/dist-packages (from matplotlib->mmengine>=0.2.0->mmcv>=2.0.0) (4.39.3)\n",
+ "Requirement already satisfied: kiwisolver>=1.0.1 in /usr/local/lib/python3.10/dist-packages (from matplotlib->mmengine>=0.2.0->mmcv>=2.0.0) (1.4.4)\n",
+ "Requirement already satisfied: pyparsing>=2.3.1 in /usr/local/lib/python3.10/dist-packages (from matplotlib->mmengine>=0.2.0->mmcv>=2.0.0) (3.0.9)\n",
+ "Requirement already satisfied: python-dateutil>=2.7 in /usr/local/lib/python3.10/dist-packages (from matplotlib->mmengine>=0.2.0->mmcv>=2.0.0) (2.8.2)\n",
+ "Requirement already satisfied: markdown-it-py<3.0.0,>=2.2.0 in /usr/local/lib/python3.10/dist-packages (from rich->mmengine>=0.2.0->mmcv>=2.0.0) (2.2.0)\n",
+ "Requirement already satisfied: pygments<3.0.0,>=2.13.0 in /usr/local/lib/python3.10/dist-packages (from rich->mmengine>=0.2.0->mmcv>=2.0.0) (2.14.0)\n",
+ "Requirement already satisfied: mdurl~=0.1 in /usr/local/lib/python3.10/dist-packages (from markdown-it-py<3.0.0,>=2.2.0->rich->mmengine>=0.2.0->mmcv>=2.0.0) (0.1.2)\n",
+ "Requirement already satisfied: six>=1.5 in /usr/local/lib/python3.10/dist-packages (from python-dateutil>=2.7->matplotlib->mmengine>=0.2.0->mmcv>=2.0.0) (1.16.0)\n",
+ "Installing collected packages: mmcv\n",
+ "Successfully installed mmcv-2.0.0\n",
+ "Cloning into 'mmaction2'...\n",
+ "remote: Enumerating objects: 21284, done.\u001B[K\n",
+ "remote: Counting objects: 100% (394/394), done.\u001B[K\n",
+ "remote: Compressing objects: 100% (287/287), done.\u001B[K\n",
+ "remote: Total 21284 (delta 175), reused 248 (delta 103), pack-reused 20890\u001B[K\n",
+ "Receiving objects: 100% (21284/21284), 68.63 MiB | 16.59 MiB/s, done.\n",
+ "Resolving deltas: 100% (14990/14990), done.\n",
+ "/content/mmaction2\n",
+ "Looking in indexes: https://pypi.org/simple, https://us-python.pkg.dev/colab-wheels/public/simple/\n",
+ "Obtaining file:///content/mmaction2\n",
+ " Preparing metadata (setup.py) ... \u001B[?25l\u001B[?25hdone\n",
+ "Collecting decord>=0.4.1 (from mmaction2==1.0.0)\n",
+ " Downloading decord-0.6.0-py3-none-manylinux2010_x86_64.whl (13.6 MB)\n",
+ "\u001B[2K \u001B[90mโโโโโโโโโโโโโโโโโโโโโโโโโโโโโโโโโโโโโโโโ\u001B[0m \u001B[32m13.6/13.6 MB\u001B[0m \u001B[31m76.9 MB/s\u001B[0m eta \u001B[36m0:00:00\u001B[0m\n",
+ "\u001B[?25hCollecting einops (from mmaction2==1.0.0)\n",
+ " Downloading einops-0.6.1-py3-none-any.whl (42 kB)\n",
+ "\u001B[2K \u001B[90mโโโโโโโโโโโโโโโโโโโโโโโโโโโโโโโโโโโโโโโโ\u001B[0m \u001B[32m42.2/42.2 kB\u001B[0m \u001B[31m4.5 MB/s\u001B[0m eta \u001B[36m0:00:00\u001B[0m\n",
+ "\u001B[?25hRequirement already satisfied: matplotlib in /usr/local/lib/python3.10/dist-packages (from mmaction2==1.0.0) (3.7.1)\n",
+ "Requirement already satisfied: numpy in /usr/local/lib/python3.10/dist-packages (from mmaction2==1.0.0) (1.22.4)\n",
+ "Requirement already satisfied: opencv-contrib-python in /usr/local/lib/python3.10/dist-packages (from mmaction2==1.0.0) (4.7.0.72)\n",
+ "Requirement already satisfied: Pillow in /usr/local/lib/python3.10/dist-packages (from mmaction2==1.0.0) (8.4.0)\n",
+ "Requirement already satisfied: scipy in /usr/local/lib/python3.10/dist-packages (from mmaction2==1.0.0) (1.10.1)\n",
+ "Requirement already satisfied: torch>=1.3 in /usr/local/lib/python3.10/dist-packages (from mmaction2==1.0.0) (2.0.0+cu118)\n",
+ "Requirement already satisfied: filelock in /usr/local/lib/python3.10/dist-packages (from torch>=1.3->mmaction2==1.0.0) (3.12.0)\n",
+ "Requirement already satisfied: typing-extensions in /usr/local/lib/python3.10/dist-packages (from torch>=1.3->mmaction2==1.0.0) (4.5.0)\n",
+ "Requirement already satisfied: sympy in /usr/local/lib/python3.10/dist-packages (from torch>=1.3->mmaction2==1.0.0) (1.11.1)\n",
+ "Requirement already satisfied: networkx in /usr/local/lib/python3.10/dist-packages (from torch>=1.3->mmaction2==1.0.0) (3.1)\n",
+ "Requirement already satisfied: jinja2 in /usr/local/lib/python3.10/dist-packages (from torch>=1.3->mmaction2==1.0.0) (3.1.2)\n",
+ "Requirement already satisfied: triton==2.0.0 in /usr/local/lib/python3.10/dist-packages (from torch>=1.3->mmaction2==1.0.0) (2.0.0)\n",
+ "Requirement already satisfied: cmake in /usr/local/lib/python3.10/dist-packages (from triton==2.0.0->torch>=1.3->mmaction2==1.0.0) (3.25.2)\n",
+ "Requirement already satisfied: lit in /usr/local/lib/python3.10/dist-packages (from triton==2.0.0->torch>=1.3->mmaction2==1.0.0) (16.0.3)\n",
+ "Requirement already satisfied: contourpy>=1.0.1 in /usr/local/lib/python3.10/dist-packages (from matplotlib->mmaction2==1.0.0) (1.0.7)\n",
+ "Requirement already satisfied: cycler>=0.10 in /usr/local/lib/python3.10/dist-packages (from matplotlib->mmaction2==1.0.0) (0.11.0)\n",
+ "Requirement already satisfied: fonttools>=4.22.0 in /usr/local/lib/python3.10/dist-packages (from matplotlib->mmaction2==1.0.0) (4.39.3)\n",
+ "Requirement already satisfied: kiwisolver>=1.0.1 in /usr/local/lib/python3.10/dist-packages (from matplotlib->mmaction2==1.0.0) (1.4.4)\n",
+ "Requirement already satisfied: packaging>=20.0 in /usr/local/lib/python3.10/dist-packages (from matplotlib->mmaction2==1.0.0) (23.1)\n",
+ "Requirement already satisfied: pyparsing>=2.3.1 in /usr/local/lib/python3.10/dist-packages (from matplotlib->mmaction2==1.0.0) (3.0.9)\n",
+ "Requirement already satisfied: python-dateutil>=2.7 in /usr/local/lib/python3.10/dist-packages (from matplotlib->mmaction2==1.0.0) (2.8.2)\n",
+ "Requirement already satisfied: six>=1.5 in /usr/local/lib/python3.10/dist-packages (from python-dateutil>=2.7->matplotlib->mmaction2==1.0.0) (1.16.0)\n",
+ "Requirement already satisfied: MarkupSafe>=2.0 in /usr/local/lib/python3.10/dist-packages (from jinja2->torch>=1.3->mmaction2==1.0.0) (2.1.2)\n",
+ "Requirement already satisfied: mpmath>=0.19 in /usr/local/lib/python3.10/dist-packages (from sympy->torch>=1.3->mmaction2==1.0.0) (1.3.0)\n",
+ "Installing collected packages: einops, decord, mmaction2\n",
+ " Running setup.py develop for mmaction2\n",
+ "Successfully installed decord-0.6.0 einops-0.6.1 mmaction2-1.0.0\n",
+ "Looking in indexes: https://pypi.org/simple, https://us-python.pkg.dev/colab-wheels/public/simple/\n",
+ "Collecting av>=9.0 (from -r requirements/optional.txt (line 1))\n",
+ " Downloading av-10.0.0-cp310-cp310-manylinux_2_17_x86_64.manylinux2014_x86_64.whl (31.0 MB)\n",
+ "\u001B[2K \u001B[90mโโโโโโโโโโโโโโโโโโโโโโโโโโโโโโโโโโโโโโโโ\u001B[0m \u001B[32m31.0/31.0 MB\u001B[0m \u001B[31m38.3 MB/s\u001B[0m eta \u001B[36m0:00:00\u001B[0m\n",
+ "\u001B[?25hRequirement already satisfied: future in /usr/local/lib/python3.10/dist-packages (from -r requirements/optional.txt (line 2)) (0.18.3)\n",
+ "Collecting fvcore (from -r requirements/optional.txt (line 3))\n",
+ " Downloading fvcore-0.1.5.post20221221.tar.gz (50 kB)\n",
+ "\u001B[2K \u001B[90mโโโโโโโโโโโโโโโโโโโโโโโโโโโโโโโโโโโโโโโโ\u001B[0m \u001B[32m50.2/50.2 kB\u001B[0m \u001B[31m6.7 MB/s\u001B[0m eta \u001B[36m0:00:00\u001B[0m\n",
+ "\u001B[?25h Preparing metadata (setup.py) ... \u001B[?25l\u001B[?25hdone\n",
+ "Requirement already satisfied: imgaug in /usr/local/lib/python3.10/dist-packages (from -r requirements/optional.txt (line 4)) (0.4.0)\n",
+ "Requirement already satisfied: librosa in /usr/local/lib/python3.10/dist-packages (from -r requirements/optional.txt (line 5)) (0.10.0.post2)\n",
+ "Collecting lmdb (from -r requirements/optional.txt (line 6))\n",
+ " Downloading lmdb-1.4.1-cp310-cp310-manylinux_2_17_x86_64.manylinux2014_x86_64.whl (299 kB)\n",
+ "\u001B[2K \u001B[90mโโโโโโโโโโโโโโโโโโโโโโโโโโโโโโโโโโโโโโ\u001B[0m \u001B[32m299.2/299.2 kB\u001B[0m \u001B[31m30.2 MB/s\u001B[0m eta \u001B[36m0:00:00\u001B[0m\n",
+ "\u001B[?25hRequirement already satisfied: moviepy in /usr/local/lib/python3.10/dist-packages (from -r requirements/optional.txt (line 7)) (1.0.3)\n",
+ "Requirement already satisfied: packaging in /usr/local/lib/python3.10/dist-packages (from -r requirements/optional.txt (line 8)) (23.1)\n",
+ "Collecting pims (from -r requirements/optional.txt (line 9))\n",
+ " Downloading PIMS-0.6.1.tar.gz (86 kB)\n",
+ "\u001B[2K \u001B[90mโโโโโโโโโโโโโโโโโโโโโโโโโโโโโโโโโโโโโโโโ\u001B[0m \u001B[32m86.0/86.0 kB\u001B[0m \u001B[31m12.2 MB/s\u001B[0m eta \u001B[36m0:00:00\u001B[0m\n",
+ "\u001B[?25h Preparing metadata (setup.py) ... \u001B[?25l\u001B[?25hdone\n",
+ "Collecting PyTurboJPEG (from -r requirements/optional.txt (line 10))\n",
+ " Downloading PyTurboJPEG-1.7.1.tar.gz (11 kB)\n",
+ " Preparing metadata (setup.py) ... \u001B[?25l\u001B[?25hdone\n",
+ "Requirement already satisfied: soundfile in /usr/local/lib/python3.10/dist-packages (from -r requirements/optional.txt (line 11)) (0.12.1)\n",
+ "Requirement already satisfied: tensorboard in /usr/local/lib/python3.10/dist-packages (from -r requirements/optional.txt (line 12)) (2.12.2)\n",
+ "Collecting wandb (from -r requirements/optional.txt (line 13))\n",
+ " Downloading wandb-0.15.2-py3-none-any.whl (2.0 MB)\n",
+ "\u001B[2K \u001B[90mโโโโโโโโโโโโโโโโโโโโโโโโโโโโโโโโโโโโโโโโ\u001B[0m \u001B[32m2.0/2.0 MB\u001B[0m \u001B[31m79.1 MB/s\u001B[0m eta \u001B[36m0:00:00\u001B[0m\n",
+ "\u001B[?25hRequirement already satisfied: numpy in /usr/local/lib/python3.10/dist-packages (from fvcore->-r requirements/optional.txt (line 3)) (1.22.4)\n",
+ "Collecting yacs>=0.1.6 (from fvcore->-r requirements/optional.txt (line 3))\n",
+ " Downloading yacs-0.1.8-py3-none-any.whl (14 kB)\n",
+ "Requirement already satisfied: pyyaml>=5.1 in /usr/local/lib/python3.10/dist-packages (from fvcore->-r requirements/optional.txt (line 3)) (6.0)\n",
+ "Requirement already satisfied: tqdm in /usr/local/lib/python3.10/dist-packages (from fvcore->-r requirements/optional.txt (line 3)) (4.65.0)\n",
+ "Requirement already satisfied: termcolor>=1.1 in /usr/local/lib/python3.10/dist-packages (from fvcore->-r requirements/optional.txt (line 3)) (2.3.0)\n",
+ "Requirement already satisfied: Pillow in /usr/local/lib/python3.10/dist-packages (from fvcore->-r requirements/optional.txt (line 3)) (8.4.0)\n",
+ "Requirement already satisfied: tabulate in /usr/local/lib/python3.10/dist-packages (from fvcore->-r requirements/optional.txt (line 3)) (0.8.10)\n",
+ "Collecting iopath>=0.1.7 (from fvcore->-r requirements/optional.txt (line 3))\n",
+ " Downloading iopath-0.1.10.tar.gz (42 kB)\n",
+ "\u001B[2K \u001B[90mโโโโโโโโโโโโโโโโโโโโโโโโโโโโโโโโโโโโโโโโ\u001B[0m \u001B[32m42.2/42.2 kB\u001B[0m \u001B[31m4.8 MB/s\u001B[0m eta \u001B[36m0:00:00\u001B[0m\n",
+ "\u001B[?25h Preparing metadata (setup.py) ... \u001B[?25l\u001B[?25hdone\n",
+ "Requirement already satisfied: six in /usr/local/lib/python3.10/dist-packages (from imgaug->-r requirements/optional.txt (line 4)) (1.16.0)\n",
+ "Requirement already satisfied: scipy in /usr/local/lib/python3.10/dist-packages (from imgaug->-r requirements/optional.txt (line 4)) (1.10.1)\n",
+ "Requirement already satisfied: matplotlib in /usr/local/lib/python3.10/dist-packages (from imgaug->-r requirements/optional.txt (line 4)) (3.7.1)\n",
+ "Requirement already satisfied: scikit-image>=0.14.2 in /usr/local/lib/python3.10/dist-packages (from imgaug->-r requirements/optional.txt (line 4)) (0.19.3)\n",
+ "Requirement already satisfied: opencv-python in /usr/local/lib/python3.10/dist-packages (from imgaug->-r requirements/optional.txt (line 4)) (4.7.0.72)\n",
+ "Requirement already satisfied: imageio in /usr/local/lib/python3.10/dist-packages (from imgaug->-r requirements/optional.txt (line 4)) (2.25.1)\n",
+ "Requirement already satisfied: Shapely in /usr/local/lib/python3.10/dist-packages (from imgaug->-r requirements/optional.txt (line 4)) (2.0.1)\n",
+ "Requirement already satisfied: audioread>=2.1.9 in /usr/local/lib/python3.10/dist-packages (from librosa->-r requirements/optional.txt (line 5)) (3.0.0)\n",
+ "Requirement already satisfied: scikit-learn>=0.20.0 in /usr/local/lib/python3.10/dist-packages (from librosa->-r requirements/optional.txt (line 5)) (1.2.2)\n",
+ "Requirement already satisfied: joblib>=0.14 in /usr/local/lib/python3.10/dist-packages (from librosa->-r requirements/optional.txt (line 5)) (1.2.0)\n",
+ "Requirement already satisfied: decorator>=4.3.0 in /usr/local/lib/python3.10/dist-packages (from librosa->-r requirements/optional.txt (line 5)) (4.4.2)\n",
+ "Requirement already satisfied: numba>=0.51.0 in /usr/local/lib/python3.10/dist-packages (from librosa->-r requirements/optional.txt (line 5)) (0.56.4)\n",
+ "Requirement already satisfied: pooch<1.7,>=1.0 in /usr/local/lib/python3.10/dist-packages (from librosa->-r requirements/optional.txt (line 5)) (1.6.0)\n",
+ "Requirement already satisfied: soxr>=0.3.2 in /usr/local/lib/python3.10/dist-packages (from librosa->-r requirements/optional.txt (line 5)) (0.3.5)\n",
+ "Requirement already satisfied: typing-extensions>=4.1.1 in /usr/local/lib/python3.10/dist-packages (from librosa->-r requirements/optional.txt (line 5)) (4.5.0)\n",
+ "Requirement already satisfied: lazy-loader>=0.1 in /usr/local/lib/python3.10/dist-packages (from librosa->-r requirements/optional.txt (line 5)) (0.2)\n",
+ "Requirement already satisfied: msgpack>=1.0 in /usr/local/lib/python3.10/dist-packages (from librosa->-r requirements/optional.txt (line 5)) (1.0.5)\n",
+ "Requirement already satisfied: requests<3.0,>=2.8.1 in /usr/local/lib/python3.10/dist-packages (from moviepy->-r requirements/optional.txt (line 7)) (2.27.1)\n",
+ "Requirement already satisfied: proglog<=1.0.0 in /usr/local/lib/python3.10/dist-packages (from moviepy->-r requirements/optional.txt (line 7)) (0.1.10)\n",
+ "Requirement already satisfied: imageio-ffmpeg>=0.2.0 in /usr/local/lib/python3.10/dist-packages (from moviepy->-r requirements/optional.txt (line 7)) (0.4.8)\n",
+ "Collecting slicerator>=0.9.8 (from pims->-r requirements/optional.txt (line 9))\n",
+ " Downloading slicerator-1.1.0-py3-none-any.whl (10 kB)\n",
+ "Requirement already satisfied: cffi>=1.0 in /usr/local/lib/python3.10/dist-packages (from soundfile->-r requirements/optional.txt (line 11)) (1.15.1)\n",
+ "Requirement already satisfied: absl-py>=0.4 in /usr/local/lib/python3.10/dist-packages (from tensorboard->-r requirements/optional.txt (line 12)) (1.4.0)\n",
+ "Requirement already satisfied: grpcio>=1.48.2 in /usr/local/lib/python3.10/dist-packages (from tensorboard->-r requirements/optional.txt (line 12)) (1.54.0)\n",
+ "Requirement already satisfied: google-auth<3,>=1.6.3 in /usr/local/lib/python3.10/dist-packages (from tensorboard->-r requirements/optional.txt (line 12)) (2.17.3)\n",
+ "Requirement already satisfied: google-auth-oauthlib<1.1,>=0.5 in /usr/local/lib/python3.10/dist-packages (from tensorboard->-r requirements/optional.txt (line 12)) (1.0.0)\n",
+ "Requirement already satisfied: markdown>=2.6.8 in /usr/local/lib/python3.10/dist-packages (from tensorboard->-r requirements/optional.txt (line 12)) (3.4.3)\n",
+ "Requirement already satisfied: protobuf>=3.19.6 in /usr/local/lib/python3.10/dist-packages (from tensorboard->-r requirements/optional.txt (line 12)) (3.20.3)\n",
+ "Requirement already satisfied: setuptools>=41.0.0 in /usr/local/lib/python3.10/dist-packages (from tensorboard->-r requirements/optional.txt (line 12)) (67.7.2)\n",
+ "Requirement already satisfied: tensorboard-data-server<0.8.0,>=0.7.0 in /usr/local/lib/python3.10/dist-packages (from tensorboard->-r requirements/optional.txt (line 12)) (0.7.0)\n",
+ "Requirement already satisfied: tensorboard-plugin-wit>=1.6.0 in /usr/local/lib/python3.10/dist-packages (from tensorboard->-r requirements/optional.txt (line 12)) (1.8.1)\n",
+ "Requirement already satisfied: werkzeug>=1.0.1 in /usr/local/lib/python3.10/dist-packages (from tensorboard->-r requirements/optional.txt (line 12)) (2.3.0)\n",
+ "Requirement already satisfied: wheel>=0.26 in /usr/local/lib/python3.10/dist-packages (from tensorboard->-r requirements/optional.txt (line 12)) (0.40.0)\n",
+ "Requirement already satisfied: Click!=8.0.0,>=7.0 in /usr/local/lib/python3.10/dist-packages (from wandb->-r requirements/optional.txt (line 13)) (8.1.3)\n",
+ "Collecting GitPython!=3.1.29,>=1.0.0 (from wandb->-r requirements/optional.txt (line 13))\n",
+ " Downloading GitPython-3.1.31-py3-none-any.whl (184 kB)\n",
+ "\u001B[2K \u001B[90mโโโโโโโโโโโโโโโโโโโโโโโโโโโโโโโโโโโโโโ\u001B[0m \u001B[32m184.3/184.3 kB\u001B[0m \u001B[31m22.9 MB/s\u001B[0m eta \u001B[36m0:00:00\u001B[0m\n",
+ "\u001B[?25hRequirement already satisfied: psutil>=5.0.0 in /usr/local/lib/python3.10/dist-packages (from wandb->-r requirements/optional.txt (line 13)) (5.9.5)\n",
+ "Collecting sentry-sdk>=1.0.0 (from wandb->-r requirements/optional.txt (line 13))\n",
+ " Downloading sentry_sdk-1.22.2-py2.py3-none-any.whl (203 kB)\n",
+ "\u001B[2K \u001B[90mโโโโโโโโโโโโโโโโโโโโโโโโโโโโโโโโโโโโโโ\u001B[0m \u001B[32m203.3/203.3 kB\u001B[0m \u001B[31m25.7 MB/s\u001B[0m eta \u001B[36m0:00:00\u001B[0m\n",
+ "\u001B[?25hCollecting docker-pycreds>=0.4.0 (from wandb->-r requirements/optional.txt (line 13))\n",
+ " Downloading docker_pycreds-0.4.0-py2.py3-none-any.whl (9.0 kB)\n",
+ "Collecting pathtools (from wandb->-r requirements/optional.txt (line 13))\n",
+ " Downloading pathtools-0.1.2.tar.gz (11 kB)\n",
+ " Preparing metadata (setup.py) ... \u001B[?25l\u001B[?25hdone\n",
+ "Collecting setproctitle (from wandb->-r requirements/optional.txt (line 13))\n",
+ " Downloading setproctitle-1.3.2-cp310-cp310-manylinux_2_5_x86_64.manylinux1_x86_64.manylinux_2_17_x86_64.manylinux2014_x86_64.whl (30 kB)\n",
+ "Requirement already satisfied: appdirs>=1.4.3 in /usr/local/lib/python3.10/dist-packages (from wandb->-r requirements/optional.txt (line 13)) (1.4.4)\n",
+ "Requirement already satisfied: pycparser in /usr/local/lib/python3.10/dist-packages (from cffi>=1.0->soundfile->-r requirements/optional.txt (line 11)) (2.21)\n",
+ "Collecting gitdb<5,>=4.0.1 (from GitPython!=3.1.29,>=1.0.0->wandb->-r requirements/optional.txt (line 13))\n",
+ " Downloading gitdb-4.0.10-py3-none-any.whl (62 kB)\n",
+ "\u001B[2K \u001B[90mโโโโโโโโโโโโโโโโโโโโโโโโโโโโโโโโโโโโโโโโ\u001B[0m \u001B[32m62.7/62.7 kB\u001B[0m \u001B[31m9.0 MB/s\u001B[0m eta \u001B[36m0:00:00\u001B[0m\n",
+ "\u001B[?25hRequirement already satisfied: cachetools<6.0,>=2.0.0 in /usr/local/lib/python3.10/dist-packages (from google-auth<3,>=1.6.3->tensorboard->-r requirements/optional.txt (line 12)) (5.3.0)\n",
+ "Requirement already satisfied: pyasn1-modules>=0.2.1 in /usr/local/lib/python3.10/dist-packages (from google-auth<3,>=1.6.3->tensorboard->-r requirements/optional.txt (line 12)) (0.3.0)\n",
+ "Requirement already satisfied: rsa<5,>=3.1.4 in /usr/local/lib/python3.10/dist-packages (from google-auth<3,>=1.6.3->tensorboard->-r requirements/optional.txt (line 12)) (4.9)\n",
+ "Requirement already satisfied: requests-oauthlib>=0.7.0 in /usr/local/lib/python3.10/dist-packages (from google-auth-oauthlib<1.1,>=0.5->tensorboard->-r requirements/optional.txt (line 12)) (1.3.1)\n",
+ "Collecting portalocker (from iopath>=0.1.7->fvcore->-r requirements/optional.txt (line 3))\n",
+ " Downloading portalocker-2.7.0-py2.py3-none-any.whl (15 kB)\n",
+ "Requirement already satisfied: llvmlite<0.40,>=0.39.0dev0 in /usr/local/lib/python3.10/dist-packages (from numba>=0.51.0->librosa->-r requirements/optional.txt (line 5)) (0.39.1)\n",
+ "Requirement already satisfied: urllib3<1.27,>=1.21.1 in /usr/local/lib/python3.10/dist-packages (from requests<3.0,>=2.8.1->moviepy->-r requirements/optional.txt (line 7)) (1.26.15)\n",
+ "Requirement already satisfied: certifi>=2017.4.17 in /usr/local/lib/python3.10/dist-packages (from requests<3.0,>=2.8.1->moviepy->-r requirements/optional.txt (line 7)) (2022.12.7)\n",
+ "Requirement already satisfied: charset-normalizer~=2.0.0 in /usr/local/lib/python3.10/dist-packages (from requests<3.0,>=2.8.1->moviepy->-r requirements/optional.txt (line 7)) (2.0.12)\n",
+ "Requirement already satisfied: idna<4,>=2.5 in /usr/local/lib/python3.10/dist-packages (from requests<3.0,>=2.8.1->moviepy->-r requirements/optional.txt (line 7)) (3.4)\n",
+ "Requirement already satisfied: networkx>=2.2 in /usr/local/lib/python3.10/dist-packages (from scikit-image>=0.14.2->imgaug->-r requirements/optional.txt (line 4)) (3.1)\n",
+ "Requirement already satisfied: tifffile>=2019.7.26 in /usr/local/lib/python3.10/dist-packages (from scikit-image>=0.14.2->imgaug->-r requirements/optional.txt (line 4)) (2023.4.12)\n",
+ "Requirement already satisfied: PyWavelets>=1.1.1 in /usr/local/lib/python3.10/dist-packages (from scikit-image>=0.14.2->imgaug->-r requirements/optional.txt (line 4)) (1.4.1)\n",
+ "Requirement already satisfied: threadpoolctl>=2.0.0 in /usr/local/lib/python3.10/dist-packages (from scikit-learn>=0.20.0->librosa->-r requirements/optional.txt (line 5)) (3.1.0)\n",
+ "Requirement already satisfied: MarkupSafe>=2.1.1 in /usr/local/lib/python3.10/dist-packages (from werkzeug>=1.0.1->tensorboard->-r requirements/optional.txt (line 12)) (2.1.2)\n",
+ "Requirement already satisfied: contourpy>=1.0.1 in /usr/local/lib/python3.10/dist-packages (from matplotlib->imgaug->-r requirements/optional.txt (line 4)) (1.0.7)\n",
+ "Requirement already satisfied: cycler>=0.10 in /usr/local/lib/python3.10/dist-packages (from matplotlib->imgaug->-r requirements/optional.txt (line 4)) (0.11.0)\n",
+ "Requirement already satisfied: fonttools>=4.22.0 in /usr/local/lib/python3.10/dist-packages (from matplotlib->imgaug->-r requirements/optional.txt (line 4)) (4.39.3)\n",
+ "Requirement already satisfied: kiwisolver>=1.0.1 in /usr/local/lib/python3.10/dist-packages (from matplotlib->imgaug->-r requirements/optional.txt (line 4)) (1.4.4)\n",
+ "Requirement already satisfied: pyparsing>=2.3.1 in /usr/local/lib/python3.10/dist-packages (from matplotlib->imgaug->-r requirements/optional.txt (line 4)) (3.0.9)\n",
+ "Requirement already satisfied: python-dateutil>=2.7 in /usr/local/lib/python3.10/dist-packages (from matplotlib->imgaug->-r requirements/optional.txt (line 4)) (2.8.2)\n",
+ "Collecting smmap<6,>=3.0.1 (from gitdb<5,>=4.0.1->GitPython!=3.1.29,>=1.0.0->wandb->-r requirements/optional.txt (line 13))\n",
+ " Downloading smmap-5.0.0-py3-none-any.whl (24 kB)\n",
+ "Requirement already satisfied: pyasn1<0.6.0,>=0.4.6 in /usr/local/lib/python3.10/dist-packages (from pyasn1-modules>=0.2.1->google-auth<3,>=1.6.3->tensorboard->-r requirements/optional.txt (line 12)) (0.5.0)\n",
+ "Requirement already satisfied: oauthlib>=3.0.0 in /usr/local/lib/python3.10/dist-packages (from requests-oauthlib>=0.7.0->google-auth-oauthlib<1.1,>=0.5->tensorboard->-r requirements/optional.txt (line 12)) (3.2.2)\n",
+ "Building wheels for collected packages: fvcore, pims, PyTurboJPEG, iopath, pathtools\n",
+ " Building wheel for fvcore (setup.py) ... \u001B[?25l\u001B[?25hdone\n",
+ " Created wheel for fvcore: filename=fvcore-0.1.5.post20221221-py3-none-any.whl size=61405 sha256=25c1e50155c8788d00eec898793c96133a746a8bb076ffc5c01f5a4dc256751e\n",
+ " Stored in directory: /root/.cache/pip/wheels/01/c0/af/77c1cf53a1be9e42a52b48e5af2169d40ec2e89f7362489dd0\n",
+ " Building wheel for pims (setup.py) ... \u001B[?25l\u001B[?25hdone\n",
+ " Created wheel for pims: filename=PIMS-0.6.1-py3-none-any.whl size=82619 sha256=59a328dc88a438c60cfb6e937e04c8a7dd55ad2a2905034cd41ff80cdbba6497\n",
+ " Stored in directory: /root/.cache/pip/wheels/cc/bf/3e/bfa77232d942f8244145f9c713b6b38f6ef04b6fb5c021c114\n",
+ " Building wheel for PyTurboJPEG (setup.py) ... \u001B[?25l\u001B[?25hdone\n",
+ " Created wheel for PyTurboJPEG: filename=PyTurboJPEG-1.7.1-py3-none-any.whl size=12243 sha256=ddf6424c85ac533335abd96dd9e98b014ea1dd4f143c88cd35ecb08d6128f411\n",
+ " Stored in directory: /root/.cache/pip/wheels/de/6e/b1/e7ba70c328c3395555cb92ca8820babb32950d867858b1948b\n",
+ " Building wheel for iopath (setup.py) ... \u001B[?25l\u001B[?25hdone\n",
+ " Created wheel for iopath: filename=iopath-0.1.10-py3-none-any.whl size=31531 sha256=db977a4344bebbdd710665e767caab4fbcf53cc6aea0707cd38d26c45718331e\n",
+ " Stored in directory: /root/.cache/pip/wheels/9a/a3/b6/ac0fcd1b4ed5cfeb3db92e6a0e476cfd48ed0df92b91080c1d\n",
+ " Building wheel for pathtools (setup.py) ... \u001B[?25l\u001B[?25hdone\n",
+ " Created wheel for pathtools: filename=pathtools-0.1.2-py3-none-any.whl size=8791 sha256=08bb5753ce029aef01f25c3e81882d93c0e040e5932e90a02a062ad058756b52\n",
+ " Stored in directory: /root/.cache/pip/wheels/e7/f3/22/152153d6eb222ee7a56ff8617d80ee5207207a8c00a7aab794\n",
+ "Successfully built fvcore pims PyTurboJPEG iopath pathtools\n",
+ "Installing collected packages: slicerator, pathtools, lmdb, av, yacs, smmap, setproctitle, sentry-sdk, PyTurboJPEG, portalocker, docker-pycreds, pims, iopath, gitdb, GitPython, fvcore, wandb\n",
+ "Successfully installed GitPython-3.1.31 PyTurboJPEG-1.7.1 av-10.0.0 docker-pycreds-0.4.0 fvcore-0.1.5.post20221221 gitdb-4.0.10 iopath-0.1.10 lmdb-1.4.1 pathtools-0.1.2 pims-0.6.1 portalocker-2.7.0 sentry-sdk-1.22.2 setproctitle-1.3.2 slicerator-1.1.0 smmap-5.0.0 wandb-0.15.2 yacs-0.1.8\n"
+ ]
+ }
+ ],
+ "source": [
+ "# install MMEngine, MMCV and MMDetection using MIM\n",
+ "%pip install -U openmim\n",
+ "!mim install mmengine\n",
+ "!mim install \"mmcv>=2.0.0\"\n",
+ "\n",
+ "# Install mmaction2\n",
+ "!rm -rf mmaction2\n",
+ "!git clone https://github.com/open-mmlab/mmaction2.git -b main\n",
+ "%cd mmaction2\n",
+ "\n",
+ "!pip install -e .\n",
+ "\n",
+ "# Install some optional requirements\n",
+ "!pip install -r requirements/optional.txt"
+ ]
+ },
+ {
+ "cell_type": "code",
+ "execution_count": 5,
+ "metadata": {
+ "colab": {
+ "base_uri": "https://localhost:8080/"
},
+ "id": "No_zZAFpWC-a",
+ "outputId": "9386dd81-2308-4adb-d3cb-798de11c035e"
+ },
+ "outputs": [
{
- "cell_type": "markdown",
- "metadata": {
- "id": "QuZG8kZ2fJ5d"
- },
- "source": [
- "## Train a recognizer on customized dataset\n",
- "\n",
- "To train a new recognizer, there are usually three things to do:\n",
- "1. Support a new dataset\n",
- "2. Modify the config\n",
- "3. Train a new recognizer"
- ]
+ "output_type": "stream",
+ "name": "stdout",
+ "text": [
+ "2.0.0+cu118 True\n",
+ "1.0.0\n",
+ "11.8\n",
+ "GCC 9.3\n",
+ "OrderedDict([('sys.platform', 'linux'), ('Python', '3.10.11 (main, Apr 5 2023, 14:15:10) [GCC 9.4.0]'), ('CUDA available', True), ('numpy_random_seed', 2147483648), ('GPU 0', 'Tesla T4'), ('CUDA_HOME', '/usr/local/cuda'), ('NVCC', 'Cuda compilation tools, release 11.8, V11.8.89'), ('GCC', 'x86_64-linux-gnu-gcc (Ubuntu 9.4.0-1ubuntu1~20.04.1) 9.4.0'), ('PyTorch', '2.0.0+cu118'), ('PyTorch compiling details', 'PyTorch built with:\\n - GCC 9.3\\n - C++ Version: 201703\\n - Intel(R) oneAPI Math Kernel Library Version 2022.2-Product Build 20220804 for Intel(R) 64 architecture applications\\n - Intel(R) MKL-DNN v2.7.3 (Git Hash 6dbeffbae1f23cbbeae17adb7b5b13f1f37c080e)\\n - OpenMP 201511 (a.k.a. OpenMP 4.5)\\n - LAPACK is enabled (usually provided by MKL)\\n - NNPACK is enabled\\n - CPU capability usage: AVX2\\n - CUDA Runtime 11.8\\n - NVCC architecture flags: -gencode;arch=compute_37,code=sm_37;-gencode;arch=compute_50,code=sm_50;-gencode;arch=compute_60,code=sm_60;-gencode;arch=compute_70,code=sm_70;-gencode;arch=compute_75,code=sm_75;-gencode;arch=compute_80,code=sm_80;-gencode;arch=compute_86,code=sm_86;-gencode;arch=compute_90,code=sm_90\\n - CuDNN 8.7\\n - Magma 2.6.1\\n - Build settings: BLAS_INFO=mkl, BUILD_TYPE=Release, CUDA_VERSION=11.8, CUDNN_VERSION=8.7.0, CXX_COMPILER=/opt/rh/devtoolset-9/root/usr/bin/c++, CXX_FLAGS= -D_GLIBCXX_USE_CXX11_ABI=0 -fabi-version=11 -Wno-deprecated -fvisibility-inlines-hidden -DUSE_PTHREADPOOL -DNDEBUG -DUSE_KINETO -DLIBKINETO_NOROCTRACER -DUSE_FBGEMM -DUSE_QNNPACK -DUSE_PYTORCH_QNNPACK -DUSE_XNNPACK -DSYMBOLICATE_MOBILE_DEBUG_HANDLE -O2 -fPIC -Wall -Wextra -Werror=return-type -Werror=non-virtual-dtor -Werror=bool-operation -Wnarrowing -Wno-missing-field-initializers -Wno-type-limits -Wno-array-bounds -Wno-unknown-pragmas -Wunused-local-typedefs -Wno-unused-parameter -Wno-unused-function -Wno-unused-result -Wno-strict-overflow -Wno-strict-aliasing -Wno-error=deprecated-declarations -Wno-stringop-overflow -Wno-psabi -Wno-error=pedantic -Wno-error=redundant-decls -Wno-error=old-style-cast -fdiagnostics-color=always -faligned-new -Wno-unused-but-set-variable -Wno-maybe-uninitialized -fno-math-errno -fno-trapping-math -Werror=format -Werror=cast-function-type -Wno-stringop-overflow, LAPACK_INFO=mkl, PERF_WITH_AVX=1, PERF_WITH_AVX2=1, PERF_WITH_AVX512=1, TORCH_DISABLE_GPU_ASSERTS=ON, TORCH_VERSION=2.0.0, USE_CUDA=ON, USE_CUDNN=ON, USE_EXCEPTION_PTR=1, USE_GFLAGS=OFF, USE_GLOG=OFF, USE_MKL=ON, USE_MKLDNN=ON, USE_MPI=OFF, USE_NCCL=1, USE_NNPACK=ON, USE_OPENMP=ON, USE_ROCM=OFF, \\n'), ('TorchVision', '0.15.1+cu118'), ('OpenCV', '4.7.0'), ('MMEngine', '0.7.3')])\n"
+ ]
+ }
+ ],
+ "source": [
+ "# Check Pytorch installation\n",
+ "import torch, torchvision\n",
+ "print(torch.__version__, torch.cuda.is_available())\n",
+ "\n",
+ "# Check MMAction2 installation\n",
+ "import mmaction\n",
+ "print(mmaction.__version__)\n",
+ "\n",
+ "# Check MMCV installation\n",
+ "from mmcv.ops import get_compiling_cuda_version, get_compiler_version\n",
+ "print(get_compiling_cuda_version())\n",
+ "print(get_compiler_version())\n",
+ "\n",
+ "# Check MMEngine installation\n",
+ "from mmengine.utils.dl_utils import collect_env\n",
+ "print(collect_env())"
+ ]
+ },
+ {
+ "cell_type": "markdown",
+ "metadata": {
+ "id": "pXf7oV5DWdab"
+ },
+ "source": [
+ "## Perform inference with a MMAction2 recognizer\n",
+ "MMAction2 already provides high level APIs to do inference and training."
+ ]
+ },
+ {
+ "cell_type": "code",
+ "execution_count": 6,
+ "metadata": {
+ "colab": {
+ "base_uri": "https://localhost:8080/"
},
+ "id": "64CW6d_AaT-Q",
+ "outputId": "ea330d8c-2e20-4dbd-d046-51d7c9ec4f7a"
+ },
+ "outputs": [
{
- "cell_type": "markdown",
- "metadata": {
- "id": "neEFyxChfgiJ"
- },
- "source": [
- "### Support a new dataset\n",
- "\n",
- "In this tutorial, we gives an example to convert the data into the format of existing datasets. Other methods and more advanced usages can be found in the [doc](/docs/tutorials/new_dataset.md)\n",
- "\n",
- "Firstly, let's download a tiny dataset obtained from [Kinetics-400](https://deepmind.com/research/open-source/open-source-datasets/kinetics/). We select 30 videos with their labels as train dataset and 10 videos with their labels as test dataset."
- ]
+ "output_type": "stream",
+ "name": "stdout",
+ "text": [
+ "--2023-05-15 03:33:08-- https://download.openmmlab.com/mmaction/recognition/tsn/tsn_r50_1x1x3_100e_kinetics400_rgb/tsn_r50_1x1x3_100e_kinetics400_rgb_20200614-e508be42.pth\n",
+ "Resolving download.openmmlab.com (download.openmmlab.com)... 163.181.82.216, 163.181.82.218, 163.181.82.213, ...\n",
+ "Connecting to download.openmmlab.com (download.openmmlab.com)|163.181.82.216|:443... connected.\n",
+ "HTTP request sent, awaiting response... 200 OK\n",
+ "Length: 97579339 (93M) [application/octet-stream]\n",
+ "Saving to: โcheckpoints/tsn_r50_1x1x3_100e_kinetics400_rgb_20200614-e508be42.pthโ\n",
+ "\n",
+ "checkpoints/tsn_r50 100%[===================>] 93.06M 26.1MB/s in 3.6s \n",
+ "\n",
+ "2023-05-15 03:33:12 (26.2 MB/s) - โcheckpoints/tsn_r50_1x1x3_100e_kinetics400_rgb_20200614-e508be42.pthโ saved [97579339/97579339]\n",
+ "\n"
+ ]
+ }
+ ],
+ "source": [
+ "!mkdir checkpoints\n",
+ "!wget -c https://download.openmmlab.com/mmaction/recognition/tsn/tsn_r50_1x1x3_100e_kinetics400_rgb/tsn_r50_1x1x3_100e_kinetics400_rgb_20200614-e508be42.pth \\\n",
+ " -O checkpoints/tsn_r50_1x1x3_100e_kinetics400_rgb_20200614-e508be42.pth"
+ ]
+ },
+ {
+ "cell_type": "code",
+ "execution_count": 7,
+ "metadata": {
+ "colab": {
+ "base_uri": "https://localhost:8080/"
},
+ "id": "HNZB7NoSabzj",
+ "outputId": "c0c2ba71-72ff-4cac-a5b8-65590f5a6bb0"
+ },
+ "outputs": [
{
- "cell_type": "code",
- "execution_count": 10,
- "metadata": {
- "colab": {
- "base_uri": "https://localhost:8080/"
- },
- "id": "gjsUj9JzgUlJ",
- "outputId": "96a0e6e9-0dd8-4c07-9fed-22b93d5c1318"
- },
- "outputs": [
- {
- "output_type": "stream",
- "name": "stdout",
- "text": [
- "rm: cannot remove 'kinetics400_tiny.zip*': No such file or directory\n",
- "--2023-05-15 03:33:27-- https://download.openmmlab.com/mmaction/kinetics400_tiny.zip\n",
- "Resolving download.openmmlab.com (download.openmmlab.com)... 163.181.82.216, 163.181.82.218, 163.181.82.213, ...\n",
- "Connecting to download.openmmlab.com (download.openmmlab.com)|163.181.82.216|:443... connected.\n",
- "HTTP request sent, awaiting response... 200 OK\n",
- "Length: 18308682 (17M) [application/zip]\n",
- "Saving to: โkinetics400_tiny.zipโ\n",
- "\n",
- "kinetics400_tiny.zi 100%[===================>] 17.46M 32.7MB/s in 0.5s \n",
- "\n",
- "2023-05-15 03:33:28 (32.7 MB/s) - โkinetics400_tiny.zipโ saved [18308682/18308682]\n",
- "\n"
- ]
- }
- ],
- "source": [
- "# download, decompress the data\n",
- "!rm kinetics400_tiny.zip*\n",
- "!rm -rf kinetics400_tiny\n",
- "!wget https://download.openmmlab.com/mmaction/kinetics400_tiny.zip\n",
- "!unzip kinetics400_tiny.zip > /dev/null"
- ]
- },
+ "output_type": "stream",
+ "name": "stdout",
+ "text": [
+ "Loads checkpoint by local backend from path: checkpoints/tsn_r50_1x1x3_100e_kinetics400_rgb_20200614-e508be42.pth\n"
+ ]
+ }
+ ],
+ "source": [
+ "from mmaction.apis import inference_recognizer, init_recognizer\n",
+ "from mmengine import Config\n",
+ "\n",
+ "\n",
+ "# Choose to use a config and initialize the recognizer\n",
+ "config = 'configs/recognition/tsn/tsn_imagenet-pretrained-r50_8xb32-1x1x3-100e_kinetics400-rgb.py'\n",
+ "config = Config.fromfile(config)\n",
+ "# Setup a checkpoint file to load\n",
+ "checkpoint = 'checkpoints/tsn_r50_1x1x3_100e_kinetics400_rgb_20200614-e508be42.pth'\n",
+ "# Initialize the recognizer\n",
+ "model = init_recognizer(config, checkpoint, device='cuda:0')"
+ ]
+ },
+ {
+ "cell_type": "code",
+ "execution_count": 8,
+ "metadata": {
+ "id": "rEMsBnpHapAn",
+ "outputId": "ec05049e-7289-4798-94fa-2b773cb23634",
+ "colab": {
+ "base_uri": "https://localhost:8080/"
+ }
+ },
+ "outputs": [
{
- "cell_type": "code",
- "execution_count": 11,
- "metadata": {
- "colab": {
- "base_uri": "https://localhost:8080/"
- },
- "id": "AbZ-o7V6hNw4",
- "outputId": "f229f352-1b43-41b7-a374-21404f618581"
- },
- "outputs": [
- {
- "output_type": "stream",
- "name": "stdout",
- "text": [
- "Reading package lists...\n",
- "Building dependency tree...\n",
- "Reading state information...\n",
- "The following NEW packages will be installed:\n",
- " tree\n",
- "0 upgraded, 1 newly installed, 0 to remove and 24 not upgraded.\n",
- "Need to get 43.0 kB of archives.\n",
- "After this operation, 115 kB of additional disk space will be used.\n",
- "Get:1 http://archive.ubuntu.com/ubuntu focal/universe amd64 tree amd64 1.8.0-1 [43.0 kB]\n",
- "Fetched 43.0 kB in 1s (48.9 kB/s)\n",
- "Selecting previously unselected package tree.\n",
- "(Reading database ... 122519 files and directories currently installed.)\n",
- "Preparing to unpack .../tree_1.8.0-1_amd64.deb ...\n",
- "Unpacking tree (1.8.0-1) ...\n",
- "Setting up tree (1.8.0-1) ...\n",
- "Processing triggers for man-db (2.9.1-1) ...\n",
- "\u001b[01;34mkinetics400_tiny\u001b[00m\n",
- "โโโ kinetics_tiny_train_video.txt\n",
- "โโโ kinetics_tiny_val_video.txt\n",
- "โโโ \u001b[01;34mtrain\u001b[00m\n",
- "โย ย โโโ 27_CSXByd3s.mp4\n",
- "โย ย โโโ 34XczvTaRiI.mp4\n",
- "โย ย โโโ A-wiliK50Zw.mp4\n",
- "โย ย โโโ D32_1gwq35E.mp4\n",
- "โย ย โโโ D92m0HsHjcQ.mp4\n",
- "โย ย โโโ DbX8mPslRXg.mp4\n",
- "โย ย โโโ FMlSTTpN3VY.mp4\n",
- "โย ย โโโ h10B9SVE-nk.mp4\n",
- "โย ย โโโ h2YqqUhnR34.mp4\n",
- "โย ย โโโ iRuyZSKhHRg.mp4\n",
- "โย ย โโโ IyfILH9lBRo.mp4\n",
- "โย ย โโโ kFC3KY2bOP8.mp4\n",
- "โย ย โโโ LvcFDgCAXQs.mp4\n",
- "โย ย โโโ O46YA8tI530.mp4\n",
- "โย ย โโโ oMrZaozOvdQ.mp4\n",
- "โย ย โโโ oXy-e_P_cAI.mp4\n",
- "โย ย โโโ P5M-hAts7MQ.mp4\n",
- "โย ย โโโ phDqGd0NKoo.mp4\n",
- "โย ย โโโ PnOe3GZRVX8.mp4\n",
- "โย ย โโโ R8HXQkdgKWA.mp4\n",
- "โย ย โโโ RqnKtCEoEcA.mp4\n",
- "โย ย โโโ soEcZZsBmDs.mp4\n",
- "โย ย โโโ TkkZPZHbAKA.mp4\n",
- "โย ย โโโ T_TMNGzVrDk.mp4\n",
- "โย ย โโโ WaS0qwP46Us.mp4\n",
- "โย ย โโโ Wh_YPQdH1Zg.mp4\n",
- "โย ย โโโ WWP5HZJsg-o.mp4\n",
- "โย ย โโโ xGY2dP0YUjA.mp4\n",
- "โย ย โโโ yLC9CtWU5ws.mp4\n",
- "โย ย โโโ ZQV4U2KQ370.mp4\n",
- "โโโ \u001b[01;34mval\u001b[00m\n",
- " โโโ 0pVGiAU6XEA.mp4\n",
- " โโโ AQrbRSnRt8M.mp4\n",
- " โโโ b6Q_b7vgc7Q.mp4\n",
- " โโโ ddvJ6-faICE.mp4\n",
- " โโโ IcLztCtvhb8.mp4\n",
- " โโโ ik4BW3-SCts.mp4\n",
- " โโโ jqRrH30V0k4.mp4\n",
- " โโโ SU_x2LQqSLs.mp4\n",
- " โโโ u4Rm6srmIS8.mp4\n",
- " โโโ y5Iu7XkTqV0.mp4\n",
- "\n",
- "2 directories, 42 files\n"
- ]
- }
- ],
- "source": [
- "# Check the directory structure of the tiny data\n",
- "\n",
- "# Install tree first\n",
- "!apt-get -q install tree\n",
- "!tree kinetics400_tiny"
- ]
+ "output_type": "stream",
+ "name": "stdout",
+ "text": [
+ "05/15 03:33:18 - mmengine - WARNING - \"FileClient\" will be deprecated in future. Please use io functions in https://mmengine.readthedocs.io/en/latest/api/fileio.html#file-io\n",
+ "05/15 03:33:18 - mmengine - WARNING - \"HardDiskBackend\" is the alias of \"LocalBackend\" and the former will be deprecated in future.\n"
+ ]
+ }
+ ],
+ "source": [
+ "# Use the recognizer to do inference\n",
+ "from operator import itemgetter\n",
+ "video = 'demo/demo.mp4'\n",
+ "label = 'tools/data/kinetics/label_map_k400.txt'\n",
+ "results = inference_recognizer(model, video)\n",
+ "\n",
+ "pred_scores = results.pred_score.tolist()\n",
+ "score_tuples = tuple(zip(range(len(pred_scores)), pred_scores))\n",
+ "score_sorted = sorted(score_tuples, key=itemgetter(1), reverse=True)\n",
+ "top5_label = score_sorted[:5]\n",
+ "\n",
+ "labels = open(label).readlines()\n",
+ "labels = [x.strip() for x in labels]\n",
+ "results = [(labels[k[0]], k[1]) for k in top5_label]\n"
+ ]
+ },
+ {
+ "cell_type": "code",
+ "execution_count": 9,
+ "metadata": {
+ "colab": {
+ "base_uri": "https://localhost:8080/"
},
+ "id": "NIyJXqfWathq",
+ "outputId": "cb25aca9-e72d-4c54-f295-4c889713cb3a"
+ },
+ "outputs": [
{
- "cell_type": "code",
- "execution_count": 12,
- "metadata": {
- "colab": {
- "base_uri": "https://localhost:8080/"
- },
- "id": "fTdi6dI0hY3g",
- "outputId": "95f22438-566c-4496-fe0c-50e128b47b5e"
- },
- "outputs": [
- {
- "output_type": "stream",
- "name": "stdout",
- "text": [
- "D32_1gwq35E.mp4 0\n",
- "iRuyZSKhHRg.mp4 1\n",
- "oXy-e_P_cAI.mp4 0\n",
- "34XczvTaRiI.mp4 1\n",
- "h2YqqUhnR34.mp4 0\n",
- "O46YA8tI530.mp4 0\n",
- "kFC3KY2bOP8.mp4 1\n",
- "WWP5HZJsg-o.mp4 1\n",
- "phDqGd0NKoo.mp4 1\n",
- "yLC9CtWU5ws.mp4 0\n",
- "27_CSXByd3s.mp4 1\n",
- "IyfILH9lBRo.mp4 1\n",
- "T_TMNGzVrDk.mp4 1\n",
- "TkkZPZHbAKA.mp4 0\n",
- "PnOe3GZRVX8.mp4 1\n",
- "soEcZZsBmDs.mp4 1\n",
- "FMlSTTpN3VY.mp4 1\n",
- "WaS0qwP46Us.mp4 0\n",
- "A-wiliK50Zw.mp4 1\n",
- "oMrZaozOvdQ.mp4 1\n",
- "ZQV4U2KQ370.mp4 0\n",
- "DbX8mPslRXg.mp4 1\n",
- "h10B9SVE-nk.mp4 1\n",
- "P5M-hAts7MQ.mp4 0\n",
- "R8HXQkdgKWA.mp4 0\n",
- "D92m0HsHjcQ.mp4 0\n",
- "RqnKtCEoEcA.mp4 0\n",
- "LvcFDgCAXQs.mp4 0\n",
- "xGY2dP0YUjA.mp4 0\n",
- "Wh_YPQdH1Zg.mp4 0\n"
- ]
- }
- ],
- "source": [
- "# After downloading the data, we need to check the annotation format\n",
- "!cat kinetics400_tiny/kinetics_tiny_train_video.txt"
- ]
+ "output_type": "stream",
+ "name": "stdout",
+ "text": [
+ "The top-5 labels with corresponding scores are:\n",
+ "arm wrestling: 1.0\n",
+ "rock scissors paper: 6.434453414527752e-09\n",
+ "shaking hands: 2.7599860175087088e-09\n",
+ "clapping: 1.3454612979302283e-09\n",
+ "massaging feet: 5.555100823784187e-10\n"
+ ]
+ }
+ ],
+ "source": [
+ "print('The top-5 labels with corresponding scores are:')\n",
+ "for result in results:\n",
+ " print(f'{result[0]}: ', result[1])"
+ ]
+ },
+ {
+ "cell_type": "markdown",
+ "metadata": {
+ "id": "QuZG8kZ2fJ5d"
+ },
+ "source": [
+ "## Train a recognizer on customized dataset\n",
+ "\n",
+ "To train a new recognizer, there are usually three things to do:\n",
+ "1. Support a new dataset\n",
+ "2. Modify the config\n",
+ "3. Train a new recognizer"
+ ]
+ },
+ {
+ "cell_type": "markdown",
+ "metadata": {
+ "id": "neEFyxChfgiJ"
+ },
+ "source": [
+ "### Support a new dataset\n",
+ "\n",
+ "In this tutorial, we gives an example to convert the data into the format of existing datasets. Other methods and more advanced usages can be found in the [doc](/docs/tutorials/new_dataset.md)\n",
+ "\n",
+ "Firstly, let's download a tiny dataset obtained from [Kinetics-400](https://deepmind.com/research/open-source/open-source-datasets/kinetics/). We select 30 videos with their labels as train dataset and 10 videos with their labels as test dataset."
+ ]
+ },
+ {
+ "cell_type": "code",
+ "execution_count": 10,
+ "metadata": {
+ "colab": {
+ "base_uri": "https://localhost:8080/"
},
+ "id": "gjsUj9JzgUlJ",
+ "outputId": "96a0e6e9-0dd8-4c07-9fed-22b93d5c1318"
+ },
+ "outputs": [
{
- "cell_type": "markdown",
- "metadata": {
- "id": "0bq0mxmEi29H"
- },
- "source": [
- "According to the format defined in [`VideoDataset`](./datasets/video_dataset.py), each line indicates a sample video with the filepath and label, which are split with a whitespace."
- ]
+ "output_type": "stream",
+ "name": "stdout",
+ "text": [
+ "rm: cannot remove 'kinetics400_tiny.zip*': No such file or directory\n",
+ "--2023-05-15 03:33:27-- https://download.openmmlab.com/mmaction/kinetics400_tiny.zip\n",
+ "Resolving download.openmmlab.com (download.openmmlab.com)... 163.181.82.216, 163.181.82.218, 163.181.82.213, ...\n",
+ "Connecting to download.openmmlab.com (download.openmmlab.com)|163.181.82.216|:443... connected.\n",
+ "HTTP request sent, awaiting response... 200 OK\n",
+ "Length: 18308682 (17M) [application/zip]\n",
+ "Saving to: โkinetics400_tiny.zipโ\n",
+ "\n",
+ "kinetics400_tiny.zi 100%[===================>] 17.46M 32.7MB/s in 0.5s \n",
+ "\n",
+ "2023-05-15 03:33:28 (32.7 MB/s) - โkinetics400_tiny.zipโ saved [18308682/18308682]\n",
+ "\n"
+ ]
+ }
+ ],
+ "source": [
+ "# download, decompress the data\n",
+ "!rm kinetics400_tiny.zip*\n",
+ "!rm -rf kinetics400_tiny\n",
+ "!wget https://download.openmmlab.com/mmaction/kinetics400_tiny.zip\n",
+ "!unzip kinetics400_tiny.zip > /dev/null"
+ ]
+ },
+ {
+ "cell_type": "code",
+ "execution_count": 11,
+ "metadata": {
+ "colab": {
+ "base_uri": "https://localhost:8080/"
},
+ "id": "AbZ-o7V6hNw4",
+ "outputId": "f229f352-1b43-41b7-a374-21404f618581"
+ },
+ "outputs": [
{
- "cell_type": "markdown",
- "metadata": {
- "id": "Ht_DGJA9jQar"
- },
- "source": [
- "### Modify the config\n",
- "\n",
- "In the next step, we need to modify the config for the training.\n",
- "To accelerate the process, we finetune a recognizer using a pre-trained recognizer."
- ]
+ "output_type": "stream",
+ "name": "stdout",
+ "text": [
+ "Reading package lists...\n",
+ "Building dependency tree...\n",
+ "Reading state information...\n",
+ "The following NEW packages will be installed:\n",
+ " tree\n",
+ "0 upgraded, 1 newly installed, 0 to remove and 24 not upgraded.\n",
+ "Need to get 43.0 kB of archives.\n",
+ "After this operation, 115 kB of additional disk space will be used.\n",
+ "Get:1 http://archive.ubuntu.com/ubuntu focal/universe amd64 tree amd64 1.8.0-1 [43.0 kB]\n",
+ "Fetched 43.0 kB in 1s (48.9 kB/s)\n",
+ "Selecting previously unselected package tree.\n",
+ "(Reading database ... 122519 files and directories currently installed.)\n",
+ "Preparing to unpack .../tree_1.8.0-1_amd64.deb ...\n",
+ "Unpacking tree (1.8.0-1) ...\n",
+ "Setting up tree (1.8.0-1) ...\n",
+ "Processing triggers for man-db (2.9.1-1) ...\n",
+ "\u001B[01;34mkinetics400_tiny\u001B[00m\n",
+ "โโโ kinetics_tiny_train_video.txt\n",
+ "โโโ kinetics_tiny_val_video.txt\n",
+ "โโโ \u001B[01;34mtrain\u001B[00m\n",
+ "โย ย โโโ 27_CSXByd3s.mp4\n",
+ "โย ย โโโ 34XczvTaRiI.mp4\n",
+ "โย ย โโโ A-wiliK50Zw.mp4\n",
+ "โย ย โโโ D32_1gwq35E.mp4\n",
+ "โย ย โโโ D92m0HsHjcQ.mp4\n",
+ "โย ย โโโ DbX8mPslRXg.mp4\n",
+ "โย ย โโโ FMlSTTpN3VY.mp4\n",
+ "โย ย โโโ h10B9SVE-nk.mp4\n",
+ "โย ย โโโ h2YqqUhnR34.mp4\n",
+ "โย ย โโโ iRuyZSKhHRg.mp4\n",
+ "โย ย โโโ IyfILH9lBRo.mp4\n",
+ "โย ย โโโ kFC3KY2bOP8.mp4\n",
+ "โย ย โโโ LvcFDgCAXQs.mp4\n",
+ "โย ย โโโ O46YA8tI530.mp4\n",
+ "โย ย โโโ oMrZaozOvdQ.mp4\n",
+ "โย ย โโโ oXy-e_P_cAI.mp4\n",
+ "โย ย โโโ P5M-hAts7MQ.mp4\n",
+ "โย ย โโโ phDqGd0NKoo.mp4\n",
+ "โย ย โโโ PnOe3GZRVX8.mp4\n",
+ "โย ย โโโ R8HXQkdgKWA.mp4\n",
+ "โย ย โโโ RqnKtCEoEcA.mp4\n",
+ "โย ย โโโ soEcZZsBmDs.mp4\n",
+ "โย ย โโโ TkkZPZHbAKA.mp4\n",
+ "โย ย โโโ T_TMNGzVrDk.mp4\n",
+ "โย ย โโโ WaS0qwP46Us.mp4\n",
+ "โย ย โโโ Wh_YPQdH1Zg.mp4\n",
+ "โย ย โโโ WWP5HZJsg-o.mp4\n",
+ "โย ย โโโ xGY2dP0YUjA.mp4\n",
+ "โย ย โโโ yLC9CtWU5ws.mp4\n",
+ "โย ย โโโ ZQV4U2KQ370.mp4\n",
+ "โโโ \u001B[01;34mval\u001B[00m\n",
+ " โโโ 0pVGiAU6XEA.mp4\n",
+ " โโโ AQrbRSnRt8M.mp4\n",
+ " โโโ b6Q_b7vgc7Q.mp4\n",
+ " โโโ ddvJ6-faICE.mp4\n",
+ " โโโ IcLztCtvhb8.mp4\n",
+ " โโโ ik4BW3-SCts.mp4\n",
+ " โโโ jqRrH30V0k4.mp4\n",
+ " โโโ SU_x2LQqSLs.mp4\n",
+ " โโโ u4Rm6srmIS8.mp4\n",
+ " โโโ y5Iu7XkTqV0.mp4\n",
+ "\n",
+ "2 directories, 42 files\n"
+ ]
+ }
+ ],
+ "source": [
+ "# Check the directory structure of the tiny data\n",
+ "\n",
+ "# Install tree first\n",
+ "!apt-get -q install tree\n",
+ "!tree kinetics400_tiny"
+ ]
+ },
+ {
+ "cell_type": "code",
+ "execution_count": 12,
+ "metadata": {
+ "colab": {
+ "base_uri": "https://localhost:8080/"
},
+ "id": "fTdi6dI0hY3g",
+ "outputId": "95f22438-566c-4496-fe0c-50e128b47b5e"
+ },
+ "outputs": [
{
- "cell_type": "code",
- "execution_count": 13,
- "metadata": {
- "id": "LjCcmCKOjktc"
- },
- "outputs": [],
- "source": [
- "cfg = Config.fromfile('./configs/recognition/tsn/tsn_imagenet-pretrained-r50_8xb32-1x1x3-100e_kinetics400-rgb.py')"
- ]
+ "output_type": "stream",
+ "name": "stdout",
+ "text": [
+ "D32_1gwq35E.mp4 0\n",
+ "iRuyZSKhHRg.mp4 1\n",
+ "oXy-e_P_cAI.mp4 0\n",
+ "34XczvTaRiI.mp4 1\n",
+ "h2YqqUhnR34.mp4 0\n",
+ "O46YA8tI530.mp4 0\n",
+ "kFC3KY2bOP8.mp4 1\n",
+ "WWP5HZJsg-o.mp4 1\n",
+ "phDqGd0NKoo.mp4 1\n",
+ "yLC9CtWU5ws.mp4 0\n",
+ "27_CSXByd3s.mp4 1\n",
+ "IyfILH9lBRo.mp4 1\n",
+ "T_TMNGzVrDk.mp4 1\n",
+ "TkkZPZHbAKA.mp4 0\n",
+ "PnOe3GZRVX8.mp4 1\n",
+ "soEcZZsBmDs.mp4 1\n",
+ "FMlSTTpN3VY.mp4 1\n",
+ "WaS0qwP46Us.mp4 0\n",
+ "A-wiliK50Zw.mp4 1\n",
+ "oMrZaozOvdQ.mp4 1\n",
+ "ZQV4U2KQ370.mp4 0\n",
+ "DbX8mPslRXg.mp4 1\n",
+ "h10B9SVE-nk.mp4 1\n",
+ "P5M-hAts7MQ.mp4 0\n",
+ "R8HXQkdgKWA.mp4 0\n",
+ "D92m0HsHjcQ.mp4 0\n",
+ "RqnKtCEoEcA.mp4 0\n",
+ "LvcFDgCAXQs.mp4 0\n",
+ "xGY2dP0YUjA.mp4 0\n",
+ "Wh_YPQdH1Zg.mp4 0\n"
+ ]
+ }
+ ],
+ "source": [
+ "# After downloading the data, we need to check the annotation format\n",
+ "!cat kinetics400_tiny/kinetics_tiny_train_video.txt"
+ ]
+ },
+ {
+ "cell_type": "markdown",
+ "metadata": {
+ "id": "0bq0mxmEi29H"
+ },
+ "source": [
+ "According to the format defined in [`VideoDataset`](./datasets/video_dataset.py), each line indicates a sample video with the filepath and label, which are split with a whitespace."
+ ]
+ },
+ {
+ "cell_type": "markdown",
+ "metadata": {
+ "id": "Ht_DGJA9jQar"
+ },
+ "source": [
+ "### Modify the config\n",
+ "\n",
+ "In the next step, we need to modify the config for the training.\n",
+ "To accelerate the process, we finetune a recognizer using a pre-trained recognizer."
+ ]
+ },
+ {
+ "cell_type": "code",
+ "execution_count": 13,
+ "metadata": {
+ "id": "LjCcmCKOjktc"
+ },
+ "outputs": [],
+ "source": [
+ "cfg = Config.fromfile('./configs/recognition/tsn/tsn_imagenet-pretrained-r50_8xb32-1x1x3-100e_kinetics400-rgb.py')"
+ ]
+ },
+ {
+ "cell_type": "markdown",
+ "metadata": {
+ "id": "tc8YhFFGjp3e"
+ },
+ "source": [
+ "Given a config that trains a TSN model on kinetics400-full dataset, we need to modify some values to use it for training TSN on Kinetics400-tiny dataset.\n"
+ ]
+ },
+ {
+ "cell_type": "code",
+ "execution_count": 14,
+ "metadata": {
+ "colab": {
+ "base_uri": "https://localhost:8080/"
},
+ "id": "tlhu9byjjt-K",
+ "outputId": "2d984a1d-93f7-493f-fd77-e19af8285f38"
+ },
+ "outputs": [
{
- "cell_type": "markdown",
- "metadata": {
- "id": "tc8YhFFGjp3e"
- },
- "source": [
- "Given a config that trains a TSN model on kinetics400-full dataset, we need to modify some values to use it for training TSN on Kinetics400-tiny dataset.\n"
- ]
+ "output_type": "stream",
+ "name": "stdout",
+ "text": [
+ "Config:\n",
+ "model = dict(\n",
+ " type='Recognizer2D',\n",
+ " backbone=dict(\n",
+ " type='ResNet',\n",
+ " pretrained='https://download.pytorch.org/models/resnet50-11ad3fa6.pth',\n",
+ " depth=50,\n",
+ " norm_eval=False),\n",
+ " cls_head=dict(\n",
+ " type='TSNHead',\n",
+ " num_classes=2,\n",
+ " in_channels=2048,\n",
+ " spatial_type='avg',\n",
+ " consensus=dict(type='AvgConsensus', dim=1),\n",
+ " dropout_ratio=0.4,\n",
+ " init_std=0.01,\n",
+ " average_clips='prob'),\n",
+ " data_preprocessor=dict(\n",
+ " type='ActionDataPreprocessor',\n",
+ " mean=[123.675, 116.28, 103.53],\n",
+ " std=[58.395, 57.12, 57.375],\n",
+ " format_shape='NCHW'),\n",
+ " train_cfg=None,\n",
+ " test_cfg=None)\n",
+ "train_cfg = dict(\n",
+ " type='EpochBasedTrainLoop', max_epochs=10, val_begin=1, val_interval=1)\n",
+ "val_cfg = dict(type='ValLoop')\n",
+ "test_cfg = dict(type='TestLoop')\n",
+ "param_scheduler = [\n",
+ " dict(\n",
+ " type='MultiStepLR',\n",
+ " begin=0,\n",
+ " end=100,\n",
+ " by_epoch=True,\n",
+ " milestones=[40, 80],\n",
+ " gamma=0.1)\n",
+ "]\n",
+ "optim_wrapper = dict(\n",
+ " optimizer=dict(\n",
+ " type='SGD', lr=7.8125e-05, momentum=0.9, weight_decay=0.0001),\n",
+ " clip_grad=dict(max_norm=40, norm_type=2))\n",
+ "default_scope = 'mmaction'\n",
+ "default_hooks = dict(\n",
+ " runtime_info=dict(type='RuntimeInfoHook'),\n",
+ " timer=dict(type='IterTimerHook'),\n",
+ " logger=dict(type='LoggerHook', interval=20, ignore_last=False),\n",
+ " param_scheduler=dict(type='ParamSchedulerHook'),\n",
+ " checkpoint=dict(\n",
+ " type='CheckpointHook', interval=3, save_best='auto', max_keep_ckpts=3),\n",
+ " sampler_seed=dict(type='DistSamplerSeedHook'),\n",
+ " sync_buffers=dict(type='SyncBuffersHook'))\n",
+ "env_cfg = dict(\n",
+ " cudnn_benchmark=False,\n",
+ " mp_cfg=dict(mp_start_method='fork', opencv_num_threads=0),\n",
+ " dist_cfg=dict(backend='nccl'))\n",
+ "log_processor = dict(type='LogProcessor', window_size=20, by_epoch=True)\n",
+ "vis_backends = [dict(type='LocalVisBackend')]\n",
+ "visualizer = dict(\n",
+ " type='ActionVisualizer', vis_backends=[dict(type='LocalVisBackend')])\n",
+ "log_level = 'INFO'\n",
+ "load_from = './checkpoints/tsn_r50_1x1x3_100e_kinetics400_rgb_20200614-e508be42.pth'\n",
+ "resume = False\n",
+ "dataset_type = 'VideoDataset'\n",
+ "data_root = 'kinetics400_tiny/train/'\n",
+ "data_root_val = 'kinetics400_tiny/val/'\n",
+ "ann_file_train = 'kinetics400_tiny/kinetics_tiny_train_video.txt'\n",
+ "ann_file_val = 'kinetics400_tiny/kinetics_tiny_val_video.txt'\n",
+ "file_client_args = dict(io_backend='disk')\n",
+ "train_pipeline = [\n",
+ " dict(type='DecordInit', io_backend='disk'),\n",
+ " dict(type='SampleFrames', clip_len=1, frame_interval=1, num_clips=3),\n",
+ " dict(type='DecordDecode'),\n",
+ " dict(type='Resize', scale=(-1, 256)),\n",
+ " dict(\n",
+ " type='MultiScaleCrop',\n",
+ " input_size=224,\n",
+ " scales=(1, 0.875, 0.75, 0.66),\n",
+ " random_crop=False,\n",
+ " max_wh_scale_gap=1),\n",
+ " dict(type='Resize', scale=(224, 224), keep_ratio=False),\n",
+ " dict(type='Flip', flip_ratio=0.5),\n",
+ " dict(type='FormatShape', input_format='NCHW'),\n",
+ " dict(type='PackActionInputs')\n",
+ "]\n",
+ "val_pipeline = [\n",
+ " dict(type='DecordInit', io_backend='disk'),\n",
+ " dict(\n",
+ " type='SampleFrames',\n",
+ " clip_len=1,\n",
+ " frame_interval=1,\n",
+ " num_clips=3,\n",
+ " test_mode=True),\n",
+ " dict(type='DecordDecode'),\n",
+ " dict(type='Resize', scale=(-1, 256)),\n",
+ " dict(type='CenterCrop', crop_size=224),\n",
+ " dict(type='FormatShape', input_format='NCHW'),\n",
+ " dict(type='PackActionInputs')\n",
+ "]\n",
+ "test_pipeline = [\n",
+ " dict(type='DecordInit', io_backend='disk'),\n",
+ " dict(\n",
+ " type='SampleFrames',\n",
+ " clip_len=1,\n",
+ " frame_interval=1,\n",
+ " num_clips=25,\n",
+ " test_mode=True),\n",
+ " dict(type='DecordDecode'),\n",
+ " dict(type='Resize', scale=(-1, 256)),\n",
+ " dict(type='TenCrop', crop_size=224),\n",
+ " dict(type='FormatShape', input_format='NCHW'),\n",
+ " dict(type='PackActionInputs')\n",
+ "]\n",
+ "train_dataloader = dict(\n",
+ " batch_size=2,\n",
+ " num_workers=2,\n",
+ " persistent_workers=True,\n",
+ " sampler=dict(type='DefaultSampler', shuffle=True),\n",
+ " dataset=dict(\n",
+ " type='VideoDataset',\n",
+ " ann_file='kinetics400_tiny/kinetics_tiny_train_video.txt',\n",
+ " data_prefix=dict(video='kinetics400_tiny/train/'),\n",
+ " pipeline=[\n",
+ " dict(type='DecordInit', io_backend='disk'),\n",
+ " dict(\n",
+ " type='SampleFrames', clip_len=1, frame_interval=1,\n",
+ " num_clips=3),\n",
+ " dict(type='DecordDecode'),\n",
+ " dict(type='Resize', scale=(-1, 256)),\n",
+ " dict(\n",
+ " type='MultiScaleCrop',\n",
+ " input_size=224,\n",
+ " scales=(1, 0.875, 0.75, 0.66),\n",
+ " random_crop=False,\n",
+ " max_wh_scale_gap=1),\n",
+ " dict(type='Resize', scale=(224, 224), keep_ratio=False),\n",
+ " dict(type='Flip', flip_ratio=0.5),\n",
+ " dict(type='FormatShape', input_format='NCHW'),\n",
+ " dict(type='PackActionInputs')\n",
+ " ]))\n",
+ "val_dataloader = dict(\n",
+ " batch_size=2,\n",
+ " num_workers=2,\n",
+ " persistent_workers=True,\n",
+ " sampler=dict(type='DefaultSampler', shuffle=False),\n",
+ " dataset=dict(\n",
+ " type='VideoDataset',\n",
+ " ann_file='kinetics400_tiny/kinetics_tiny_val_video.txt',\n",
+ " data_prefix=dict(video='kinetics400_tiny/val/'),\n",
+ " pipeline=[\n",
+ " dict(type='DecordInit', io_backend='disk'),\n",
+ " dict(\n",
+ " type='SampleFrames',\n",
+ " clip_len=1,\n",
+ " frame_interval=1,\n",
+ " num_clips=3,\n",
+ " test_mode=True),\n",
+ " dict(type='DecordDecode'),\n",
+ " dict(type='Resize', scale=(-1, 256)),\n",
+ " dict(type='CenterCrop', crop_size=224),\n",
+ " dict(type='FormatShape', input_format='NCHW'),\n",
+ " dict(type='PackActionInputs')\n",
+ " ],\n",
+ " test_mode=True))\n",
+ "test_dataloader = dict(\n",
+ " batch_size=1,\n",
+ " num_workers=2,\n",
+ " persistent_workers=True,\n",
+ " sampler=dict(type='DefaultSampler', shuffle=False),\n",
+ " dataset=dict(\n",
+ " type='VideoDataset',\n",
+ " ann_file='kinetics400_tiny/kinetics_tiny_val_video.txt',\n",
+ " data_prefix=dict(video='kinetics400_tiny/val/'),\n",
+ " pipeline=[\n",
+ " dict(type='DecordInit', io_backend='disk'),\n",
+ " dict(\n",
+ " type='SampleFrames',\n",
+ " clip_len=1,\n",
+ " frame_interval=1,\n",
+ " num_clips=25,\n",
+ " test_mode=True),\n",
+ " dict(type='DecordDecode'),\n",
+ " dict(type='Resize', scale=(-1, 256)),\n",
+ " dict(type='TenCrop', crop_size=224),\n",
+ " dict(type='FormatShape', input_format='NCHW'),\n",
+ " dict(type='PackActionInputs')\n",
+ " ],\n",
+ " test_mode=True))\n",
+ "val_evaluator = dict(type='AccMetric')\n",
+ "test_evaluator = dict(type='AccMetric')\n",
+ "auto_scale_lr = dict(enable=False, base_batch_size=256)\n",
+ "work_dir = './tutorial_exps'\n",
+ "\n"
+ ]
+ }
+ ],
+ "source": [
+ "from mmengine.runner import set_random_seed\n",
+ "\n",
+ "# Modify dataset type and path\n",
+ "cfg.data_root = 'kinetics400_tiny/train/'\n",
+ "cfg.data_root_val = 'kinetics400_tiny/val/'\n",
+ "cfg.ann_file_train = 'kinetics400_tiny/kinetics_tiny_train_video.txt'\n",
+ "cfg.ann_file_val = 'kinetics400_tiny/kinetics_tiny_val_video.txt'\n",
+ "\n",
+ "\n",
+ "cfg.test_dataloader.dataset.ann_file = 'kinetics400_tiny/kinetics_tiny_val_video.txt'\n",
+ "cfg.test_dataloader.dataset.data_prefix.video = 'kinetics400_tiny/val/'\n",
+ "\n",
+ "cfg.train_dataloader.dataset.ann_file = 'kinetics400_tiny/kinetics_tiny_train_video.txt'\n",
+ "cfg.train_dataloader.dataset.data_prefix.video = 'kinetics400_tiny/train/'\n",
+ "\n",
+ "cfg.val_dataloader.dataset.ann_file = 'kinetics400_tiny/kinetics_tiny_val_video.txt'\n",
+ "cfg.val_dataloader.dataset.data_prefix.video = 'kinetics400_tiny/val/'\n",
+ "\n",
+ "\n",
+ "# Modify num classes of the model in cls_head\n",
+ "cfg.model.cls_head.num_classes = 2\n",
+ "# We can use the pre-trained TSN model\n",
+ "cfg.load_from = './checkpoints/tsn_r50_1x1x3_100e_kinetics400_rgb_20200614-e508be42.pth'\n",
+ "\n",
+ "# Set up working dir to save files and logs.\n",
+ "cfg.work_dir = './tutorial_exps'\n",
+ "\n",
+ "# The original learning rate (LR) is set for 8-GPU training.\n",
+ "# We divide it by 8 since we only use one GPU.\n",
+ "cfg.train_dataloader.batch_size = cfg.train_dataloader.batch_size // 16\n",
+ "cfg.val_dataloader.batch_size = cfg.val_dataloader.batch_size // 16\n",
+ "cfg.optim_wrapper.optimizer.lr = cfg.optim_wrapper.optimizer.lr / 8 / 16\n",
+ "cfg.train_cfg.max_epochs = 10\n",
+ "\n",
+ "cfg.train_dataloader.num_workers = 2\n",
+ "cfg.val_dataloader.num_workers = 2\n",
+ "cfg.test_dataloader.num_workers = 2\n",
+ "\n",
+ "# We can initialize the logger for training and have a look\n",
+ "# at the final config used for training\n",
+ "print(f'Config:\\n{cfg.pretty_text}')\n"
+ ]
+ },
+ {
+ "cell_type": "markdown",
+ "metadata": {
+ "id": "tES-qnZ3k38Z"
+ },
+ "source": [
+ "### Train a new recognizer\n",
+ "\n",
+ "Finally, lets initialize the dataset and recognizer, then train a new recognizer!"
+ ]
+ },
+ {
+ "cell_type": "code",
+ "execution_count": 15,
+ "metadata": {
+ "colab": {
+ "base_uri": "https://localhost:8080/"
},
+ "id": "dDBWkdDRk6oz",
+ "outputId": "044b9e09-2038-41c9-d5a3-8a74ae11ade2"
+ },
+ "outputs": [
{
- "cell_type": "code",
- "execution_count": 14,
- "metadata": {
- "colab": {
- "base_uri": "https://localhost:8080/"
- },
- "id": "tlhu9byjjt-K",
- "outputId": "2d984a1d-93f7-493f-fd77-e19af8285f38"
- },
- "outputs": [
- {
- "output_type": "stream",
- "name": "stdout",
- "text": [
- "Config:\n",
- "model = dict(\n",
- " type='Recognizer2D',\n",
- " backbone=dict(\n",
- " type='ResNet',\n",
- " pretrained='https://download.pytorch.org/models/resnet50-11ad3fa6.pth',\n",
- " depth=50,\n",
- " norm_eval=False),\n",
- " cls_head=dict(\n",
- " type='TSNHead',\n",
- " num_classes=2,\n",
- " in_channels=2048,\n",
- " spatial_type='avg',\n",
- " consensus=dict(type='AvgConsensus', dim=1),\n",
- " dropout_ratio=0.4,\n",
- " init_std=0.01,\n",
- " average_clips='prob'),\n",
- " data_preprocessor=dict(\n",
- " type='ActionDataPreprocessor',\n",
- " mean=[123.675, 116.28, 103.53],\n",
- " std=[58.395, 57.12, 57.375],\n",
- " format_shape='NCHW'),\n",
- " train_cfg=None,\n",
- " test_cfg=None)\n",
- "train_cfg = dict(\n",
- " type='EpochBasedTrainLoop', max_epochs=10, val_begin=1, val_interval=1)\n",
- "val_cfg = dict(type='ValLoop')\n",
- "test_cfg = dict(type='TestLoop')\n",
- "param_scheduler = [\n",
- " dict(\n",
- " type='MultiStepLR',\n",
- " begin=0,\n",
- " end=100,\n",
- " by_epoch=True,\n",
- " milestones=[40, 80],\n",
- " gamma=0.1)\n",
- "]\n",
- "optim_wrapper = dict(\n",
- " optimizer=dict(\n",
- " type='SGD', lr=7.8125e-05, momentum=0.9, weight_decay=0.0001),\n",
- " clip_grad=dict(max_norm=40, norm_type=2))\n",
- "default_scope = 'mmaction'\n",
- "default_hooks = dict(\n",
- " runtime_info=dict(type='RuntimeInfoHook'),\n",
- " timer=dict(type='IterTimerHook'),\n",
- " logger=dict(type='LoggerHook', interval=20, ignore_last=False),\n",
- " param_scheduler=dict(type='ParamSchedulerHook'),\n",
- " checkpoint=dict(\n",
- " type='CheckpointHook', interval=3, save_best='auto', max_keep_ckpts=3),\n",
- " sampler_seed=dict(type='DistSamplerSeedHook'),\n",
- " sync_buffers=dict(type='SyncBuffersHook'))\n",
- "env_cfg = dict(\n",
- " cudnn_benchmark=False,\n",
- " mp_cfg=dict(mp_start_method='fork', opencv_num_threads=0),\n",
- " dist_cfg=dict(backend='nccl'))\n",
- "log_processor = dict(type='LogProcessor', window_size=20, by_epoch=True)\n",
- "vis_backends = [dict(type='LocalVisBackend')]\n",
- "visualizer = dict(\n",
- " type='ActionVisualizer', vis_backends=[dict(type='LocalVisBackend')])\n",
- "log_level = 'INFO'\n",
- "load_from = './checkpoints/tsn_r50_1x1x3_100e_kinetics400_rgb_20200614-e508be42.pth'\n",
- "resume = False\n",
- "dataset_type = 'VideoDataset'\n",
- "data_root = 'kinetics400_tiny/train/'\n",
- "data_root_val = 'kinetics400_tiny/val/'\n",
- "ann_file_train = 'kinetics400_tiny/kinetics_tiny_train_video.txt'\n",
- "ann_file_val = 'kinetics400_tiny/kinetics_tiny_val_video.txt'\n",
- "file_client_args = dict(io_backend='disk')\n",
- "train_pipeline = [\n",
- " dict(type='DecordInit', io_backend='disk'),\n",
- " dict(type='SampleFrames', clip_len=1, frame_interval=1, num_clips=3),\n",
- " dict(type='DecordDecode'),\n",
- " dict(type='Resize', scale=(-1, 256)),\n",
- " dict(\n",
- " type='MultiScaleCrop',\n",
- " input_size=224,\n",
- " scales=(1, 0.875, 0.75, 0.66),\n",
- " random_crop=False,\n",
- " max_wh_scale_gap=1),\n",
- " dict(type='Resize', scale=(224, 224), keep_ratio=False),\n",
- " dict(type='Flip', flip_ratio=0.5),\n",
- " dict(type='FormatShape', input_format='NCHW'),\n",
- " dict(type='PackActionInputs')\n",
- "]\n",
- "val_pipeline = [\n",
- " dict(type='DecordInit', io_backend='disk'),\n",
- " dict(\n",
- " type='SampleFrames',\n",
- " clip_len=1,\n",
- " frame_interval=1,\n",
- " num_clips=3,\n",
- " test_mode=True),\n",
- " dict(type='DecordDecode'),\n",
- " dict(type='Resize', scale=(-1, 256)),\n",
- " dict(type='CenterCrop', crop_size=224),\n",
- " dict(type='FormatShape', input_format='NCHW'),\n",
- " dict(type='PackActionInputs')\n",
- "]\n",
- "test_pipeline = [\n",
- " dict(type='DecordInit', io_backend='disk'),\n",
- " dict(\n",
- " type='SampleFrames',\n",
- " clip_len=1,\n",
- " frame_interval=1,\n",
- " num_clips=25,\n",
- " test_mode=True),\n",
- " dict(type='DecordDecode'),\n",
- " dict(type='Resize', scale=(-1, 256)),\n",
- " dict(type='TenCrop', crop_size=224),\n",
- " dict(type='FormatShape', input_format='NCHW'),\n",
- " dict(type='PackActionInputs')\n",
- "]\n",
- "train_dataloader = dict(\n",
- " batch_size=2,\n",
- " num_workers=2,\n",
- " persistent_workers=True,\n",
- " sampler=dict(type='DefaultSampler', shuffle=True),\n",
- " dataset=dict(\n",
- " type='VideoDataset',\n",
- " ann_file='kinetics400_tiny/kinetics_tiny_train_video.txt',\n",
- " data_prefix=dict(video='kinetics400_tiny/train/'),\n",
- " pipeline=[\n",
- " dict(type='DecordInit', io_backend='disk'),\n",
- " dict(\n",
- " type='SampleFrames', clip_len=1, frame_interval=1,\n",
- " num_clips=3),\n",
- " dict(type='DecordDecode'),\n",
- " dict(type='Resize', scale=(-1, 256)),\n",
- " dict(\n",
- " type='MultiScaleCrop',\n",
- " input_size=224,\n",
- " scales=(1, 0.875, 0.75, 0.66),\n",
- " random_crop=False,\n",
- " max_wh_scale_gap=1),\n",
- " dict(type='Resize', scale=(224, 224), keep_ratio=False),\n",
- " dict(type='Flip', flip_ratio=0.5),\n",
- " dict(type='FormatShape', input_format='NCHW'),\n",
- " dict(type='PackActionInputs')\n",
- " ]))\n",
- "val_dataloader = dict(\n",
- " batch_size=2,\n",
- " num_workers=2,\n",
- " persistent_workers=True,\n",
- " sampler=dict(type='DefaultSampler', shuffle=False),\n",
- " dataset=dict(\n",
- " type='VideoDataset',\n",
- " ann_file='kinetics400_tiny/kinetics_tiny_val_video.txt',\n",
- " data_prefix=dict(video='kinetics400_tiny/val/'),\n",
- " pipeline=[\n",
- " dict(type='DecordInit', io_backend='disk'),\n",
- " dict(\n",
- " type='SampleFrames',\n",
- " clip_len=1,\n",
- " frame_interval=1,\n",
- " num_clips=3,\n",
- " test_mode=True),\n",
- " dict(type='DecordDecode'),\n",
- " dict(type='Resize', scale=(-1, 256)),\n",
- " dict(type='CenterCrop', crop_size=224),\n",
- " dict(type='FormatShape', input_format='NCHW'),\n",
- " dict(type='PackActionInputs')\n",
- " ],\n",
- " test_mode=True))\n",
- "test_dataloader = dict(\n",
- " batch_size=1,\n",
- " num_workers=2,\n",
- " persistent_workers=True,\n",
- " sampler=dict(type='DefaultSampler', shuffle=False),\n",
- " dataset=dict(\n",
- " type='VideoDataset',\n",
- " ann_file='kinetics400_tiny/kinetics_tiny_val_video.txt',\n",
- " data_prefix=dict(video='kinetics400_tiny/val/'),\n",
- " pipeline=[\n",
- " dict(type='DecordInit', io_backend='disk'),\n",
- " dict(\n",
- " type='SampleFrames',\n",
- " clip_len=1,\n",
- " frame_interval=1,\n",
- " num_clips=25,\n",
- " test_mode=True),\n",
- " dict(type='DecordDecode'),\n",
- " dict(type='Resize', scale=(-1, 256)),\n",
- " dict(type='TenCrop', crop_size=224),\n",
- " dict(type='FormatShape', input_format='NCHW'),\n",
- " dict(type='PackActionInputs')\n",
- " ],\n",
- " test_mode=True))\n",
- "val_evaluator = dict(type='AccMetric')\n",
- "test_evaluator = dict(type='AccMetric')\n",
- "auto_scale_lr = dict(enable=False, base_batch_size=256)\n",
- "work_dir = './tutorial_exps'\n",
- "\n"
- ]
- }
- ],
- "source": [
- "from mmengine.runner import set_random_seed\n",
- "\n",
- "# Modify dataset type and path\n",
- "cfg.data_root = 'kinetics400_tiny/train/'\n",
- "cfg.data_root_val = 'kinetics400_tiny/val/'\n",
- "cfg.ann_file_train = 'kinetics400_tiny/kinetics_tiny_train_video.txt'\n",
- "cfg.ann_file_val = 'kinetics400_tiny/kinetics_tiny_val_video.txt'\n",
- "\n",
- "\n",
- "cfg.test_dataloader.dataset.ann_file = 'kinetics400_tiny/kinetics_tiny_val_video.txt'\n",
- "cfg.test_dataloader.dataset.data_prefix.video = 'kinetics400_tiny/val/'\n",
- "\n",
- "cfg.train_dataloader.dataset.ann_file = 'kinetics400_tiny/kinetics_tiny_train_video.txt'\n",
- "cfg.train_dataloader.dataset.data_prefix.video = 'kinetics400_tiny/train/'\n",
- "\n",
- "cfg.val_dataloader.dataset.ann_file = 'kinetics400_tiny/kinetics_tiny_val_video.txt'\n",
- "cfg.val_dataloader.dataset.data_prefix.video = 'kinetics400_tiny/val/'\n",
- "\n",
- "\n",
- "# Modify num classes of the model in cls_head\n",
- "cfg.model.cls_head.num_classes = 2\n",
- "# We can use the pre-trained TSN model\n",
- "cfg.load_from = './checkpoints/tsn_r50_1x1x3_100e_kinetics400_rgb_20200614-e508be42.pth'\n",
- "\n",
- "# Set up working dir to save files and logs.\n",
- "cfg.work_dir = './tutorial_exps'\n",
- "\n",
- "# The original learning rate (LR) is set for 8-GPU training.\n",
- "# We divide it by 8 since we only use one GPU.\n",
- "cfg.train_dataloader.batch_size = cfg.train_dataloader.batch_size // 16\n",
- "cfg.val_dataloader.batch_size = cfg.val_dataloader.batch_size // 16\n",
- "cfg.optim_wrapper.optimizer.lr = cfg.optim_wrapper.optimizer.lr / 8 / 16\n",
- "cfg.train_cfg.max_epochs = 10\n",
- "\n",
- "cfg.train_dataloader.num_workers = 2\n",
- "cfg.val_dataloader.num_workers = 2\n",
- "cfg.test_dataloader.num_workers = 2\n",
- "\n",
- "# We can initialize the logger for training and have a look\n",
- "# at the final config used for training\n",
- "print(f'Config:\\n{cfg.pretty_text}')\n"
- ]
+ "output_type": "stream",
+ "name": "stdout",
+ "text": [
+ "05/15 03:33:34 - mmengine - INFO - \n",
+ "------------------------------------------------------------\n",
+ "System environment:\n",
+ " sys.platform: linux\n",
+ " Python: 3.10.11 (main, Apr 5 2023, 14:15:10) [GCC 9.4.0]\n",
+ " CUDA available: True\n",
+ " numpy_random_seed: 1853452922\n",
+ " GPU 0: Tesla T4\n",
+ " CUDA_HOME: /usr/local/cuda\n",
+ " NVCC: Cuda compilation tools, release 11.8, V11.8.89\n",
+ " GCC: x86_64-linux-gnu-gcc (Ubuntu 9.4.0-1ubuntu1~20.04.1) 9.4.0\n",
+ " PyTorch: 2.0.0+cu118\n",
+ " PyTorch compiling details: PyTorch built with:\n",
+ " - GCC 9.3\n",
+ " - C++ Version: 201703\n",
+ " - Intel(R) oneAPI Math Kernel Library Version 2022.2-Product Build 20220804 for Intel(R) 64 architecture applications\n",
+ " - Intel(R) MKL-DNN v2.7.3 (Git Hash 6dbeffbae1f23cbbeae17adb7b5b13f1f37c080e)\n",
+ " - OpenMP 201511 (a.k.a. OpenMP 4.5)\n",
+ " - LAPACK is enabled (usually provided by MKL)\n",
+ " - NNPACK is enabled\n",
+ " - CPU capability usage: AVX2\n",
+ " - CUDA Runtime 11.8\n",
+ " - NVCC architecture flags: -gencode;arch=compute_37,code=sm_37;-gencode;arch=compute_50,code=sm_50;-gencode;arch=compute_60,code=sm_60;-gencode;arch=compute_70,code=sm_70;-gencode;arch=compute_75,code=sm_75;-gencode;arch=compute_80,code=sm_80;-gencode;arch=compute_86,code=sm_86;-gencode;arch=compute_90,code=sm_90\n",
+ " - CuDNN 8.7\n",
+ " - Magma 2.6.1\n",
+ " - Build settings: BLAS_INFO=mkl, BUILD_TYPE=Release, CUDA_VERSION=11.8, CUDNN_VERSION=8.7.0, CXX_COMPILER=/opt/rh/devtoolset-9/root/usr/bin/c++, CXX_FLAGS= -D_GLIBCXX_USE_CXX11_ABI=0 -fabi-version=11 -Wno-deprecated -fvisibility-inlines-hidden -DUSE_PTHREADPOOL -DNDEBUG -DUSE_KINETO -DLIBKINETO_NOROCTRACER -DUSE_FBGEMM -DUSE_QNNPACK -DUSE_PYTORCH_QNNPACK -DUSE_XNNPACK -DSYMBOLICATE_MOBILE_DEBUG_HANDLE -O2 -fPIC -Wall -Wextra -Werror=return-type -Werror=non-virtual-dtor -Werror=bool-operation -Wnarrowing -Wno-missing-field-initializers -Wno-type-limits -Wno-array-bounds -Wno-unknown-pragmas -Wunused-local-typedefs -Wno-unused-parameter -Wno-unused-function -Wno-unused-result -Wno-strict-overflow -Wno-strict-aliasing -Wno-error=deprecated-declarations -Wno-stringop-overflow -Wno-psabi -Wno-error=pedantic -Wno-error=redundant-decls -Wno-error=old-style-cast -fdiagnostics-color=always -faligned-new -Wno-unused-but-set-variable -Wno-maybe-uninitialized -fno-math-errno -fno-trapping-math -Werror=format -Werror=cast-function-type -Wno-stringop-overflow, LAPACK_INFO=mkl, PERF_WITH_AVX=1, PERF_WITH_AVX2=1, PERF_WITH_AVX512=1, TORCH_DISABLE_GPU_ASSERTS=ON, TORCH_VERSION=2.0.0, USE_CUDA=ON, USE_CUDNN=ON, USE_EXCEPTION_PTR=1, USE_GFLAGS=OFF, USE_GLOG=OFF, USE_MKL=ON, USE_MKLDNN=ON, USE_MPI=OFF, USE_NCCL=1, USE_NNPACK=ON, USE_OPENMP=ON, USE_ROCM=OFF, \n",
+ "\n",
+ " TorchVision: 0.15.1+cu118\n",
+ " OpenCV: 4.7.0\n",
+ " MMEngine: 0.7.3\n",
+ "\n",
+ "Runtime environment:\n",
+ " cudnn_benchmark: False\n",
+ " mp_cfg: {'mp_start_method': 'fork', 'opencv_num_threads': 0}\n",
+ " dist_cfg: {'backend': 'nccl'}\n",
+ " seed: None\n",
+ " Distributed launcher: none\n",
+ " Distributed training: False\n",
+ " GPU number: 1\n",
+ "------------------------------------------------------------\n",
+ "\n",
+ "05/15 03:33:34 - mmengine - INFO - Config:\n",
+ "model = dict(\n",
+ " type='Recognizer2D',\n",
+ " backbone=dict(\n",
+ " type='ResNet',\n",
+ " pretrained='https://download.pytorch.org/models/resnet50-11ad3fa6.pth',\n",
+ " depth=50,\n",
+ " norm_eval=False),\n",
+ " cls_head=dict(\n",
+ " type='TSNHead',\n",
+ " num_classes=2,\n",
+ " in_channels=2048,\n",
+ " spatial_type='avg',\n",
+ " consensus=dict(type='AvgConsensus', dim=1),\n",
+ " dropout_ratio=0.4,\n",
+ " init_std=0.01,\n",
+ " average_clips='prob'),\n",
+ " data_preprocessor=dict(\n",
+ " type='ActionDataPreprocessor',\n",
+ " mean=[123.675, 116.28, 103.53],\n",
+ " std=[58.395, 57.12, 57.375],\n",
+ " format_shape='NCHW'),\n",
+ " train_cfg=None,\n",
+ " test_cfg=None)\n",
+ "train_cfg = dict(\n",
+ " type='EpochBasedTrainLoop', max_epochs=10, val_begin=1, val_interval=1)\n",
+ "val_cfg = dict(type='ValLoop')\n",
+ "test_cfg = dict(type='TestLoop')\n",
+ "param_scheduler = [\n",
+ " dict(\n",
+ " type='MultiStepLR',\n",
+ " begin=0,\n",
+ " end=100,\n",
+ " by_epoch=True,\n",
+ " milestones=[40, 80],\n",
+ " gamma=0.1)\n",
+ "]\n",
+ "optim_wrapper = dict(\n",
+ " optimizer=dict(\n",
+ " type='SGD', lr=7.8125e-05, momentum=0.9, weight_decay=0.0001),\n",
+ " clip_grad=dict(max_norm=40, norm_type=2))\n",
+ "default_scope = 'mmaction'\n",
+ "default_hooks = dict(\n",
+ " runtime_info=dict(type='RuntimeInfoHook'),\n",
+ " timer=dict(type='IterTimerHook'),\n",
+ " logger=dict(type='LoggerHook', interval=20, ignore_last=False),\n",
+ " param_scheduler=dict(type='ParamSchedulerHook'),\n",
+ " checkpoint=dict(\n",
+ " type='CheckpointHook', interval=3, save_best='auto', max_keep_ckpts=3),\n",
+ " sampler_seed=dict(type='DistSamplerSeedHook'),\n",
+ " sync_buffers=dict(type='SyncBuffersHook'))\n",
+ "env_cfg = dict(\n",
+ " cudnn_benchmark=False,\n",
+ " mp_cfg=dict(mp_start_method='fork', opencv_num_threads=0),\n",
+ " dist_cfg=dict(backend='nccl'))\n",
+ "log_processor = dict(type='LogProcessor', window_size=20, by_epoch=True)\n",
+ "vis_backends = [dict(type='LocalVisBackend')]\n",
+ "visualizer = dict(\n",
+ " type='ActionVisualizer', vis_backends=[dict(type='LocalVisBackend')])\n",
+ "log_level = 'INFO'\n",
+ "load_from = './checkpoints/tsn_r50_1x1x3_100e_kinetics400_rgb_20200614-e508be42.pth'\n",
+ "resume = False\n",
+ "dataset_type = 'VideoDataset'\n",
+ "data_root = 'kinetics400_tiny/train/'\n",
+ "data_root_val = 'kinetics400_tiny/val/'\n",
+ "ann_file_train = 'kinetics400_tiny/kinetics_tiny_train_video.txt'\n",
+ "ann_file_val = 'kinetics400_tiny/kinetics_tiny_val_video.txt'\n",
+ "file_client_args = dict(io_backend='disk')\n",
+ "train_pipeline = [\n",
+ " dict(type='DecordInit', io_backend='disk'),\n",
+ " dict(type='SampleFrames', clip_len=1, frame_interval=1, num_clips=3),\n",
+ " dict(type='DecordDecode'),\n",
+ " dict(type='Resize', scale=(-1, 256)),\n",
+ " dict(\n",
+ " type='MultiScaleCrop',\n",
+ " input_size=224,\n",
+ " scales=(1, 0.875, 0.75, 0.66),\n",
+ " random_crop=False,\n",
+ " max_wh_scale_gap=1),\n",
+ " dict(type='Resize', scale=(224, 224), keep_ratio=False),\n",
+ " dict(type='Flip', flip_ratio=0.5),\n",
+ " dict(type='FormatShape', input_format='NCHW'),\n",
+ " dict(type='PackActionInputs')\n",
+ "]\n",
+ "val_pipeline = [\n",
+ " dict(type='DecordInit', io_backend='disk'),\n",
+ " dict(\n",
+ " type='SampleFrames',\n",
+ " clip_len=1,\n",
+ " frame_interval=1,\n",
+ " num_clips=3,\n",
+ " test_mode=True),\n",
+ " dict(type='DecordDecode'),\n",
+ " dict(type='Resize', scale=(-1, 256)),\n",
+ " dict(type='CenterCrop', crop_size=224),\n",
+ " dict(type='FormatShape', input_format='NCHW'),\n",
+ " dict(type='PackActionInputs')\n",
+ "]\n",
+ "test_pipeline = [\n",
+ " dict(type='DecordInit', io_backend='disk'),\n",
+ " dict(\n",
+ " type='SampleFrames',\n",
+ " clip_len=1,\n",
+ " frame_interval=1,\n",
+ " num_clips=25,\n",
+ " test_mode=True),\n",
+ " dict(type='DecordDecode'),\n",
+ " dict(type='Resize', scale=(-1, 256)),\n",
+ " dict(type='TenCrop', crop_size=224),\n",
+ " dict(type='FormatShape', input_format='NCHW'),\n",
+ " dict(type='PackActionInputs')\n",
+ "]\n",
+ "train_dataloader = dict(\n",
+ " batch_size=2,\n",
+ " num_workers=2,\n",
+ " persistent_workers=True,\n",
+ " sampler=dict(type='DefaultSampler', shuffle=True),\n",
+ " dataset=dict(\n",
+ " type='VideoDataset',\n",
+ " ann_file='kinetics400_tiny/kinetics_tiny_train_video.txt',\n",
+ " data_prefix=dict(video='kinetics400_tiny/train/'),\n",
+ " pipeline=[\n",
+ " dict(type='DecordInit', io_backend='disk'),\n",
+ " dict(\n",
+ " type='SampleFrames', clip_len=1, frame_interval=1,\n",
+ " num_clips=3),\n",
+ " dict(type='DecordDecode'),\n",
+ " dict(type='Resize', scale=(-1, 256)),\n",
+ " dict(\n",
+ " type='MultiScaleCrop',\n",
+ " input_size=224,\n",
+ " scales=(1, 0.875, 0.75, 0.66),\n",
+ " random_crop=False,\n",
+ " max_wh_scale_gap=1),\n",
+ " dict(type='Resize', scale=(224, 224), keep_ratio=False),\n",
+ " dict(type='Flip', flip_ratio=0.5),\n",
+ " dict(type='FormatShape', input_format='NCHW'),\n",
+ " dict(type='PackActionInputs')\n",
+ " ]))\n",
+ "val_dataloader = dict(\n",
+ " batch_size=2,\n",
+ " num_workers=2,\n",
+ " persistent_workers=True,\n",
+ " sampler=dict(type='DefaultSampler', shuffle=False),\n",
+ " dataset=dict(\n",
+ " type='VideoDataset',\n",
+ " ann_file='kinetics400_tiny/kinetics_tiny_val_video.txt',\n",
+ " data_prefix=dict(video='kinetics400_tiny/val/'),\n",
+ " pipeline=[\n",
+ " dict(type='DecordInit', io_backend='disk'),\n",
+ " dict(\n",
+ " type='SampleFrames',\n",
+ " clip_len=1,\n",
+ " frame_interval=1,\n",
+ " num_clips=3,\n",
+ " test_mode=True),\n",
+ " dict(type='DecordDecode'),\n",
+ " dict(type='Resize', scale=(-1, 256)),\n",
+ " dict(type='CenterCrop', crop_size=224),\n",
+ " dict(type='FormatShape', input_format='NCHW'),\n",
+ " dict(type='PackActionInputs')\n",
+ " ],\n",
+ " test_mode=True))\n",
+ "test_dataloader = dict(\n",
+ " batch_size=1,\n",
+ " num_workers=2,\n",
+ " persistent_workers=True,\n",
+ " sampler=dict(type='DefaultSampler', shuffle=False),\n",
+ " dataset=dict(\n",
+ " type='VideoDataset',\n",
+ " ann_file='kinetics400_tiny/kinetics_tiny_val_video.txt',\n",
+ " data_prefix=dict(video='kinetics400_tiny/val/'),\n",
+ " pipeline=[\n",
+ " dict(type='DecordInit', io_backend='disk'),\n",
+ " dict(\n",
+ " type='SampleFrames',\n",
+ " clip_len=1,\n",
+ " frame_interval=1,\n",
+ " num_clips=25,\n",
+ " test_mode=True),\n",
+ " dict(type='DecordDecode'),\n",
+ " dict(type='Resize', scale=(-1, 256)),\n",
+ " dict(type='TenCrop', crop_size=224),\n",
+ " dict(type='FormatShape', input_format='NCHW'),\n",
+ " dict(type='PackActionInputs')\n",
+ " ],\n",
+ " test_mode=True))\n",
+ "val_evaluator = dict(type='AccMetric')\n",
+ "test_evaluator = dict(type='AccMetric')\n",
+ "auto_scale_lr = dict(enable=False, base_batch_size=256)\n",
+ "work_dir = './tutorial_exps'\n",
+ "\n",
+ "05/15 03:33:35 - mmengine - INFO - Distributed training is not used, all SyncBatchNorm (SyncBN) layers in the model will be automatically reverted to BatchNormXd layers if they are used.\n",
+ "05/15 03:33:35 - mmengine - INFO - Hooks will be executed in the following order:\n",
+ "before_run:\n",
+ "(VERY_HIGH ) RuntimeInfoHook \n",
+ "(BELOW_NORMAL) LoggerHook \n",
+ " -------------------- \n",
+ "before_train:\n",
+ "(VERY_HIGH ) RuntimeInfoHook \n",
+ "(NORMAL ) IterTimerHook \n",
+ "(VERY_LOW ) CheckpointHook \n",
+ " -------------------- \n",
+ "before_train_epoch:\n",
+ "(VERY_HIGH ) RuntimeInfoHook \n",
+ "(NORMAL ) IterTimerHook \n",
+ "(NORMAL ) DistSamplerSeedHook \n",
+ " -------------------- \n",
+ "before_train_iter:\n",
+ "(VERY_HIGH ) RuntimeInfoHook \n",
+ "(NORMAL ) IterTimerHook \n",
+ " -------------------- \n",
+ "after_train_iter:\n",
+ "(VERY_HIGH ) RuntimeInfoHook \n",
+ "(NORMAL ) IterTimerHook \n",
+ "(BELOW_NORMAL) LoggerHook \n",
+ "(LOW ) ParamSchedulerHook \n",
+ "(VERY_LOW ) CheckpointHook \n",
+ " -------------------- \n",
+ "after_train_epoch:\n",
+ "(NORMAL ) IterTimerHook \n",
+ "(NORMAL ) SyncBuffersHook \n",
+ "(LOW ) ParamSchedulerHook \n",
+ "(VERY_LOW ) CheckpointHook \n",
+ " -------------------- \n",
+ "before_val_epoch:\n",
+ "(NORMAL ) IterTimerHook \n",
+ "(NORMAL ) SyncBuffersHook \n",
+ " -------------------- \n",
+ "before_val_iter:\n",
+ "(NORMAL ) IterTimerHook \n",
+ " -------------------- \n",
+ "after_val_iter:\n",
+ "(NORMAL ) IterTimerHook \n",
+ "(BELOW_NORMAL) LoggerHook \n",
+ " -------------------- \n",
+ "after_val_epoch:\n",
+ "(VERY_HIGH ) RuntimeInfoHook \n",
+ "(NORMAL ) IterTimerHook \n",
+ "(BELOW_NORMAL) LoggerHook \n",
+ "(LOW ) ParamSchedulerHook \n",
+ "(VERY_LOW ) CheckpointHook \n",
+ " -------------------- \n",
+ "after_train:\n",
+ "(VERY_LOW ) CheckpointHook \n",
+ " -------------------- \n",
+ "before_test_epoch:\n",
+ "(NORMAL ) IterTimerHook \n",
+ " -------------------- \n",
+ "before_test_iter:\n",
+ "(NORMAL ) IterTimerHook \n",
+ " -------------------- \n",
+ "after_test_iter:\n",
+ "(NORMAL ) IterTimerHook \n",
+ "(BELOW_NORMAL) LoggerHook \n",
+ " -------------------- \n",
+ "after_test_epoch:\n",
+ "(VERY_HIGH ) RuntimeInfoHook \n",
+ "(NORMAL ) IterTimerHook \n",
+ "(BELOW_NORMAL) LoggerHook \n",
+ " -------------------- \n",
+ "after_run:\n",
+ "(BELOW_NORMAL) LoggerHook \n",
+ " -------------------- \n",
+ "Loads checkpoint by http backend from path: https://download.pytorch.org/models/resnet50-11ad3fa6.pth\n"
+ ]
},
{
- "cell_type": "markdown",
- "metadata": {
- "id": "tES-qnZ3k38Z"
- },
- "source": [
- "### Train a new recognizer\n",
- "\n",
- "Finally, lets initialize the dataset and recognizer, then train a new recognizer!"
- ]
+ "output_type": "stream",
+ "name": "stderr",
+ "text": [
+ "Downloading: \"https://download.pytorch.org/models/resnet50-11ad3fa6.pth\" to /root/.cache/torch/hub/checkpoints/resnet50-11ad3fa6.pth\n"
+ ]
},
{
- "cell_type": "code",
- "execution_count": 15,
- "metadata": {
- "colab": {
- "base_uri": "https://localhost:8080/"
- },
- "id": "dDBWkdDRk6oz",
- "outputId": "044b9e09-2038-41c9-d5a3-8a74ae11ade2"
- },
- "outputs": [
- {
- "output_type": "stream",
- "name": "stdout",
- "text": [
- "05/15 03:33:34 - mmengine - INFO - \n",
- "------------------------------------------------------------\n",
- "System environment:\n",
- " sys.platform: linux\n",
- " Python: 3.10.11 (main, Apr 5 2023, 14:15:10) [GCC 9.4.0]\n",
- " CUDA available: True\n",
- " numpy_random_seed: 1853452922\n",
- " GPU 0: Tesla T4\n",
- " CUDA_HOME: /usr/local/cuda\n",
- " NVCC: Cuda compilation tools, release 11.8, V11.8.89\n",
- " GCC: x86_64-linux-gnu-gcc (Ubuntu 9.4.0-1ubuntu1~20.04.1) 9.4.0\n",
- " PyTorch: 2.0.0+cu118\n",
- " PyTorch compiling details: PyTorch built with:\n",
- " - GCC 9.3\n",
- " - C++ Version: 201703\n",
- " - Intel(R) oneAPI Math Kernel Library Version 2022.2-Product Build 20220804 for Intel(R) 64 architecture applications\n",
- " - Intel(R) MKL-DNN v2.7.3 (Git Hash 6dbeffbae1f23cbbeae17adb7b5b13f1f37c080e)\n",
- " - OpenMP 201511 (a.k.a. OpenMP 4.5)\n",
- " - LAPACK is enabled (usually provided by MKL)\n",
- " - NNPACK is enabled\n",
- " - CPU capability usage: AVX2\n",
- " - CUDA Runtime 11.8\n",
- " - NVCC architecture flags: -gencode;arch=compute_37,code=sm_37;-gencode;arch=compute_50,code=sm_50;-gencode;arch=compute_60,code=sm_60;-gencode;arch=compute_70,code=sm_70;-gencode;arch=compute_75,code=sm_75;-gencode;arch=compute_80,code=sm_80;-gencode;arch=compute_86,code=sm_86;-gencode;arch=compute_90,code=sm_90\n",
- " - CuDNN 8.7\n",
- " - Magma 2.6.1\n",
- " - Build settings: BLAS_INFO=mkl, BUILD_TYPE=Release, CUDA_VERSION=11.8, CUDNN_VERSION=8.7.0, CXX_COMPILER=/opt/rh/devtoolset-9/root/usr/bin/c++, CXX_FLAGS= -D_GLIBCXX_USE_CXX11_ABI=0 -fabi-version=11 -Wno-deprecated -fvisibility-inlines-hidden -DUSE_PTHREADPOOL -DNDEBUG -DUSE_KINETO -DLIBKINETO_NOROCTRACER -DUSE_FBGEMM -DUSE_QNNPACK -DUSE_PYTORCH_QNNPACK -DUSE_XNNPACK -DSYMBOLICATE_MOBILE_DEBUG_HANDLE -O2 -fPIC -Wall -Wextra -Werror=return-type -Werror=non-virtual-dtor -Werror=bool-operation -Wnarrowing -Wno-missing-field-initializers -Wno-type-limits -Wno-array-bounds -Wno-unknown-pragmas -Wunused-local-typedefs -Wno-unused-parameter -Wno-unused-function -Wno-unused-result -Wno-strict-overflow -Wno-strict-aliasing -Wno-error=deprecated-declarations -Wno-stringop-overflow -Wno-psabi -Wno-error=pedantic -Wno-error=redundant-decls -Wno-error=old-style-cast -fdiagnostics-color=always -faligned-new -Wno-unused-but-set-variable -Wno-maybe-uninitialized -fno-math-errno -fno-trapping-math -Werror=format -Werror=cast-function-type -Wno-stringop-overflow, LAPACK_INFO=mkl, PERF_WITH_AVX=1, PERF_WITH_AVX2=1, PERF_WITH_AVX512=1, TORCH_DISABLE_GPU_ASSERTS=ON, TORCH_VERSION=2.0.0, USE_CUDA=ON, USE_CUDNN=ON, USE_EXCEPTION_PTR=1, USE_GFLAGS=OFF, USE_GLOG=OFF, USE_MKL=ON, USE_MKLDNN=ON, USE_MPI=OFF, USE_NCCL=1, USE_NNPACK=ON, USE_OPENMP=ON, USE_ROCM=OFF, \n",
- "\n",
- " TorchVision: 0.15.1+cu118\n",
- " OpenCV: 4.7.0\n",
- " MMEngine: 0.7.3\n",
- "\n",
- "Runtime environment:\n",
- " cudnn_benchmark: False\n",
- " mp_cfg: {'mp_start_method': 'fork', 'opencv_num_threads': 0}\n",
- " dist_cfg: {'backend': 'nccl'}\n",
- " seed: None\n",
- " Distributed launcher: none\n",
- " Distributed training: False\n",
- " GPU number: 1\n",
- "------------------------------------------------------------\n",
- "\n",
- "05/15 03:33:34 - mmengine - INFO - Config:\n",
- "model = dict(\n",
- " type='Recognizer2D',\n",
- " backbone=dict(\n",
- " type='ResNet',\n",
- " pretrained='https://download.pytorch.org/models/resnet50-11ad3fa6.pth',\n",
- " depth=50,\n",
- " norm_eval=False),\n",
- " cls_head=dict(\n",
- " type='TSNHead',\n",
- " num_classes=2,\n",
- " in_channels=2048,\n",
- " spatial_type='avg',\n",
- " consensus=dict(type='AvgConsensus', dim=1),\n",
- " dropout_ratio=0.4,\n",
- " init_std=0.01,\n",
- " average_clips='prob'),\n",
- " data_preprocessor=dict(\n",
- " type='ActionDataPreprocessor',\n",
- " mean=[123.675, 116.28, 103.53],\n",
- " std=[58.395, 57.12, 57.375],\n",
- " format_shape='NCHW'),\n",
- " train_cfg=None,\n",
- " test_cfg=None)\n",
- "train_cfg = dict(\n",
- " type='EpochBasedTrainLoop', max_epochs=10, val_begin=1, val_interval=1)\n",
- "val_cfg = dict(type='ValLoop')\n",
- "test_cfg = dict(type='TestLoop')\n",
- "param_scheduler = [\n",
- " dict(\n",
- " type='MultiStepLR',\n",
- " begin=0,\n",
- " end=100,\n",
- " by_epoch=True,\n",
- " milestones=[40, 80],\n",
- " gamma=0.1)\n",
- "]\n",
- "optim_wrapper = dict(\n",
- " optimizer=dict(\n",
- " type='SGD', lr=7.8125e-05, momentum=0.9, weight_decay=0.0001),\n",
- " clip_grad=dict(max_norm=40, norm_type=2))\n",
- "default_scope = 'mmaction'\n",
- "default_hooks = dict(\n",
- " runtime_info=dict(type='RuntimeInfoHook'),\n",
- " timer=dict(type='IterTimerHook'),\n",
- " logger=dict(type='LoggerHook', interval=20, ignore_last=False),\n",
- " param_scheduler=dict(type='ParamSchedulerHook'),\n",
- " checkpoint=dict(\n",
- " type='CheckpointHook', interval=3, save_best='auto', max_keep_ckpts=3),\n",
- " sampler_seed=dict(type='DistSamplerSeedHook'),\n",
- " sync_buffers=dict(type='SyncBuffersHook'))\n",
- "env_cfg = dict(\n",
- " cudnn_benchmark=False,\n",
- " mp_cfg=dict(mp_start_method='fork', opencv_num_threads=0),\n",
- " dist_cfg=dict(backend='nccl'))\n",
- "log_processor = dict(type='LogProcessor', window_size=20, by_epoch=True)\n",
- "vis_backends = [dict(type='LocalVisBackend')]\n",
- "visualizer = dict(\n",
- " type='ActionVisualizer', vis_backends=[dict(type='LocalVisBackend')])\n",
- "log_level = 'INFO'\n",
- "load_from = './checkpoints/tsn_r50_1x1x3_100e_kinetics400_rgb_20200614-e508be42.pth'\n",
- "resume = False\n",
- "dataset_type = 'VideoDataset'\n",
- "data_root = 'kinetics400_tiny/train/'\n",
- "data_root_val = 'kinetics400_tiny/val/'\n",
- "ann_file_train = 'kinetics400_tiny/kinetics_tiny_train_video.txt'\n",
- "ann_file_val = 'kinetics400_tiny/kinetics_tiny_val_video.txt'\n",
- "file_client_args = dict(io_backend='disk')\n",
- "train_pipeline = [\n",
- " dict(type='DecordInit', io_backend='disk'),\n",
- " dict(type='SampleFrames', clip_len=1, frame_interval=1, num_clips=3),\n",
- " dict(type='DecordDecode'),\n",
- " dict(type='Resize', scale=(-1, 256)),\n",
- " dict(\n",
- " type='MultiScaleCrop',\n",
- " input_size=224,\n",
- " scales=(1, 0.875, 0.75, 0.66),\n",
- " random_crop=False,\n",
- " max_wh_scale_gap=1),\n",
- " dict(type='Resize', scale=(224, 224), keep_ratio=False),\n",
- " dict(type='Flip', flip_ratio=0.5),\n",
- " dict(type='FormatShape', input_format='NCHW'),\n",
- " dict(type='PackActionInputs')\n",
- "]\n",
- "val_pipeline = [\n",
- " dict(type='DecordInit', io_backend='disk'),\n",
- " dict(\n",
- " type='SampleFrames',\n",
- " clip_len=1,\n",
- " frame_interval=1,\n",
- " num_clips=3,\n",
- " test_mode=True),\n",
- " dict(type='DecordDecode'),\n",
- " dict(type='Resize', scale=(-1, 256)),\n",
- " dict(type='CenterCrop', crop_size=224),\n",
- " dict(type='FormatShape', input_format='NCHW'),\n",
- " dict(type='PackActionInputs')\n",
- "]\n",
- "test_pipeline = [\n",
- " dict(type='DecordInit', io_backend='disk'),\n",
- " dict(\n",
- " type='SampleFrames',\n",
- " clip_len=1,\n",
- " frame_interval=1,\n",
- " num_clips=25,\n",
- " test_mode=True),\n",
- " dict(type='DecordDecode'),\n",
- " dict(type='Resize', scale=(-1, 256)),\n",
- " dict(type='TenCrop', crop_size=224),\n",
- " dict(type='FormatShape', input_format='NCHW'),\n",
- " dict(type='PackActionInputs')\n",
- "]\n",
- "train_dataloader = dict(\n",
- " batch_size=2,\n",
- " num_workers=2,\n",
- " persistent_workers=True,\n",
- " sampler=dict(type='DefaultSampler', shuffle=True),\n",
- " dataset=dict(\n",
- " type='VideoDataset',\n",
- " ann_file='kinetics400_tiny/kinetics_tiny_train_video.txt',\n",
- " data_prefix=dict(video='kinetics400_tiny/train/'),\n",
- " pipeline=[\n",
- " dict(type='DecordInit', io_backend='disk'),\n",
- " dict(\n",
- " type='SampleFrames', clip_len=1, frame_interval=1,\n",
- " num_clips=3),\n",
- " dict(type='DecordDecode'),\n",
- " dict(type='Resize', scale=(-1, 256)),\n",
- " dict(\n",
- " type='MultiScaleCrop',\n",
- " input_size=224,\n",
- " scales=(1, 0.875, 0.75, 0.66),\n",
- " random_crop=False,\n",
- " max_wh_scale_gap=1),\n",
- " dict(type='Resize', scale=(224, 224), keep_ratio=False),\n",
- " dict(type='Flip', flip_ratio=0.5),\n",
- " dict(type='FormatShape', input_format='NCHW'),\n",
- " dict(type='PackActionInputs')\n",
- " ]))\n",
- "val_dataloader = dict(\n",
- " batch_size=2,\n",
- " num_workers=2,\n",
- " persistent_workers=True,\n",
- " sampler=dict(type='DefaultSampler', shuffle=False),\n",
- " dataset=dict(\n",
- " type='VideoDataset',\n",
- " ann_file='kinetics400_tiny/kinetics_tiny_val_video.txt',\n",
- " data_prefix=dict(video='kinetics400_tiny/val/'),\n",
- " pipeline=[\n",
- " dict(type='DecordInit', io_backend='disk'),\n",
- " dict(\n",
- " type='SampleFrames',\n",
- " clip_len=1,\n",
- " frame_interval=1,\n",
- " num_clips=3,\n",
- " test_mode=True),\n",
- " dict(type='DecordDecode'),\n",
- " dict(type='Resize', scale=(-1, 256)),\n",
- " dict(type='CenterCrop', crop_size=224),\n",
- " dict(type='FormatShape', input_format='NCHW'),\n",
- " dict(type='PackActionInputs')\n",
- " ],\n",
- " test_mode=True))\n",
- "test_dataloader = dict(\n",
- " batch_size=1,\n",
- " num_workers=2,\n",
- " persistent_workers=True,\n",
- " sampler=dict(type='DefaultSampler', shuffle=False),\n",
- " dataset=dict(\n",
- " type='VideoDataset',\n",
- " ann_file='kinetics400_tiny/kinetics_tiny_val_video.txt',\n",
- " data_prefix=dict(video='kinetics400_tiny/val/'),\n",
- " pipeline=[\n",
- " dict(type='DecordInit', io_backend='disk'),\n",
- " dict(\n",
- " type='SampleFrames',\n",
- " clip_len=1,\n",
- " frame_interval=1,\n",
- " num_clips=25,\n",
- " test_mode=True),\n",
- " dict(type='DecordDecode'),\n",
- " dict(type='Resize', scale=(-1, 256)),\n",
- " dict(type='TenCrop', crop_size=224),\n",
- " dict(type='FormatShape', input_format='NCHW'),\n",
- " dict(type='PackActionInputs')\n",
- " ],\n",
- " test_mode=True))\n",
- "val_evaluator = dict(type='AccMetric')\n",
- "test_evaluator = dict(type='AccMetric')\n",
- "auto_scale_lr = dict(enable=False, base_batch_size=256)\n",
- "work_dir = './tutorial_exps'\n",
- "\n",
- "05/15 03:33:35 - mmengine - INFO - Distributed training is not used, all SyncBatchNorm (SyncBN) layers in the model will be automatically reverted to BatchNormXd layers if they are used.\n",
- "05/15 03:33:35 - mmengine - INFO - Hooks will be executed in the following order:\n",
- "before_run:\n",
- "(VERY_HIGH ) RuntimeInfoHook \n",
- "(BELOW_NORMAL) LoggerHook \n",
- " -------------------- \n",
- "before_train:\n",
- "(VERY_HIGH ) RuntimeInfoHook \n",
- "(NORMAL ) IterTimerHook \n",
- "(VERY_LOW ) CheckpointHook \n",
- " -------------------- \n",
- "before_train_epoch:\n",
- "(VERY_HIGH ) RuntimeInfoHook \n",
- "(NORMAL ) IterTimerHook \n",
- "(NORMAL ) DistSamplerSeedHook \n",
- " -------------------- \n",
- "before_train_iter:\n",
- "(VERY_HIGH ) RuntimeInfoHook \n",
- "(NORMAL ) IterTimerHook \n",
- " -------------------- \n",
- "after_train_iter:\n",
- "(VERY_HIGH ) RuntimeInfoHook \n",
- "(NORMAL ) IterTimerHook \n",
- "(BELOW_NORMAL) LoggerHook \n",
- "(LOW ) ParamSchedulerHook \n",
- "(VERY_LOW ) CheckpointHook \n",
- " -------------------- \n",
- "after_train_epoch:\n",
- "(NORMAL ) IterTimerHook \n",
- "(NORMAL ) SyncBuffersHook \n",
- "(LOW ) ParamSchedulerHook \n",
- "(VERY_LOW ) CheckpointHook \n",
- " -------------------- \n",
- "before_val_epoch:\n",
- "(NORMAL ) IterTimerHook \n",
- "(NORMAL ) SyncBuffersHook \n",
- " -------------------- \n",
- "before_val_iter:\n",
- "(NORMAL ) IterTimerHook \n",
- " -------------------- \n",
- "after_val_iter:\n",
- "(NORMAL ) IterTimerHook \n",
- "(BELOW_NORMAL) LoggerHook \n",
- " -------------------- \n",
- "after_val_epoch:\n",
- "(VERY_HIGH ) RuntimeInfoHook \n",
- "(NORMAL ) IterTimerHook \n",
- "(BELOW_NORMAL) LoggerHook \n",
- "(LOW ) ParamSchedulerHook \n",
- "(VERY_LOW ) CheckpointHook \n",
- " -------------------- \n",
- "after_train:\n",
- "(VERY_LOW ) CheckpointHook \n",
- " -------------------- \n",
- "before_test_epoch:\n",
- "(NORMAL ) IterTimerHook \n",
- " -------------------- \n",
- "before_test_iter:\n",
- "(NORMAL ) IterTimerHook \n",
- " -------------------- \n",
- "after_test_iter:\n",
- "(NORMAL ) IterTimerHook \n",
- "(BELOW_NORMAL) LoggerHook \n",
- " -------------------- \n",
- "after_test_epoch:\n",
- "(VERY_HIGH ) RuntimeInfoHook \n",
- "(NORMAL ) IterTimerHook \n",
- "(BELOW_NORMAL) LoggerHook \n",
- " -------------------- \n",
- "after_run:\n",
- "(BELOW_NORMAL) LoggerHook \n",
- " -------------------- \n",
- "Loads checkpoint by http backend from path: https://download.pytorch.org/models/resnet50-11ad3fa6.pth\n"
- ]
- },
- {
- "output_type": "stream",
- "name": "stderr",
- "text": [
- "Downloading: \"https://download.pytorch.org/models/resnet50-11ad3fa6.pth\" to /root/.cache/torch/hub/checkpoints/resnet50-11ad3fa6.pth\n"
- ]
- },
- {
- "output_type": "stream",
- "name": "stdout",
- "text": [
- "05/15 03:33:37 - mmengine - INFO - These parameters in pretrained checkpoint are not loaded: {'fc.weight', 'fc.bias'}\n",
- "Loads checkpoint by local backend from path: ./checkpoints/tsn_r50_1x1x3_100e_kinetics400_rgb_20200614-e508be42.pth\n",
- "The model and loaded state dict do not match exactly\n",
- "\n",
- "size mismatch for cls_head.fc_cls.weight: copying a param with shape torch.Size([400, 2048]) from checkpoint, the shape in current model is torch.Size([2, 2048]).\n",
- "size mismatch for cls_head.fc_cls.bias: copying a param with shape torch.Size([400]) from checkpoint, the shape in current model is torch.Size([2]).\n",
- "05/15 03:33:37 - mmengine - INFO - Load checkpoint from ./checkpoints/tsn_r50_1x1x3_100e_kinetics400_rgb_20200614-e508be42.pth\n",
- "05/15 03:33:37 - mmengine - WARNING - \"FileClient\" will be deprecated in future. Please use io functions in https://mmengine.readthedocs.io/en/latest/api/fileio.html#file-io\n",
- "05/15 03:33:37 - mmengine - INFO - Checkpoints will be saved to /content/mmaction2/tutorial_exps.\n",
- "05/15 03:33:41 - mmengine - INFO - Exp name: tsn_imagenet-pretrained-r50_8xb32-1x1x3-100e_kinetics400-rgb_20230515_033334\n",
- "05/15 03:33:41 - mmengine - INFO - Epoch(train) [1][15/15] lr: 7.8125e-05 eta: 0:00:31 time: 0.2334 data_time: 0.0793 memory: 2917 grad_norm: 11.9900 loss: 0.6971 top1_acc: 1.0000 top5_acc: 1.0000 loss_cls: 0.6971\n",
- "05/15 03:33:42 - mmengine - INFO - Epoch(val) [1][5/5] acc/top1: 0.3000 acc/top5: 1.0000 acc/mean1: 0.3000 data_time: 0.1994 time: 0.2254\n",
- "05/15 03:33:42 - mmengine - INFO - The best checkpoint with 0.3000 acc/top1 at 1 epoch is saved to best_acc_top1_epoch_1.pth.\n",
- "05/15 03:33:46 - mmengine - INFO - Exp name: tsn_imagenet-pretrained-r50_8xb32-1x1x3-100e_kinetics400-rgb_20230515_033334\n",
- "05/15 03:33:46 - mmengine - INFO - Epoch(train) [2][15/15] lr: 7.8125e-05 eta: 0:00:29 time: 0.2373 data_time: 0.1369 memory: 961 grad_norm: 12.4935 loss: 0.7158 top1_acc: 0.5000 top5_acc: 1.0000 loss_cls: 0.7158\n",
- "05/15 03:33:48 - mmengine - INFO - Epoch(val) [2][5/5] acc/top1: 0.7000 acc/top5: 1.0000 acc/mean1: 0.7000 data_time: 0.2692 time: 0.3006\n",
- "05/15 03:33:48 - mmengine - INFO - The previous best checkpoint /content/mmaction2/tutorial_exps/best_acc_top1_epoch_1.pth is removed\n",
- "05/15 03:33:48 - mmengine - INFO - The best checkpoint with 0.7000 acc/top1 at 2 epoch is saved to best_acc_top1_epoch_2.pth.\n",
- "05/15 03:33:51 - mmengine - INFO - Exp name: tsn_imagenet-pretrained-r50_8xb32-1x1x3-100e_kinetics400-rgb_20230515_033334\n",
- "05/15 03:33:51 - mmengine - INFO - Epoch(train) [3][15/15] lr: 7.8125e-05 eta: 0:00:24 time: 0.2112 data_time: 0.1163 memory: 961 grad_norm: 13.4063 loss: 0.7338 top1_acc: 0.0000 top5_acc: 1.0000 loss_cls: 0.7338\n",
- "05/15 03:33:51 - mmengine - INFO - Saving checkpoint at 3 epochs\n",
- "05/15 03:33:53 - mmengine - INFO - Epoch(val) [3][5/5] acc/top1: 0.4000 acc/top5: 1.0000 acc/mean1: 0.4000 data_time: 0.1669 time: 0.1906\n",
- "05/15 03:33:56 - mmengine - INFO - Exp name: tsn_imagenet-pretrained-r50_8xb32-1x1x3-100e_kinetics400-rgb_20230515_033334\n",
- "05/15 03:33:56 - mmengine - INFO - Epoch(train) [4][15/15] lr: 7.8125e-05 eta: 0:00:19 time: 0.1750 data_time: 0.0907 memory: 961 grad_norm: 12.4322 loss: 0.6894 top1_acc: 0.0000 top5_acc: 1.0000 loss_cls: 0.6894\n",
- "05/15 03:33:57 - mmengine - INFO - Epoch(val) [4][5/5] acc/top1: 0.7000 acc/top5: 1.0000 acc/mean1: 0.7000 data_time: 0.1791 time: 0.2030\n",
- "05/15 03:34:00 - mmengine - INFO - Exp name: tsn_imagenet-pretrained-r50_8xb32-1x1x3-100e_kinetics400-rgb_20230515_033334\n",
- "05/15 03:34:00 - mmengine - INFO - Epoch(train) [5][15/15] lr: 7.8125e-05 eta: 0:00:16 time: 0.2016 data_time: 0.1155 memory: 961 grad_norm: 11.5982 loss: 0.6940 top1_acc: 0.0000 top5_acc: 1.0000 loss_cls: 0.6940\n",
- "05/15 03:34:02 - mmengine - INFO - Epoch(val) [5][5/5] acc/top1: 0.7000 acc/top5: 1.0000 acc/mean1: 0.7000 data_time: 0.3145 time: 0.3455\n",
- "05/15 03:34:05 - mmengine - INFO - Exp name: tsn_imagenet-pretrained-r50_8xb32-1x1x3-100e_kinetics400-rgb_20230515_033334\n",
- "05/15 03:34:05 - mmengine - INFO - Epoch(train) [6][15/15] lr: 7.8125e-05 eta: 0:00:13 time: 0.2366 data_time: 0.1440 memory: 961 grad_norm: 12.0952 loss: 0.6667 top1_acc: 0.0000 top5_acc: 1.0000 loss_cls: 0.6667\n",
- "05/15 03:34:05 - mmengine - INFO - Saving checkpoint at 6 epochs\n",
- "05/15 03:34:08 - mmengine - INFO - Epoch(val) [6][5/5] acc/top1: 0.6000 acc/top5: 1.0000 acc/mean1: 0.6000 data_time: 0.2172 time: 0.2403\n",
- "05/15 03:34:10 - mmengine - INFO - Exp name: tsn_imagenet-pretrained-r50_8xb32-1x1x3-100e_kinetics400-rgb_20230515_033334\n",
- "05/15 03:34:10 - mmengine - INFO - Epoch(train) [7][15/15] lr: 7.8125e-05 eta: 0:00:09 time: 0.1784 data_time: 0.0942 memory: 961 grad_norm: 12.4209 loss: 0.6570 top1_acc: 1.0000 top5_acc: 1.0000 loss_cls: 0.6570\n",
- "05/15 03:34:11 - mmengine - INFO - Epoch(val) [7][5/5] acc/top1: 0.9000 acc/top5: 1.0000 acc/mean1: 0.9000 data_time: 0.1898 time: 0.2118\n",
- "05/15 03:34:11 - mmengine - INFO - The previous best checkpoint /content/mmaction2/tutorial_exps/best_acc_top1_epoch_2.pth is removed\n",
- "05/15 03:34:12 - mmengine - INFO - The best checkpoint with 0.9000 acc/top1 at 7 epoch is saved to best_acc_top1_epoch_7.pth.\n",
- "05/15 03:34:15 - mmengine - INFO - Exp name: tsn_imagenet-pretrained-r50_8xb32-1x1x3-100e_kinetics400-rgb_20230515_033334\n",
- "05/15 03:34:15 - mmengine - INFO - Epoch(train) [8][15/15] lr: 7.8125e-05 eta: 0:00:06 time: 0.2073 data_time: 0.1220 memory: 961 grad_norm: 11.4271 loss: 0.6241 top1_acc: 1.0000 top5_acc: 1.0000 loss_cls: 0.6241\n",
- "05/15 03:34:17 - mmengine - INFO - Epoch(val) [8][5/5] acc/top1: 1.0000 acc/top5: 1.0000 acc/mean1: 1.0000 data_time: 0.3497 time: 0.3890\n",
- "05/15 03:34:17 - mmengine - INFO - The previous best checkpoint /content/mmaction2/tutorial_exps/best_acc_top1_epoch_7.pth is removed\n",
- "05/15 03:34:18 - mmengine - INFO - The best checkpoint with 1.0000 acc/top1 at 8 epoch is saved to best_acc_top1_epoch_8.pth.\n",
- "05/15 03:34:21 - mmengine - INFO - Exp name: tsn_imagenet-pretrained-r50_8xb32-1x1x3-100e_kinetics400-rgb_20230515_033334\n",
- "05/15 03:34:21 - mmengine - INFO - Epoch(train) [9][15/15] lr: 7.8125e-05 eta: 0:00:03 time: 0.2309 data_time: 0.1390 memory: 961 grad_norm: 12.3066 loss: 0.6451 top1_acc: 0.5000 top5_acc: 1.0000 loss_cls: 0.6451\n",
- "05/15 03:34:21 - mmengine - INFO - Saving checkpoint at 9 epochs\n",
- "05/15 03:34:23 - mmengine - INFO - Epoch(val) [9][5/5] acc/top1: 1.0000 acc/top5: 1.0000 acc/mean1: 1.0000 data_time: 0.2023 time: 0.2256\n",
- "05/15 03:34:26 - mmengine - INFO - Exp name: tsn_imagenet-pretrained-r50_8xb32-1x1x3-100e_kinetics400-rgb_20230515_033334\n",
- "05/15 03:34:26 - mmengine - INFO - Epoch(train) [10][15/15] lr: 7.8125e-05 eta: 0:00:00 time: 0.1733 data_time: 0.0951 memory: 961 grad_norm: 11.1461 loss: 0.5931 top1_acc: 1.0000 top5_acc: 1.0000 loss_cls: 0.5931\n",
- "05/15 03:34:26 - mmengine - INFO - Saving checkpoint at 10 epochs\n",
- "05/15 03:34:27 - mmengine - INFO - Epoch(val) [10][5/5] acc/top1: 1.0000 acc/top5: 1.0000 acc/mean1: 1.0000 data_time: 0.1836 time: 0.2048\n"
- ]
- },
- {
- "output_type": "execute_result",
- "data": {
- "text/plain": [
- "Recognizer2D(\n",
- " (data_preprocessor): ActionDataPreprocessor()\n",
- " (backbone): ResNet(\n",
- " (conv1): ConvModule(\n",
- " (conv): Conv2d(3, 64, kernel_size=(7, 7), stride=(2, 2), padding=(3, 3), bias=False)\n",
- " (bn): BatchNorm2d(64, eps=1e-05, momentum=0.1, affine=True, track_running_stats=True)\n",
- " (activate): ReLU(inplace=True)\n",
- " )\n",
- " (maxpool): MaxPool2d(kernel_size=3, stride=2, padding=1, dilation=1, ceil_mode=False)\n",
- " (layer1): Sequential(\n",
- " (0): Bottleneck(\n",
- " (conv1): ConvModule(\n",
- " (conv): Conv2d(64, 64, kernel_size=(1, 1), stride=(1, 1), bias=False)\n",
- " (bn): BatchNorm2d(64, eps=1e-05, momentum=0.1, affine=True, track_running_stats=True)\n",
- " (activate): ReLU(inplace=True)\n",
- " )\n",
- " (conv2): ConvModule(\n",
- " (conv): Conv2d(64, 64, kernel_size=(3, 3), stride=(1, 1), padding=(1, 1), bias=False)\n",
- " (bn): BatchNorm2d(64, eps=1e-05, momentum=0.1, affine=True, track_running_stats=True)\n",
- " (activate): ReLU(inplace=True)\n",
- " )\n",
- " (conv3): ConvModule(\n",
- " (conv): Conv2d(64, 256, kernel_size=(1, 1), stride=(1, 1), bias=False)\n",
- " (bn): BatchNorm2d(256, eps=1e-05, momentum=0.1, affine=True, track_running_stats=True)\n",
- " )\n",
- " (relu): ReLU(inplace=True)\n",
- " (downsample): ConvModule(\n",
- " (conv): Conv2d(64, 256, kernel_size=(1, 1), stride=(1, 1), bias=False)\n",
- " (bn): BatchNorm2d(256, eps=1e-05, momentum=0.1, affine=True, track_running_stats=True)\n",
- " )\n",
- " )\n",
- " (1): Bottleneck(\n",
- " (conv1): ConvModule(\n",
- " (conv): Conv2d(256, 64, kernel_size=(1, 1), stride=(1, 1), bias=False)\n",
- " (bn): BatchNorm2d(64, eps=1e-05, momentum=0.1, affine=True, track_running_stats=True)\n",
- " (activate): ReLU(inplace=True)\n",
- " )\n",
- " (conv2): ConvModule(\n",
- " (conv): Conv2d(64, 64, kernel_size=(3, 3), stride=(1, 1), padding=(1, 1), bias=False)\n",
- " (bn): BatchNorm2d(64, eps=1e-05, momentum=0.1, affine=True, track_running_stats=True)\n",
- " (activate): ReLU(inplace=True)\n",
- " )\n",
- " (conv3): ConvModule(\n",
- " (conv): Conv2d(64, 256, kernel_size=(1, 1), stride=(1, 1), bias=False)\n",
- " (bn): BatchNorm2d(256, eps=1e-05, momentum=0.1, affine=True, track_running_stats=True)\n",
- " )\n",
- " (relu): ReLU(inplace=True)\n",
- " )\n",
- " (2): Bottleneck(\n",
- " (conv1): ConvModule(\n",
- " (conv): Conv2d(256, 64, kernel_size=(1, 1), stride=(1, 1), bias=False)\n",
- " (bn): BatchNorm2d(64, eps=1e-05, momentum=0.1, affine=True, track_running_stats=True)\n",
- " (activate): ReLU(inplace=True)\n",
- " )\n",
- " (conv2): ConvModule(\n",
- " (conv): Conv2d(64, 64, kernel_size=(3, 3), stride=(1, 1), padding=(1, 1), bias=False)\n",
- " (bn): BatchNorm2d(64, eps=1e-05, momentum=0.1, affine=True, track_running_stats=True)\n",
- " (activate): ReLU(inplace=True)\n",
- " )\n",
- " (conv3): ConvModule(\n",
- " (conv): Conv2d(64, 256, kernel_size=(1, 1), stride=(1, 1), bias=False)\n",
- " (bn): BatchNorm2d(256, eps=1e-05, momentum=0.1, affine=True, track_running_stats=True)\n",
- " )\n",
- " (relu): ReLU(inplace=True)\n",
- " )\n",
- " )\n",
- " (layer2): Sequential(\n",
- " (0): Bottleneck(\n",
- " (conv1): ConvModule(\n",
- " (conv): Conv2d(256, 128, kernel_size=(1, 1), stride=(1, 1), bias=False)\n",
- " (bn): BatchNorm2d(128, eps=1e-05, momentum=0.1, affine=True, track_running_stats=True)\n",
- " (activate): ReLU(inplace=True)\n",
- " )\n",
- " (conv2): ConvModule(\n",
- " (conv): Conv2d(128, 128, kernel_size=(3, 3), stride=(2, 2), padding=(1, 1), bias=False)\n",
- " (bn): BatchNorm2d(128, eps=1e-05, momentum=0.1, affine=True, track_running_stats=True)\n",
- " (activate): ReLU(inplace=True)\n",
- " )\n",
- " (conv3): ConvModule(\n",
- " (conv): Conv2d(128, 512, kernel_size=(1, 1), stride=(1, 1), bias=False)\n",
- " (bn): BatchNorm2d(512, eps=1e-05, momentum=0.1, affine=True, track_running_stats=True)\n",
- " )\n",
- " (relu): ReLU(inplace=True)\n",
- " (downsample): ConvModule(\n",
- " (conv): Conv2d(256, 512, kernel_size=(1, 1), stride=(2, 2), bias=False)\n",
- " (bn): BatchNorm2d(512, eps=1e-05, momentum=0.1, affine=True, track_running_stats=True)\n",
- " )\n",
- " )\n",
- " (1): Bottleneck(\n",
- " (conv1): ConvModule(\n",
- " (conv): Conv2d(512, 128, kernel_size=(1, 1), stride=(1, 1), bias=False)\n",
- " (bn): BatchNorm2d(128, eps=1e-05, momentum=0.1, affine=True, track_running_stats=True)\n",
- " (activate): ReLU(inplace=True)\n",
- " )\n",
- " (conv2): ConvModule(\n",
- " (conv): Conv2d(128, 128, kernel_size=(3, 3), stride=(1, 1), padding=(1, 1), bias=False)\n",
- " (bn): BatchNorm2d(128, eps=1e-05, momentum=0.1, affine=True, track_running_stats=True)\n",
- " (activate): ReLU(inplace=True)\n",
- " )\n",
- " (conv3): ConvModule(\n",
- " (conv): Conv2d(128, 512, kernel_size=(1, 1), stride=(1, 1), bias=False)\n",
- " (bn): BatchNorm2d(512, eps=1e-05, momentum=0.1, affine=True, track_running_stats=True)\n",
- " )\n",
- " (relu): ReLU(inplace=True)\n",
- " )\n",
- " (2): Bottleneck(\n",
- " (conv1): ConvModule(\n",
- " (conv): Conv2d(512, 128, kernel_size=(1, 1), stride=(1, 1), bias=False)\n",
- " (bn): BatchNorm2d(128, eps=1e-05, momentum=0.1, affine=True, track_running_stats=True)\n",
- " (activate): ReLU(inplace=True)\n",
- " )\n",
- " (conv2): ConvModule(\n",
- " (conv): Conv2d(128, 128, kernel_size=(3, 3), stride=(1, 1), padding=(1, 1), bias=False)\n",
- " (bn): BatchNorm2d(128, eps=1e-05, momentum=0.1, affine=True, track_running_stats=True)\n",
- " (activate): ReLU(inplace=True)\n",
- " )\n",
- " (conv3): ConvModule(\n",
- " (conv): Conv2d(128, 512, kernel_size=(1, 1), stride=(1, 1), bias=False)\n",
- " (bn): BatchNorm2d(512, eps=1e-05, momentum=0.1, affine=True, track_running_stats=True)\n",
- " )\n",
- " (relu): ReLU(inplace=True)\n",
- " )\n",
- " (3): Bottleneck(\n",
- " (conv1): ConvModule(\n",
- " (conv): Conv2d(512, 128, kernel_size=(1, 1), stride=(1, 1), bias=False)\n",
- " (bn): BatchNorm2d(128, eps=1e-05, momentum=0.1, affine=True, track_running_stats=True)\n",
- " (activate): ReLU(inplace=True)\n",
- " )\n",
- " (conv2): ConvModule(\n",
- " (conv): Conv2d(128, 128, kernel_size=(3, 3), stride=(1, 1), padding=(1, 1), bias=False)\n",
- " (bn): BatchNorm2d(128, eps=1e-05, momentum=0.1, affine=True, track_running_stats=True)\n",
- " (activate): ReLU(inplace=True)\n",
- " )\n",
- " (conv3): ConvModule(\n",
- " (conv): Conv2d(128, 512, kernel_size=(1, 1), stride=(1, 1), bias=False)\n",
- " (bn): BatchNorm2d(512, eps=1e-05, momentum=0.1, affine=True, track_running_stats=True)\n",
- " )\n",
- " (relu): ReLU(inplace=True)\n",
- " )\n",
- " )\n",
- " (layer3): Sequential(\n",
- " (0): Bottleneck(\n",
- " (conv1): ConvModule(\n",
- " (conv): Conv2d(512, 256, kernel_size=(1, 1), stride=(1, 1), bias=False)\n",
- " (bn): BatchNorm2d(256, eps=1e-05, momentum=0.1, affine=True, track_running_stats=True)\n",
- " (activate): ReLU(inplace=True)\n",
- " )\n",
- " (conv2): ConvModule(\n",
- " (conv): Conv2d(256, 256, kernel_size=(3, 3), stride=(2, 2), padding=(1, 1), bias=False)\n",
- " (bn): BatchNorm2d(256, eps=1e-05, momentum=0.1, affine=True, track_running_stats=True)\n",
- " (activate): ReLU(inplace=True)\n",
- " )\n",
- " (conv3): ConvModule(\n",
- " (conv): Conv2d(256, 1024, kernel_size=(1, 1), stride=(1, 1), bias=False)\n",
- " (bn): BatchNorm2d(1024, eps=1e-05, momentum=0.1, affine=True, track_running_stats=True)\n",
- " )\n",
- " (relu): ReLU(inplace=True)\n",
- " (downsample): ConvModule(\n",
- " (conv): Conv2d(512, 1024, kernel_size=(1, 1), stride=(2, 2), bias=False)\n",
- " (bn): BatchNorm2d(1024, eps=1e-05, momentum=0.1, affine=True, track_running_stats=True)\n",
- " )\n",
- " )\n",
- " (1): Bottleneck(\n",
- " (conv1): ConvModule(\n",
- " (conv): Conv2d(1024, 256, kernel_size=(1, 1), stride=(1, 1), bias=False)\n",
- " (bn): BatchNorm2d(256, eps=1e-05, momentum=0.1, affine=True, track_running_stats=True)\n",
- " (activate): ReLU(inplace=True)\n",
- " )\n",
- " (conv2): ConvModule(\n",
- " (conv): Conv2d(256, 256, kernel_size=(3, 3), stride=(1, 1), padding=(1, 1), bias=False)\n",
- " (bn): BatchNorm2d(256, eps=1e-05, momentum=0.1, affine=True, track_running_stats=True)\n",
- " (activate): ReLU(inplace=True)\n",
- " )\n",
- " (conv3): ConvModule(\n",
- " (conv): Conv2d(256, 1024, kernel_size=(1, 1), stride=(1, 1), bias=False)\n",
- " (bn): BatchNorm2d(1024, eps=1e-05, momentum=0.1, affine=True, track_running_stats=True)\n",
- " )\n",
- " (relu): ReLU(inplace=True)\n",
- " )\n",
- " (2): Bottleneck(\n",
- " (conv1): ConvModule(\n",
- " (conv): Conv2d(1024, 256, kernel_size=(1, 1), stride=(1, 1), bias=False)\n",
- " (bn): BatchNorm2d(256, eps=1e-05, momentum=0.1, affine=True, track_running_stats=True)\n",
- " (activate): ReLU(inplace=True)\n",
- " )\n",
- " (conv2): ConvModule(\n",
- " (conv): Conv2d(256, 256, kernel_size=(3, 3), stride=(1, 1), padding=(1, 1), bias=False)\n",
- " (bn): BatchNorm2d(256, eps=1e-05, momentum=0.1, affine=True, track_running_stats=True)\n",
- " (activate): ReLU(inplace=True)\n",
- " )\n",
- " (conv3): ConvModule(\n",
- " (conv): Conv2d(256, 1024, kernel_size=(1, 1), stride=(1, 1), bias=False)\n",
- " (bn): BatchNorm2d(1024, eps=1e-05, momentum=0.1, affine=True, track_running_stats=True)\n",
- " )\n",
- " (relu): ReLU(inplace=True)\n",
- " )\n",
- " (3): Bottleneck(\n",
- " (conv1): ConvModule(\n",
- " (conv): Conv2d(1024, 256, kernel_size=(1, 1), stride=(1, 1), bias=False)\n",
- " (bn): BatchNorm2d(256, eps=1e-05, momentum=0.1, affine=True, track_running_stats=True)\n",
- " (activate): ReLU(inplace=True)\n",
- " )\n",
- " (conv2): ConvModule(\n",
- " (conv): Conv2d(256, 256, kernel_size=(3, 3), stride=(1, 1), padding=(1, 1), bias=False)\n",
- " (bn): BatchNorm2d(256, eps=1e-05, momentum=0.1, affine=True, track_running_stats=True)\n",
- " (activate): ReLU(inplace=True)\n",
- " )\n",
- " (conv3): ConvModule(\n",
- " (conv): Conv2d(256, 1024, kernel_size=(1, 1), stride=(1, 1), bias=False)\n",
- " (bn): BatchNorm2d(1024, eps=1e-05, momentum=0.1, affine=True, track_running_stats=True)\n",
- " )\n",
- " (relu): ReLU(inplace=True)\n",
- " )\n",
- " (4): Bottleneck(\n",
- " (conv1): ConvModule(\n",
- " (conv): Conv2d(1024, 256, kernel_size=(1, 1), stride=(1, 1), bias=False)\n",
- " (bn): BatchNorm2d(256, eps=1e-05, momentum=0.1, affine=True, track_running_stats=True)\n",
- " (activate): ReLU(inplace=True)\n",
- " )\n",
- " (conv2): ConvModule(\n",
- " (conv): Conv2d(256, 256, kernel_size=(3, 3), stride=(1, 1), padding=(1, 1), bias=False)\n",
- " (bn): BatchNorm2d(256, eps=1e-05, momentum=0.1, affine=True, track_running_stats=True)\n",
- " (activate): ReLU(inplace=True)\n",
- " )\n",
- " (conv3): ConvModule(\n",
- " (conv): Conv2d(256, 1024, kernel_size=(1, 1), stride=(1, 1), bias=False)\n",
- " (bn): BatchNorm2d(1024, eps=1e-05, momentum=0.1, affine=True, track_running_stats=True)\n",
- " )\n",
- " (relu): ReLU(inplace=True)\n",
- " )\n",
- " (5): Bottleneck(\n",
- " (conv1): ConvModule(\n",
- " (conv): Conv2d(1024, 256, kernel_size=(1, 1), stride=(1, 1), bias=False)\n",
- " (bn): BatchNorm2d(256, eps=1e-05, momentum=0.1, affine=True, track_running_stats=True)\n",
- " (activate): ReLU(inplace=True)\n",
- " )\n",
- " (conv2): ConvModule(\n",
- " (conv): Conv2d(256, 256, kernel_size=(3, 3), stride=(1, 1), padding=(1, 1), bias=False)\n",
- " (bn): BatchNorm2d(256, eps=1e-05, momentum=0.1, affine=True, track_running_stats=True)\n",
- " (activate): ReLU(inplace=True)\n",
- " )\n",
- " (conv3): ConvModule(\n",
- " (conv): Conv2d(256, 1024, kernel_size=(1, 1), stride=(1, 1), bias=False)\n",
- " (bn): BatchNorm2d(1024, eps=1e-05, momentum=0.1, affine=True, track_running_stats=True)\n",
- " )\n",
- " (relu): ReLU(inplace=True)\n",
- " )\n",
- " )\n",
- " (layer4): Sequential(\n",
- " (0): Bottleneck(\n",
- " (conv1): ConvModule(\n",
- " (conv): Conv2d(1024, 512, kernel_size=(1, 1), stride=(1, 1), bias=False)\n",
- " (bn): BatchNorm2d(512, eps=1e-05, momentum=0.1, affine=True, track_running_stats=True)\n",
- " (activate): ReLU(inplace=True)\n",
- " )\n",
- " (conv2): ConvModule(\n",
- " (conv): Conv2d(512, 512, kernel_size=(3, 3), stride=(2, 2), padding=(1, 1), bias=False)\n",
- " (bn): BatchNorm2d(512, eps=1e-05, momentum=0.1, affine=True, track_running_stats=True)\n",
- " (activate): ReLU(inplace=True)\n",
- " )\n",
- " (conv3): ConvModule(\n",
- " (conv): Conv2d(512, 2048, kernel_size=(1, 1), stride=(1, 1), bias=False)\n",
- " (bn): BatchNorm2d(2048, eps=1e-05, momentum=0.1, affine=True, track_running_stats=True)\n",
- " )\n",
- " (relu): ReLU(inplace=True)\n",
- " (downsample): ConvModule(\n",
- " (conv): Conv2d(1024, 2048, kernel_size=(1, 1), stride=(2, 2), bias=False)\n",
- " (bn): BatchNorm2d(2048, eps=1e-05, momentum=0.1, affine=True, track_running_stats=True)\n",
- " )\n",
- " )\n",
- " (1): Bottleneck(\n",
- " (conv1): ConvModule(\n",
- " (conv): Conv2d(2048, 512, kernel_size=(1, 1), stride=(1, 1), bias=False)\n",
- " (bn): BatchNorm2d(512, eps=1e-05, momentum=0.1, affine=True, track_running_stats=True)\n",
- " (activate): ReLU(inplace=True)\n",
- " )\n",
- " (conv2): ConvModule(\n",
- " (conv): Conv2d(512, 512, kernel_size=(3, 3), stride=(1, 1), padding=(1, 1), bias=False)\n",
- " (bn): BatchNorm2d(512, eps=1e-05, momentum=0.1, affine=True, track_running_stats=True)\n",
- " (activate): ReLU(inplace=True)\n",
- " )\n",
- " (conv3): ConvModule(\n",
- " (conv): Conv2d(512, 2048, kernel_size=(1, 1), stride=(1, 1), bias=False)\n",
- " (bn): BatchNorm2d(2048, eps=1e-05, momentum=0.1, affine=True, track_running_stats=True)\n",
- " )\n",
- " (relu): ReLU(inplace=True)\n",
- " )\n",
- " (2): Bottleneck(\n",
- " (conv1): ConvModule(\n",
- " (conv): Conv2d(2048, 512, kernel_size=(1, 1), stride=(1, 1), bias=False)\n",
- " (bn): BatchNorm2d(512, eps=1e-05, momentum=0.1, affine=True, track_running_stats=True)\n",
- " (activate): ReLU(inplace=True)\n",
- " )\n",
- " (conv2): ConvModule(\n",
- " (conv): Conv2d(512, 512, kernel_size=(3, 3), stride=(1, 1), padding=(1, 1), bias=False)\n",
- " (bn): BatchNorm2d(512, eps=1e-05, momentum=0.1, affine=True, track_running_stats=True)\n",
- " (activate): ReLU(inplace=True)\n",
- " )\n",
- " (conv3): ConvModule(\n",
- " (conv): Conv2d(512, 2048, kernel_size=(1, 1), stride=(1, 1), bias=False)\n",
- " (bn): BatchNorm2d(2048, eps=1e-05, momentum=0.1, affine=True, track_running_stats=True)\n",
- " )\n",
- " (relu): ReLU(inplace=True)\n",
- " )\n",
- " )\n",
- " )\n",
- " (cls_head): TSNHead(\n",
- " (loss_cls): CrossEntropyLoss()\n",
- " (consensus): AvgConsensus()\n",
- " (avg_pool): AdaptiveAvgPool2d(output_size=(1, 1))\n",
- " (dropout): Dropout(p=0.4, inplace=False)\n",
- " (fc_cls): Linear(in_features=2048, out_features=2, bias=True)\n",
- " )\n",
- ")"
- ]
- },
- "metadata": {},
- "execution_count": 15
- }
- ],
- "source": [
- "import os.path as osp\n",
- "import mmengine\n",
- "from mmengine.runner import Runner\n",
- "\n",
- "# Create work_dir\n",
- "mmengine.mkdir_or_exist(osp.abspath(cfg.work_dir))\n",
- "\n",
- "# build the runner from config\n",
- "runner = Runner.from_cfg(cfg)\n",
- "\n",
- "# start training\n",
- "runner.train()"
- ]
+ "output_type": "stream",
+ "name": "stdout",
+ "text": [
+ "05/15 03:33:37 - mmengine - INFO - These parameters in pretrained checkpoint are not loaded: {'fc.weight', 'fc.bias'}\n",
+ "Loads checkpoint by local backend from path: ./checkpoints/tsn_r50_1x1x3_100e_kinetics400_rgb_20200614-e508be42.pth\n",
+ "The model and loaded state dict do not match exactly\n",
+ "\n",
+ "size mismatch for cls_head.fc_cls.weight: copying a param with shape torch.Size([400, 2048]) from checkpoint, the shape in current model is torch.Size([2, 2048]).\n",
+ "size mismatch for cls_head.fc_cls.bias: copying a param with shape torch.Size([400]) from checkpoint, the shape in current model is torch.Size([2]).\n",
+ "05/15 03:33:37 - mmengine - INFO - Load checkpoint from ./checkpoints/tsn_r50_1x1x3_100e_kinetics400_rgb_20200614-e508be42.pth\n",
+ "05/15 03:33:37 - mmengine - WARNING - \"FileClient\" will be deprecated in future. Please use io functions in https://mmengine.readthedocs.io/en/latest/api/fileio.html#file-io\n",
+ "05/15 03:33:37 - mmengine - INFO - Checkpoints will be saved to /content/mmaction2/tutorial_exps.\n",
+ "05/15 03:33:41 - mmengine - INFO - Exp name: tsn_imagenet-pretrained-r50_8xb32-1x1x3-100e_kinetics400-rgb_20230515_033334\n",
+ "05/15 03:33:41 - mmengine - INFO - Epoch(train) [1][15/15] lr: 7.8125e-05 eta: 0:00:31 time: 0.2334 data_time: 0.0793 memory: 2917 grad_norm: 11.9900 loss: 0.6971 top1_acc: 1.0000 top5_acc: 1.0000 loss_cls: 0.6971\n",
+ "05/15 03:33:42 - mmengine - INFO - Epoch(val) [1][5/5] acc/top1: 0.3000 acc/top5: 1.0000 acc/mean1: 0.3000 data_time: 0.1994 time: 0.2254\n",
+ "05/15 03:33:42 - mmengine - INFO - The best checkpoint with 0.3000 acc/top1 at 1 epoch is saved to best_acc_top1_epoch_1.pth.\n",
+ "05/15 03:33:46 - mmengine - INFO - Exp name: tsn_imagenet-pretrained-r50_8xb32-1x1x3-100e_kinetics400-rgb_20230515_033334\n",
+ "05/15 03:33:46 - mmengine - INFO - Epoch(train) [2][15/15] lr: 7.8125e-05 eta: 0:00:29 time: 0.2373 data_time: 0.1369 memory: 961 grad_norm: 12.4935 loss: 0.7158 top1_acc: 0.5000 top5_acc: 1.0000 loss_cls: 0.7158\n",
+ "05/15 03:33:48 - mmengine - INFO - Epoch(val) [2][5/5] acc/top1: 0.7000 acc/top5: 1.0000 acc/mean1: 0.7000 data_time: 0.2692 time: 0.3006\n",
+ "05/15 03:33:48 - mmengine - INFO - The previous best checkpoint /content/mmaction2/tutorial_exps/best_acc_top1_epoch_1.pth is removed\n",
+ "05/15 03:33:48 - mmengine - INFO - The best checkpoint with 0.7000 acc/top1 at 2 epoch is saved to best_acc_top1_epoch_2.pth.\n",
+ "05/15 03:33:51 - mmengine - INFO - Exp name: tsn_imagenet-pretrained-r50_8xb32-1x1x3-100e_kinetics400-rgb_20230515_033334\n",
+ "05/15 03:33:51 - mmengine - INFO - Epoch(train) [3][15/15] lr: 7.8125e-05 eta: 0:00:24 time: 0.2112 data_time: 0.1163 memory: 961 grad_norm: 13.4063 loss: 0.7338 top1_acc: 0.0000 top5_acc: 1.0000 loss_cls: 0.7338\n",
+ "05/15 03:33:51 - mmengine - INFO - Saving checkpoint at 3 epochs\n",
+ "05/15 03:33:53 - mmengine - INFO - Epoch(val) [3][5/5] acc/top1: 0.4000 acc/top5: 1.0000 acc/mean1: 0.4000 data_time: 0.1669 time: 0.1906\n",
+ "05/15 03:33:56 - mmengine - INFO - Exp name: tsn_imagenet-pretrained-r50_8xb32-1x1x3-100e_kinetics400-rgb_20230515_033334\n",
+ "05/15 03:33:56 - mmengine - INFO - Epoch(train) [4][15/15] lr: 7.8125e-05 eta: 0:00:19 time: 0.1750 data_time: 0.0907 memory: 961 grad_norm: 12.4322 loss: 0.6894 top1_acc: 0.0000 top5_acc: 1.0000 loss_cls: 0.6894\n",
+ "05/15 03:33:57 - mmengine - INFO - Epoch(val) [4][5/5] acc/top1: 0.7000 acc/top5: 1.0000 acc/mean1: 0.7000 data_time: 0.1791 time: 0.2030\n",
+ "05/15 03:34:00 - mmengine - INFO - Exp name: tsn_imagenet-pretrained-r50_8xb32-1x1x3-100e_kinetics400-rgb_20230515_033334\n",
+ "05/15 03:34:00 - mmengine - INFO - Epoch(train) [5][15/15] lr: 7.8125e-05 eta: 0:00:16 time: 0.2016 data_time: 0.1155 memory: 961 grad_norm: 11.5982 loss: 0.6940 top1_acc: 0.0000 top5_acc: 1.0000 loss_cls: 0.6940\n",
+ "05/15 03:34:02 - mmengine - INFO - Epoch(val) [5][5/5] acc/top1: 0.7000 acc/top5: 1.0000 acc/mean1: 0.7000 data_time: 0.3145 time: 0.3455\n",
+ "05/15 03:34:05 - mmengine - INFO - Exp name: tsn_imagenet-pretrained-r50_8xb32-1x1x3-100e_kinetics400-rgb_20230515_033334\n",
+ "05/15 03:34:05 - mmengine - INFO - Epoch(train) [6][15/15] lr: 7.8125e-05 eta: 0:00:13 time: 0.2366 data_time: 0.1440 memory: 961 grad_norm: 12.0952 loss: 0.6667 top1_acc: 0.0000 top5_acc: 1.0000 loss_cls: 0.6667\n",
+ "05/15 03:34:05 - mmengine - INFO - Saving checkpoint at 6 epochs\n",
+ "05/15 03:34:08 - mmengine - INFO - Epoch(val) [6][5/5] acc/top1: 0.6000 acc/top5: 1.0000 acc/mean1: 0.6000 data_time: 0.2172 time: 0.2403\n",
+ "05/15 03:34:10 - mmengine - INFO - Exp name: tsn_imagenet-pretrained-r50_8xb32-1x1x3-100e_kinetics400-rgb_20230515_033334\n",
+ "05/15 03:34:10 - mmengine - INFO - Epoch(train) [7][15/15] lr: 7.8125e-05 eta: 0:00:09 time: 0.1784 data_time: 0.0942 memory: 961 grad_norm: 12.4209 loss: 0.6570 top1_acc: 1.0000 top5_acc: 1.0000 loss_cls: 0.6570\n",
+ "05/15 03:34:11 - mmengine - INFO - Epoch(val) [7][5/5] acc/top1: 0.9000 acc/top5: 1.0000 acc/mean1: 0.9000 data_time: 0.1898 time: 0.2118\n",
+ "05/15 03:34:11 - mmengine - INFO - The previous best checkpoint /content/mmaction2/tutorial_exps/best_acc_top1_epoch_2.pth is removed\n",
+ "05/15 03:34:12 - mmengine - INFO - The best checkpoint with 0.9000 acc/top1 at 7 epoch is saved to best_acc_top1_epoch_7.pth.\n",
+ "05/15 03:34:15 - mmengine - INFO - Exp name: tsn_imagenet-pretrained-r50_8xb32-1x1x3-100e_kinetics400-rgb_20230515_033334\n",
+ "05/15 03:34:15 - mmengine - INFO - Epoch(train) [8][15/15] lr: 7.8125e-05 eta: 0:00:06 time: 0.2073 data_time: 0.1220 memory: 961 grad_norm: 11.4271 loss: 0.6241 top1_acc: 1.0000 top5_acc: 1.0000 loss_cls: 0.6241\n",
+ "05/15 03:34:17 - mmengine - INFO - Epoch(val) [8][5/5] acc/top1: 1.0000 acc/top5: 1.0000 acc/mean1: 1.0000 data_time: 0.3497 time: 0.3890\n",
+ "05/15 03:34:17 - mmengine - INFO - The previous best checkpoint /content/mmaction2/tutorial_exps/best_acc_top1_epoch_7.pth is removed\n",
+ "05/15 03:34:18 - mmengine - INFO - The best checkpoint with 1.0000 acc/top1 at 8 epoch is saved to best_acc_top1_epoch_8.pth.\n",
+ "05/15 03:34:21 - mmengine - INFO - Exp name: tsn_imagenet-pretrained-r50_8xb32-1x1x3-100e_kinetics400-rgb_20230515_033334\n",
+ "05/15 03:34:21 - mmengine - INFO - Epoch(train) [9][15/15] lr: 7.8125e-05 eta: 0:00:03 time: 0.2309 data_time: 0.1390 memory: 961 grad_norm: 12.3066 loss: 0.6451 top1_acc: 0.5000 top5_acc: 1.0000 loss_cls: 0.6451\n",
+ "05/15 03:34:21 - mmengine - INFO - Saving checkpoint at 9 epochs\n",
+ "05/15 03:34:23 - mmengine - INFO - Epoch(val) [9][5/5] acc/top1: 1.0000 acc/top5: 1.0000 acc/mean1: 1.0000 data_time: 0.2023 time: 0.2256\n",
+ "05/15 03:34:26 - mmengine - INFO - Exp name: tsn_imagenet-pretrained-r50_8xb32-1x1x3-100e_kinetics400-rgb_20230515_033334\n",
+ "05/15 03:34:26 - mmengine - INFO - Epoch(train) [10][15/15] lr: 7.8125e-05 eta: 0:00:00 time: 0.1733 data_time: 0.0951 memory: 961 grad_norm: 11.1461 loss: 0.5931 top1_acc: 1.0000 top5_acc: 1.0000 loss_cls: 0.5931\n",
+ "05/15 03:34:26 - mmengine - INFO - Saving checkpoint at 10 epochs\n",
+ "05/15 03:34:27 - mmengine - INFO - Epoch(val) [10][5/5] acc/top1: 1.0000 acc/top5: 1.0000 acc/mean1: 1.0000 data_time: 0.1836 time: 0.2048\n"
+ ]
},
{
- "cell_type": "markdown",
- "metadata": {
- "id": "zdSd7oTLlxIf"
- },
- "source": [
- "### Understand the log\n",
- "From the log, we can have a basic understanding the training process and know how well the recognizer is trained.\n",
- "\n",
- "Firstly, the ResNet-50 backbone pre-trained on ImageNet is loaded, this is a common practice since training from scratch is more cost. The log shows that all the weights of the ResNet-50 backbone are loaded except the `fc.bias` and `fc.weight`.\n",
- "\n",
- "Second, since the dataset we are using is small, we loaded a TSN model and finetune it for action recognition.\n",
- "The original TSN is trained on original Kinetics-400 dataset which contains 400 classes but Kinetics-400 Tiny dataset only have 2 classes. Therefore, the last FC layer of the pre-trained TSN for classification has different weight shape and is not used.\n",
- "\n",
- "Third, after training, the recognizer is evaluated by the default evaluation. The results show that the recognizer achieves 100% top1 accuracy and 100% top5 accuracy on the val dataset,\n",
- " \n",
- "Not bad!"
+ "output_type": "execute_result",
+ "data": {
+ "text/plain": [
+ "Recognizer2D(\n",
+ " (data_preprocessor): ActionDataPreprocessor()\n",
+ " (backbone): ResNet(\n",
+ " (conv1): ConvModule(\n",
+ " (conv): Conv2d(3, 64, kernel_size=(7, 7), stride=(2, 2), padding=(3, 3), bias=False)\n",
+ " (bn): BatchNorm2d(64, eps=1e-05, momentum=0.1, affine=True, track_running_stats=True)\n",
+ " (activate): ReLU(inplace=True)\n",
+ " )\n",
+ " (maxpool): MaxPool2d(kernel_size=3, stride=2, padding=1, dilation=1, ceil_mode=False)\n",
+ " (layer1): Sequential(\n",
+ " (0): Bottleneck(\n",
+ " (conv1): ConvModule(\n",
+ " (conv): Conv2d(64, 64, kernel_size=(1, 1), stride=(1, 1), bias=False)\n",
+ " (bn): BatchNorm2d(64, eps=1e-05, momentum=0.1, affine=True, track_running_stats=True)\n",
+ " (activate): ReLU(inplace=True)\n",
+ " )\n",
+ " (conv2): ConvModule(\n",
+ " (conv): Conv2d(64, 64, kernel_size=(3, 3), stride=(1, 1), padding=(1, 1), bias=False)\n",
+ " (bn): BatchNorm2d(64, eps=1e-05, momentum=0.1, affine=True, track_running_stats=True)\n",
+ " (activate): ReLU(inplace=True)\n",
+ " )\n",
+ " (conv3): ConvModule(\n",
+ " (conv): Conv2d(64, 256, kernel_size=(1, 1), stride=(1, 1), bias=False)\n",
+ " (bn): BatchNorm2d(256, eps=1e-05, momentum=0.1, affine=True, track_running_stats=True)\n",
+ " )\n",
+ " (relu): ReLU(inplace=True)\n",
+ " (downsample): ConvModule(\n",
+ " (conv): Conv2d(64, 256, kernel_size=(1, 1), stride=(1, 1), bias=False)\n",
+ " (bn): BatchNorm2d(256, eps=1e-05, momentum=0.1, affine=True, track_running_stats=True)\n",
+ " )\n",
+ " )\n",
+ " (1): Bottleneck(\n",
+ " (conv1): ConvModule(\n",
+ " (conv): Conv2d(256, 64, kernel_size=(1, 1), stride=(1, 1), bias=False)\n",
+ " (bn): BatchNorm2d(64, eps=1e-05, momentum=0.1, affine=True, track_running_stats=True)\n",
+ " (activate): ReLU(inplace=True)\n",
+ " )\n",
+ " (conv2): ConvModule(\n",
+ " (conv): Conv2d(64, 64, kernel_size=(3, 3), stride=(1, 1), padding=(1, 1), bias=False)\n",
+ " (bn): BatchNorm2d(64, eps=1e-05, momentum=0.1, affine=True, track_running_stats=True)\n",
+ " (activate): ReLU(inplace=True)\n",
+ " )\n",
+ " (conv3): ConvModule(\n",
+ " (conv): Conv2d(64, 256, kernel_size=(1, 1), stride=(1, 1), bias=False)\n",
+ " (bn): BatchNorm2d(256, eps=1e-05, momentum=0.1, affine=True, track_running_stats=True)\n",
+ " )\n",
+ " (relu): ReLU(inplace=True)\n",
+ " )\n",
+ " (2): Bottleneck(\n",
+ " (conv1): ConvModule(\n",
+ " (conv): Conv2d(256, 64, kernel_size=(1, 1), stride=(1, 1), bias=False)\n",
+ " (bn): BatchNorm2d(64, eps=1e-05, momentum=0.1, affine=True, track_running_stats=True)\n",
+ " (activate): ReLU(inplace=True)\n",
+ " )\n",
+ " (conv2): ConvModule(\n",
+ " (conv): Conv2d(64, 64, kernel_size=(3, 3), stride=(1, 1), padding=(1, 1), bias=False)\n",
+ " (bn): BatchNorm2d(64, eps=1e-05, momentum=0.1, affine=True, track_running_stats=True)\n",
+ " (activate): ReLU(inplace=True)\n",
+ " )\n",
+ " (conv3): ConvModule(\n",
+ " (conv): Conv2d(64, 256, kernel_size=(1, 1), stride=(1, 1), bias=False)\n",
+ " (bn): BatchNorm2d(256, eps=1e-05, momentum=0.1, affine=True, track_running_stats=True)\n",
+ " )\n",
+ " (relu): ReLU(inplace=True)\n",
+ " )\n",
+ " )\n",
+ " (layer2): Sequential(\n",
+ " (0): Bottleneck(\n",
+ " (conv1): ConvModule(\n",
+ " (conv): Conv2d(256, 128, kernel_size=(1, 1), stride=(1, 1), bias=False)\n",
+ " (bn): BatchNorm2d(128, eps=1e-05, momentum=0.1, affine=True, track_running_stats=True)\n",
+ " (activate): ReLU(inplace=True)\n",
+ " )\n",
+ " (conv2): ConvModule(\n",
+ " (conv): Conv2d(128, 128, kernel_size=(3, 3), stride=(2, 2), padding=(1, 1), bias=False)\n",
+ " (bn): BatchNorm2d(128, eps=1e-05, momentum=0.1, affine=True, track_running_stats=True)\n",
+ " (activate): ReLU(inplace=True)\n",
+ " )\n",
+ " (conv3): ConvModule(\n",
+ " (conv): Conv2d(128, 512, kernel_size=(1, 1), stride=(1, 1), bias=False)\n",
+ " (bn): BatchNorm2d(512, eps=1e-05, momentum=0.1, affine=True, track_running_stats=True)\n",
+ " )\n",
+ " (relu): ReLU(inplace=True)\n",
+ " (downsample): ConvModule(\n",
+ " (conv): Conv2d(256, 512, kernel_size=(1, 1), stride=(2, 2), bias=False)\n",
+ " (bn): BatchNorm2d(512, eps=1e-05, momentum=0.1, affine=True, track_running_stats=True)\n",
+ " )\n",
+ " )\n",
+ " (1): Bottleneck(\n",
+ " (conv1): ConvModule(\n",
+ " (conv): Conv2d(512, 128, kernel_size=(1, 1), stride=(1, 1), bias=False)\n",
+ " (bn): BatchNorm2d(128, eps=1e-05, momentum=0.1, affine=True, track_running_stats=True)\n",
+ " (activate): ReLU(inplace=True)\n",
+ " )\n",
+ " (conv2): ConvModule(\n",
+ " (conv): Conv2d(128, 128, kernel_size=(3, 3), stride=(1, 1), padding=(1, 1), bias=False)\n",
+ " (bn): BatchNorm2d(128, eps=1e-05, momentum=0.1, affine=True, track_running_stats=True)\n",
+ " (activate): ReLU(inplace=True)\n",
+ " )\n",
+ " (conv3): ConvModule(\n",
+ " (conv): Conv2d(128, 512, kernel_size=(1, 1), stride=(1, 1), bias=False)\n",
+ " (bn): BatchNorm2d(512, eps=1e-05, momentum=0.1, affine=True, track_running_stats=True)\n",
+ " )\n",
+ " (relu): ReLU(inplace=True)\n",
+ " )\n",
+ " (2): Bottleneck(\n",
+ " (conv1): ConvModule(\n",
+ " (conv): Conv2d(512, 128, kernel_size=(1, 1), stride=(1, 1), bias=False)\n",
+ " (bn): BatchNorm2d(128, eps=1e-05, momentum=0.1, affine=True, track_running_stats=True)\n",
+ " (activate): ReLU(inplace=True)\n",
+ " )\n",
+ " (conv2): ConvModule(\n",
+ " (conv): Conv2d(128, 128, kernel_size=(3, 3), stride=(1, 1), padding=(1, 1), bias=False)\n",
+ " (bn): BatchNorm2d(128, eps=1e-05, momentum=0.1, affine=True, track_running_stats=True)\n",
+ " (activate): ReLU(inplace=True)\n",
+ " )\n",
+ " (conv3): ConvModule(\n",
+ " (conv): Conv2d(128, 512, kernel_size=(1, 1), stride=(1, 1), bias=False)\n",
+ " (bn): BatchNorm2d(512, eps=1e-05, momentum=0.1, affine=True, track_running_stats=True)\n",
+ " )\n",
+ " (relu): ReLU(inplace=True)\n",
+ " )\n",
+ " (3): Bottleneck(\n",
+ " (conv1): ConvModule(\n",
+ " (conv): Conv2d(512, 128, kernel_size=(1, 1), stride=(1, 1), bias=False)\n",
+ " (bn): BatchNorm2d(128, eps=1e-05, momentum=0.1, affine=True, track_running_stats=True)\n",
+ " (activate): ReLU(inplace=True)\n",
+ " )\n",
+ " (conv2): ConvModule(\n",
+ " (conv): Conv2d(128, 128, kernel_size=(3, 3), stride=(1, 1), padding=(1, 1), bias=False)\n",
+ " (bn): BatchNorm2d(128, eps=1e-05, momentum=0.1, affine=True, track_running_stats=True)\n",
+ " (activate): ReLU(inplace=True)\n",
+ " )\n",
+ " (conv3): ConvModule(\n",
+ " (conv): Conv2d(128, 512, kernel_size=(1, 1), stride=(1, 1), bias=False)\n",
+ " (bn): BatchNorm2d(512, eps=1e-05, momentum=0.1, affine=True, track_running_stats=True)\n",
+ " )\n",
+ " (relu): ReLU(inplace=True)\n",
+ " )\n",
+ " )\n",
+ " (layer3): Sequential(\n",
+ " (0): Bottleneck(\n",
+ " (conv1): ConvModule(\n",
+ " (conv): Conv2d(512, 256, kernel_size=(1, 1), stride=(1, 1), bias=False)\n",
+ " (bn): BatchNorm2d(256, eps=1e-05, momentum=0.1, affine=True, track_running_stats=True)\n",
+ " (activate): ReLU(inplace=True)\n",
+ " )\n",
+ " (conv2): ConvModule(\n",
+ " (conv): Conv2d(256, 256, kernel_size=(3, 3), stride=(2, 2), padding=(1, 1), bias=False)\n",
+ " (bn): BatchNorm2d(256, eps=1e-05, momentum=0.1, affine=True, track_running_stats=True)\n",
+ " (activate): ReLU(inplace=True)\n",
+ " )\n",
+ " (conv3): ConvModule(\n",
+ " (conv): Conv2d(256, 1024, kernel_size=(1, 1), stride=(1, 1), bias=False)\n",
+ " (bn): BatchNorm2d(1024, eps=1e-05, momentum=0.1, affine=True, track_running_stats=True)\n",
+ " )\n",
+ " (relu): ReLU(inplace=True)\n",
+ " (downsample): ConvModule(\n",
+ " (conv): Conv2d(512, 1024, kernel_size=(1, 1), stride=(2, 2), bias=False)\n",
+ " (bn): BatchNorm2d(1024, eps=1e-05, momentum=0.1, affine=True, track_running_stats=True)\n",
+ " )\n",
+ " )\n",
+ " (1): Bottleneck(\n",
+ " (conv1): ConvModule(\n",
+ " (conv): Conv2d(1024, 256, kernel_size=(1, 1), stride=(1, 1), bias=False)\n",
+ " (bn): BatchNorm2d(256, eps=1e-05, momentum=0.1, affine=True, track_running_stats=True)\n",
+ " (activate): ReLU(inplace=True)\n",
+ " )\n",
+ " (conv2): ConvModule(\n",
+ " (conv): Conv2d(256, 256, kernel_size=(3, 3), stride=(1, 1), padding=(1, 1), bias=False)\n",
+ " (bn): BatchNorm2d(256, eps=1e-05, momentum=0.1, affine=True, track_running_stats=True)\n",
+ " (activate): ReLU(inplace=True)\n",
+ " )\n",
+ " (conv3): ConvModule(\n",
+ " (conv): Conv2d(256, 1024, kernel_size=(1, 1), stride=(1, 1), bias=False)\n",
+ " (bn): BatchNorm2d(1024, eps=1e-05, momentum=0.1, affine=True, track_running_stats=True)\n",
+ " )\n",
+ " (relu): ReLU(inplace=True)\n",
+ " )\n",
+ " (2): Bottleneck(\n",
+ " (conv1): ConvModule(\n",
+ " (conv): Conv2d(1024, 256, kernel_size=(1, 1), stride=(1, 1), bias=False)\n",
+ " (bn): BatchNorm2d(256, eps=1e-05, momentum=0.1, affine=True, track_running_stats=True)\n",
+ " (activate): ReLU(inplace=True)\n",
+ " )\n",
+ " (conv2): ConvModule(\n",
+ " (conv): Conv2d(256, 256, kernel_size=(3, 3), stride=(1, 1), padding=(1, 1), bias=False)\n",
+ " (bn): BatchNorm2d(256, eps=1e-05, momentum=0.1, affine=True, track_running_stats=True)\n",
+ " (activate): ReLU(inplace=True)\n",
+ " )\n",
+ " (conv3): ConvModule(\n",
+ " (conv): Conv2d(256, 1024, kernel_size=(1, 1), stride=(1, 1), bias=False)\n",
+ " (bn): BatchNorm2d(1024, eps=1e-05, momentum=0.1, affine=True, track_running_stats=True)\n",
+ " )\n",
+ " (relu): ReLU(inplace=True)\n",
+ " )\n",
+ " (3): Bottleneck(\n",
+ " (conv1): ConvModule(\n",
+ " (conv): Conv2d(1024, 256, kernel_size=(1, 1), stride=(1, 1), bias=False)\n",
+ " (bn): BatchNorm2d(256, eps=1e-05, momentum=0.1, affine=True, track_running_stats=True)\n",
+ " (activate): ReLU(inplace=True)\n",
+ " )\n",
+ " (conv2): ConvModule(\n",
+ " (conv): Conv2d(256, 256, kernel_size=(3, 3), stride=(1, 1), padding=(1, 1), bias=False)\n",
+ " (bn): BatchNorm2d(256, eps=1e-05, momentum=0.1, affine=True, track_running_stats=True)\n",
+ " (activate): ReLU(inplace=True)\n",
+ " )\n",
+ " (conv3): ConvModule(\n",
+ " (conv): Conv2d(256, 1024, kernel_size=(1, 1), stride=(1, 1), bias=False)\n",
+ " (bn): BatchNorm2d(1024, eps=1e-05, momentum=0.1, affine=True, track_running_stats=True)\n",
+ " )\n",
+ " (relu): ReLU(inplace=True)\n",
+ " )\n",
+ " (4): Bottleneck(\n",
+ " (conv1): ConvModule(\n",
+ " (conv): Conv2d(1024, 256, kernel_size=(1, 1), stride=(1, 1), bias=False)\n",
+ " (bn): BatchNorm2d(256, eps=1e-05, momentum=0.1, affine=True, track_running_stats=True)\n",
+ " (activate): ReLU(inplace=True)\n",
+ " )\n",
+ " (conv2): ConvModule(\n",
+ " (conv): Conv2d(256, 256, kernel_size=(3, 3), stride=(1, 1), padding=(1, 1), bias=False)\n",
+ " (bn): BatchNorm2d(256, eps=1e-05, momentum=0.1, affine=True, track_running_stats=True)\n",
+ " (activate): ReLU(inplace=True)\n",
+ " )\n",
+ " (conv3): ConvModule(\n",
+ " (conv): Conv2d(256, 1024, kernel_size=(1, 1), stride=(1, 1), bias=False)\n",
+ " (bn): BatchNorm2d(1024, eps=1e-05, momentum=0.1, affine=True, track_running_stats=True)\n",
+ " )\n",
+ " (relu): ReLU(inplace=True)\n",
+ " )\n",
+ " (5): Bottleneck(\n",
+ " (conv1): ConvModule(\n",
+ " (conv): Conv2d(1024, 256, kernel_size=(1, 1), stride=(1, 1), bias=False)\n",
+ " (bn): BatchNorm2d(256, eps=1e-05, momentum=0.1, affine=True, track_running_stats=True)\n",
+ " (activate): ReLU(inplace=True)\n",
+ " )\n",
+ " (conv2): ConvModule(\n",
+ " (conv): Conv2d(256, 256, kernel_size=(3, 3), stride=(1, 1), padding=(1, 1), bias=False)\n",
+ " (bn): BatchNorm2d(256, eps=1e-05, momentum=0.1, affine=True, track_running_stats=True)\n",
+ " (activate): ReLU(inplace=True)\n",
+ " )\n",
+ " (conv3): ConvModule(\n",
+ " (conv): Conv2d(256, 1024, kernel_size=(1, 1), stride=(1, 1), bias=False)\n",
+ " (bn): BatchNorm2d(1024, eps=1e-05, momentum=0.1, affine=True, track_running_stats=True)\n",
+ " )\n",
+ " (relu): ReLU(inplace=True)\n",
+ " )\n",
+ " )\n",
+ " (layer4): Sequential(\n",
+ " (0): Bottleneck(\n",
+ " (conv1): ConvModule(\n",
+ " (conv): Conv2d(1024, 512, kernel_size=(1, 1), stride=(1, 1), bias=False)\n",
+ " (bn): BatchNorm2d(512, eps=1e-05, momentum=0.1, affine=True, track_running_stats=True)\n",
+ " (activate): ReLU(inplace=True)\n",
+ " )\n",
+ " (conv2): ConvModule(\n",
+ " (conv): Conv2d(512, 512, kernel_size=(3, 3), stride=(2, 2), padding=(1, 1), bias=False)\n",
+ " (bn): BatchNorm2d(512, eps=1e-05, momentum=0.1, affine=True, track_running_stats=True)\n",
+ " (activate): ReLU(inplace=True)\n",
+ " )\n",
+ " (conv3): ConvModule(\n",
+ " (conv): Conv2d(512, 2048, kernel_size=(1, 1), stride=(1, 1), bias=False)\n",
+ " (bn): BatchNorm2d(2048, eps=1e-05, momentum=0.1, affine=True, track_running_stats=True)\n",
+ " )\n",
+ " (relu): ReLU(inplace=True)\n",
+ " (downsample): ConvModule(\n",
+ " (conv): Conv2d(1024, 2048, kernel_size=(1, 1), stride=(2, 2), bias=False)\n",
+ " (bn): BatchNorm2d(2048, eps=1e-05, momentum=0.1, affine=True, track_running_stats=True)\n",
+ " )\n",
+ " )\n",
+ " (1): Bottleneck(\n",
+ " (conv1): ConvModule(\n",
+ " (conv): Conv2d(2048, 512, kernel_size=(1, 1), stride=(1, 1), bias=False)\n",
+ " (bn): BatchNorm2d(512, eps=1e-05, momentum=0.1, affine=True, track_running_stats=True)\n",
+ " (activate): ReLU(inplace=True)\n",
+ " )\n",
+ " (conv2): ConvModule(\n",
+ " (conv): Conv2d(512, 512, kernel_size=(3, 3), stride=(1, 1), padding=(1, 1), bias=False)\n",
+ " (bn): BatchNorm2d(512, eps=1e-05, momentum=0.1, affine=True, track_running_stats=True)\n",
+ " (activate): ReLU(inplace=True)\n",
+ " )\n",
+ " (conv3): ConvModule(\n",
+ " (conv): Conv2d(512, 2048, kernel_size=(1, 1), stride=(1, 1), bias=False)\n",
+ " (bn): BatchNorm2d(2048, eps=1e-05, momentum=0.1, affine=True, track_running_stats=True)\n",
+ " )\n",
+ " (relu): ReLU(inplace=True)\n",
+ " )\n",
+ " (2): Bottleneck(\n",
+ " (conv1): ConvModule(\n",
+ " (conv): Conv2d(2048, 512, kernel_size=(1, 1), stride=(1, 1), bias=False)\n",
+ " (bn): BatchNorm2d(512, eps=1e-05, momentum=0.1, affine=True, track_running_stats=True)\n",
+ " (activate): ReLU(inplace=True)\n",
+ " )\n",
+ " (conv2): ConvModule(\n",
+ " (conv): Conv2d(512, 512, kernel_size=(3, 3), stride=(1, 1), padding=(1, 1), bias=False)\n",
+ " (bn): BatchNorm2d(512, eps=1e-05, momentum=0.1, affine=True, track_running_stats=True)\n",
+ " (activate): ReLU(inplace=True)\n",
+ " )\n",
+ " (conv3): ConvModule(\n",
+ " (conv): Conv2d(512, 2048, kernel_size=(1, 1), stride=(1, 1), bias=False)\n",
+ " (bn): BatchNorm2d(2048, eps=1e-05, momentum=0.1, affine=True, track_running_stats=True)\n",
+ " )\n",
+ " (relu): ReLU(inplace=True)\n",
+ " )\n",
+ " )\n",
+ " )\n",
+ " (cls_head): TSNHead(\n",
+ " (loss_cls): CrossEntropyLoss()\n",
+ " (consensus): AvgConsensus()\n",
+ " (avg_pool): AdaptiveAvgPool2d(output_size=(1, 1))\n",
+ " (dropout): Dropout(p=0.4, inplace=False)\n",
+ " (fc_cls): Linear(in_features=2048, out_features=2, bias=True)\n",
+ " )\n",
+ ")"
]
+ },
+ "metadata": {},
+ "execution_count": 15
+ }
+ ],
+ "source": [
+ "import os.path as osp\n",
+ "import mmengine\n",
+ "from mmengine.runner import Runner\n",
+ "\n",
+ "# Create work_dir\n",
+ "mmengine.mkdir_or_exist(osp.abspath(cfg.work_dir))\n",
+ "\n",
+ "# build the runner from config\n",
+ "runner = Runner.from_cfg(cfg)\n",
+ "\n",
+ "# start training\n",
+ "runner.train()"
+ ]
+ },
+ {
+ "cell_type": "markdown",
+ "metadata": {
+ "id": "zdSd7oTLlxIf"
+ },
+ "source": [
+ "### Understand the log\n",
+ "From the log, we can have a basic understanding the training process and know how well the recognizer is trained.\n",
+ "\n",
+ "Firstly, the ResNet-50 backbone pre-trained on ImageNet is loaded, this is a common practice since training from scratch is more cost. The log shows that all the weights of the ResNet-50 backbone are loaded except the `fc.bias` and `fc.weight`.\n",
+ "\n",
+ "Second, since the dataset we are using is small, we loaded a TSN model and finetune it for action recognition.\n",
+ "The original TSN is trained on original Kinetics-400 dataset which contains 400 classes but Kinetics-400 Tiny dataset only have 2 classes. Therefore, the last FC layer of the pre-trained TSN for classification has different weight shape and is not used.\n",
+ "\n",
+ "Third, after training, the recognizer is evaluated by the default evaluation. The results show that the recognizer achieves 100% top1 accuracy and 100% top5 accuracy on the val dataset,\n",
+ " \n",
+ "Not bad!"
+ ]
+ },
+ {
+ "cell_type": "markdown",
+ "metadata": {
+ "id": "ryVoSfZVmogw"
+ },
+ "source": [
+ "## Test the trained recognizer\n",
+ "\n",
+ "After finetuning the recognizer, let's check the prediction results!"
+ ]
+ },
+ {
+ "cell_type": "code",
+ "execution_count": 16,
+ "metadata": {
+ "colab": {
+ "base_uri": "https://localhost:8080/"
},
+ "id": "eyY3hCMwyTct",
+ "outputId": "34fbbdc5-b9fd-4fd2-8030-3ba56b10adbf"
+ },
+ "outputs": [
{
- "cell_type": "markdown",
- "metadata": {
- "id": "ryVoSfZVmogw"
- },
- "source": [
- "## Test the trained recognizer\n",
- "\n",
- "After finetuning the recognizer, let's check the prediction results!"
- ]
+ "output_type": "stream",
+ "name": "stdout",
+ "text": [
+ "05/15 03:34:36 - mmengine - INFO - Epoch(test) [10/10] acc/top1: 0.9000 acc/top5: 1.0000 acc/mean1: 0.9000 data_time: 0.0586 time: 0.7817\n"
+ ]
},
{
- "cell_type": "code",
- "execution_count": 16,
- "metadata": {
- "colab": {
- "base_uri": "https://localhost:8080/"
- },
- "id": "eyY3hCMwyTct",
- "outputId": "34fbbdc5-b9fd-4fd2-8030-3ba56b10adbf"
- },
- "outputs": [
- {
- "output_type": "stream",
- "name": "stdout",
- "text": [
- "05/15 03:34:36 - mmengine - INFO - Epoch(test) [10/10] acc/top1: 0.9000 acc/top5: 1.0000 acc/mean1: 0.9000 data_time: 0.0586 time: 0.7817\n"
- ]
- },
- {
- "output_type": "execute_result",
- "data": {
- "text/plain": [
- "{'acc/top1': 0.9, 'acc/top5': 1.0, 'acc/mean1': 0.9}"
- ]
- },
- "metadata": {},
- "execution_count": 16
- }
- ],
- "source": [
- "runner.test()"
+ "output_type": "execute_result",
+ "data": {
+ "text/plain": [
+ "{'acc/top1': 0.9, 'acc/top5': 1.0, 'acc/mean1': 0.9}"
]
+ },
+ "metadata": {},
+ "execution_count": 16
}
- ],
- "metadata": {
- "accelerator": "GPU",
- "colab": {
- "provenance": [],
- "toc_visible": true
- },
- "kernelspec": {
- "display_name": "mmact_dev",
- "language": "python",
- "name": "python3"
- },
- "language_info": {
- "codemirror_mode": {
- "name": "ipython",
- "version": 3
- },
- "file_extension": ".py",
- "mimetype": "text/x-python",
- "name": "python",
- "nbconvert_exporter": "python",
- "pygments_lexer": "ipython3",
- "version": "3.7.12"
- },
- "vscode": {
- "interpreter": {
- "hash": "189c342a4747645665e89db23000ac4d4edb7a87c4cd0b2f881610f468fb778d"
- }
- }
+ ],
+ "source": [
+ "runner.test()"
+ ]
+ }
+ ],
+ "metadata": {
+ "accelerator": "GPU",
+ "colab": {
+ "provenance": [],
+ "toc_visible": true
+ },
+ "kernelspec": {
+ "display_name": "mmact_dev",
+ "language": "python",
+ "name": "python3"
+ },
+ "language_info": {
+ "codemirror_mode": {
+ "name": "ipython",
+ "version": 3
+ },
+ "file_extension": ".py",
+ "mimetype": "text/x-python",
+ "name": "python",
+ "nbconvert_exporter": "python",
+ "pygments_lexer": "ipython3",
+ "version": "3.7.12"
},
- "nbformat": 4,
- "nbformat_minor": 0
+ "vscode": {
+ "interpreter": {
+ "hash": "189c342a4747645665e89db23000ac4d4edb7a87c4cd0b2f881610f468fb778d"
+ }
+ }
+ },
+ "nbformat": 4,
+ "nbformat_minor": 0
}
diff --git a/demo/webcam_demo.py b/demo/webcam_demo.py
index cdd8585540..de87c8aa32 100644
--- a/demo/webcam_demo.py
+++ b/demo/webcam_demo.py
@@ -139,7 +139,7 @@ def inference():
# Forward the model
with torch.no_grad():
result = model.test_step(cur_data)[0]
- scores = result.pred_scores.item.tolist()
+ scores = result.pred_score.tolist()
scores = np.array(scores)
score_cache.append(scores)
scores_sum += scores
diff --git a/docs/en/get_started/guide_to_framework.md b/docs/en/get_started/guide_to_framework.md
index c65d65331b..3dc1c2314b 100644
--- a/docs/en/get_started/guide_to_framework.md
+++ b/docs/en/get_started/guide_to_framework.md
@@ -179,7 +179,8 @@ class VideoPack(BaseTransform):
def transform(self, results):
packed_results = dict()
inputs = to_tensor(results['imgs'])
- data_sample = ActionDataSample().set_gt_labels(results['label'])
+ data_sample = ActionDataSample()
+ data_sample.set_gt_label(results['label'])
metainfo = {k: results[k] for k in self.meta_keys if k in results}
data_sample.set_metainfo(metainfo)
packed_results['inputs'] = inputs
@@ -219,7 +220,7 @@ print('num_clips: ', data_sample.num_clips)
print('clip_len: ', data_sample.clip_len)
# Get label of the inputs
-print('label: ', data_sample.gt_labels.item)
+print('label: ', data_sample.gt_label)
```
```
@@ -321,7 +322,7 @@ print('num_clips: ', data_sample.num_clips)
print('clip_len: ', data_sample.clip_len)
# Get label of the inputs
-print('label: ', data_sample.gt_labels.item)
+print('label: ', data_sample.gt_label)
from mmengine.runner import Runner
@@ -481,7 +482,7 @@ class ClsHeadZelda(BaseModule):
def loss(self, feats, data_samples):
cls_scores = self(feats)
- labels = torch.stack([x.gt_labels.item for x in data_samples])
+ labels = torch.stack([x.gt_label for x in data_samples])
labels = labels.squeeze()
if labels.shape == torch.Size([]):
@@ -589,8 +590,8 @@ with torch.no_grad():
data_batch_test = copy.deepcopy(batched_packed_results)
data = model.data_preprocessor(data_batch_test, training=False)
predictions = model(**data, mode='predict')
-print('Label of Sample[0]', predictions[0].gt_labels.item)
-print('Scores of Sample[0]', predictions[0].pred_scores.item)
+print('Label of Sample[0]', predictions[0].gt_label)
+print('Scores of Sample[0]', predictions[0].pred_score)
```
```shell
@@ -661,8 +662,8 @@ class AccuracyMetric(BaseMetric):
data_samples = copy.deepcopy(data_samples)
for data_sample in data_samples:
result = dict()
- scores = data_sample['pred_scores']['item'].cpu().numpy()
- label = data_sample['gt_labels']['item'].item()
+ scores = data_sample['pred_score'].cpu().numpy()
+ label = data_sample['gt_label'].item()
result['scores'] = scores
result['label'] = label
self.results.append(result)
diff --git a/docs/en/get_started/installation.md b/docs/en/get_started/installation.md
index 8cc64b7798..1685f97478 100644
--- a/docs/en/get_started/installation.md
+++ b/docs/en/get_started/installation.md
@@ -121,7 +121,7 @@ label_file = 'tools/data/kinetics/label_map_k400.txt'
model = init_recognizer(config_file, checkpoint_file, device='cpu') # or device='cuda:0'
pred_result = inference_recognizer(model, video_file)
-pred_scores = pred_result.pred_scores.item.tolist()
+pred_scores = pred_result.pred_score.tolist()
score_tuples = tuple(zip(range(len(pred_scores)), pred_scores))
score_sorted = sorted(score_tuples, key=itemgetter(1), reverse=True)
top5_label = score_sorted[:5]
diff --git a/docs/en/notes/changelog.md b/docs/en/notes/changelog.md
index 66dfd3b144..f6383f2863 100644
--- a/docs/en/notes/changelog.md
+++ b/docs/en/notes/changelog.md
@@ -1,5 +1,33 @@
# Changelog
+## 1.2.0 (10/12/2023)
+
+**Highlights**
+
+- Support the Training of ActionClip
+- Support VindLU multi-modality algorithm
+- Support MobileOne TSN/TSM
+
+**New Features**
+
+- Support the Training of ActionClip ([2620](https://github.com/open-mmlab/mmaction2/pull/2620))
+- Support video retrieval dataset MSVD ([2622](https://github.com/open-mmlab/mmaction2/pull/2622))
+- Support VindLU multi-modality algorithm ([2667](https://github.com/open-mmlab/mmaction2/pull/2667))
+- Support Dense Regression Network for Video Grounding ([2668](https://github.com/open-mmlab/mmaction2/pull/2668))
+
+**Improvements**
+
+- Support Video Demos ([2602](https://github.com/open-mmlab/mmaction2/pull/2602))
+- Support Audio Demos ([2603](https://github.com/open-mmlab/mmaction2/pull/2603))
+- Add README_zh-CN.md for Swin and VideoMAE ([2621](https://github.com/open-mmlab/mmaction2/pull/2621))
+- Support MobileOne TSN/TSM ([2656](https://github.com/open-mmlab/mmaction2/pull/2656))
+- Support SlowOnly K700 feature to train localization models ([2673](https://github.com/open-mmlab/mmaction2/pull/2673))
+
+**Bug Fixes**
+
+- Refine ActionDataSample structure ([2658](https://github.com/open-mmlab/mmaction2/pull/2658))
+- Fix MPS device ([2619](https://github.com/open-mmlab/mmaction2/pull/2619))
+
## 1.1.0 (7/3/2023)
**Highlights**
diff --git a/docs/en/user_guides/prepare_dataset.md b/docs/en/user_guides/prepare_dataset.md
index c13d448106..35f2aacc5f 100644
--- a/docs/en/user_guides/prepare_dataset.md
+++ b/docs/en/user_guides/prepare_dataset.md
@@ -8,6 +8,7 @@ MMAction2 supports many existing datasets. In this chapter, we will lead you to
- [Use a custom dataset](#use-a-custom-dataset)
- [Action Recognition](#action-recognition)
- [Skeleton-based Action Recognition](#skeleton-based-action-recognition)
+ - [Audio-based Action Recognition](#audio-based-action-recognition)
- [Spatio-temporal Action Detection](#spatio-temporal-action-detection)
- [Temporal Action Localization](#temporal-action-localization)
- [Use mixed datasets for training](#use-mixed-datasets-for-training)
@@ -24,7 +25,7 @@ To make video decoding faster, we support several efficient video loading librar
## Use built-in datasets
-MMAction2 already supports many datasets, we provide shell scripts for data preparation under the path `$MMACTION2/tools/data/`, please refer to [supported datasets](../datasetzoo_satatistics.md) for details to prepare specific datasets.
+MMAction2 already supports many datasets, we provide shell scripts for data preparation under the path `$MMACTION2/tools/data/`, please refer to [supported datasets](https://mmaction2.readthedocs.io/en/latest/datasetzoo_statistics.html) for details to prepare specific datasets.
## Use a custom dataset
@@ -32,6 +33,7 @@ The simplest way is to convert your dataset to existing dataset formats:
- `RawFrameDataset` and `VideoDataset` for [Action Recognition](#action-recognition)
- `PoseDataset` for [Skeleton-based Action Recognition](#skeleton-based-action-recognition)
+- `AudioDataset` for [Audio-based Action Recognition](#Audio-based-action-recognition)
- `AVADataset` for [Spatio-temporal Action Detection](#spatio-temporal-action-detection)
- `ActivityNetDataset` for [Temporal Action Localization](#temporal-action-localization)
@@ -172,6 +174,46 @@ The task recognizes the action class based on the skeleton sequence (time sequen
Support other keypoint formats needs further modification, please refer to [customize dataset](../advanced_guides/customize_dataset.md).
+### Audio-based Action Recognition
+
+MMAction2 provides support for audio-based action recognition tasks utilizing the `AudioDataset`. This task employs mel spectrogram features as input. An example annotation file format is as follows:
+
+```
+ihWykL5mYRI.npy 300 153
+lumzQD42AN8.npy 240 321
+sWFRmD9Of4s.npy 250 250
+w_IpfgRsBVA.npy 300 356
+```
+
+Each line represents a training sample. Taking the first line as an example, `ihWykL5mYRI.npy` corresponds to the filename of the mel spectrogram feature. The value `300` represents the total number of frames of the original video corresponding to this mel spectrogram feature, and `153` denotes the class label. We take the following two steps to perpare the mel spectrogram feature data:
+
+First, extract `audios` from videos:
+
+```shell
+cd $MMACTION2
+python tools/data/extract_audio.py ${ROOT} ${DST_ROOT} [--ext ${EXT}] [--num-workers ${N_WORKERS}] \
+ [--level ${LEVEL}]
+```
+
+- `ROOT`: The root directory of the videos.
+- `DST_ROOT`: The destination root directory of the audios.
+- `EXT`: Extension of the video files. e.g., `mp4`.
+- `N_WORKERS`: Number of processes to be used.
+
+Next, offline generate the `mel spectrogram features` from the audios:
+
+```shell
+cd $MMACTION2
+python tools/data/build_audio_features.py ${AUDIO_HOME_PATH} ${SPECTROGRAM_SAVE_PATH} [--level ${LEVEL}] \
+ [--ext $EXT] [--num-workers $N_WORKERS] [--part $PART]
+```
+
+- `AUDIO_HOME_PATH`: The root directory of the audio files.
+- `SPECTROGRAM_SAVE_PATH`: The destination root directory of the audio features.
+- `EXT`: Extension of the audio files. e.g., `m4a`.
+- `N_WORKERS`: Number of processes to be used.
+- `PART`: Determines how many parts to be splited and which part to run. e.g., `2/5` means splitting all files into 5-fold and executing the 2nd part. This is useful if you have several machines.
+
### Spatio-temporal Action Detection
MMAction2 supports the task based on `AVADataset`. The annotation contains groundtruth bbox and proposal bbox.
diff --git a/docs/zh_cn/get_started/guide_to_framework.md b/docs/zh_cn/get_started/guide_to_framework.md
index b92c376b5d..0dc6462195 100644
--- a/docs/zh_cn/get_started/guide_to_framework.md
+++ b/docs/zh_cn/get_started/guide_to_framework.md
@@ -180,7 +180,7 @@ class VideoPack(BaseTransform):
def transform(self, results):
packed_results = dict()
inputs = to_tensor(results['imgs'])
- data_sample = ActionDataSample().set_gt_labels(results['label'])
+ data_sample = ActionDataSample().set_gt_label(results['label'])
metainfo = {k: results[k] for k in self.meta_keys if k in results}
data_sample.set_metainfo(metainfo)
packed_results['inputs'] = inputs
@@ -220,7 +220,7 @@ print('num_clips: ', data_sample.num_clips)
print('clip_len: ', data_sample.clip_len)
# ่ทๅ่พๅ
ฅ็ๆ ็ญพ
-print('label: ', data_sample.gt_labels.item)
+print('label: ', data_sample.gt_label)
```
```
@@ -322,7 +322,7 @@ print('num_clips: ', data_sample.num_clips)
print('clip_len: ', data_sample.clip_len)
# ่ทๅ่พๅ
ฅ็ๆ ็ญพ
-print('label: ', data_sample.gt_labels.item)
+print('label: ', data_sample.gt_label)
from mmengine.runner import Runner
@@ -482,7 +482,7 @@ class ClsHeadZelda(BaseModule):
def loss(self, feats, data_samples):
cls_scores = self(feats)
- labels = torch.stack([x.gt_labels.item for x in data_samples])
+ labels = torch.stack([x.gt_label for x in data_samples])
labels = labels.squeeze()
if labels.shape == torch.Size([]):
@@ -590,8 +590,8 @@ with torch.no_grad():
data_batch_test = copy.deepcopy(batched_packed_results)
data = model.data_preprocessor(data_batch_test, training=False)
predictions = model(**data, mode='predict')
-print('Label of Sample[0]', predictions[0].gt_labels.item)
-print('Scores of Sample[0]', predictions[0].pred_scores.item)
+print('Label of Sample[0]', predictions[0].gt_label)
+print('Scores of Sample[0]', predictions[0].pred_score)
```
```shell
@@ -662,8 +662,8 @@ class AccuracyMetric(BaseMetric):
data_samples = copy.deepcopy(data_samples)
for data_sample in data_samples:
result = dict()
- scores = data_sample['pred_scores']['item'].cpu().numpy()
- label = data_sample['gt_labels']['item'].item()
+ scores = data_sample['pred_score'].cpu().numpy()
+ label = data_sample['gt_label'].item()
result['scores'] = scores
result['label'] = label
self.results.append(result)
diff --git a/docs/zh_cn/get_started/installation.md b/docs/zh_cn/get_started/installation.md
index 0e144ce6eb..091a8a5e03 100644
--- a/docs/zh_cn/get_started/installation.md
+++ b/docs/zh_cn/get_started/installation.md
@@ -120,7 +120,7 @@ label_file = 'tools/data/kinetics/label_map_k400.txt'
model = init_recognizer(config_file, checkpoint_file, device='cpu') # or device='cuda:0'
pred_result = inference_recognizer(model, video_file)
-pred_scores = pred_result.pred_scores.item.tolist()
+pred_scores = pred_result.pred_score.tolist()
score_tuples = tuple(zip(range(len(pred_scores)), pred_scores))
score_sorted = sorted(score_tuples, key=itemgetter(1), reverse=True)
top5_label = score_sorted[:5]
diff --git a/docs/zh_cn/user_guides/prepare_dataset.md b/docs/zh_cn/user_guides/prepare_dataset.md
index b8cdfee69b..44348580bc 100644
--- a/docs/zh_cn/user_guides/prepare_dataset.md
+++ b/docs/zh_cn/user_guides/prepare_dataset.md
@@ -8,6 +8,7 @@ MMAction2 ๆฏๆ่ฎธๅค็ฐๆ็ๆฐๆฎ้ใๅจๆฌ็ซ ไธญ๏ผๆไปฌๅฐๅผๅฏผๆจๅ
- [ไฝฟ็จ่ชๅฎไนๆฐๆฎ้](#ไฝฟ็จ่ชๅฎไนๆฐๆฎ้)
- [ๅจไฝ่ฏๅซ](#ๅจไฝ่ฏๅซ)
- [ๅบไบ้ชจ้ชผ็ๅจไฝ่ฏๅซ](#ๅบไบ้ชจ้ชผ็ๅจไฝ่ฏๅซ)
+ - [ๅบไบ้ณ้ข็ๅจไฝ่ฏๅซ](#ๅบไบ้ณ้ข็ๅจไฝ่ฏๅซ)
- [ๆถ็ฉบๅจไฝๆฃๆต](#ๆถ็ฉบๅจไฝๆฃๆต)
- [ๆถๅบๅจไฝๅฎไฝ](#ๆถๅบๅจไฝๅฎไฝ)
- [ไฝฟ็จๆททๅๆฐๆฎ้่ฟ่ก่ฎญ็ป](#ไฝฟ็จๆททๅๆฐๆฎ้่ฟ่ก่ฎญ็ป)
@@ -20,7 +21,7 @@ MMAction2 ๆฏๆไธค็ง็ฑปๅ็ๆฐๆฎๆ ผๅผ๏ผๅๅงๅธงๅ่ง้ขใๅ่
ๅจไน
## ไฝฟ็จๅ
็ฝฎๆฐๆฎ้
-MMAction2 ๅทฒ็ปๆฏๆ่ฎธๅคๆฐๆฎ้๏ผๆไปฌๅจ่ทฏๅพ `$MMACTION2/tools/data/` ไธๆไพไบ็จไบๆฐๆฎๅๅค็ shell ่ๆฌ๏ผ่ฏทๅ่[ๆฏๆ็ๆฐๆฎ้](../datasetzoo_satatistics.md)ไปฅ่ทๅๅๅค็นๅฎๆฐๆฎ้็่ฏฆ็ปไฟกๆฏใ
+MMAction2 ๅทฒ็ปๆฏๆ่ฎธๅคๆฐๆฎ้๏ผๆไปฌๅจ่ทฏๅพ `$MMACTION2/tools/data/` ไธๆไพไบ็จไบๆฐๆฎๅๅค็ shell ่ๆฌ๏ผ่ฏทๅ่[ๆฏๆ็ๆฐๆฎ้](https://mmaction2.readthedocs.io/zh_CN/latest/datasetzoo_statistics.html)ไปฅ่ทๅๅๅค็นๅฎๆฐๆฎ้็่ฏฆ็ปไฟกๆฏใ
## ไฝฟ็จ่ชๅฎไนๆฐๆฎ้
@@ -28,6 +29,7 @@ MMAction2 ๅทฒ็ปๆฏๆ่ฎธๅคๆฐๆฎ้๏ผๆไปฌๅจ่ทฏๅพ `$MMACTION2/tools/data/`
- `RawFrameDataset` ๅ `VideoDataset` ็จไบ[ๅจไฝ่ฏๅซ](#ๅจไฝ่ฏๅซ)
- `PoseDataset` ็จไบ[ๅบไบ้ชจ้ชผ็ๅจไฝ่ฏๅซ](#ๅบไบ้ชจ้ชผ็ๅจไฝ่ฏๅซ)
+- `AudioDataset` ็จไบ[ๅบไบ้ณ้ขๅจไฝ่ฏๅซ](#ๅบไบ้ณ้ขๅจไฝ่ฏๅซ)
- `AVADataset` ็จไบ[ๆถ็ฉบๅจไฝๆฃๆต](#ๆถ็ฉบๅจไฝๆฃๆต)
- `ActivityNetDataset` ็จไบ[ๆถๅบๅจไฝๅฎไฝ](#ๆถๅบๅจไฝๅฎไฝ)
@@ -163,6 +165,46 @@ data = dict(
ๆฏๆๅ
ถไปๅ
ณ้ฎ็นๆ ผๅผ้่ฆ่ฟ่ก่ฟไธๆญฅไฟฎๆน๏ผ่ฏทๅ่[่ชๅฎไนๆฐๆฎ้](../advanced_guides/customize_dataset.md)ใ
+### ๅบไบ้ณ้ข็ๅจไฝ่ฏๅซ
+
+MMAction2 ๆฏๆๅบไบ `AudioDataset` ็้ณ้ขๅจไฝ่ฏๅซไปปๅกใ่ฏฅไปปๅกไฝฟ็จๆข
ๅฐ้ข่ฐฑ็นๅพไฝไธบ่พๅ
ฅ, ๆณจ้ๆไปถๆ ผๅผ็คบไพๅฆไธ๏ผ
+
+```
+ihWykL5mYRI.npy 300 153
+lumzQD42AN8.npy 240 321
+sWFRmD9Of4s.npy 250 250
+w_IpfgRsBVA.npy 300 356
+```
+
+ๆฏไธ่กไปฃ่กจไธไธช่ฎญ็ปๆ ทๆฌ๏ผไปฅ็ฌฌไธ่กไธบไพ๏ผ`ihWykL5mYRI.npy` ไธบๆข
ๅฐ้ข่ฐฑ็นๅพ็ๆไปถๅ๏ผ`300` ไธบ่ฏฅๆข
ๅฐ้ข่ฐฑ็นๅพๆไปถๅฏนๅบ็ๅ่ง้ขๆไปถ็ๆปๅธงๆฐ๏ผ`153` ไธบ็ฑปๅซๆ ็ญพใๆไปฌๅไปฅไธไธค้ถๆฎต็ๆๆ้่ฆ็ๆข
ๅฐ้ข่ฐฑ็นๅพๆไปถๆฐๆฎ๏ผ
+
+้ฆๅ
๏ผ้่ฟ่ง้ขๆไปถๆๅ`้ณ้ขๆไปถ`:
+
+```
+cd $MMACTION2
+python tools/data/extract_audio.py ${ROOT} ${DST_ROOT} [--ext ${EXT}] [--num-workers ${N_WORKERS}] \
+ [--level ${LEVEL}]
+```
+
+- `ROOT`: ่ง้ข็ๆ น็ฎๅฝใ
+- `DST_ROOT`: ๅญๆพ็ๆ้ณ้ข็ๆ น็ฎๅฝใ
+- `EXT`: ่ง้ข็ๅ็ผๅ๏ผๅฆ `mp4`ใ
+- `N_WORKERS`: ไฝฟ็จ็่ฟ็จๆฐ้ใ
+
+ไธไธๆญฅ๏ผไป้ณ้ขๆไปถ็ๆ`ๆข
ๅฐ้ข่ฐฑ็นๅพ`:
+
+```
+cd $MMACTION2
+python tools/data/build_audio_features.py ${AUDIO_HOME_PATH} ${SPECTROGRAM_SAVE_PATH} [--level ${LEVEL}] \
+ [--ext $EXT] [--num-workers $N_WORKERS] [--part $PART]
+```
+
+- `AUDIO_HOME_PATH`: ้ณ้ขๆไปถ็ๆ น็ฎๅฝใ
+- `SPECTROGRAM_SAVE_PATH`: ๅญๆพ็ๆ้ณ้ข็นๅพ็ๆ น็ฎๅฝใ
+- `EXT`: ้ณ้ข็ๅ็ผๅ๏ผๅฆ `m4a`ใ
+- `N_WORKERS`: ไฝฟ็จ็่ฟ็จๆฐ้ใ
+- `PART`: ๅฐๅฎๆด็่งฃ็ ไปปๅกๅไธบๅ ้จๅๅนถๆง่กๅ
ถไธญไธไปฝใๅฆ `2/5` ่กจ็คบๅฐๆๆๅพ
่งฃ็ ๆฐๆฎๅๆ 5 ไปฝ๏ผๅนถๅฏนๅ
ถไธญ็็ฌฌ 2 ไปฝ่ฟ่ก่งฃ็ ใ่ฟไธ้้กนๅจ็จๆทๆๅคๅฐๆบๅจๆถๅๆฅไฝ็จใ
+
### ๆถ็ฉบๅจไฝๆฃๆต
MMAction2 ๆฏๆๅบไบ `AVADataset` ็ๆถ็ฉบๅจไฝๆฃๆตไปปๅกใๆณจ้ๅ
ๅซ็ๅฎ่พน็ๆกๅๆ่ฎฎ่พน็ๆกใ
diff --git a/mmaction/__init__.py b/mmaction/__init__.py
index e6453c9d44..bb5c805905 100644
--- a/mmaction/__init__.py
+++ b/mmaction/__init__.py
@@ -6,7 +6,7 @@
from .version import __version__
mmcv_minimum_version = '2.0.0rc4'
-mmcv_maximum_version = '2.1.0'
+mmcv_maximum_version = '2.2.0'
mmcv_version = digit_version(mmcv.__version__)
mmengine_minimum_version = '0.7.1'
diff --git a/mmaction/apis/inference.py b/mmaction/apis/inference.py
index 27a4136780..4b2b4f8c4b 100644
--- a/mmaction/apis/inference.py
+++ b/mmaction/apis/inference.py
@@ -1,4 +1,5 @@
# Copyright (c) OpenMMLab. All rights reserved.
+import os.path as osp
from pathlib import Path
from typing import List, Optional, Tuple, Union
@@ -69,7 +70,7 @@ def inference_recognizer(model: nn.Module,
Returns:
:obj:`ActionDataSample`: The inference results. Specifically, the
- predicted scores are saved at ``result.pred_scores.item``.
+ predicted scores are saved at ``result.pred_score``.
"""
if test_pipeline is None:
@@ -81,8 +82,11 @@ def inference_recognizer(model: nn.Module,
input_flag = None
if isinstance(video, dict):
input_flag = 'dict'
- elif isinstance(video, str):
- input_flag = 'video'
+ elif isinstance(video, str) and osp.exists(video):
+ if video.endswith('.npy'):
+ input_flag = 'audio'
+ else:
+ input_flag = 'video'
else:
raise RuntimeError(f'The type of argument `video` is not supported: '
f'{type(video)}')
@@ -91,6 +95,12 @@ def inference_recognizer(model: nn.Module,
data = video
if input_flag == 'video':
data = dict(filename=video, label=-1, start_index=0, modality='RGB')
+ if input_flag == 'audio':
+ data = dict(
+ audio_path=video,
+ total_frames=len(np.load(video)),
+ start_index=0,
+ label=-1)
data = test_pipeline(data)
data = pseudo_collate([data])
@@ -121,7 +131,7 @@ def inference_skeleton(model: nn.Module,
Returns:
:obj:`ActionDataSample`: The inference results. Specifically, the
- predicted scores are saved at ``result.pred_scores.item``.
+ predicted scores are saved at ``result.pred_score``.
"""
if test_pipeline is None:
cfg = model.cfg
diff --git a/mmaction/apis/inferencers/actionrecog_inferencer.py b/mmaction/apis/inferencers/actionrecog_inferencer.py
index f45f137b59..cc6e60b0da 100644
--- a/mmaction/apis/inferencers/actionrecog_inferencer.py
+++ b/mmaction/apis/inferencers/actionrecog_inferencer.py
@@ -356,6 +356,6 @@ def pred2dict(self, data_sample: ActionDataSample) -> Dict:
dict: The output dictionary.
"""
result = {}
- result['pred_labels'] = data_sample.pred_labels.item.tolist()
- result['pred_scores'] = data_sample.pred_scores.item.tolist()
+ result['pred_labels'] = data_sample.pred_label.tolist()
+ result['pred_scores'] = data_sample.pred_score.tolist()
return result
diff --git a/mmaction/configs/recognition/swin/swin_base_p244_w877_in1k_pre_8xb8_amp_32x2x1_30e_kinetics400_rgb.py b/mmaction/configs/recognition/swin/swin_base_p244_w877_in1k_pre_8xb8_amp_32x2x1_30e_kinetics400_rgb.py
index 187ebf4a62..e3d3377630 100644
--- a/mmaction/configs/recognition/swin/swin_base_p244_w877_in1k_pre_8xb8_amp_32x2x1_30e_kinetics400_rgb.py
+++ b/mmaction/configs/recognition/swin/swin_base_p244_w877_in1k_pre_8xb8_amp_32x2x1_30e_kinetics400_rgb.py
@@ -28,6 +28,7 @@
cls_head=dict(in_channels=1024)))
# dataset settings
+dataset_type = VideoDataset
data_root = 'data/kinetics400/videos_train'
data_root_val = 'data/kinetics400/videos_val'
ann_file_train = 'data/kinetics400/kinetics400_train_list_videos.txt'
diff --git a/mmaction/configs/recognition/swin/swin_large_p244_w877_in22k_pre_16xb8_amp_32x2x1_30e_kinetics700_rgb.py b/mmaction/configs/recognition/swin/swin_large_p244_w877_in22k_pre_16xb8_amp_32x2x1_30e_kinetics700_rgb.py
new file mode 100644
index 0000000000..a0a7b84303
--- /dev/null
+++ b/mmaction/configs/recognition/swin/swin_large_p244_w877_in22k_pre_16xb8_amp_32x2x1_30e_kinetics700_rgb.py
@@ -0,0 +1,56 @@
+# Copyright (c) OpenMMLab. All rights reserved.
+from mmengine.config import read_base
+
+with read_base():
+ from .swin_large_p244_w877_in22k_pre_8xb8_amp_32x2x1_30e_kinetics400_rgb import * # noqa: E501
+
+model.update(dict(cls_head=dict(num_classes=700)))
+
+# dataset
+data_root = 'data/kinetics700/videos_train'
+data_root_val = 'data/kinetics700/videos_val'
+ann_file_train = 'data/kinetics700/kinetics700_train_list_videos.txt'
+ann_file_val = 'data/kinetics700/kinetics700_val_list_videos.txt'
+ann_file_test = 'data/kinetics700/kinetics700_val_list_videos.txt'
+
+dataset_type = VideoDataset
+train_dataloader = dict(
+ batch_size=8,
+ num_workers=8,
+ persistent_workers=True,
+ sampler=dict(type=DefaultSampler, shuffle=True),
+ dataset=dict(
+ type=dataset_type,
+ ann_file=ann_file_train,
+ data_prefix=dict(video=data_root),
+ pipeline=train_pipeline))
+val_dataloader = dict(
+ batch_size=8,
+ num_workers=8,
+ persistent_workers=True,
+ sampler=dict(type=DefaultSampler, shuffle=False),
+ dataset=dict(
+ type=dataset_type,
+ ann_file=ann_file_val,
+ data_prefix=dict(video=data_root_val),
+ pipeline=val_pipeline,
+ test_mode=True))
+test_dataloader = dict(
+ batch_size=1,
+ num_workers=8,
+ persistent_workers=True,
+ sampler=dict(type=DefaultSampler, shuffle=False),
+ dataset=dict(
+ type=dataset_type,
+ ann_file=ann_file_test,
+ data_prefix=dict(video=data_root_val),
+ pipeline=test_pipeline,
+ test_mode=True))
+
+optim_wrapper.update(dict(optimizer=dict(lr=2e-3)))
+
+# Default setting for scaling LR automatically
+# - `enable` means enable scaling LR automatically
+# or not by default.
+# - `base_batch_size` = (16 GPUs) x (8 samples per GPU).
+auto_scale_lr.update(dict(enable=False, base_batch_size=128))
diff --git a/mmaction/configs/recognition/swin/swin_large_p244_w877_in22k_pre_8xb8_amp_32x2x1_30e_kinetics400_rgb.py b/mmaction/configs/recognition/swin/swin_large_p244_w877_in22k_pre_8xb8_amp_32x2x1_30e_kinetics400_rgb.py
new file mode 100644
index 0000000000..b4c23ecfea
--- /dev/null
+++ b/mmaction/configs/recognition/swin/swin_large_p244_w877_in22k_pre_8xb8_amp_32x2x1_30e_kinetics400_rgb.py
@@ -0,0 +1,156 @@
+# Copyright (c) OpenMMLab. All rights reserved.
+from mmengine.config import read_base
+
+with read_base():
+ from ..._base_.models.swin_tiny import *
+ from ..._base_.default_runtime import *
+
+from mmengine.dataset import DefaultSampler
+from mmengine.optim import AmpOptimWrapper, CosineAnnealingLR, LinearLR
+from mmengine.runner import EpochBasedTrainLoop, TestLoop, ValLoop
+from torch.optim import AdamW
+
+from mmaction.datasets import (CenterCrop, DecordDecode, DecordInit, Flip,
+ FormatShape, PackActionInputs,
+ RandomResizedCrop, Resize, SampleFrames,
+ ThreeCrop, VideoDataset)
+from mmaction.engine import SwinOptimWrapperConstructor
+from mmaction.evaluation import AccMetric
+
+model.update(
+ dict(
+ backbone=dict(
+ arch='large',
+ drop_path_rate=0.4,
+ pretrained= # noqa: E251
+ 'https://download.openmmlab.com/mmaction/v1.0/recognition/swin/swin_large_patch4_window7_224_22k.pth' # noqa: E501
+ ),
+ cls_head=dict(in_channels=1536)))
+
+# dataset settings
+dataset_type = VideoDataset
+data_root = 'data/kinetics400/videos_train'
+data_root_val = 'data/kinetics400/videos_val'
+ann_file_train = 'data/kinetics400/kinetics400_train_list_videos.txt'
+ann_file_val = 'data/kinetics400/kinetics400_val_list_videos.txt'
+ann_file_test = 'data/kinetics400/kinetics400_val_list_videos.txt'
+
+file_client_args = dict(io_backend='disk')
+train_pipeline = [
+ dict(type=DecordInit, **file_client_args),
+ dict(type=SampleFrames, clip_len=32, frame_interval=2, num_clips=1),
+ dict(type=DecordDecode),
+ dict(type=Resize, scale=(-1, 256)),
+ dict(type=RandomResizedCrop),
+ dict(type=Resize, scale=(224, 224), keep_ratio=False),
+ dict(type=Flip, flip_ratio=0.5),
+ dict(type=FormatShape, input_format='NCTHW'),
+ dict(type=PackActionInputs)
+]
+val_pipeline = [
+ dict(type=DecordInit, **file_client_args),
+ dict(
+ type=SampleFrames,
+ clip_len=32,
+ frame_interval=2,
+ num_clips=1,
+ test_mode=True),
+ dict(type=DecordDecode),
+ dict(type=Resize, scale=(-1, 256)),
+ dict(type=CenterCrop, crop_size=224),
+ dict(type=FormatShape, input_format='NCTHW'),
+ dict(type=PackActionInputs)
+]
+test_pipeline = [
+ dict(type=DecordInit, **file_client_args),
+ dict(
+ type=SampleFrames,
+ clip_len=32,
+ frame_interval=2,
+ num_clips=4,
+ test_mode=True),
+ dict(type=DecordDecode),
+ dict(type=Resize, scale=(-1, 224)),
+ dict(type=ThreeCrop, crop_size=224),
+ dict(type=FormatShape, input_format='NCTHW'),
+ dict(type=PackActionInputs)
+]
+
+train_dataloader = dict(
+ batch_size=8,
+ num_workers=8,
+ persistent_workers=True,
+ sampler=dict(type=DefaultSampler, shuffle=True),
+ dataset=dict(
+ type=dataset_type,
+ ann_file=ann_file_train,
+ data_prefix=dict(video=data_root),
+ pipeline=train_pipeline))
+val_dataloader = dict(
+ batch_size=8,
+ num_workers=8,
+ persistent_workers=True,
+ sampler=dict(type=DefaultSampler, shuffle=False),
+ dataset=dict(
+ type=dataset_type,
+ ann_file=ann_file_val,
+ data_prefix=dict(video=data_root_val),
+ pipeline=val_pipeline,
+ test_mode=True))
+test_dataloader = dict(
+ batch_size=1,
+ num_workers=8,
+ persistent_workers=True,
+ sampler=dict(type=DefaultSampler, shuffle=False),
+ dataset=dict(
+ type=dataset_type,
+ ann_file=ann_file_test,
+ data_prefix=dict(video=data_root_val),
+ pipeline=test_pipeline,
+ test_mode=True))
+
+val_evaluator = dict(type=AccMetric)
+test_evaluator = val_evaluator
+
+train_cfg = dict(
+ type=EpochBasedTrainLoop, max_epochs=30, val_begin=1, val_interval=3)
+val_cfg = dict(type=ValLoop)
+test_cfg = dict(type=TestLoop)
+
+optim_wrapper = dict(
+ type=AmpOptimWrapper,
+ optimizer=dict(type=AdamW, lr=1e-3, betas=(0.9, 0.999), weight_decay=0.05),
+ constructor=SwinOptimWrapperConstructor,
+ paramwise_cfg=dict(
+ absolute_pos_embed=dict(decay_mult=0.),
+ relative_position_bias_table=dict(decay_mult=0.),
+ norm=dict(decay_mult=0.),
+ backbone=dict(lr_mult=0.1)))
+
+param_scheduler = [
+ dict(
+ type=LinearLR,
+ start_factor=0.1,
+ by_epoch=True,
+ begin=0,
+ end=2.5,
+ convert_to_iter_based=True),
+ dict(
+ type=CosineAnnealingLR,
+ T_max=30,
+ eta_min=0,
+ by_epoch=True,
+ begin=0,
+ end=30)
+]
+
+default_hooks.update(
+ dict(
+ checkpoint=dict(interval=3, max_keep_ckpts=5),
+ logger=dict(interval=100)))
+
+# Default setting for scaling LR automatically
+# - `enable` means enable scaling LR automatically
+# or not by default.
+# - `base_batch_size` = (8 GPUs) x (8 samples per GPU).
+auto_scale_lr = dict(enable=False, base_batch_size=64)
diff --git a/mmaction/configs/recognition/swin/swin_small_p244_w877_in1k_pre_32xb4_amp_32x2x1_30e_kinetics710_rgb.py b/mmaction/configs/recognition/swin/swin_small_p244_w877_in1k_pre_32xb4_amp_32x2x1_30e_kinetics710_rgb.py
new file mode 100644
index 0000000000..a16bca3af6
--- /dev/null
+++ b/mmaction/configs/recognition/swin/swin_small_p244_w877_in1k_pre_32xb4_amp_32x2x1_30e_kinetics710_rgb.py
@@ -0,0 +1,108 @@
+# Copyright (c) OpenMMLab. All rights reserved.
+from mmengine.config import read_base
+
+with read_base():
+ from .swin_small_p244_w877_in1k_pre_8xb8_amp_32x2x1_30e_kinetics400_rgb import * # noqa: E501
+
+from mmengine.dataset import DefaultSampler
+from torch.utils.data import ConcatDataset
+
+model.update(dict(cls_head=dict(num_classes=710)))
+
+k400_data_root = 'data/kinetics400/videos_train'
+k600_data_root = 'data/kinetics600/videos'
+k700_data_root = 'data/kinetics700/videos'
+k400_data_root_val = 'data/kinetics400/videos_val'
+k600_data_root_val = k600_data_root
+k700_data_root_val = k700_data_root
+
+k400_ann_file_train = 'data/kinetics710/k400_train_list_videos.txt'
+k600_ann_file_train = 'data/kinetics710/k600_train_list_videos.txt'
+k700_ann_file_train = 'data/kinetics710/k700_train_list_videos.txt'
+
+k400_ann_file_val = 'data/kinetics710/k400_val_list_videos.txt'
+k600_ann_file_val = 'data/kinetics710/k600_val_list_videos.txt'
+k700_ann_file_val = 'data/kinetics710/k700_val_list_videos.txt'
+
+k400_trainset = dict(
+ type=VideoDataset,
+ ann_file=k400_ann_file_train,
+ data_prefix=dict(video=k400_data_root),
+ pipeline=train_pipeline)
+k600_trainset = dict(
+ type=VideoDataset,
+ ann_file=k600_ann_file_train,
+ data_prefix=dict(video=k600_data_root),
+ pipeline=train_pipeline)
+k700_trainset = dict(
+ type=VideoDataset,
+ ann_file=k700_ann_file_train,
+ data_prefix=dict(video=k700_data_root),
+ pipeline=train_pipeline)
+
+k400_valset = dict(
+ type=VideoDataset,
+ ann_file=k400_ann_file_val,
+ data_prefix=dict(video=k400_data_root_val),
+ pipeline=val_pipeline,
+ test_mode=True)
+k600_valset = dict(
+ type=VideoDataset,
+ ann_file=k600_ann_file_val,
+ data_prefix=dict(video=k600_data_root_val),
+ pipeline=val_pipeline,
+ test_mode=True)
+k700_valset = dict(
+ type=VideoDataset,
+ ann_file=k700_ann_file_val,
+ data_prefix=dict(video=k700_data_root_val),
+ pipeline=val_pipeline,
+ test_mode=True)
+
+k400_testset = k400_valset.copy()
+k600_testset = k600_valset.copy()
+k700_testset = k700_valset.copy()
+k400_testset['pipeline'] = test_pipeline
+k600_testset['pipeline'] = test_pipeline
+k700_testset['pipeline'] = test_pipeline
+
+k710_trainset = dict(
+ type=ConcatDataset,
+ datasets=[k400_trainset, k600_trainset, k700_trainset],
+ _delete_=True)
+k710_valset = dict(
+ type=ConcatDataset,
+ datasets=[k400_valset, k600_valset, k700_valset],
+ _delete_=True)
+k710_testset = dict(
+ type=ConcatDataset,
+ datasets=[k400_testset, k600_testset, k700_testset],
+ _delete_=True,
+)
+
+train_dataloader = dict(
+ batch_size=4,
+ num_workers=8,
+ persistent_workers=True,
+ sampler=dict(type=DefaultSampler, shuffle=True),
+ dataset=k710_trainset)
+val_dataloader = dict(
+ batch_size=4,
+ num_workers=8,
+ persistent_workers=True,
+ sampler=dict(type=DefaultSampler, shuffle=False),
+ dataset=k710_valset)
+test_dataloader = dict(
+ batch_size=1,
+ num_workers=8,
+ persistent_workers=True,
+ sampler=dict(type=DefaultSampler, shuffle=False),
+ dataset=k710_testset)
+
+optim_wrapper.update(dict(optimizer=dict(lr=2e-3)))
+
+# Default setting for scaling LR automatically
+# - `enable` means enable scaling LR automatically
+# or not by default.
+# - `base_batch_size` = (16 GPUs) x (8 samples per GPU).
+auto_scale_lr.update(dict(enable=False, base_batch_size=128))
diff --git a/mmaction/configs/recognition/swin/swin_small_p244_w877_in1k_pre_8xb8_amp_32x2x1_30e_kinetics400_rgb.py b/mmaction/configs/recognition/swin/swin_small_p244_w877_in1k_pre_8xb8_amp_32x2x1_30e_kinetics400_rgb.py
new file mode 100644
index 0000000000..1536ee72f3
--- /dev/null
+++ b/mmaction/configs/recognition/swin/swin_small_p244_w877_in1k_pre_8xb8_amp_32x2x1_30e_kinetics400_rgb.py
@@ -0,0 +1,155 @@
+# Copyright (c) OpenMMLab. All rights reserved.
+from mmengine.config import read_base
+
+with read_base():
+ from ..._base_.models.swin_tiny import *
+ from ..._base_.default_runtime import *
+
+from mmengine.dataset import DefaultSampler
+from mmengine.optim import AmpOptimWrapper, CosineAnnealingLR, LinearLR
+from mmengine.runner import EpochBasedTrainLoop, TestLoop, ValLoop
+from torch.optim import AdamW
+
+from mmaction.datasets import (CenterCrop, DecordDecode, DecordInit, Flip,
+ FormatShape, PackActionInputs,
+ RandomResizedCrop, Resize, SampleFrames,
+ ThreeCrop, VideoDataset)
+from mmaction.engine import SwinOptimWrapperConstructor
+from mmaction.evaluation import AccMetric
+
+model.update(
+ dict(
+ backbone=dict(
+ arch='small',
+ drop_path_rate=0.2,
+ pretrained= # noqa: E251
+ 'https://download.openmmlab.com/mmaction/v1.0/recognition/swin/swin_small_patch4_window7_224.pth' # noqa: E501
+ )))
+
+# dataset settings
+dataset_type = VideoDataset
+data_root = 'data/kinetics400/videos_train'
+data_root_val = 'data/kinetics400/videos_val'
+ann_file_train = 'data/kinetics400/kinetics400_train_list_videos.txt'
+ann_file_val = 'data/kinetics400/kinetics400_val_list_videos.txt'
+ann_file_test = 'data/kinetics400/kinetics400_val_list_videos.txt'
+
+file_client_args = dict(io_backend='disk')
+train_pipeline = [
+ dict(type=DecordInit, **file_client_args),
+ dict(type=SampleFrames, clip_len=32, frame_interval=2, num_clips=1),
+ dict(type=DecordDecode),
+ dict(type=Resize, scale=(-1, 256)),
+ dict(type=RandomResizedCrop),
+ dict(type=Resize, scale=(224, 224), keep_ratio=False),
+ dict(type=Flip, flip_ratio=0.5),
+ dict(type=FormatShape, input_format='NCTHW'),
+ dict(type=PackActionInputs)
+]
+val_pipeline = [
+ dict(type=DecordInit, **file_client_args),
+ dict(
+ type=SampleFrames,
+ clip_len=32,
+ frame_interval=2,
+ num_clips=1,
+ test_mode=True),
+ dict(type=DecordDecode),
+ dict(type=Resize, scale=(-1, 256)),
+ dict(type=CenterCrop, crop_size=224),
+ dict(type=FormatShape, input_format='NCTHW'),
+ dict(type=PackActionInputs)
+]
+test_pipeline = [
+ dict(type=DecordInit, **file_client_args),
+ dict(
+ type=SampleFrames,
+ clip_len=32,
+ frame_interval=2,
+ num_clips=4,
+ test_mode=True),
+ dict(type=DecordDecode),
+ dict(type=Resize, scale=(-1, 224)),
+ dict(type=ThreeCrop, crop_size=224),
+ dict(type=FormatShape, input_format='NCTHW'),
+ dict(type=PackActionInputs)
+]
+
+train_dataloader = dict(
+ batch_size=8,
+ num_workers=8,
+ persistent_workers=True,
+ sampler=dict(type=DefaultSampler, shuffle=True),
+ dataset=dict(
+ type=dataset_type,
+ ann_file=ann_file_train,
+ data_prefix=dict(video=data_root),
+ pipeline=train_pipeline))
+val_dataloader = dict(
+ batch_size=8,
+ num_workers=8,
+ persistent_workers=True,
+ sampler=dict(type=DefaultSampler, shuffle=False),
+ dataset=dict(
+ type=dataset_type,
+ ann_file=ann_file_val,
+ data_prefix=dict(video=data_root_val),
+ pipeline=val_pipeline,
+ test_mode=True))
+test_dataloader = dict(
+ batch_size=1,
+ num_workers=8,
+ persistent_workers=True,
+ sampler=dict(type=DefaultSampler, shuffle=False),
+ dataset=dict(
+ type=dataset_type,
+ ann_file=ann_file_test,
+ data_prefix=dict(video=data_root_val),
+ pipeline=test_pipeline,
+ test_mode=True))
+
+val_evaluator = dict(type=AccMetric)
+test_evaluator = val_evaluator
+
+train_cfg = dict(
+ type=EpochBasedTrainLoop, max_epochs=30, val_begin=1, val_interval=3)
+val_cfg = dict(type=ValLoop)
+test_cfg = dict(type=TestLoop)
+
+optim_wrapper = dict(
+ type=AmpOptimWrapper,
+ optimizer=dict(type=AdamW, lr=1e-3, betas=(0.9, 0.999), weight_decay=0.02),
+ constructor=SwinOptimWrapperConstructor,
+ paramwise_cfg=dict(
+ absolute_pos_embed=dict(decay_mult=0.),
+ relative_position_bias_table=dict(decay_mult=0.),
+ norm=dict(decay_mult=0.),
+ backbone=dict(lr_mult=0.1)))
+
+param_scheduler = [
+ dict(
+ type=LinearLR,
+ start_factor=0.1,
+ by_epoch=True,
+ begin=0,
+ end=2.5,
+ convert_to_iter_based=True),
+ dict(
+ type=CosineAnnealingLR,
+ T_max=30,
+ eta_min=0,
+ by_epoch=True,
+ begin=0,
+ end=30)
+]
+
+default_hooks.update(
+ dict(
+ checkpoint=dict(interval=3, max_keep_ckpts=5),
+ logger=dict(interval=100)))
+
+# Default setting for scaling LR automatically
+# - `enable` means enable scaling LR automatically
+# or not by default.
+# - `base_batch_size` = (8 GPUs) x (8 samples per GPU).
+auto_scale_lr = dict(enable=False, base_batch_size=64)
diff --git a/mmaction/configs/recognition/swin/swin_tiny_p244_w877_in1k_pre_8xb8_amp_32x2x1_30e_kinetics400_rgb.py b/mmaction/configs/recognition/swin/swin_tiny_p244_w877_in1k_pre_8xb8_amp_32x2x1_30e_kinetics400_rgb.py
new file mode 100644
index 0000000000..4d7fa07d55
--- /dev/null
+++ b/mmaction/configs/recognition/swin/swin_tiny_p244_w877_in1k_pre_8xb8_amp_32x2x1_30e_kinetics400_rgb.py
@@ -0,0 +1,153 @@
+# Copyright (c) OpenMMLab. All rights reserved.
+from mmengine.config import read_base
+
+with read_base():
+ from ..._base_.models.swin_tiny import *
+ from ..._base_.default_runtime import *
+
+from mmengine.dataset import DefaultSampler
+from mmengine.optim import AmpOptimWrapper, CosineAnnealingLR, LinearLR
+from mmengine.runner import EpochBasedTrainLoop, TestLoop, ValLoop
+from torch.optim import AdamW
+
+from mmaction.datasets import (CenterCrop, DecordDecode, DecordInit, Flip,
+ FormatShape, PackActionInputs,
+ RandomResizedCrop, Resize, SampleFrames,
+ ThreeCrop, VideoDataset)
+from mmaction.engine import SwinOptimWrapperConstructor
+from mmaction.evaluation import AccMetric
+
+model.update(
+ dict(
+ backbone=dict(
+ pretrained= # noqa: E251
+ 'https://download.openmmlab.com/mmaction/v1.0/recognition/swin/swin_tiny_patch4_window7_224.pth' # noqa: E501
+ )))
+
+# dataset settings
+dataset_type = VideoDataset
+data_root = 'data/kinetics400/videos_train'
+data_root_val = 'data/kinetics400/videos_val'
+ann_file_train = 'data/kinetics400/kinetics400_train_list_videos.txt'
+ann_file_val = 'data/kinetics400/kinetics400_val_list_videos.txt'
+ann_file_test = 'data/kinetics400/kinetics400_val_list_videos.txt'
+
+file_client_args = dict(io_backend='disk')
+train_pipeline = [
+ dict(type=DecordInit, **file_client_args),
+ dict(type=SampleFrames, clip_len=32, frame_interval=2, num_clips=1),
+ dict(type=DecordDecode),
+ dict(type=Resize, scale=(-1, 256)),
+ dict(type=RandomResizedCrop),
+ dict(type=Resize, scale=(224, 224), keep_ratio=False),
+ dict(type=Flip, flip_ratio=0.5),
+ dict(type=FormatShape, input_format='NCTHW'),
+ dict(type=PackActionInputs)
+]
+val_pipeline = [
+ dict(type=DecordInit, **file_client_args),
+ dict(
+ type=SampleFrames,
+ clip_len=32,
+ frame_interval=2,
+ num_clips=1,
+ test_mode=True),
+ dict(type=DecordDecode),
+ dict(type=Resize, scale=(-1, 256)),
+ dict(type=CenterCrop, crop_size=224),
+ dict(type=FormatShape, input_format='NCTHW'),
+ dict(type=PackActionInputs)
+]
+test_pipeline = [
+ dict(type=DecordInit, **file_client_args),
+ dict(
+ type=SampleFrames,
+ clip_len=32,
+ frame_interval=2,
+ num_clips=4,
+ test_mode=True),
+ dict(type=DecordDecode),
+ dict(type=Resize, scale=(-1, 224)),
+ dict(type=ThreeCrop, crop_size=224),
+ dict(type=FormatShape, input_format='NCTHW'),
+ dict(type=PackActionInputs)
+]
+
+train_dataloader = dict(
+ batch_size=8,
+ num_workers=8,
+ persistent_workers=True,
+ sampler=dict(type=DefaultSampler, shuffle=True),
+ dataset=dict(
+ type=dataset_type,
+ ann_file=ann_file_train,
+ data_prefix=dict(video=data_root),
+ pipeline=train_pipeline))
+val_dataloader = dict(
+ batch_size=8,
+ num_workers=8,
+ persistent_workers=True,
+ sampler=dict(type=DefaultSampler, shuffle=False),
+ dataset=dict(
+ type=dataset_type,
+ ann_file=ann_file_val,
+ data_prefix=dict(video=data_root_val),
+ pipeline=val_pipeline,
+ test_mode=True))
+test_dataloader = dict(
+ batch_size=1,
+ num_workers=8,
+ persistent_workers=True,
+ sampler=dict(type=DefaultSampler, shuffle=False),
+ dataset=dict(
+ type=dataset_type,
+ ann_file=ann_file_test,
+ data_prefix=dict(video=data_root_val),
+ pipeline=test_pipeline,
+ test_mode=True))
+
+val_evaluator = dict(type=AccMetric)
+test_evaluator = val_evaluator
+
+train_cfg = dict(
+ type=EpochBasedTrainLoop, max_epochs=30, val_begin=1, val_interval=3)
+val_cfg = dict(type=ValLoop)
+test_cfg = dict(type=TestLoop)
+
+optim_wrapper = dict(
+ type=AmpOptimWrapper,
+ optimizer=dict(type=AdamW, lr=1e-3, betas=(0.9, 0.999), weight_decay=0.02),
+ constructor=SwinOptimWrapperConstructor,
+ paramwise_cfg=dict(
+ absolute_pos_embed=dict(decay_mult=0.),
+ relative_position_bias_table=dict(decay_mult=0.),
+ norm=dict(decay_mult=0.),
+ backbone=dict(lr_mult=0.1)))
+
+param_scheduler = [
+ dict(
+ type=LinearLR,
+ start_factor=0.1,
+ by_epoch=True,
+ begin=0,
+ end=2.5,
+ convert_to_iter_based=True),
+ dict(
+ type=CosineAnnealingLR,
+ T_max=30,
+ eta_min=0,
+ by_epoch=True,
+ begin=0,
+ end=30)
+]
+
+default_hooks.update(
+ dict(
+ checkpoint=dict(interval=3, max_keep_ckpts=5),
+ logger=dict(interval=100)))
+
+# Default setting for scaling LR automatically
+# - `enable` means enable scaling LR automatically
+# or not by default.
+# - `base_batch_size` = (8 GPUs) x (8 samples per GPU).
+auto_scale_lr = dict(enable=False, base_batch_size=64)
diff --git a/mmaction/datasets/__init__.py b/mmaction/datasets/__init__.py
index ded946b727..eef565309d 100644
--- a/mmaction/datasets/__init__.py
+++ b/mmaction/datasets/__init__.py
@@ -3,6 +3,8 @@
from .audio_dataset import AudioDataset
from .ava_dataset import AVADataset, AVAKineticsDataset
from .base import BaseActionDataset
+from .charades_sta_dataset import CharadesSTADataset
+from .msrvtt_datasets import MSRVTTVQA, MSRVTTVQAMC, MSRVTTRetrieval
from .pose_dataset import PoseDataset
from .rawframe_dataset import RawframeDataset
from .repeat_aug_dataset import RepeatAugDataset, repeat_pseudo_collate
@@ -13,5 +15,6 @@
__all__ = [
'AVADataset', 'AVAKineticsDataset', 'ActivityNetDataset', 'AudioDataset',
'BaseActionDataset', 'PoseDataset', 'RawframeDataset', 'RepeatAugDataset',
- 'VideoDataset', 'repeat_pseudo_collate', 'VideoTextDataset'
+ 'VideoDataset', 'repeat_pseudo_collate', 'VideoTextDataset',
+ 'MSRVTTRetrieval', 'MSRVTTVQA', 'MSRVTTVQAMC', 'CharadesSTADataset'
]
diff --git a/mmaction/datasets/audio_dataset.py b/mmaction/datasets/audio_dataset.py
index 42c98fb091..07aae25143 100644
--- a/mmaction/datasets/audio_dataset.py
+++ b/mmaction/datasets/audio_dataset.py
@@ -1,27 +1,21 @@
# Copyright (c) OpenMMLab. All rights reserved.
import os.path as osp
-from typing import Callable, List, Optional, Union
+from typing import Callable, Dict, List, Optional, Union
-import torch
from mmengine.utils import check_file_exist
from mmaction.registry import DATASETS
-from mmaction.utils import ConfigType
from .base import BaseActionDataset
@DATASETS.register_module()
class AudioDataset(BaseActionDataset):
- """Audio dataset for action recognition. Annotation file can be that of the
- rawframe dataset, or:
+ """Audio dataset for action recognition.
- .. code-block:: txt
- some/directory-1.wav 163 1
- some/directory-2.wav 122 1
- some/directory-3.wav 258 2
- some/directory-4.wav 234 2
- some/directory-5.wav 295 3
- some/directory-6.wav 121 3
+ The ann_file is a text file with multiple lines, and each line indicates
+ a sample audio or extracted audio feature with the filepath, total frames
+ of the raw video and label, which are split with a whitespace.
+ Example of a annotation file:
.. code-block:: txt
some/directory-1.npy 163 1
@@ -33,26 +27,22 @@ class AudioDataset(BaseActionDataset):
Args:
ann_file (str): Path to the annotation file.
- pipeline (List[Union[dict, ConfigDict, Callable]]): A sequence of
- data transforms.
- data_prefix (dict or ConfigDict, optional): Path to a directory where
+ pipeline (list[dict | callable]): A sequence of data transforms.
+ data_prefix (dict): Path to a directory where
audios are held. Defaults to ``dict(audio='')``.
multi_class (bool): Determines whether it is a multi-class
recognition dataset. Defaults to False.
num_classes (int, optional): Number of classes in the dataset.
Defaults to None.
- suffix (str): The suffix of the audio file. Defaults to ``.wav``.
"""
def __init__(self,
ann_file: str,
- pipeline: List[Union[ConfigType, Callable]],
- data_prefix: ConfigType = dict(audio=''),
+ pipeline: List[Union[Dict, Callable]],
+ data_prefix: Dict = dict(audio=''),
multi_class: bool = False,
num_classes: Optional[int] = None,
- suffix: str = '.wav',
**kwargs) -> None:
- self.suffix = suffix
super().__init__(
ann_file,
pipeline,
@@ -62,8 +52,8 @@ def __init__(self,
modality='Audio',
**kwargs)
- def load_data_list(self) -> List[dict]:
- """Load annotation file to get video information."""
+ def load_data_list(self) -> List[Dict]:
+ """Load annotation file to get audio information."""
check_file_exist(self.ann_file)
data_list = []
with open(self.ann_file, 'r') as fin:
@@ -73,25 +63,18 @@ def load_data_list(self) -> List[dict]:
idx = 0
filename = line_split[idx]
if self.data_prefix['audio'] is not None:
- if not filename.endswith(self.suffix):
- filename = osp.join(self.data_prefix['audio'],
- filename + self.suffix)
- else:
- filename = osp.join(self.data_prefix['audio'],
- filename)
+ filename = osp.join(self.data_prefix['audio'], filename)
video_info['audio_path'] = filename
idx += 1
# idx for total_frames
video_info['total_frames'] = int(line_split[idx])
idx += 1
- # idx for label[s]
+ # idx for label
label = [int(x) for x in line_split[idx:]]
assert label, f'missing label in line: {line}'
if self.multi_class:
assert self.num_classes is not None
- onehot = torch.zeros(self.num_classes)
- onehot[label] = 1.0
- video_info['label'] = onehot
+ video_info['label'] = label
else:
assert len(label) == 1
video_info['label'] = label[0]
diff --git a/mmaction/datasets/ava_dataset.py b/mmaction/datasets/ava_dataset.py
index e744dc9f5e..c1ba4a6b41 100644
--- a/mmaction/datasets/ava_dataset.py
+++ b/mmaction/datasets/ava_dataset.py
@@ -203,7 +203,6 @@ def parse_img_record(self, img_records: List[dict]) -> tuple:
labels.append(label)
entity_ids.append(img_record['entity_id'])
-
bboxes = np.stack(bboxes)
labels = np.stack(labels)
entity_ids = np.stack(entity_ids)
diff --git a/mmaction/datasets/charades_sta_dataset.py b/mmaction/datasets/charades_sta_dataset.py
new file mode 100644
index 0000000000..aca9c9a6bb
--- /dev/null
+++ b/mmaction/datasets/charades_sta_dataset.py
@@ -0,0 +1,124 @@
+# Copyright (c) OpenMMLab. All rights reserved.
+import os
+from typing import Callable, List, Optional, Union
+
+import mmengine
+import numpy as np
+import torch
+from mmengine.fileio import exists
+
+from mmaction.registry import DATASETS
+from mmaction.utils import ConfigType
+from .base import BaseActionDataset
+
+try:
+ import nltk
+ nltk_imported = True
+except ImportError:
+ nltk_imported = False
+
+
+@DATASETS.register_module()
+class CharadesSTADataset(BaseActionDataset):
+
+ def __init__(self,
+ ann_file: str,
+ pipeline: List[Union[dict, Callable]],
+ word2id_file: str,
+ fps_file: str,
+ duration_file: str,
+ num_frames_file: str,
+ window_size: int,
+ ft_overlap: float,
+ data_prefix: Optional[ConfigType] = dict(video=''),
+ test_mode: bool = False,
+ **kwargs):
+ if not nltk_imported:
+ raise ImportError('nltk is required for CharadesSTADataset')
+
+ self.fps_info = mmengine.load(fps_file)
+ self.duration_info = mmengine.load(duration_file)
+ self.num_frames = mmengine.load(num_frames_file)
+ self.word2id = mmengine.load(word2id_file)
+ self.ft_interval = int(window_size * (1 - ft_overlap))
+
+ super().__init__(
+ ann_file,
+ pipeline=pipeline,
+ data_prefix=data_prefix,
+ test_mode=test_mode,
+ **kwargs)
+
+ def load_data_list(self) -> List[dict]:
+ """Load annotation file to get video information."""
+ exists(self.ann_file)
+ data_list = []
+ with open(self.ann_file) as f:
+ anno_database = f.readlines()
+
+ for item in anno_database:
+ first_part, query_sentence = item.strip().split('##')
+ query_sentence = query_sentence.replace('.', '')
+ query_words = nltk.word_tokenize(query_sentence)
+ query_tokens = [self.word2id[word] for word in query_words]
+ query_length = len(query_tokens)
+ query_tokens = torch.from_numpy(np.array(query_tokens))
+
+ vid_name, start_time, end_time = first_part.split()
+ duration = float(self.duration_info[vid_name])
+ fps = float(self.fps_info[vid_name])
+
+ gt_start_time = float(start_time)
+ gt_end_time = float(end_time)
+
+ gt_bbox = (gt_start_time / duration, min(gt_end_time / duration,
+ 1))
+
+ num_frames = int(self.num_frames[vid_name])
+ proposal_frames = self.get_proposals(num_frames)
+
+ proposals = proposal_frames / num_frames
+ proposals = torch.from_numpy(proposals)
+ proposal_indexes = proposal_frames / self.ft_interval
+ proposal_indexes = proposal_indexes.astype(np.int32)
+
+ info = dict(
+ vid_name=vid_name,
+ fps=fps,
+ num_frames=num_frames,
+ duration=duration,
+ query_tokens=query_tokens,
+ query_length=query_length,
+ gt_start_time=gt_start_time,
+ gt_end_time=gt_end_time,
+ gt_bbox=gt_bbox,
+ proposals=proposals,
+ num_proposals=proposals.shape[0],
+ proposal_indexes=proposal_indexes)
+ data_list.append(info)
+ return data_list
+
+ def get_proposals(self, num_frames):
+ proposals = (num_frames - 1) / 32 * np.arange(33)
+ proposals = proposals.astype(np.int32)
+ proposals = np.stack([proposals[:-1], proposals[1:]]).T
+ return proposals
+
+ def get_data_info(self, idx: int) -> dict:
+ """Get annotation by index."""
+ data_info = super().get_data_info(idx)
+ vid_name = data_info['vid_name']
+ feature_path = os.path.join(self.data_prefix['video'],
+ f'{vid_name}.pt')
+ vid_feature = torch.load(feature_path)
+ proposal_feats = []
+ proposal_indexes = data_info['proposal_indexes'].clip(
+ max=vid_feature.shape[0] - 1)
+ for s, e in proposal_indexes:
+ prop_feature, _ = vid_feature[s:e + 1].max(dim=0)
+ proposal_feats.append(prop_feature)
+
+ proposal_feats = torch.stack(proposal_feats)
+
+ data_info['raw_feature'] = proposal_feats
+ return data_info
diff --git a/mmaction/datasets/msrvtt_datasets.py b/mmaction/datasets/msrvtt_datasets.py
new file mode 100644
index 0000000000..058734c01d
--- /dev/null
+++ b/mmaction/datasets/msrvtt_datasets.py
@@ -0,0 +1,116 @@
+# Copyright (c) OpenMMLab. All rights reserved.
+import json
+import os.path as osp
+import re
+from collections import Counter
+from typing import Dict, List
+
+from mmengine.fileio import exists
+
+from mmaction.registry import DATASETS
+from .base import BaseActionDataset
+
+
+@DATASETS.register_module()
+class MSRVTTVQA(BaseActionDataset):
+ """MSR-VTT Video Question Answering dataset."""
+
+ def load_data_list(self) -> List[Dict]:
+ """Load annotation file to get video information."""
+ exists(self.ann_file)
+ data_list = []
+
+ with open(self.ann_file) as f:
+ data_lines = json.load(f)
+ for data in data_lines:
+ answers = data['answer']
+ if isinstance(answers, str):
+ answers = [answers]
+ count = Counter(answers)
+ answer_weight = [i / len(answers) for i in count.values()]
+ data_item = dict(
+ question_id=data['question_id'],
+ filename=osp.join(self.data_prefix['video'],
+ data['video']),
+ question=pre_text(data['question']),
+ gt_answer=list(count.keys()),
+ gt_answer_weight=answer_weight)
+ data_list.append(data_item)
+
+ return data_list
+
+
+@DATASETS.register_module()
+class MSRVTTVQAMC(BaseActionDataset):
+ """MSR-VTT VQA multiple choices dataset."""
+
+ def load_data_list(self) -> List[Dict]:
+ """Load annotation file to get video information."""
+ exists(self.ann_file)
+ data_list = []
+
+ with open(self.ann_file) as f:
+ data_lines = json.load(f)
+ for data in data_lines:
+ data_item = dict(
+ filename=osp.join(self.data_prefix['video'],
+ data['video']),
+ label=data['answer'],
+ caption_options=[pre_text(c) for c in data['caption']])
+ data_list.append(data_item)
+
+ return data_list
+
+
+@DATASETS.register_module()
+class MSRVTTRetrieval(BaseActionDataset):
+ """MSR-VTT Retrieval dataset."""
+
+ def load_data_list(self) -> List[Dict]:
+ """Load annotation file to get video information."""
+ exists(self.ann_file)
+ data_list = []
+
+ with open(self.ann_file) as f:
+ data_lines = json.load(f)
+ video_idx = 0
+ text_idx = 0
+ for data in data_lines:
+ # don't consider multiple videos or multiple captions
+ video_path = osp.join(self.data_prefix['video'], data['video'])
+ data_item = dict(
+ filename=video_path,
+ text=[],
+ gt_video_id=[],
+ gt_text_id=[])
+ if isinstance(data['caption'], str):
+ data['caption'] = [data['caption']]
+
+ for text in data['caption']:
+ text = pre_text(text)
+ data_item['text'].append(text)
+ data_item['gt_video_id'].append(video_idx)
+ data_item['gt_text_id'].append(text_idx)
+ text_idx += 1
+
+ video_idx += 1
+ data_list.append(data_item)
+ self.num_videos = video_idx
+ self.num_texts = text_idx
+
+ return data_list
+
+
+def pre_text(text, max_l=None):
+ text = re.sub(r"([,.'!?\"()*#:;~])", '', text.lower())
+ text = text.replace('-', ' ').replace('/',
+ ' ').replace('', 'person')
+
+ text = re.sub(r'\s{2,}', ' ', text)
+ text = text.rstrip('\n').strip(' ')
+
+ if max_l: # truncate
+ words = text.split(' ')
+ if len(words) > max_l:
+ text = ' '.join(words[:max_l])
+ return text
diff --git a/mmaction/datasets/pose_dataset.py b/mmaction/datasets/pose_dataset.py
index a06a7f7c0d..ef2de64bb9 100644
--- a/mmaction/datasets/pose_dataset.py
+++ b/mmaction/datasets/pose_dataset.py
@@ -3,6 +3,7 @@
from typing import Callable, Dict, List, Optional, Union
import mmengine
+from mmengine.logging import MMLogger
from mmaction.registry import DATASETS
from .base import BaseActionDataset
@@ -29,14 +30,29 @@ class PoseDataset(BaseActionDataset):
For NTURGB+D 120, allowed choices are 'xsub_train',
'xsub_val', 'xset_train', 'xset_val'. For FineGYM,
allowed choices are 'train', 'val'. Defaults to None.
+ valid_ratio (float, optional): The valid_ratio for videos in
+ KineticsPose. For a video with n frames, it is a valid
+ training sample only if n * valid_ratio frames have human
+ pose. None means not applicable (only applicable to Kinetics
+ Pose).Defaults to None.
+ box_thr (float): The threshold for human proposals. Only boxes
+ with confidence score larger than `box_thr` is kept. None
+ means not applicable (only applicable to Kinetics). Allowed
+ choices are 0.5, 0.6, 0.7, 0.8, 0.9. Defaults to 0.5.
"""
def __init__(self,
ann_file: str,
pipeline: List[Union[Dict, Callable]],
split: Optional[str] = None,
+ valid_ratio: Optional[float] = None,
+ box_thr: float = 0.5,
**kwargs) -> None:
self.split = split
+ self.box_thr = box_thr
+ assert box_thr in [.5, .6, .7, .8, .9]
+ self.valid_ratio = valid_ratio
+
super().__init__(
ann_file, pipeline=pipeline, modality='Pose', **kwargs)
@@ -62,3 +78,41 @@ def load_data_list(self) -> List[Dict]:
item['frame_dir'] = osp.join(self.data_prefix['video'],
item['frame_dir'])
return data_list
+
+ def filter_data(self) -> List[Dict]:
+ """Filter out invalid samples."""
+ if self.valid_ratio is not None and isinstance(
+ self.valid_ratio, float) and self.valid_ratio > 0:
+ self.data_list = [
+ x for x in self.data_list if x['valid'][self.box_thr] /
+ x['total_frames'] >= self.valid_ratio
+ ]
+ for item in self.data_list:
+ assert 'box_score' in item,\
+ 'if valid_ratio is a positive number,' \
+ 'item should have field `box_score`'
+ anno_inds = (item['box_score'] >= self.box_thr)
+ item['anno_inds'] = anno_inds
+
+ logger = MMLogger.get_current_instance()
+ logger.info(
+ f'{len(self.data_list)} videos remain after valid thresholding')
+
+ return self.data_list
+
+ def get_data_info(self, idx: int) -> Dict:
+ """Get annotation by index."""
+ data_info = super().get_data_info(idx)
+
+ # Sometimes we may need to load skeleton from the file
+ if 'skeleton' in self.data_prefix:
+ identifier = 'filename' if 'filename' in data_info \
+ else 'frame_dir'
+ ske_name = data_info[identifier]
+ ske_path = osp.join(self.data_prefix['skeleton'],
+ ske_name + '.pkl')
+ ske = mmengine.load(ske_path)
+ for k in ske:
+ data_info[k] = ske[k]
+
+ return data_info
diff --git a/mmaction/datasets/transforms/__init__.py b/mmaction/datasets/transforms/__init__.py
index d8b8cc4eb3..3d1ee91e27 100644
--- a/mmaction/datasets/transforms/__init__.py
+++ b/mmaction/datasets/transforms/__init__.py
@@ -1,43 +1,41 @@
# Copyright (c) OpenMMLab. All rights reserved.
from .formatting import (FormatAudioShape, FormatGCNInput, FormatShape,
PackActionInputs, PackLocalizationInputs, Transpose)
-from .loading import (ArrayDecode, AudioDecode, AudioDecodeInit,
- AudioFeatureSelector, BuildPseudoClip, DecordDecode,
- DecordInit, DenseSampleFrames,
+from .loading import (ArrayDecode, AudioFeatureSelector, BuildPseudoClip,
+ DecordDecode, DecordInit, DenseSampleFrames,
GenerateLocalizationLabels, ImageDecode,
LoadAudioFeature, LoadHVULabel, LoadLocalizationFeature,
LoadProposals, LoadRGBFromFile, OpenCVDecode, OpenCVInit,
PIMSDecode, PIMSInit, PyAVDecode, PyAVDecodeMotionVector,
PyAVInit, RawFrameDecode, SampleAVAFrames, SampleFrames,
UniformSample, UntrimmedSampleFrames)
-from .pose_transforms import (GeneratePoseTarget, GenSkeFeat, JointToBone,
- LoadKineticsPose, MergeSkeFeat, MMCompact,
- MMDecode, MMUniformSampleFrames, PadTo,
- PoseCompact, PoseDecode, PreNormalize2D,
- PreNormalize3D, ToMotion, UniformSampleFrames)
-from .processing import (AudioAmplify, CenterCrop, ColorJitter, Flip, Fuse,
- MelSpectrogram, MultiScaleCrop, RandomCrop,
- RandomRescale, RandomResizedCrop, Resize, TenCrop,
- ThreeCrop)
+from .pose_transforms import (DecompressPose, GeneratePoseTarget, GenSkeFeat,
+ JointToBone, MergeSkeFeat, MMCompact, MMDecode,
+ MMUniformSampleFrames, PadTo, PoseCompact,
+ PoseDecode, PreNormalize2D, PreNormalize3D,
+ ToMotion, UniformSampleFrames)
+from .processing import (CenterCrop, ColorJitter, Flip, Fuse, MultiScaleCrop,
+ RandomCrop, RandomRescale, RandomResizedCrop, Resize,
+ TenCrop, ThreeCrop)
from .text_transforms import CLIPTokenize
from .wrappers import ImgAug, PytorchVideoWrapper, TorchVisionWrapper
__all__ = [
- 'ArrayDecode', 'AudioAmplify', 'AudioDecode', 'AudioDecodeInit',
- 'AudioFeatureSelector', 'BuildPseudoClip', 'CenterCrop', 'ColorJitter',
- 'DecordDecode', 'DecordInit', 'DecordInit', 'DenseSampleFrames', 'Flip',
- 'FormatAudioShape', 'FormatGCNInput', 'FormatShape', 'Fuse', 'GenSkeFeat',
- 'GenerateLocalizationLabels', 'GeneratePoseTarget', 'ImageDecode',
- 'ImgAug', 'JointToBone', 'LoadAudioFeature', 'LoadHVULabel',
- 'LoadKineticsPose', 'LoadLocalizationFeature', 'LoadProposals',
- 'LoadRGBFromFile', 'MelSpectrogram', 'MergeSkeFeat', 'MultiScaleCrop',
- 'OpenCVDecode', 'OpenCVInit', 'OpenCVInit', 'PIMSDecode', 'PIMSInit',
- 'PackActionInputs', 'PackLocalizationInputs', 'PadTo', 'PoseCompact',
- 'PoseDecode', 'PreNormalize2D', 'PreNormalize3D', 'PyAVDecode',
- 'PyAVDecodeMotionVector', 'PyAVInit', 'PyAVInit', 'PytorchVideoWrapper',
- 'RandomCrop', 'RandomRescale', 'RandomResizedCrop', 'RawFrameDecode',
- 'Resize', 'SampleAVAFrames', 'SampleFrames', 'TenCrop', 'ThreeCrop',
- 'ToMotion', 'TorchVisionWrapper', 'Transpose', 'UniformSample',
- 'UniformSampleFrames', 'UntrimmedSampleFrames', 'MMUniformSampleFrames',
- 'MMDecode', 'MMCompact', 'CLIPTokenize'
+ 'ArrayDecode', 'AudioFeatureSelector', 'BuildPseudoClip', 'CenterCrop',
+ 'ColorJitter', 'DecordDecode', 'DecordInit', 'DecordInit',
+ 'DenseSampleFrames', 'Flip', 'FormatAudioShape', 'FormatGCNInput',
+ 'FormatShape', 'Fuse', 'GenSkeFeat', 'GenerateLocalizationLabels',
+ 'GeneratePoseTarget', 'ImageDecode', 'ImgAug', 'JointToBone',
+ 'LoadAudioFeature', 'LoadHVULabel', 'DecompressPose',
+ 'LoadLocalizationFeature', 'LoadProposals', 'LoadRGBFromFile',
+ 'MergeSkeFeat', 'MultiScaleCrop', 'OpenCVDecode', 'OpenCVInit',
+ 'OpenCVInit', 'PIMSDecode', 'PIMSInit', 'PackActionInputs',
+ 'PackLocalizationInputs', 'PadTo', 'PoseCompact', 'PoseDecode',
+ 'PreNormalize2D', 'PreNormalize3D', 'PyAVDecode', 'PyAVDecodeMotionVector',
+ 'PyAVInit', 'PyAVInit', 'PytorchVideoWrapper', 'RandomCrop',
+ 'RandomRescale', 'RandomResizedCrop', 'RawFrameDecode', 'Resize',
+ 'SampleAVAFrames', 'SampleFrames', 'TenCrop', 'ThreeCrop', 'ToMotion',
+ 'TorchVisionWrapper', 'Transpose', 'UniformSample', 'UniformSampleFrames',
+ 'UntrimmedSampleFrames', 'MMUniformSampleFrames', 'MMDecode', 'MMCompact',
+ 'CLIPTokenize'
]
diff --git a/mmaction/datasets/transforms/formatting.py b/mmaction/datasets/transforms/formatting.py
index 6ca61a4ccc..0ae1475c8b 100644
--- a/mmaction/datasets/transforms/formatting.py
+++ b/mmaction/datasets/transforms/formatting.py
@@ -4,7 +4,7 @@
import numpy as np
import torch
from mmcv.transforms import BaseTransform, to_tensor
-from mmengine.structures import InstanceData, LabelData
+from mmengine.structures import InstanceData
from mmaction.registry import TRANSFORMS
from mmaction.structures import ActionDataSample
@@ -12,23 +12,16 @@
@TRANSFORMS.register_module()
class PackActionInputs(BaseTransform):
- """Pack the input data for the recognition.
-
- PackActionInputs first packs one of 'imgs', 'keypoint' and 'audios' into
- the `packed_results['inputs']`, which are the three basic input modalities
- for the task of rgb-based, skeleton-based and audio-based action
- recognition, as well as spatio-temporal action detection in the case
- of 'img'. Next, it prepares a `data_sample` for the task of action
- recognition (only a single label of `torch.LongTensor` format, which is
- saved in the `data_sample.gt_labels.item`) or spatio-temporal action
- detection respectively. Then, it saves the meta keys defined in
- the `meta_keys` in `data_sample.metainfo`, and packs the `data_sample`
- into the `packed_results['data_samples']`.
+ """Pack the inputs data.
Args:
+ collect_keys (tuple[str], optional): The keys to be collected
+ to ``packed_results['inputs']``. Defaults to ``
meta_keys (Sequence[str]): The meta keys to saved in the
`metainfo` of the `data_sample`.
Defaults to ``('img_shape', 'img_key', 'video_id', 'timestamp')``.
+ algorithm_keys (Sequence[str]): The keys of custom elements to be used
+ in the algorithm. Defaults to an empty tuple.
"""
mapping_table = {
@@ -37,13 +30,15 @@ class PackActionInputs(BaseTransform):
}
def __init__(
- self,
- collect_keys: Optional[Tuple[str]] = None,
- meta_keys: Sequence[str] = ('img_shape', 'img_key', 'video_id',
- 'timestamp')
+ self,
+ collect_keys: Optional[Tuple[str]] = None,
+ meta_keys: Sequence[str] = ('img_shape', 'img_key', 'video_id',
+ 'timestamp'),
+ algorithm_keys: Sequence[str] = (),
) -> None:
self.collect_keys = collect_keys
self.meta_keys = meta_keys
+ self.algorithm_keys = algorithm_keys
def transform(self, results: Dict) -> Dict:
"""The transform function of :class:`PackActionInputs`.
@@ -95,10 +90,14 @@ def transform(self, results: Dict) -> Dict:
bboxes=to_tensor(results['proposals']))
if 'label' in results:
- label_data = LabelData()
- label_data.item = to_tensor(results['label'])
- data_sample.gt_labels = label_data
+ data_sample.set_gt_label(results['label'])
+ # Set custom algorithm keys
+ for key in self.algorithm_keys:
+ if key in results:
+ data_sample.set_field(results[key], key)
+
+ # Set meta keys
img_meta = {k: results[k] for k in self.meta_keys if k in results}
data_sample.set_metainfo(img_meta)
packed_results['data_samples'] = data_sample
@@ -146,18 +145,17 @@ def transform(self, results):
for key in self.keys:
if key not in results:
continue
- if key == 'gt_bbox':
- instance_data = InstanceData()
- instance_data[key] = to_tensor(results[key])
- data_sample.gt_instances = instance_data
elif key == 'proposals':
instance_data = InstanceData()
instance_data[key] = to_tensor(results[key])
data_sample.proposals = instance_data
else:
- raise NotImplementedError(
- f"Key '{key}' is not supported in `PackLocalizationInputs`"
- )
+ if hasattr(data_sample, 'gt_instances'):
+ data_sample.gt_instances[key] = to_tensor(results[key])
+ else:
+ instance_data = InstanceData()
+ instance_data[key] = to_tensor(results[key])
+ data_sample.gt_instances = instance_data
img_meta = {k: results[k] for k in self.meta_keys if k in results}
data_sample.set_metainfo(img_meta)
@@ -204,16 +202,20 @@ class FormatShape(BaseTransform):
"""Format final imgs shape to the given input_format.
Required keys:
+
- imgs (optional)
- heatmap_imgs (optional)
+ - modality (optional)
- num_clips
- clip_len
Modified Keys:
- - imgs (optional)
- - input_shape (optional)
+
+ - imgs
Added Keys:
+
+ - input_shape
- heatmap_input_shape (optional)
Args:
@@ -227,7 +229,7 @@ def __init__(self, input_format: str, collapse: bool = False) -> None:
self.input_format = input_format
self.collapse = collapse
if self.input_format not in [
- 'NCTHW', 'NCHW', 'NCHW_Flow', 'NCTHW_Heatmap', 'NPTCHW'
+ 'NCTHW', 'NCHW', 'NCTHW_Heatmap', 'NPTCHW'
]:
raise ValueError(
f'The input format {self.input_format} is invalid.')
@@ -300,36 +302,14 @@ def transform(self, results: Dict) -> Dict:
elif self.input_format == 'NCHW':
imgs = results['imgs']
imgs = np.transpose(imgs, (0, 3, 1, 2))
+ if 'modality' in results and results['modality'] == 'Flow':
+ clip_len = results['clip_len']
+ imgs = imgs.reshape((-1, clip_len * imgs.shape[1]) +
+ imgs.shape[2:])
# M x C x H x W
results['imgs'] = imgs
results['input_shape'] = imgs.shape
- elif self.input_format == 'NCHW_Flow':
- num_imgs = len(results['imgs'])
- assert num_imgs % 2 == 0
- n = num_imgs // 2
- h, w = results['imgs'][0].shape
- x_flow = np.empty((n, h, w), dtype=np.float32)
- y_flow = np.empty((n, h, w), dtype=np.float32)
- for i in range(n):
- x_flow[i] = results['imgs'][2 * i]
- y_flow[i] = results['imgs'][2 * i + 1]
- imgs = np.stack([x_flow, y_flow], axis=-1)
-
- num_clips = results['num_clips']
- clip_len = results['clip_len']
- imgs = imgs.reshape((-1, num_clips, clip_len) + imgs.shape[1:])
- # N_crops x N_clips x T x H x W x C
- imgs = np.transpose(imgs, (0, 1, 2, 5, 3, 4))
- # N_crops x N_clips x T x C x H x W
- imgs = imgs.reshape((-1, imgs.shape[2] * imgs.shape[3]) +
- imgs.shape[4:])
- # M' x C' x H x W
- # M' = N_crops x N_clips
- # C' = T x C
- results['imgs'] = imgs
- results['input_shape'] = imgs.shape
-
elif self.input_format == 'NPTCHW':
num_proposals = results['num_proposals']
num_clips = results['num_clips']
@@ -361,8 +341,17 @@ def __repr__(self) -> str:
class FormatAudioShape(BaseTransform):
"""Format final audio shape to the given input_format.
- Required keys are ``audios``, ``num_clips`` and ``clip_len``, added or
- modified keys are ``audios`` and ``input_shape``.
+ Required Keys:
+
+ - audios
+
+ Modified Keys:
+
+ - audios
+
+ Added Keys:
+
+ - input_shape
Args:
input_format (str): Define the final imgs format.
@@ -374,7 +363,7 @@ def __init__(self, input_format: str) -> None:
raise ValueError(
f'The input format {self.input_format} is invalid.')
- def transform(self, results: dict) -> dict:
+ def transform(self, results: Dict) -> Dict:
"""Performs the FormatShape formatting.
Args:
@@ -389,7 +378,7 @@ def transform(self, results: dict) -> dict:
results['input_shape'] = audios.shape
return results
- def __repr__(self):
+ def __repr__(self) -> str:
repr_str = self.__class__.__name__
repr_str += f"(input_format='{self.input_format}')"
return repr_str
diff --git a/mmaction/datasets/transforms/loading.py b/mmaction/datasets/transforms/loading.py
index e876143cd3..8d789ab4c3 100644
--- a/mmaction/datasets/transforms/loading.py
+++ b/mmaction/datasets/transforms/loading.py
@@ -1418,11 +1418,7 @@ def transform(self, results: dict) -> dict:
for i, frame_idx in enumerate(results['frame_inds']):
# Avoid loading duplicated frames
if frame_idx in cache:
- if modality == 'RGB':
- imgs.append(cp.deepcopy(imgs[cache[frame_idx]]))
- else:
- imgs.append(cp.deepcopy(imgs[2 * cache[frame_idx]]))
- imgs.append(cp.deepcopy(imgs[2 * cache[frame_idx] + 1]))
+ imgs.append(cp.deepcopy(imgs[cache[frame_idx]]))
continue
else:
cache[frame_idx] = i
@@ -1443,7 +1439,7 @@ def transform(self, results: dict) -> dict:
x_frame = mmcv.imfrombytes(x_img_bytes, flag='grayscale')
y_img_bytes = self.file_client.get(y_filepath)
y_frame = mmcv.imfrombytes(y_img_bytes, flag='grayscale')
- imgs.extend([x_frame, y_frame])
+ imgs.append(np.stack([x_frame, y_frame], axis=-1))
else:
raise NotImplementedError
@@ -1621,105 +1617,39 @@ def transform(self, results):
@TRANSFORMS.register_module()
-class AudioDecodeInit(BaseTransform):
- """Using librosa to initialize the audio reader.
-
- Required keys are ``audio_path``, added or modified keys are ``length``,
- ``sample_rate``, ``audios``.
-
- Args:
- io_backend (str): io backend where frames are store.
- Defaults to ``disk``.
- sample_rate (int): Audio sampling times per second. Defaults to 16000.
- pad_method (str): Padding method. Defaults to ``zero``.
- """
-
- def __init__(self,
- io_backend: str = 'disk',
- sample_rate: int = 16000,
- pad_method: str = 'zero',
- **kwargs) -> None:
- self.io_backend = io_backend
- self.sample_rate = sample_rate
- if pad_method in ['random', 'zero']:
- self.pad_method = pad_method
- else:
- raise NotImplementedError
- self.kwargs = kwargs
- self.file_client = None
-
- @staticmethod
- def _zero_pad(shape: int) -> np.ndarray:
- """Zero padding method."""
- return np.zeros(shape, dtype=np.float32)
-
- @staticmethod
- def _random_pad(shape: int) -> np.ndarray:
- """Random padding method."""
- # librosa load raw audio file into a distribution of -1~+1
- return np.random.rand(shape).astype(np.float32) * 2 - 1
-
- def transform(self, results: dict) -> dict:
- """Perform the librosa initialization.
-
- Args:
- results (dict): The resulting dict to be modified and passed
- to the next transform in pipeline.
- """
- try:
- import librosa
- except ImportError:
- raise ImportError('Please install librosa first.')
+class LoadAudioFeature(BaseTransform):
+ """Load offline extracted audio features.
- if self.file_client is None:
- self.file_client = FileClient(self.io_backend, **self.kwargs)
- if osp.exists(results['audio_path']):
- file_obj = io.BytesIO(self.file_client.get(results['audio_path']))
- y, sr = librosa.load(file_obj, sr=self.sample_rate)
- else:
- # Generate a random dummy 10s input
- pad_func = getattr(self, f'_{self.pad_method}_pad')
- y = pad_func(int(round(10.0 * self.sample_rate)))
- sr = self.sample_rate
+ Required Keys:
- results['length'] = y.shape[0]
- results['sample_rate'] = sr
- results['audios'] = y
- return results
-
- def __repr__(self):
- repr_str = (f'{self.__class__.__name__}('
- f'io_backend={self.io_backend}, '
- f'sample_rate={self.sample_rate}, '
- f'pad_method={self.pad_method})')
- return repr_str
+ - audio_path
+ Added Keys:
-@TRANSFORMS.register_module()
-class LoadAudioFeature(BaseTransform):
- """Load offline extracted audio features.
+ - length
+ - audios
- Required keys are "audio_path", added or modified keys are "length",
- audios".
+ Args:
+ pad_method (str): Padding method. Defaults to ``'zero'``.
"""
- def __init__(self, pad_method='zero'):
+ def __init__(self, pad_method: str = 'zero') -> None:
if pad_method not in ['zero', 'random']:
raise NotImplementedError
self.pad_method = pad_method
@staticmethod
- def _zero_pad(shape):
+ def _zero_pad(shape: int) -> np.ndarray:
"""Zero padding method."""
return np.zeros(shape, dtype=np.float32)
@staticmethod
- def _random_pad(shape):
+ def _random_pad(shape: int) -> np.ndarray:
"""Random padding method."""
# spectrogram is normalized into a distribution of 0~1
return np.random.rand(shape).astype(np.float32)
- def transform(self, results):
+ def transform(self, results: Dict) -> Dict:
"""Perform the numpy loading.
Args:
@@ -1738,68 +1668,12 @@ def transform(self, results):
results['audios'] = feature_map
return results
- def __repr__(self):
+ def __repr__(self) -> str:
repr_str = (f'{self.__class__.__name__}('
f'pad_method={self.pad_method})')
return repr_str
-@TRANSFORMS.register_module()
-class AudioDecode(BaseTransform):
- """Sample the audio w.r.t. the frames selected.
-
- Args:
- fixed_length (int): As the audio clip selected by frames sampled may
- not be exactly the same, ``fixed_length`` will truncate or pad them
- into the same size. Defaults to 32000.
-
- Required keys are ``frame_inds``, ``num_clips``, ``total_frames``,
- ``length``, added or modified keys are ``audios``, ``audios_shape``.
- """
-
- def __init__(self, fixed_length: int = 32000) -> None:
- self.fixed_length = fixed_length
-
- def transform(self, results: dict) -> dict:
- """Perform the ``AudioDecode`` to pick audio clips."""
- audio = results['audios']
- frame_inds = results['frame_inds']
- num_clips = results['num_clips']
- resampled_clips = list()
- frame_inds = frame_inds.reshape(num_clips, -1)
- for clip_idx in range(num_clips):
- clip_frame_inds = frame_inds[clip_idx]
- start_idx = max(
- 0,
- int(
- round((clip_frame_inds[0] + 1) / results['total_frames'] *
- results['length'])))
- end_idx = min(
- results['length'],
- int(
- round((clip_frame_inds[-1] + 1) / results['total_frames'] *
- results['length'])))
- cropped_audio = audio[start_idx:end_idx]
- if cropped_audio.shape[0] >= self.fixed_length:
- truncated_audio = cropped_audio[:self.fixed_length]
- else:
- truncated_audio = np.pad(
- cropped_audio,
- ((0, self.fixed_length - cropped_audio.shape[0])),
- mode='constant')
-
- resampled_clips.append(truncated_audio)
-
- results['audios'] = np.array(resampled_clips)
- results['audios_shape'] = results['audios'].shape
- return results
-
- def __repr__(self):
- repr_str = self.__class__.__name__
- repr_str += f"(fixed_length='{self.fixed_length}')"
- return repr_str
-
-
@TRANSFORMS.register_module()
class BuildPseudoClip(BaseTransform):
"""Build pseudo clips with one single image by repeating it n times.
@@ -1840,19 +1714,32 @@ def __repr__(self):
class AudioFeatureSelector(BaseTransform):
"""Sample the audio feature w.r.t. the frames selected.
- Required keys are "audios", "frame_inds", "num_clips", "length",
- "total_frames", added or modified keys are "audios", "audios_shape".
+ Required Keys:
+
+ - audios
+ - frame_inds
+ - num_clips
+ - length
+ - total_frames
+
+ Modified Keys:
+
+ - audios
+
+ Added Keys:
+
+ - audios_shape
Args:
fixed_length (int): As the features selected by frames sampled may
not be exactly the same, `fixed_length` will truncate or pad them
- into the same size. Default: 128.
+ into the same size. Defaults to 128.
"""
- def __init__(self, fixed_length=128):
+ def __init__(self, fixed_length: int = 128) -> None:
self.fixed_length = fixed_length
- def transform(self, results):
+ def transform(self, results: Dict) -> Dict:
"""Perform the ``AudioFeatureSelector`` to pick audio feature clips.
Args:
@@ -1891,7 +1778,7 @@ def transform(self, results):
results['audios_shape'] = results['audios'].shape
return results
- def __repr__(self):
+ def __repr__(self) -> str:
repr_str = (f'{self.__class__.__name__}('
f'fix_length={self.fixed_length})')
return repr_str
diff --git a/mmaction/datasets/transforms/pose_transforms.py b/mmaction/datasets/transforms/pose_transforms.py
index 0420f4ec8d..8627a79f96 100644
--- a/mmaction/datasets/transforms/pose_transforms.py
+++ b/mmaction/datasets/transforms/pose_transforms.py
@@ -1,12 +1,11 @@
# Copyright (c) OpenMMLab. All rights reserved.
-import copy as cp
-import pickle
from typing import Dict, List, Optional, Tuple, Union
import numpy as np
+import scipy
from mmcv.transforms import BaseTransform, KeyMapper
from mmengine.dataset import Compose
-from mmengine.fileio import FileClient
+from packaging import version as pv
from scipy.stats import mode
from torch.nn.modules.utils import _pair
@@ -14,93 +13,65 @@
from .loading import DecordDecode, DecordInit
from .processing import _combine_quadruple
+if pv.parse(scipy.__version__) < pv.parse('1.11.0'):
+ get_mode = mode
+else:
+ from functools import partial
+ get_mode = partial(mode, keepdims=True)
+
@TRANSFORMS.register_module()
-class LoadKineticsPose(BaseTransform):
- """Load Kinetics Pose given filename (The format should be pickle)
+class DecompressPose(BaseTransform):
+ """Load Compressed Pose.
+
+ Required Keys:
+
+ - frame_inds
+ - total_frames
+ - keypoint
+ - anno_inds (optional)
- Required keys are "filename", "total_frames", "img_shape", "frame_inds",
- "anno_inds" (for mmpose source, optional), added or modified keys are
- "keypoint", "keypoint_score".
+ Modified Keys:
+
+ - keypoint
+ - frame_inds
+
+ Added Keys:
+
+ - keypoint_score
+ - num_person
Args:
- io_backend (str): IO backend where frames are stored. Default: 'disk'.
squeeze (bool): Whether to remove frames with no human pose.
- Default: True.
- max_person (int): The max number of persons in a frame. Default: 10.
- keypoint_weight (dict): The weight of keypoints. We set the confidence
- score of a person as the weighted sum of confidence scores of each
- joint. Persons with low confidence scores are dropped (if exceed
- max_person). Default: dict(face=1, torso=2, limb=3).
- source (str): The sources of the keypoints used. Choices are 'mmpose'
- and 'openpose-18'. Default: 'mmpose'.
- kwargs (dict, optional): Arguments for FileClient.
+ Defaults to True.
+ max_person (int): The max number of persons in a frame. Defaults to 10.
"""
- def __init__(self,
- io_backend='disk',
- squeeze=True,
- max_person=100,
- keypoint_weight=dict(face=1, torso=2, limb=3),
- source='mmpose',
- **kwargs):
-
- self.io_backend = io_backend
+ def __init__(self, squeeze: bool = True, max_person: int = 10) -> None:
self.squeeze = squeeze
self.max_person = max_person
- self.keypoint_weight = cp.deepcopy(keypoint_weight)
- self.source = source
- if source == 'openpose-18':
- self.kpsubset = dict(
- face=[0, 14, 15, 16, 17],
- torso=[1, 2, 8, 5, 11],
- limb=[3, 4, 6, 7, 9, 10, 12, 13])
- elif source == 'mmpose':
- self.kpsubset = dict(
- face=[0, 1, 2, 3, 4],
- torso=[5, 6, 11, 12],
- limb=[7, 8, 9, 10, 13, 14, 15, 16])
- else:
- raise NotImplementedError('Unknown source of Kinetics Pose')
-
- self.kwargs = kwargs
- self.file_client = None
-
- def transform(self, results):
- """Perform the kinetics pose decoding.
+ def transform(self, results: Dict) -> Dict:
+ """Perform the pose decoding.
Args:
results (dict): The resulting dict to be modified and passed
to the next transform in pipeline.
"""
- assert 'filename' in results
- filename = results.pop('filename')
-
- # only applicable to source == 'mmpose'
- anno_inds = None
- if 'anno_inds' in results:
- assert self.source == 'mmpose'
- anno_inds = results.pop('anno_inds')
- results.pop('box_score', None)
-
- if self.file_client is None:
- self.file_client = FileClient(self.io_backend, **self.kwargs)
-
- bytes = self.file_client.get(filename)
-
- # only the kp array is in the pickle file, each kp include x, y, score.
- kps = pickle.loads(bytes)
+ required_keys = ['total_frames', 'frame_inds', 'keypoint']
+ for k in required_keys:
+ assert k in results
total_frames = results['total_frames']
-
frame_inds = results.pop('frame_inds')
+ keypoint = results['keypoint']
- if anno_inds is not None:
- kps = kps[anno_inds]
- frame_inds = frame_inds[anno_inds]
+ if 'anno_inds' in results:
+ frame_inds = frame_inds[results['anno_inds']]
+ keypoint = keypoint[results['anno_inds']]
- frame_inds = list(frame_inds)
+ assert np.all(np.diff(frame_inds) >= 0), \
+ 'frame_inds should be monotonical increasing'
def mapinds(inds):
uni = np.unique(inds)
@@ -112,63 +83,43 @@ def mapinds(inds):
frame_inds = mapinds(frame_inds)
total_frames = np.max(frame_inds) + 1
- # write it back
results['total_frames'] = total_frames
- h, w = results['img_shape']
- if self.source == 'openpose-18':
- kps[:, :, 0] *= w
- kps[:, :, 1] *= h
+ num_joints = keypoint.shape[1]
+ num_person = get_mode(frame_inds)[-1][0]
- num_kp = kps.shape[1]
- num_person = mode(frame_inds)[-1]
- # Ensure compatibility with lower version of scipy
- if isinstance(num_person, np.ndarray):
- num_person = num_person[0]
-
- new_kp = np.zeros([num_person, total_frames, num_kp, 2],
+ new_kp = np.zeros([num_person, total_frames, num_joints, 2],
dtype=np.float16)
- new_kpscore = np.zeros([num_person, total_frames, num_kp],
+ new_kpscore = np.zeros([num_person, total_frames, num_joints],
dtype=np.float16)
- # 32768 is enough
- num_person_frame = np.zeros([total_frames], dtype=np.int16)
+ nperson_per_frame = np.zeros([total_frames], dtype=np.int16)
- for frame_ind, kp in zip(frame_inds, kps):
- person_ind = num_person_frame[frame_ind]
+ for frame_ind, kp in zip(frame_inds, keypoint):
+ person_ind = nperson_per_frame[frame_ind]
new_kp[person_ind, frame_ind] = kp[:, :2]
new_kpscore[person_ind, frame_ind] = kp[:, 2]
- num_person_frame[frame_ind] += 1
-
- kpgrp = self.kpsubset
- weight = self.keypoint_weight
- results['num_person'] = num_person
+ nperson_per_frame[frame_ind] += 1
if num_person > self.max_person:
for i in range(total_frames):
- np_frame = num_person_frame[i]
- val = new_kpscore[:np_frame, i]
-
- val = (
- np.sum(val[:, kpgrp['face']], 1) * weight['face'] +
- np.sum(val[:, kpgrp['torso']], 1) * weight['torso'] +
- np.sum(val[:, kpgrp['limb']], 1) * weight['limb'])
- inds = sorted(range(np_frame), key=lambda x: -val[x])
- new_kpscore[:np_frame, i] = new_kpscore[inds, i]
- new_kp[:np_frame, i] = new_kp[inds, i]
- results['num_person'] = self.max_person
-
- results['keypoint'] = new_kp[:self.max_person]
- results['keypoint_score'] = new_kpscore[:self.max_person]
+ nperson = nperson_per_frame[i]
+ val = new_kpscore[:nperson, i]
+ score_sum = val.sum(-1)
+
+ inds = sorted(range(nperson), key=lambda x: -score_sum[x])
+ new_kpscore[:nperson, i] = new_kpscore[inds, i]
+ new_kp[:nperson, i] = new_kp[inds, i]
+ num_person = self.max_person
+ results['num_person'] = num_person
+
+ results['keypoint'] = new_kp[:num_person]
+ results['keypoint_score'] = new_kpscore[:num_person]
return results
- def __repr__(self):
+ def __repr__(self) -> str:
repr_str = (f'{self.__class__.__name__}('
- f'io_backend={self.io_backend}, '
f'squeeze={self.squeeze}, '
- f'max_person={self.max_person}, '
- f'keypoint_weight={self.keypoint_weight}, '
- f'source={self.source}, '
- f'kwargs={self.kwargs})')
+ f'max_person={self.max_person})')
return repr_str
diff --git a/mmaction/datasets/transforms/processing.py b/mmaction/datasets/transforms/processing.py
index 13637dcf38..6d54c6bf24 100644
--- a/mmaction/datasets/transforms/processing.py
+++ b/mmaction/datasets/transforms/processing.py
@@ -613,8 +613,9 @@ class Resize(BaseTransform):
keep_ratio (bool): If set to True, Images will be resized without
changing the aspect ratio. Otherwise, it will resize images to a
given size. Default: True.
- interpolation (str): Algorithm used for interpolation:
- "nearest" | "bilinear". Default: "bilinear".
+ interpolation (str): Algorithm used for interpolation,
+ accepted values are "nearest", "bilinear", "bicubic", "area",
+ "lanczos". Default: "bilinear".
lazy (bool): Determine whether to apply lazy operation. Default: False.
"""
@@ -1276,117 +1277,6 @@ def __repr__(self):
return repr_str
-@TRANSFORMS.register_module()
-class AudioAmplify(BaseTransform):
- """Amplify the waveform.
-
- Required keys are ``audios``, added or modified keys are ``audios``,
- ``amplify_ratio``.
-
- Args:
- ratio (float): The ratio used to amplify the audio waveform.
- """
-
- def __init__(self, ratio: float) -> None:
- if isinstance(ratio, float):
- self.ratio = ratio
- else:
- raise TypeError('Amplification ratio should be float.')
-
- def transform(self, results: dict) -> dict:
- """Perform the audio amplification.
-
- Args:
- results (dict): The resulting dict to be modified and passed
- to the next transform in pipeline.
- """
-
- assert 'audios' in results
- results['audios'] *= self.ratio
- results['amplify_ratio'] = self.ratio
-
- return results
-
- def __repr__(self):
- repr_str = f'{self.__class__.__name__}(ratio={self.ratio})'
- return repr_str
-
-
-@TRANSFORMS.register_module()
-class MelSpectrogram(BaseTransform):
- """MelSpectrogram. Transfer an audio wave into a melspectogram figure.
-
- Required keys are ``audios``, ``sample_rate``, ``num_clips``, added or
- modified keys are ``audios``.
-
- Args:
- window_size (int): The window size in millisecond. Defaults to 32.
- step_size (int): The step size in millisecond. Defaults to 16.
- n_mels (int): Number of mels. Defaults to 80.
- fixed_length (int): The sample length of melspectrogram maybe not
- exactly as wished due to different fps, fix the length for batch
- collation by truncating or padding. Defaults to 128.
- """
-
- def __init__(self,
- window_size: int = 32,
- step_size: int = 16,
- n_mels: int = 80,
- fixed_length: int = 128) -> None:
- if all(
- isinstance(x, int)
- for x in [window_size, step_size, n_mels, fixed_length]):
- self.window_size = window_size
- self.step_size = step_size
- self.n_mels = n_mels
- self.fixed_length = fixed_length
- else:
- raise TypeError('All arguments should be int.')
-
- def transform(self, results: dict) -> dict:
- """Perform MelSpectrogram transformation.
-
- Args:
- results (dict): The resulting dict to be modified and passed
- to the next transform in pipeline.
- """
- try:
- import librosa
- except ImportError:
- raise ImportError('Install librosa first.')
- signals = results['audios']
- sample_rate = results['sample_rate']
- n_fft = int(round(sample_rate * self.window_size / 1000))
- hop_length = int(round(sample_rate * self.step_size / 1000))
- melspectrograms = list()
- for clip_idx in range(results['num_clips']):
- clip_signal = signals[clip_idx]
- mel = librosa.feature.melspectrogram(
- y=clip_signal,
- sr=sample_rate,
- n_fft=n_fft,
- hop_length=hop_length,
- n_mels=self.n_mels)
- if mel.shape[0] >= self.fixed_length:
- mel = mel[:self.fixed_length, :]
- else:
- mel = np.pad(
- mel, ((0, self.fixed_length - mel.shape[0]), (0, 0)),
- mode='edge')
- melspectrograms.append(mel)
-
- results['audios'] = np.array(melspectrograms)
- return results
-
- def __repr__(self):
- repr_str = (f'{self.__class__.__name__}'
- f'(window_size={self.window_size}), '
- f'step_size={self.step_size}, '
- f'n_mels={self.n_mels}, '
- f'fixed_length={self.fixed_length})')
- return repr_str
-
-
@TRANSFORMS.register_module()
class RandomErasing(BaseTransform):
"""Randomly selects a rectangle region in an image and erase pixels.
diff --git a/mmaction/engine/runner/__init__.py b/mmaction/engine/runner/__init__.py
index c7dc511ea8..9bc36f001b 100644
--- a/mmaction/engine/runner/__init__.py
+++ b/mmaction/engine/runner/__init__.py
@@ -1,4 +1,7 @@
# Copyright (c) OpenMMLab. All rights reserved.
from .multi_loop import MultiLoaderEpochBasedTrainLoop
+from .retrieval_loop import RetrievalTestLoop, RetrievalValLoop
-__all__ = ['MultiLoaderEpochBasedTrainLoop']
+__all__ = [
+ 'MultiLoaderEpochBasedTrainLoop', 'RetrievalValLoop', 'RetrievalTestLoop'
+]
diff --git a/mmaction/engine/runner/retrieval_loop.py b/mmaction/engine/runner/retrieval_loop.py
new file mode 100644
index 0000000000..dc884876da
--- /dev/null
+++ b/mmaction/engine/runner/retrieval_loop.py
@@ -0,0 +1,168 @@
+# Copyright (c) OpenMMLab. All rights reserved.
+
+import torch
+from mmengine.model import is_model_wrapper
+from mmengine.runner import TestLoop, ValLoop, autocast
+
+from mmaction.registry import LOOPS
+
+
+@LOOPS.register_module()
+class RetrievalValLoop(ValLoop):
+ """Loop for multimodal retrieval val.
+
+ Args:
+ runner (Runner): A reference of runner.
+ dataloader (Dataloader or dict): A dataloader object or a dict to
+ build a dataloader.
+ evaluator (Evaluator or dict or list): Used for computing metrics.
+ fp16 (bool): Whether to enable fp16 valing. Defaults to
+ False.
+ """
+
+ def run(self) -> dict:
+ """Launch val."""
+ self.runner.call_hook('before_val')
+ self.runner.call_hook('before_val_epoch')
+ self.runner.model.eval()
+
+ feats_local = []
+ data_samples_local = []
+
+ for idx, data_batch in enumerate(self.dataloader):
+ with torch.no_grad():
+ self.runner.call_hook(
+ 'before_val_iter', batch_idx=idx, data_batch=data_batch)
+ # predictions should be sequence of BaseDataElement
+ with autocast(enabled=self.fp16):
+ if is_model_wrapper(self.runner.model):
+ data_preprocessor = self.runner.model.module.data_preprocessor # noqa: E501
+ else:
+ data_preprocessor = self.runner.model.data_preprocessor
+
+ # get features for retrieval instead of data samples
+ data_batch = data_preprocessor(data_batch, False)
+ feats = self.runner.model._run_forward(
+ data_batch, mode='tensor')
+ feats_local.append(feats)
+ data_samples_local.extend(data_batch['data_samples'])
+ self.runner.call_hook(
+ 'after_val_iter',
+ batch_idx=idx,
+ data_batch=data_batch,
+ outputs=feats)
+
+ # concatenate different features
+ feats_local = {
+ k: torch.cat([dic[k] for dic in feats_local])
+ for k in feats_local[0]
+ }
+
+ # get predictions
+ if is_model_wrapper(self.runner.model):
+ predict_all_fn = self.runner.model.module.predict_all
+ else:
+ predict_all_fn = self.runner.model.predict_all
+
+ num_videos = self.dataloader.dataset.num_videos
+ num_texts = self.dataloader.dataset.num_texts
+ with torch.no_grad():
+ with autocast(enabled=self.fp16):
+ i2t_data_samples, t2i_data_samples = predict_all_fn(
+ feats_local,
+ data_samples_local,
+ num_images=num_videos,
+ num_texts=num_texts,
+ )
+ # process in evaluator and compute metrics
+ self.evaluator.process(i2t_data_samples, None)
+ i2t_metrics = self.evaluator.evaluate(num_videos)
+ i2t_metrics = {f'i2t/{k}': v for k, v in i2t_metrics.items()}
+ self.evaluator.process(t2i_data_samples, None)
+ t2i_metrics = self.evaluator.evaluate(num_texts)
+ t2i_metrics = {f't2i/{k}': v for k, v in t2i_metrics.items()}
+ metrics = {**i2t_metrics, **t2i_metrics}
+ self.runner.call_hook('after_val_epoch', metrics=metrics)
+ self.runner.call_hook('after_val')
+ return metrics
+
+
+@LOOPS.register_module()
+class RetrievalTestLoop(TestLoop):
+ """Loop for multimodal retrieval test.
+
+ Args:
+ runner (Runner): A reference of runner.
+ dataloader (Dataloader or dict): A dataloader object or a dict to
+ build a dataloader.
+ evaluator (Evaluator or dict or list): Used for computing metrics.
+ fp16 (bool): Whether to enable fp16 testing. Defaults to
+ False.
+ """
+
+ def run(self) -> dict:
+ """Launch test."""
+ self.runner.call_hook('before_test')
+ self.runner.call_hook('before_test_epoch')
+ self.runner.model.eval()
+
+ feats_local = []
+ data_samples_local = []
+
+ for idx, data_batch in enumerate(self.dataloader):
+ with torch.no_grad():
+ self.runner.call_hook(
+ 'before_test_iter', batch_idx=idx, data_batch=data_batch)
+ # predictions should be sequence of BaseDataElement
+ with autocast(enabled=self.fp16):
+ if is_model_wrapper(self.runner.model):
+ data_preprocessor = self.runner.model.module.data_preprocessor # noqa: E501
+ else:
+ data_preprocessor = self.runner.model.data_preprocessor
+ # get features for retrieval instead of data samples
+ data_batch = data_preprocessor(data_batch, False)
+ feats = self.runner.model._run_forward(
+ data_batch, mode='tensor')
+ feats_local.append(feats)
+ data_samples_local.extend(data_batch['data_samples'])
+ self.runner.call_hook(
+ 'after_test_iter',
+ batch_idx=idx,
+ data_batch=data_batch,
+ outputs=feats)
+
+ # concatenate different features
+ feats_local = {
+ k: torch.cat([dic[k] for dic in feats_local])
+ for k in feats_local[0]
+ }
+
+ # get predictions
+ if is_model_wrapper(self.runner.model):
+ predict_all_fn = self.runner.model.module.predict_all
+ else:
+ predict_all_fn = self.runner.model.predict_all
+
+ num_videos = self.dataloader.dataset.num_videos
+ num_texts = self.dataloader.dataset.num_texts
+ with torch.no_grad():
+ with autocast(enabled=self.fp16):
+ i2t_data_samples, t2i_data_samples = predict_all_fn(
+ feats_local,
+ data_samples_local,
+ num_images=num_videos,
+ num_texts=num_texts,
+ )
+
+ # process in evaluator and compute metrics
+ self.evaluator.process(i2t_data_samples, None)
+ i2t_metrics = self.evaluator.evaluate(num_videos)
+ i2t_metrics = {f'i2t/{k}': v for k, v in i2t_metrics.items()}
+ self.evaluator.process(t2i_data_samples, None)
+ t2i_metrics = self.evaluator.evaluate(num_texts)
+ t2i_metrics = {f't2i/{k}': v for k, v in t2i_metrics.items()}
+ metrics = {**i2t_metrics, **t2i_metrics}
+
+ self.runner.call_hook('after_test_epoch', metrics=metrics)
+ self.runner.call_hook('after_test')
+ return metrics
diff --git a/mmaction/evaluation/functional/multisports_utils.py b/mmaction/evaluation/functional/multisports_utils.py
index 516828c701..72643d977f 100644
--- a/mmaction/evaluation/functional/multisports_utils.py
+++ b/mmaction/evaluation/functional/multisports_utils.py
@@ -7,6 +7,7 @@
from collections import defaultdict
import numpy as np
+from mmengine.logging import MMLogger
from rich.progress import track
@@ -314,7 +315,7 @@ def tubescore(tt):
def frameAP(GT, alldets, thr, print_info=True):
-
+ logger = MMLogger.get_current_instance()
vlist = GT['test_videos'][0]
results = {}
@@ -326,7 +327,7 @@ def frameAP(GT, alldets, thr, print_info=True):
'basketball save', 'basketball jump ball'
]:
if print_info:
- print('do not evaluate {}'.format(label))
+ logger.info('do not evaluate {}'.format(label))
continue
# det format: # noqa: E501
detections = alldets[alldets[:, 2] == ilabel, :]
@@ -355,7 +356,7 @@ def frameAP(GT, alldets, thr, print_info=True):
gt_num = sum([g.shape[0] for g in gt.values()])
if gt_num == 0:
if print_info:
- print('no such label', ilabel, label)
+ logger.info('no such label', ilabel, label)
continue
fp = 0 # false positives
tp = 0 # true positives
@@ -395,15 +396,15 @@ def frameAP(GT, alldets, thr, print_info=True):
class_result[label] = pr_to_ap_voc(results[label]) * 100
frameap_result = np.mean(ap)
if print_info:
- print('frameAP_{}\n'.format(thr))
+ logger.info('frameAP_{}\n'.format(thr))
for label in class_result:
- print('{:20s} {:8.2f}'.format(label, class_result[label]))
- print('{:20s} {:8.2f}'.format('mAP', frameap_result))
+ logger.info('{:20s} {:8.2f}'.format(label, class_result[label]))
+ logger.info('{:20s} {:8.2f}'.format('mAP', frameap_result))
return frameap_result
def videoAP(GT, alldets, thr, print_info=True):
-
+ logger = MMLogger.get_current_instance()
vlist = GT['test_videos'][0]
res = {}
@@ -414,7 +415,7 @@ def videoAP(GT, alldets, thr, print_info=True):
'basketball save', 'basketball jump ball'
]:
if print_info:
- print('do not evaluate{}'.format(GT['labels'][ilabel]))
+ logger.info('do not evaluate{}'.format(GT['labels'][ilabel]))
continue
detections = alldets[ilabel]
# load ground-truth
@@ -438,7 +439,7 @@ def videoAP(GT, alldets, thr, print_info=True):
tp = 0 # true positives
if gt_num == 0:
if print_info:
- print('no such label', ilabel, GT['labels'][ilabel])
+ logger.info('no such label', ilabel, GT['labels'][ilabel])
continue
is_gt_box_detected = {}
for i, j in enumerate(
@@ -471,10 +472,10 @@ def videoAP(GT, alldets, thr, print_info=True):
for label in res:
class_result[label] = pr_to_ap_voc(res[label]) * 100
if print_info:
- print('VideoAP_{}\n'.format(thr))
+ logger.info('VideoAP_{}\n'.format(thr))
for label in class_result:
- print('{:20s} {:8.2f}'.format(label, class_result[label]))
- print('{:20s} {:8.2f}'.format('mAP', videoap_result))
+ logger.info('{:20s} {:8.2f}'.format(label, class_result[label]))
+ logger.info('{:20s} {:8.2f}'.format('mAP', videoap_result))
return videoap_result
diff --git a/mmaction/evaluation/metrics/__init__.py b/mmaction/evaluation/metrics/__init__.py
index 8bf22c6672..fd50aded2e 100644
--- a/mmaction/evaluation/metrics/__init__.py
+++ b/mmaction/evaluation/metrics/__init__.py
@@ -2,10 +2,13 @@
from .acc_metric import AccMetric, ConfusionMatrix
from .anet_metric import ANetMetric
from .ava_metric import AVAMetric
+from .multimodal_metric import VQAMCACC, ReportVQA, RetrievalRecall, VQAAcc
from .multisports_metric import MultiSportsMetric
from .retrieval_metric import RetrievalMetric
+from .video_grounding_metric import RecallatTopK
__all__ = [
'AccMetric', 'AVAMetric', 'ANetMetric', 'ConfusionMatrix',
- 'MultiSportsMetric', 'RetrievalMetric'
+ 'MultiSportsMetric', 'RetrievalMetric', 'VQAAcc', 'ReportVQA', 'VQAMCACC',
+ 'RetrievalRecall', 'RecallatTopK'
]
diff --git a/mmaction/evaluation/metrics/acc_metric.py b/mmaction/evaluation/metrics/acc_metric.py
index 9abc20fa6c..04985e5938 100644
--- a/mmaction/evaluation/metrics/acc_metric.py
+++ b/mmaction/evaluation/metrics/acc_metric.py
@@ -75,17 +75,23 @@ def process(self, data_batch: Sequence[Tuple[Any, Dict]],
data_samples = copy.deepcopy(data_samples)
for data_sample in data_samples:
result = dict()
- pred = data_sample['pred_scores']
- label = data_sample['gt_labels']
- for item_name, score in pred.items():
- pred[item_name] = score.cpu().numpy()
+ pred = data_sample['pred_score']
+ label = data_sample['gt_label']
+
+ # Ad-hoc for RGBPoseConv3D
+ if isinstance(pred, dict):
+ for item_name, score in pred.items():
+ pred[item_name] = score.cpu().numpy()
+ else:
+ pred = pred.cpu().numpy()
+
result['pred'] = pred
- if label['item'].size(0) == 1:
+ if label.size(0) == 1:
# single-label
- result['label'] = label['item'].item()
+ result['label'] = label.item()
else:
# multi-label
- result['label'] = label['item'].cpu().numpy()
+ result['label'] = label.cpu().numpy()
self.results.append(result)
def compute_metrics(self, results: List) -> Dict:
@@ -100,39 +106,41 @@ def compute_metrics(self, results: List) -> Dict:
"""
labels = [x['label'] for x in results]
- if len(results[0]['pred']) == 1:
- preds = [x['pred']['item'] for x in results]
- return self.calculate(preds, labels)
-
eval_results = dict()
- for item_name in results[0]['pred'].keys():
- preds = [x['pred'][item_name] for x in results]
- eval_result = self.calculate(preds, labels)
- eval_results.update(
- {f'{item_name}_{k}': v
- for k, v in eval_result.items()})
-
# Ad-hoc for RGBPoseConv3D
- if len(results[0]['pred']) == 2 and \
- 'rgb' in results[0]['pred'] and \
- 'pose' in results[0]['pred']:
-
- rgb = [x['pred']['rgb'] for x in results]
- pose = [x['pred']['pose'] for x in results]
-
- preds = {
- '1:1': get_weighted_score([rgb, pose], [1, 1]),
- '2:1': get_weighted_score([rgb, pose], [2, 1]),
- '1:2': get_weighted_score([rgb, pose], [1, 2])
- }
- for k in preds:
- eval_result = self.calculate(preds[k], labels)
- eval_results.update({
- f'RGBPose_{k}_{key}': v
- for key, v in eval_result.items()
- })
-
- return eval_results
+ if isinstance(results[0]['pred'], dict):
+
+ for item_name in results[0]['pred'].keys():
+ preds = [x['pred'][item_name] for x in results]
+ eval_result = self.calculate(preds, labels)
+ eval_results.update(
+ {f'{item_name}_{k}': v
+ for k, v in eval_result.items()})
+
+ if len(results[0]['pred']) == 2 and \
+ 'rgb' in results[0]['pred'] and \
+ 'pose' in results[0]['pred']:
+
+ rgb = [x['pred']['rgb'] for x in results]
+ pose = [x['pred']['pose'] for x in results]
+
+ preds = {
+ '1:1': get_weighted_score([rgb, pose], [1, 1]),
+ '2:1': get_weighted_score([rgb, pose], [2, 1]),
+ '1:2': get_weighted_score([rgb, pose], [1, 2])
+ }
+ for k in preds:
+ eval_result = self.calculate(preds[k], labels)
+ eval_results.update({
+ f'RGBPose_{k}_{key}': v
+ for key, v in eval_result.items()
+ })
+ return eval_results
+
+ # Simple Acc Calculation
+ else:
+ preds = [x['pred'] for x in results]
+ return self.calculate(preds, labels)
def calculate(self, preds: List[np.ndarray],
labels: List[Union[int, np.ndarray]]) -> Dict:
@@ -238,13 +246,13 @@ def __init__(self,
def process(self, data_batch, data_samples: Sequence[dict]) -> None:
for data_sample in data_samples:
- pred_scores = data_sample.get('pred_scores')
- gt_label = data_sample['gt_labels']['item']
+ pred_scores = data_sample.get('pred_score')
+ gt_label = data_sample['gt_label']
if pred_scores is not None:
- pred_label = pred_scores['item'].argmax(dim=0, keepdim=True)
- self.num_classes = pred_scores['item'].size(0)
+ pred_label = pred_scores.argmax(dim=0, keepdim=True)
+ self.num_classes = pred_scores.size(0)
else:
- pred_label = data_sample['pred_labels']['item']
+ pred_label = data_sample['pred_label']
self.results.append({
'pred_label': pred_label,
diff --git a/mmaction/evaluation/metrics/multimodal_metric.py b/mmaction/evaluation/metrics/multimodal_metric.py
new file mode 100644
index 0000000000..2c144ac10a
--- /dev/null
+++ b/mmaction/evaluation/metrics/multimodal_metric.py
@@ -0,0 +1,565 @@
+# Copyright (c) OpenMMLab. All rights reserved.
+# Copied from mmpretrain
+# Partly adopted from https://github.com/GT-Vision-Lab/VQA
+# Copyright (c) 2014, Aishwarya Agrawal
+from typing import List, Optional, Sequence, Union
+
+import mmengine
+import numpy as np
+import torch
+import torch.nn.functional as F
+from mmengine.evaluator import BaseMetric
+from mmengine.logging import MMLogger
+from mmengine.utils import is_seq_of
+
+from mmaction.registry import METRICS
+from mmaction.structures.action_data_sample import format_label
+from .acc_metric import to_tensor
+
+
+def _process_punctuation(inText):
+ import re
+ outText = inText
+ punct = [
+ ';', r'/', '[', ']', '"', '{', '}', '(', ')', '=', '+', '\\', '_', '-',
+ '>', '<', '@', '`', ',', '?', '!'
+ ]
+ commaStrip = re.compile('(\d)(,)(\d)') # noqa: W605
+ periodStrip = re.compile('(?!<=\d)(\.)(?!\d)') # noqa: W605
+ for p in punct:
+ if (p + ' ' in inText or ' ' + p in inText) or (re.search(
+ commaStrip, inText) is not None):
+ outText = outText.replace(p, '')
+ else:
+ outText = outText.replace(p, ' ')
+ outText = periodStrip.sub('', outText, re.UNICODE)
+ return outText
+
+
+def _process_digit_article(inText):
+ outText = []
+ tempText = inText.lower().split()
+ articles = ['a', 'an', 'the']
+ manualMap = {
+ 'none': '0',
+ 'zero': '0',
+ 'one': '1',
+ 'two': '2',
+ 'three': '3',
+ 'four': '4',
+ 'five': '5',
+ 'six': '6',
+ 'seven': '7',
+ 'eight': '8',
+ 'nine': '9',
+ 'ten': '10',
+ }
+ contractions = {
+ 'aint': "ain't",
+ 'arent': "aren't",
+ 'cant': "can't",
+ 'couldve': "could've",
+ 'couldnt': "couldn't",
+ "couldn'tve": "couldn't've",
+ "couldnt've": "couldn't've",
+ 'didnt': "didn't",
+ 'doesnt': "doesn't",
+ 'dont': "don't",
+ 'hadnt': "hadn't",
+ "hadnt've": "hadn't've",
+ "hadn'tve": "hadn't've",
+ 'hasnt': "hasn't",
+ 'havent': "haven't",
+ 'hed': "he'd",
+ "hed've": "he'd've",
+ "he'dve": "he'd've",
+ 'hes': "he's",
+ 'howd': "how'd",
+ 'howll': "how'll",
+ 'hows': "how's",
+ "Id've": "I'd've",
+ "I'dve": "I'd've",
+ 'Im': "I'm",
+ 'Ive': "I've",
+ 'isnt': "isn't",
+ 'itd': "it'd",
+ "itd've": "it'd've",
+ "it'dve": "it'd've",
+ 'itll': "it'll",
+ "let's": "let's",
+ 'maam': "ma'am",
+ 'mightnt': "mightn't",
+ "mightnt've": "mightn't've",
+ "mightn'tve": "mightn't've",
+ 'mightve': "might've",
+ 'mustnt': "mustn't",
+ 'mustve': "must've",
+ 'neednt': "needn't",
+ 'notve': "not've",
+ 'oclock': "o'clock",
+ 'oughtnt': "oughtn't",
+ "ow's'at": "'ow's'at",
+ "'ows'at": "'ow's'at",
+ "'ow'sat": "'ow's'at",
+ 'shant': "shan't",
+ "shed've": "she'd've",
+ "she'dve": "she'd've",
+ "she's": "she's",
+ 'shouldve': "should've",
+ 'shouldnt': "shouldn't",
+ "shouldnt've": "shouldn't've",
+ "shouldn'tve": "shouldn't've",
+ "somebody'd": 'somebodyd',
+ "somebodyd've": "somebody'd've",
+ "somebody'dve": "somebody'd've",
+ 'somebodyll': "somebody'll",
+ 'somebodys': "somebody's",
+ 'someoned': "someone'd",
+ "someoned've": "someone'd've",
+ "someone'dve": "someone'd've",
+ 'someonell': "someone'll",
+ 'someones': "someone's",
+ 'somethingd': "something'd",
+ "somethingd've": "something'd've",
+ "something'dve": "something'd've",
+ 'somethingll': "something'll",
+ 'thats': "that's",
+ 'thered': "there'd",
+ "thered've": "there'd've",
+ "there'dve": "there'd've",
+ 'therere': "there're",
+ 'theres': "there's",
+ 'theyd': "they'd",
+ "theyd've": "they'd've",
+ "they'dve": "they'd've",
+ 'theyll': "they'll",
+ 'theyre': "they're",
+ 'theyve': "they've",
+ 'twas': "'twas",
+ 'wasnt': "wasn't",
+ "wed've": "we'd've",
+ "we'dve": "we'd've",
+ 'weve': "we've",
+ 'werent': "weren't",
+ 'whatll': "what'll",
+ 'whatre': "what're",
+ 'whats': "what's",
+ 'whatve': "what've",
+ 'whens': "when's",
+ 'whered': "where'd",
+ 'wheres': "where's",
+ 'whereve': "where've",
+ 'whod': "who'd",
+ "whod've": "who'd've",
+ "who'dve": "who'd've",
+ 'wholl': "who'll",
+ 'whos': "who's",
+ 'whove': "who've",
+ 'whyll': "why'll",
+ 'whyre': "why're",
+ 'whys': "why's",
+ 'wont': "won't",
+ 'wouldve': "would've",
+ 'wouldnt': "wouldn't",
+ "wouldnt've": "wouldn't've",
+ "wouldn'tve": "wouldn't've",
+ 'yall': "y'all",
+ "yall'll": "y'all'll",
+ "y'allll": "y'all'll",
+ "yall'd've": "y'all'd've",
+ "y'alld've": "y'all'd've",
+ "y'all'dve": "y'all'd've",
+ 'youd': "you'd",
+ "youd've": "you'd've",
+ "you'dve": "you'd've",
+ 'youll': "you'll",
+ 'youre': "you're",
+ 'youve': "you've",
+ }
+ for word in tempText:
+ word = manualMap.setdefault(word, word)
+ if word not in articles:
+ outText.append(word)
+ for wordId, word in enumerate(outText):
+ if word in contractions:
+ outText[wordId] = contractions[word]
+ outText = ' '.join(outText)
+ return outText
+
+
+@METRICS.register_module()
+class VQAAcc(BaseMetric):
+ '''VQA Acc metric.
+ Args:
+
+ collect_device (str): Device name used for collecting results from
+ different ranks during distributed training. Must be 'cpu' or
+ 'gpu'. Defaults to 'cpu'.
+ prefix (str, optional): The prefix that will be added in the metric
+ names to disambiguate homonymous metrics of different evaluators.
+ If prefix is not provided in the argument, self.default_prefix
+ will be used instead. Should be modified according to the
+ `retrieval_type` for unambiguous results. Defaults to TR.
+ '''
+ default_prefix = 'VQA'
+
+ def __init__(self,
+ full_score_weight: float = 0.3,
+ collect_device: str = 'cpu',
+ prefix: Optional[str] = None):
+ super().__init__(collect_device=collect_device, prefix=prefix)
+ self.full_score_weight = full_score_weight
+
+ def process(self, data_batch, data_samples):
+ """Process one batch of data samples.
+
+ The processed results should be stored in ``self.results``, which will
+ be used to computed the metrics when all batches have been processed.
+
+ Args:
+ data_batch: A batch of data from the dataloader.
+ data_samples (Sequence[dict]): A batch of outputs from the model.
+ """
+ for sample in data_samples:
+ gt_answer = sample.get('gt_answer')
+ gt_answer_weight = sample.get('gt_answer_weight')
+ if isinstance(gt_answer, str):
+ gt_answer = [gt_answer]
+ if gt_answer_weight is None:
+ gt_answer_weight = [1. / (len(gt_answer))] * len(gt_answer)
+
+ result = {
+ 'pred_answer': sample.get('pred_answer'),
+ 'gt_answer': gt_answer,
+ 'gt_answer_weight': gt_answer_weight,
+ }
+
+ self.results.append(result)
+
+ def compute_metrics(self, results: List):
+ """Compute the metrics from processed results.
+
+ Args:
+ results (dict): The processed results of each batch.
+
+ Returns:
+ Dict: The computed metrics. The keys are the names of the metrics,
+ and the values are corresponding results.
+ """
+ acc = []
+ for result in results:
+ pred_answer = self._process_answer(result['pred_answer'])
+ gt_answer = [
+ self._process_answer(answer) for answer in result['gt_answer']
+ ]
+ answer_weight = result['gt_answer_weight']
+
+ weight_sum = 0
+ for i, gt in enumerate(gt_answer):
+ if gt == pred_answer:
+ weight_sum += answer_weight[i]
+ vqa_acc = min(1.0, weight_sum / self.full_score_weight)
+ acc.append(vqa_acc)
+
+ accuracy = sum(acc) / len(acc) * 100
+
+ metrics = {'acc': accuracy}
+ return metrics
+
+ def _process_answer(self, answer):
+ answer = answer.replace('\n', ' ')
+ answer = answer.replace('\t', ' ')
+ answer = answer.strip()
+ answer = _process_punctuation(answer)
+ answer = _process_digit_article(answer)
+ return answer
+
+
+@METRICS.register_module()
+class ReportVQA(BaseMetric):
+ """Dump VQA result to the standard json format for VQA evaluation.
+
+ Args:
+ file_path (str): The file path to save the result file.
+ collect_device (str): Device name used for collecting results from
+ different ranks during distributed training. Must be 'cpu' or
+ 'gpu'. Defaults to 'cpu'.
+ prefix (str, optional): The prefix that will be added in the metric
+ names to disambiguate homonymous metrics of different evaluators.
+ If prefix is not provided in the argument, self.default_prefix
+ will be used instead. Should be modified according to the
+ `retrieval_type` for unambiguous results. Defaults to TR.
+ """
+ default_prefix = 'VQA'
+
+ def __init__(self,
+ file_path: str,
+ collect_device: str = 'cpu',
+ prefix: Optional[str] = None):
+ super().__init__(collect_device=collect_device, prefix=prefix)
+ if not file_path.endswith('.json'):
+ raise ValueError('The output file must be a json file.')
+ self.file_path = file_path
+
+ def process(self, data_batch, data_samples) -> None:
+ """transfer tensors in predictions to CPU."""
+ for sample in data_samples:
+ question_id = sample['question_id']
+ pred_answer = sample['pred_answer']
+
+ result = {
+ 'question_id': int(question_id),
+ 'answer': pred_answer,
+ }
+
+ self.results.append(result)
+
+ def compute_metrics(self, results: List):
+ """Dump the result to json file."""
+ mmengine.dump(results, self.file_path)
+ logger = MMLogger.get_current_instance()
+ logger.info(f'Results has been saved to {self.file_path}.')
+ return {}
+
+
+@METRICS.register_module()
+class VQAMCACC(BaseMetric):
+ '''VQA multiple choice Acc metric.
+ Args:
+
+ collect_device (str): Device name used for collecting results from
+ different ranks during distributed training. Must be 'cpu' or
+ 'gpu'. Defaults to 'cpu'.
+ prefix (str, optional): The prefix that will be added in the metric
+ names to disambiguate homonymous metrics of different evaluators.
+ If prefix is not provided in the argument, self.default_prefix
+ will be used instead. Should be modified according to the
+ `retrieval_type` for unambiguous results. Defaults to TR.
+ '''
+ default_prefix = 'VQAMC'
+
+ def __init__(self,
+ collect_device: str = 'cpu',
+ prefix: Optional[str] = None):
+ super().__init__(collect_device=collect_device, prefix=prefix)
+
+ def process(self, data_batch, data_samples):
+ """Process one batch of data samples.
+
+ The processed results should be stored in ``self.results``, which will
+ be used to computed the metrics when all batches have been processed.
+
+ Args:
+ data_batch: A batch of data from the dataloader.
+ data_samples (Sequence[dict]): A batch of outputs from the model.
+ """
+ for sample in data_samples:
+ # gt_labels in datasample is a LabelData
+ label = sample['gt_label'].item()
+ result = {
+ 'pred_label': sample.get('pred_label'),
+ 'gt_label': label,
+ }
+
+ self.results.append(result)
+
+ def compute_metrics(self, results: List):
+ """Compute the metrics from processed results.
+
+ Args:
+ results (dict): The processed results of each batch.
+
+ Returns:
+ Dict: The computed metrics. The keys are the names of the metrics,
+ and the values are corresponding results.
+ """
+ preds = np.array([x['pred_label'] for x in results])
+ labels = np.array([x['gt_label'] for x in results])
+
+ accuracy = np.sum(preds == labels) / len(preds) * 100
+
+ metrics = {'acc': accuracy}
+ return metrics
+
+
+@METRICS.register_module()
+class RetrievalRecall(BaseMetric):
+ r"""Recall evaluation metric for image retrieval.
+
+ Args:
+ topk (int | Sequence[int]): If the ground truth label matches one of
+ the best **k** predictions, the sample will be regard as a positive
+ prediction. If the parameter is a tuple, all of top-k recall will
+ be calculated and outputted together. Defaults to 1.
+ collect_device (str): Device name used for collecting results from
+ different ranks during distributed training. Must be 'cpu' or
+ 'gpu'. Defaults to 'cpu'.
+ prefix (str, optional): The prefix that will be added in the metric
+ names to disambiguate homonymous metrics of different evaluators.
+ If prefix is not provided in the argument, self.default_prefix
+ will be used instead. Defaults to None.
+
+ """
+ default_prefix: Optional[str] = 'retrieval'
+
+ def __init__(self,
+ topk: Union[int, Sequence[int]],
+ collect_device: str = 'cpu',
+ prefix: Optional[str] = None) -> None:
+ topk = (topk, ) if isinstance(topk, int) else topk
+
+ for k in topk:
+ if k <= 0:
+ raise ValueError('`topk` must be a ingter larger than 0 '
+ 'or seq of ingter larger than 0.')
+
+ self.topk = topk
+ super().__init__(collect_device=collect_device, prefix=prefix)
+
+ def process(self, data_batch: Sequence[dict],
+ data_samples: Sequence[dict]):
+ """Process one batch of data and predictions.
+
+ The processed results should be stored in ``self.results``, which will
+ be used to computed the metrics when all batches have been processed.
+
+ Args:
+ data_batch (Sequence[dict]): A batch of data from the dataloader.
+ predictions (Sequence[dict]): A batch of outputs from the model.
+ """
+ for data_sample in data_samples:
+ pred_score = data_sample['pred_score'].cpu()
+ gt_label = format_label(data_sample['gt_label'])
+
+ if 'gt_score' in data_sample:
+ target = data_sample.get('gt_score').clone()
+ else:
+ num_classes = pred_score.size()[-1]
+ target = F.one_hot(gt_label, num_classes)
+
+ # Because the retrieval output logit vector will be much larger
+ # compared to the normal classification, to save resources, the
+ # evaluation results are computed each batch here and then reduce
+ # all results at the end.
+ result = RetrievalRecall.calculate(
+ pred_score.unsqueeze(0), target.unsqueeze(0), topk=self.topk)
+ self.results.append(result)
+
+ def compute_metrics(self, results: List):
+ """Compute the metrics from processed results.
+
+ Args:
+ results (list): The processed results of each batch.
+
+ Returns:
+ Dict: The computed metrics. The keys are the names of the metrics,
+ and the values are corresponding results.
+ """
+ result_metrics = dict()
+ for i, k in enumerate(self.topk):
+ recall_at_k = sum([r[i].item() for r in results]) / len(results)
+ result_metrics[f'Recall@{k}'] = recall_at_k
+
+ return result_metrics
+
+ @staticmethod
+ def calculate(pred: Union[np.ndarray, torch.Tensor],
+ target: Union[np.ndarray, torch.Tensor],
+ topk: Union[int, Sequence[int]],
+ pred_indices: (bool) = False,
+ target_indices: (bool) = False) -> float:
+ """Calculate the average recall.
+
+ Args:
+ pred (torch.Tensor | np.ndarray | Sequence): The prediction
+ results. A :obj:`torch.Tensor` or :obj:`np.ndarray` with
+ shape ``(N, M)`` or a sequence of index/onehot
+ format labels.
+ target (torch.Tensor | np.ndarray | Sequence): The prediction
+ results. A :obj:`torch.Tensor` or :obj:`np.ndarray` with
+ shape ``(N, M)`` or a sequence of index/onehot
+ format labels.
+ topk (int, Sequence[int]): Predictions with the k-th highest
+ scores are considered as positive.
+ pred_indices (bool): Whether the ``pred`` is a sequence of
+ category index labels. Defaults to False.
+ target_indices (bool): Whether the ``target`` is a sequence of
+ category index labels. Defaults to False.
+
+ Returns:
+ List[float]: the average recalls.
+ """
+ topk = (topk, ) if isinstance(topk, int) else topk
+ for k in topk:
+ if k <= 0:
+ raise ValueError('`topk` must be a ingter larger than 0 '
+ 'or seq of ingter larger than 0.')
+
+ max_keep = max(topk)
+ pred = _format_pred(pred, max_keep, pred_indices)
+ target = _format_target(target, target_indices)
+
+ assert len(pred) == len(target), (
+ f'Length of `pred`({len(pred)}) and `target` ({len(target)}) '
+ f'must be the same.')
+
+ num_samples = len(pred)
+ results = []
+ for k in topk:
+ recalls = torch.zeros(num_samples)
+ for i, (sample_pred,
+ sample_target) in enumerate(zip(pred, target)):
+ sample_pred = np.array(to_tensor(sample_pred).cpu())
+ sample_target = np.array(to_tensor(sample_target).cpu())
+ recalls[i] = int(np.in1d(sample_pred[:k], sample_target).max())
+ results.append(recalls.mean() * 100)
+ return results
+
+
+def _format_pred(label, topk=None, is_indices=False):
+ """format various label to List[indices]."""
+ if is_indices:
+ assert isinstance(label, Sequence), \
+ '`pred` must be Sequence of indices when' \
+ f' `pred_indices` set to True, but get {type(label)}'
+ for i, sample_pred in enumerate(label):
+ assert is_seq_of(sample_pred, int) or isinstance(
+ sample_pred, (np.ndarray, torch.Tensor)), \
+ '`pred` should be Sequence of indices when `pred_indices`' \
+ f'set to True. but pred[{i}] is {sample_pred}'
+ if topk:
+ label[i] = sample_pred[:min(topk, len(sample_pred))]
+ return label
+ if isinstance(label, np.ndarray):
+ label = torch.from_numpy(label)
+ elif not isinstance(label, torch.Tensor):
+ raise TypeError(f'The pred must be type of torch.tensor, '
+ f'np.ndarray or Sequence but get {type(label)}.')
+ topk = topk if topk else label.size()[-1]
+ _, indices = label.topk(topk)
+ return indices
+
+
+def _format_target(label, is_indices=False):
+ """format various label to List[indices]."""
+ if is_indices:
+ assert isinstance(label, Sequence), \
+ '`target` must be Sequence of indices when' \
+ f' `target_indices` set to True, but get {type(label)}'
+ for i, sample_gt in enumerate(label):
+ assert is_seq_of(sample_gt, int) or isinstance(
+ sample_gt, (np.ndarray, torch.Tensor)), \
+ '`target` should be Sequence of indices when ' \
+ f'`target_indices` set to True. but target[{i}] is {sample_gt}'
+ return label
+
+ if isinstance(label, np.ndarray):
+ label = torch.from_numpy(label)
+ elif isinstance(label, Sequence) and not mmengine.is_str(label):
+ label = torch.tensor(label)
+ elif not isinstance(label, torch.Tensor):
+ raise TypeError(f'The pred must be type of torch.tensor, '
+ f'np.ndarray or Sequence but get {type(label)}.')
+
+ indices = [sample_gt.nonzero().squeeze(-1) for sample_gt in label]
+ return indices
diff --git a/mmaction/evaluation/metrics/video_grounding_metric.py b/mmaction/evaluation/metrics/video_grounding_metric.py
new file mode 100644
index 0000000000..310db64452
--- /dev/null
+++ b/mmaction/evaluation/metrics/video_grounding_metric.py
@@ -0,0 +1,66 @@
+# Copyright (c) OpenMMLab. All rights reserved.
+from typing import Any, Optional, Sequence, Tuple
+
+from mmengine.evaluator import BaseMetric
+
+from mmaction.registry import METRICS
+
+
+@METRICS.register_module()
+class RecallatTopK(BaseMetric):
+ """ActivityNet dataset evaluation metric."""
+
+ def __init__(self,
+ topK_list: Tuple[int] = (1, 5),
+ threshold: float = 0.5,
+ collect_device: str = 'cpu',
+ prefix: Optional[str] = None):
+ super().__init__(collect_device=collect_device, prefix=prefix)
+ self.topK_list = topK_list
+ self.threshold = threshold
+
+ def process(self, data_batch: Sequence[Tuple[Any, dict]],
+ predictions: Sequence[dict]) -> None:
+ """Process one batch of data samples and predictions. The processed
+ results should be stored in ``self.results``, which will be used to
+ compute the metrics when all batches have been processed.
+
+ Args:
+ data_batch (Sequence[Tuple[Any, dict]]): A batch of data
+ from the dataloader.
+ predictions (Sequence[dict]): A batch of outputs from
+ the model.
+ """
+ for pred in predictions:
+ self.results.append(pred)
+
+ def compute_metrics(self, results: list) -> dict:
+ """Compute the metrics from processed results.
+
+ Args:
+ results (list): The processed results of each batch.
+ Returns:
+ dict: The computed metrics. The keys are the names of the metrics,
+ and the values are corresponding results.
+ """
+ eval_results = dict()
+ for topK in self.topK_list:
+ total = len(results)
+ correct = 0.0
+ for result in results:
+ gt = result['gt']
+ predictions = result['predictions'][:topK]
+ for prediction in predictions:
+ IoU = self.calculate_IoU(gt, prediction)
+ if IoU > self.threshold:
+ correct += 1
+ break
+ acc = correct / total
+ eval_results[f'Recall@Top{topK}_IoU={self.threshold}'] = acc
+ return eval_results
+
+ def calculate_IoU(self, i0, i1):
+ union = (min(i0[0], i1[0]), max(i0[1], i1[1]))
+ inter = (max(i0[0], i1[0]), min(i0[1], i1[1]))
+ iou = (inter[1] - inter[0]) / (union[1] - union[0])
+ return iou
diff --git a/mmaction/models/__init__.py b/mmaction/models/__init__.py
index 6c53b29254..08f7d41f52 100644
--- a/mmaction/models/__init__.py
+++ b/mmaction/models/__init__.py
@@ -5,6 +5,7 @@
from .heads import * # noqa: F401,F403
from .localizers import * # noqa: F401,F403
from .losses import * # noqa: F401,F403
+from .multimodal import * # noqa: F401,F403
from .necks import * # noqa: F401,F403
from .recognizers import * # noqa: F401,F403
from .roi_heads import * # noqa: F401,F403
diff --git a/mmaction/models/backbones/__init__.py b/mmaction/models/backbones/__init__.py
index 2f4eb4a7e3..8a69a057d6 100644
--- a/mmaction/models/backbones/__init__.py
+++ b/mmaction/models/backbones/__init__.py
@@ -33,3 +33,10 @@
'TimeSformer', 'UniFormer', 'UniFormerV2', 'VisionTransformer', 'X3D',
'RGBPoseConv3D'
]
+
+try:
+ from .mobileone_tsm import MobileOneTSM # noqa: F401
+ __all__.append('MobileOneTSM')
+
+except (ImportError, ModuleNotFoundError):
+ pass
diff --git a/mmaction/models/backbones/mobileone_tsm.py b/mmaction/models/backbones/mobileone_tsm.py
new file mode 100644
index 0000000000..96722faf68
--- /dev/null
+++ b/mmaction/models/backbones/mobileone_tsm.py
@@ -0,0 +1,140 @@
+# Copyright (c) OpenMMLab. All rights reserved.
+
+import torch.nn as nn
+from mmengine.logging import MMLogger
+from mmengine.runner.checkpoint import (_load_checkpoint,
+ _load_checkpoint_with_prefix)
+from mmpretrain.models import MobileOne
+
+from mmaction.registry import MODELS
+from .resnet_tsm import TemporalShift
+
+
+@MODELS.register_module()
+class MobileOneTSM(MobileOne):
+ """MobileOne backbone for TSM.
+
+ Args:
+ arch (str | dict): MobileOne architecture. If use string, choose
+ from 's0', 's1', 's2', 's3' and 's4'. If use dict, it should
+ have below keys:
+
+ - num_blocks (Sequence[int]): Number of blocks in each stage.
+ - width_factor (Sequence[float]): Width factor in each stage.
+ - num_conv_branches (Sequence[int]): Number of conv branches
+ in each stage.
+ - num_se_blocks (Sequence[int]): Number of SE layers in each
+ stage, all the SE layers are placed in the subsequent order
+ in each stage.
+
+ Defaults to 's0'.
+ num_segments (int): Number of frame segments. Defaults to 8.
+ is_shift (bool): Whether to make temporal shift in reset layers.
+ Defaults to True.
+ shift_div (int): Number of div for shift. Defaults to 8.
+ pretraind2d (bool): Whether to load pretrained 2D model.
+ Defaults to True.
+ **kwargs (keyword arguments, optional): Arguments for MobileOne.
+ """
+
+ def __init__(self,
+ arch: str,
+ num_segments: int = 8,
+ is_shift: bool = True,
+ shift_div: int = 8,
+ pretrained2d: bool = True,
+ **kwargs):
+ super().__init__(arch, **kwargs)
+ self.num_segments = num_segments
+ self.is_shift = is_shift
+ self.shift_div = shift_div
+ self.pretrained2d = pretrained2d
+ self.init_structure()
+
+ def make_temporal_shift(self):
+ """Make temporal shift for some layers.
+
+ To make reparameterization work, we can only build the shift layer
+ before the 'block', instead of the 'blockres'
+ """
+
+ def make_block_temporal(stage, num_segments):
+ """Make temporal shift on some blocks.
+
+ Args:
+ stage (nn.Module): Model layers to be shifted.
+ num_segments (int): Number of frame segments.
+
+ Returns:
+ nn.Module: The shifted blocks.
+ """
+ blocks = list(stage.children())
+ for i, b in enumerate(blocks):
+ blocks[i] = TemporalShift(
+ b, num_segments=num_segments, shift_div=self.shift_div)
+ return nn.Sequential(*blocks)
+
+ self.stage0 = make_block_temporal(
+ nn.Sequential(self.stage0), self.num_segments)[0]
+ for i in range(1, 5):
+ temporal_stage = make_block_temporal(
+ getattr(self, f'stage{i}'), self.num_segments)
+ setattr(self, f'stage{i}', temporal_stage)
+
+ def init_structure(self):
+ """Initiate the parameters either from existing checkpoint or from
+ scratch."""
+ if self.is_shift:
+ self.make_temporal_shift()
+
+ def load_original_weights(self, logger):
+ assert self.init_cfg.get('type') == 'Pretrained', (
+ 'Please specify '
+ 'init_cfg to use pretrained 2d checkpoint')
+ self.pretrained = self.init_cfg.get('checkpoint')
+ prefix = self.init_cfg.get('prefix')
+ if prefix is not None:
+ original_state_dict = _load_checkpoint_with_prefix(
+ prefix, self.pretrained, map_location='cpu')
+ else:
+ original_state_dict = _load_checkpoint(
+ self.pretrained, map_location='cpu')
+ if 'state_dict' in original_state_dict:
+ original_state_dict = original_state_dict['state_dict']
+
+ wrapped_layers_map = dict()
+ for name, module in self.named_modules():
+ ori_name = name
+ for wrap_prefix in ['.net']:
+ if wrap_prefix in ori_name:
+ ori_name = ori_name.replace(wrap_prefix, '')
+ wrapped_layers_map[ori_name] = name
+
+ # convert wrapped keys
+ for param_name in list(original_state_dict.keys()):
+ layer_name = '.'.join(param_name.split('.')[:-1])
+ if layer_name in wrapped_layers_map:
+ wrapped_name = param_name.replace(
+ layer_name, wrapped_layers_map[layer_name])
+ original_state_dict[wrapped_name] = original_state_dict.pop(
+ param_name)
+
+ msg = self.load_state_dict(original_state_dict, strict=True)
+ logger.info(msg)
+
+ def init_weights(self):
+ """Initiate the parameters either from existing checkpoint or from
+ scratch."""
+ if self.pretrained2d:
+ logger = MMLogger.get_current_instance()
+ self.load_original_weights(logger)
+ else:
+ super().init_weights()
+
+ def forward(self, x):
+ """unpack tuple result."""
+ x = super().forward(x)
+ if isinstance(x, tuple):
+ assert len(x) == 1
+ x = x[0]
+ return x
diff --git a/mmaction/models/backbones/vit_mae.py b/mmaction/models/backbones/vit_mae.py
index 31210beba2..e549122fbc 100644
--- a/mmaction/models/backbones/vit_mae.py
+++ b/mmaction/models/backbones/vit_mae.py
@@ -12,12 +12,6 @@
from mmaction.registry import MODELS
from mmaction.utils import ConfigType, OptConfigType
-try:
- from mmdet.registry import MODELS as MMDET_MODELS
- mmdet_imported = True
-except (ImportError, ModuleNotFoundError):
- mmdet_imported = False
-
class Attention(BaseModule):
"""Multi-head Self-attention.
@@ -387,7 +381,3 @@ def forward(self, x: Tensor) -> Tensor:
return self.fc_norm(x.mean(1))
return x[:, 0]
-
-
-if mmdet_imported:
- MMDET_MODELS.register_module()(VisionTransformer)
diff --git a/mmaction/models/data_preprocessors/data_preprocessor.py b/mmaction/models/data_preprocessors/data_preprocessor.py
index 891cb8f386..0376318ff7 100644
--- a/mmaction/models/data_preprocessors/data_preprocessor.py
+++ b/mmaction/models/data_preprocessors/data_preprocessor.py
@@ -84,7 +84,7 @@ def forward(self,
data = self.cast_data(data)
if isinstance(data, dict):
return self.forward_onesample(data, training=training)
- elif isinstance(data, tuple):
+ elif isinstance(data, (tuple, list)):
outputs = []
for data_sample in data:
output = self.forward_onesample(data_sample, training=training)
diff --git a/mmaction/models/heads/base.py b/mmaction/models/heads/base.py
index c39da5aa9a..8febe1df5b 100644
--- a/mmaction/models/heads/base.py
+++ b/mmaction/models/heads/base.py
@@ -6,7 +6,6 @@
import torch.nn as nn
import torch.nn.functional as F
from mmengine.model import BaseModule
-from mmengine.structures import LabelData
from mmaction.evaluation import top_k_accuracy
from mmaction.registry import MODELS
@@ -112,7 +111,7 @@ def loss_by_feat(self, cls_scores: torch.Tensor,
Returns:
dict: A dictionary of loss components.
"""
- labels = [x.gt_labels.item for x in data_samples]
+ labels = [x.gt_label for x in data_samples]
labels = torch.stack(labels).to(cls_scores.device)
labels = labels.squeeze()
@@ -175,7 +174,7 @@ def predict_by_feat(self, cls_scores: torch.Tensor,
(B*num_segs, num_classes)
data_samples (list[:obj:`ActionDataSample`]): The
annotation data of every samples. It usually includes
- information such as `gt_labels`.
+ information such as `gt_label`.
Returns:
List[:obj:`ActionDataSample`]: Recognition results wrapped
@@ -187,10 +186,8 @@ def predict_by_feat(self, cls_scores: torch.Tensor,
for data_sample, score, pred_label in zip(data_samples, cls_scores,
pred_labels):
- prediction = LabelData(item=score)
- pred_label = LabelData(item=pred_label)
- data_sample.pred_scores = prediction
- data_sample.pred_labels = pred_label
+ data_sample.set_pred_score(score)
+ data_sample.set_pred_label(pred_label)
return data_samples
def average_clip(self,
diff --git a/mmaction/models/heads/omni_head.py b/mmaction/models/heads/omni_head.py
index f5084dde06..7a62cf56da 100644
--- a/mmaction/models/heads/omni_head.py
+++ b/mmaction/models/heads/omni_head.py
@@ -87,10 +87,7 @@ def loss_by_feat(self, cls_scores: Union[Tensor, Tuple[Tensor]],
Returns:
dict: A dictionary of loss components.
"""
- if hasattr(data_samples[0], 'gt_labels'):
- labels = [x.gt_labels.item for x in data_samples]
- else:
- labels = [x.gt_label.label for x in data_samples]
+ labels = [x.gt_label for x in data_samples]
labels = torch.stack(labels).to(cls_scores.device)
labels = labels.squeeze()
diff --git a/mmaction/models/heads/rgbpose_head.py b/mmaction/models/heads/rgbpose_head.py
index 69da4efed9..880e37f084 100644
--- a/mmaction/models/heads/rgbpose_head.py
+++ b/mmaction/models/heads/rgbpose_head.py
@@ -5,7 +5,6 @@
import torch.nn as nn
import torch.nn.functional as F
from mmengine.model.weight_init import normal_init
-from mmengine.structures import LabelData
from mmaction.evaluation import top_k_accuracy
from mmaction.registry import MODELS
@@ -110,7 +109,7 @@ def loss_by_feat(self, cls_scores: Dict[str, torch.Tensor],
Returns:
dict: A dictionary of loss components.
"""
- labels = torch.stack([x.gt_labels.item for x in data_samples])
+ labels = torch.stack([x.gt_label for x in data_samples])
labels = labels.squeeze()
if labels.shape == torch.Size([]):
@@ -192,34 +191,26 @@ def predict_by_feat(self, cls_scores: Dict[str, torch.Tensor],
classification scores,
data_samples (list[:obj:`ActionDataSample`]): The
annotation data of every samples. It usually includes
- information such as `gt_labels`.
+ information such as `gt_label`.
Returns:
list[:obj:`ActionDataSample`]: Recognition results wrapped
by :obj:`ActionDataSample`.
"""
- pred_scores = [LabelData() for _ in range(len(data_samples))]
- pred_labels = [LabelData() for _ in range(len(data_samples))]
+ pred_scores = [dict() for _ in range(len(data_samples))]
for name in self.loss_components:
cls_score = cls_scores[name]
- cls_score, pred_label = \
- self.predict_by_scores(cls_score, data_samples)
- for pred_score, pred_label, score, label in zip(
- pred_scores, pred_labels, cls_score, pred_label):
- pred_score.set_data({f'{name}': score})
- pred_label.set_data({f'{name}': label})
-
- for data_sample, pred_score, pred_label in zip(data_samples,
- pred_scores,
- pred_labels):
- data_sample.pred_scores = pred_score
- data_sample.pred_labels = pred_label
+ cls_score = self.predict_by_scores(cls_score, data_samples)
+ for pred_score, score in zip(pred_scores, cls_score):
+ pred_score[f'{name}'] = score
+ for data_sample, pred_score, in zip(data_samples, pred_scores):
+ data_sample.set_pred_score(pred_score)
return data_samples
def predict_by_scores(self, cls_scores: torch.Tensor,
- data_samples: SampleList) -> Tuple:
+ data_samples: SampleList) -> torch.Tensor:
"""Transform a batch of output features extracted from the head into
prediction results.
@@ -230,11 +221,9 @@ def predict_by_scores(self, cls_scores: torch.Tensor,
data of every samples.
Returns:
- tuple: A tuple of the averaged classification scores and
- prediction labels.
+ torch.Tensor: The averaged classification scores.
"""
num_segs = cls_scores.shape[0] // len(data_samples)
cls_scores = self.average_clip(cls_scores, num_segs=num_segs)
- pred_labels = cls_scores.argmax(dim=-1, keepdim=True).detach()
- return cls_scores, pred_labels
+ return cls_scores
diff --git a/mmaction/models/heads/tpn_head.py b/mmaction/models/heads/tpn_head.py
index 6f32f65109..fb2fa4e907 100644
--- a/mmaction/models/heads/tpn_head.py
+++ b/mmaction/models/heads/tpn_head.py
@@ -2,6 +2,7 @@
from typing import Optional
import torch.nn as nn
+from mmengine.device import get_device
from torch import Tensor
from mmaction.registry import MODELS
@@ -26,8 +27,7 @@ def __init__(self, *args, **kwargs) -> None:
def _init_new_cls(self) -> None:
self.new_cls = nn.Conv3d(self.in_channels, self.num_classes, 1, 1, 0)
- if next(self.fc_cls.parameters()).is_cuda:
- self.new_cls = self.new_cls.cuda()
+ self.new_cls = self.new_cls.to(get_device())
self.new_cls.weight.copy_(self.fc_cls.weight[..., None, None, None])
self.new_cls.bias.copy_(self.fc_cls.bias)
diff --git a/mmaction/models/localizers/__init__.py b/mmaction/models/localizers/__init__.py
index 26e016410b..debd9a16f4 100644
--- a/mmaction/models/localizers/__init__.py
+++ b/mmaction/models/localizers/__init__.py
@@ -1,6 +1,7 @@
# Copyright (c) OpenMMLab. All rights reserved.
from .bmn import BMN
from .bsn import PEM, TEM
+from .drn.drn import DRN
from .tcanet import TCANet
-__all__ = ['TEM', 'PEM', 'BMN', 'TCANet']
+__all__ = ['TEM', 'PEM', 'BMN', 'TCANet', 'DRN']
diff --git a/mmaction/models/localizers/drn/drn.py b/mmaction/models/localizers/drn/drn.py
new file mode 100644
index 0000000000..869791e6bb
--- /dev/null
+++ b/mmaction/models/localizers/drn/drn.py
@@ -0,0 +1,260 @@
+# Copyright (c) OpenMMLab. All rights reserved.
+from typing import Sequence
+
+import numpy as np
+import torch
+import torch.nn as nn
+from mmengine.model import BaseModel
+
+from mmaction.registry import MODELS
+from mmaction.utils import OptConfigType
+from ..utils import soft_nms
+from .drn_utils import FPN, Backbone, FCOSModule, QueryEncoder
+
+
+@MODELS.register_module()
+class DRN(BaseModel):
+ """Dense Regression Network for Video Grounding.
+
+ Please refer `Dense Regression Network for Video Grounding
+ `_.
+ Code Reference: https://github.com/Alvin-Zeng/DRN
+
+ Args:
+ vocab_size (int): number of all possible words in the query.
+ Defaults to 1301.
+ hidden_dim (int): the hidden dimension of the LSTM in the
+ language model. Defaults to 512.
+ embed_dim (int): the embedding dimension of the query. Defaults
+ to 300.
+ bidirection (bool): if True, use bi-direction LSTM in the
+ language model. Defaults to True.
+ first_output_dim (int): the output dimension of the first layer
+ in the backbone. Defaults to 256.
+ fpn_feature_dim (int): the output dimension of the FPN. Defaults
+ to 512.
+ feature_dim (int): the dimension of the video clip feature.
+ lstm_layers (int): the number of LSTM layers in the language model.
+ Defaults to 1.
+ fcos_pre_nms_top_n (int): value of Top-N in the FCOS module before
+ nms. Defaults to 32.
+ fcos_inference_thr (float): threshold in the FOCS inference. BBoxes
+ with scores higher than this threshold are regarded as positive.
+ Defaults to 0.05.
+ fcos_prior_prob (float): A prior probability of the positive bboexes.
+ Used to initialized the bias of the classification head.
+ Defaults to 0.01.
+ focal_alpha (float):Focal loss hyper-parameter alpha.
+ Defaults to 0.25.
+ focal_gamma (float): Focal loss hyper-parameter gamma.
+ Defaults to 2.0.
+ fpn_stride (Sequence[int]): the strides in the FPN. Defaults to
+ [1, 2, 4].
+ fcos_nms_thr (float): NMS threshold in the FOCS module.
+ Defaults to 0.6.
+ fcos_conv_layers (int): number of convolution layers in FCOS.
+ Defaults to 1.
+ fcos_num_class (int): number of classes in FCOS.
+ Defaults to 2.
+ is_first_stage (bool): if true, the model is in the first stage
+ training.
+ is_second_stage (bool): if true, the model is in the second stage
+ training.
+ """
+
+ def __init__(self,
+ vocab_size: int = 1301,
+ hidden_dim: int = 512,
+ embed_dim: int = 300,
+ bidirection: bool = True,
+ first_output_dim: int = 256,
+ fpn_feature_dim: int = 512,
+ feature_dim: int = 4096,
+ lstm_layers: int = 1,
+ fcos_pre_nms_top_n: int = 32,
+ fcos_inference_thr: float = 0.05,
+ fcos_prior_prob: float = 0.01,
+ focal_alpha: float = 0.25,
+ focal_gamma: float = 2.0,
+ fpn_stride: Sequence[int] = [1, 2, 4],
+ fcos_nms_thr: float = 0.6,
+ fcos_conv_layers: int = 1,
+ fcos_num_class: int = 2,
+ is_first_stage: bool = False,
+ is_second_stage: bool = False,
+ init_cfg: OptConfigType = None,
+ **kwargs) -> None:
+ super(DRN, self).__init__(init_cfg)
+
+ self.query_encoder = QueryEncoder(
+ vocab_size=vocab_size,
+ hidden_dim=hidden_dim,
+ embed_dim=embed_dim,
+ num_layers=lstm_layers,
+ bidirection=bidirection)
+
+ channels_list = [
+ (feature_dim + 256, first_output_dim, 3, 1),
+ (first_output_dim, first_output_dim * 2, 3, 2),
+ (first_output_dim * 2, first_output_dim * 4, 3, 2),
+ ]
+ self.backbone_net = Backbone(channels_list)
+
+ self.fpn = FPN(
+ in_channels_list=[256, 512, 1024], out_channels=fpn_feature_dim)
+
+ self.fcos = FCOSModule(
+ in_channels=fpn_feature_dim,
+ fcos_num_class=fcos_num_class,
+ fcos_conv_layers=fcos_conv_layers,
+ fcos_prior_prob=fcos_prior_prob,
+ fcos_inference_thr=fcos_inference_thr,
+ fcos_pre_nms_top_n=fcos_pre_nms_top_n,
+ fcos_nms_thr=fcos_nms_thr,
+ test_detections_per_img=32,
+ fpn_stride=fpn_stride,
+ focal_alpha=focal_alpha,
+ focal_gamma=focal_gamma,
+ is_first_stage=is_first_stage,
+ is_second_stage=is_second_stage)
+
+ self.prop_fc = nn.Linear(feature_dim, feature_dim)
+ self.position_transform = nn.Linear(3, 256)
+
+ qInput = []
+ for t in range(len(channels_list)):
+ if t > 0:
+ qInput += [nn.Linear(1024, channels_list[t - 1][1])]
+ else:
+ qInput += [nn.Linear(1024, feature_dim)]
+ self.qInput = nn.ModuleList(qInput)
+
+ self.is_second_stage = is_second_stage
+
+ def forward(self, inputs, data_samples, mode, **kwargs):
+ props_features = torch.stack(inputs)
+ batch_size = props_features.shape[0]
+ device = props_features.device
+ proposals = torch.stack([
+ sample.proposals['proposals'] for sample in data_samples
+ ]).to(device)
+ gt_bbox = torch.stack([
+ sample.gt_instances['gt_bbox'] for sample in data_samples
+ ]).to(device)
+
+ video_info = [i.metainfo for i in data_samples]
+ query_tokens_ = [i['query_tokens'] for i in video_info]
+ query_length = [i['query_length'] for i in video_info]
+ query_length = torch.from_numpy(np.array(query_length))
+
+ max_query_len = max([i.shape[0] for i in query_tokens_])
+ query_tokens = torch.zeros(batch_size, max_query_len)
+ for idx, query_token in enumerate(query_tokens_):
+ query_len = query_token.shape[0]
+ query_tokens[idx, :query_len] = query_token
+
+ query_tokens = query_tokens.to(device).long()
+ query_length = query_length.to(device).long() # should be on CPU!
+
+ sort_index = query_length.argsort(descending=True)
+ box_lists, loss_dict = self._forward(query_tokens[sort_index],
+ query_length[sort_index],
+ props_features[sort_index],
+ proposals[sort_index],
+ gt_bbox[sort_index])
+ if mode == 'loss':
+ return loss_dict
+ elif mode == 'predict':
+ # only support batch size = 1
+ bbox = box_lists[0]
+
+ per_vid_detections = bbox['detections']
+ per_vid_scores = bbox['scores']
+
+ props_pred = torch.cat(
+ (per_vid_detections, per_vid_scores.unsqueeze(-1)), dim=-1)
+
+ props_pred = props_pred.cpu().numpy()
+ props_pred = sorted(props_pred, key=lambda x: x[-1], reverse=True)
+ props_pred = np.array(props_pred)
+
+ props_pred = soft_nms(
+ props_pred,
+ alpha=0.4,
+ low_threshold=0.5,
+ high_threshold=0.9,
+ top_k=5)
+ result = {
+ 'vid_name': data_samples[0].metainfo['vid_name'],
+ 'gt': gt_bbox[0].cpu().numpy(),
+ 'predictions': props_pred,
+ }
+ return [result]
+
+ raise ValueError(f'Unsupported mode {mode}!')
+
+ def nms_temporal(self, start, end, score, overlap=0.45):
+ pick = []
+ assert len(start) == len(score)
+ assert len(end) == len(score)
+ if len(start) == 0:
+ return pick
+
+ union = end - start
+ # sort and get index
+ intervals = [
+ i[0] for i in sorted(enumerate(score), key=lambda x: x[1])
+ ]
+
+ while len(intervals) > 0:
+ i = intervals[-1]
+ pick.append(i)
+
+ xx1 = [max(start[i], start[j]) for j in intervals[:-1]]
+ xx2 = [min(end[i], end[j]) for j in intervals[:-1]]
+ inter = [max(0., k2 - k1) for k1, k2 in zip(xx1, xx2)]
+ o = [
+ inter[u] / (union[i] + union[intervals[u]] - inter[u])
+ for u in range(len(intervals) - 1)
+ ]
+ I_new = []
+ for j in range(len(o)):
+ if o[j] <= overlap:
+ I_new.append(intervals[j])
+ intervals = I_new
+ return np.array(pick)
+
+ def _forward(self, query_tokens, query_length, props_features,
+ props_start_end, gt_bbox):
+
+ position_info = [props_start_end, props_start_end]
+ position_feats = []
+ query_features = self.query_encoder(query_tokens, query_length)
+ for i in range(len(query_features)):
+ query_features[i] = self.qInput[i](query_features[i])
+ if i > 1:
+ position_info.append(
+ torch.cat([
+ props_start_end[:, ::2 * (i - 1), [0]],
+ props_start_end[:, 1::2 * (i - 1), [1]]
+ ],
+ dim=-1))
+ props_duration = position_info[i][:, :, 1] - position_info[i][:, :,
+ 0]
+ props_duration = props_duration.unsqueeze(-1)
+ position_feat = torch.cat((position_info[i], props_duration),
+ dim=-1).float()
+ position_feats.append(
+ self.position_transform(position_feat).permute(0, 2, 1))
+
+ props_features = self.prop_fc(props_features)
+
+ inputs = props_features.permute(0, 2, 1)
+ outputs = self.backbone_net(inputs, query_features, position_feats)
+ outputs = self.fpn(outputs)
+
+ if self.is_second_stage:
+ outputs = [_.detach() for _ in outputs]
+ box_lists, loss_dict = self.fcos(outputs, gt_bbox.float())
+
+ return box_lists, loss_dict
diff --git a/mmaction/models/localizers/drn/drn_utils/FPN.py b/mmaction/models/localizers/drn/drn_utils/FPN.py
new file mode 100644
index 0000000000..1170ac5cf3
--- /dev/null
+++ b/mmaction/models/localizers/drn/drn_utils/FPN.py
@@ -0,0 +1,44 @@
+# Copyright (c) OpenMMLab. All rights reserved.
+from typing import List, Tuple
+
+import torch.nn.functional as F
+from torch import Tensor, nn
+
+from .backbone import conv_block
+
+
+class FPN(nn.Module):
+
+ def __init__(self, in_channels_list: List, out_channels: int) -> None:
+ super(FPN, self).__init__()
+
+ inner_blocks = []
+ layer_blocks = []
+ for idx, in_channels in enumerate(in_channels_list, 1):
+ inner_block = conv_block(in_channels, out_channels, 1, 1)
+ layer_block = conv_block(out_channels, out_channels, 3, 1)
+
+ inner_blocks.append(inner_block)
+ layer_blocks.append(layer_block)
+
+ self.inner_blocks = nn.ModuleList(inner_blocks)
+ self.layer_blocks = nn.ModuleList(layer_blocks)
+
+ def forward(self, x: Tensor) -> Tuple[Tensor]:
+ # process the last lowest resolution feat and
+ # first feed it into 1 x 1 conv
+ last_inner = self.inner_blocks[-1](x[-1])
+ results = [self.layer_blocks[-1](last_inner)]
+
+ for feature, inner_block, layer_block in zip(
+ x[:-1][::-1], self.inner_blocks[:-1][::-1],
+ self.layer_blocks[:-1][::-1]):
+ if not inner_block:
+ continue
+ inner_top_down = F.interpolate(
+ last_inner, scale_factor=2, mode='nearest')
+ inner_lateral = inner_block(feature)
+ last_inner = inner_lateral + inner_top_down
+ results.insert(0, layer_block(last_inner))
+
+ return tuple(results)
diff --git a/mmaction/models/localizers/drn/drn_utils/__init__.py b/mmaction/models/localizers/drn/drn_utils/__init__.py
new file mode 100644
index 0000000000..4d371a5055
--- /dev/null
+++ b/mmaction/models/localizers/drn/drn_utils/__init__.py
@@ -0,0 +1,7 @@
+# Copyright (c) OpenMMLab. All rights reserved.
+from .backbone import Backbone
+from .fcos import FCOSModule
+from .FPN import FPN
+from .language_module import QueryEncoder
+
+__all__ = ['Backbone', 'FPN', 'QueryEncoder', 'FCOSModule']
diff --git a/mmaction/models/localizers/drn/drn_utils/backbone.py b/mmaction/models/localizers/drn/drn_utils/backbone.py
new file mode 100644
index 0000000000..ac2c6338d0
--- /dev/null
+++ b/mmaction/models/localizers/drn/drn_utils/backbone.py
@@ -0,0 +1,48 @@
+# Copyright (c) OpenMMLab. All rights reserved.
+from typing import List, Tuple
+
+import torch
+from torch import Tensor, nn
+
+
+def conv_block(in_channels: int,
+ out_channels: int,
+ kernel_size: int = 3,
+ stride: int = 1) -> nn.Module:
+ module = nn.Sequential(
+ nn.Conv1d(
+ in_channels,
+ out_channels,
+ kernel_size=kernel_size,
+ stride=stride,
+ padding=(kernel_size - 1) // 2,
+ bias=False), nn.BatchNorm1d(out_channels), nn.ReLU())
+ return module
+
+
+class Backbone(nn.Module):
+
+ def __init__(self, channels_list: List[tuple]) -> None:
+ super(Backbone, self).__init__()
+
+ self.num_layers = len(channels_list)
+ layers = []
+ for idx, channels_config in enumerate(channels_list):
+ layer = conv_block(*channels_config)
+ layers.append(layer)
+ self.layers = nn.ModuleList(layers)
+
+ def forward(self, x: Tensor, query_fts: Tensor,
+ position_fts: Tensor) -> Tuple[Tensor]:
+ results = []
+
+ for idx in range(self.num_layers):
+ query_ft = query_fts[idx].unsqueeze(1).permute(0, 2, 1)
+ position_ft = position_fts[idx]
+ x = query_ft * x
+ if idx == 0:
+ x = torch.cat([x, position_ft], dim=1)
+ x = self.layers[idx](x)
+ results.append(x)
+
+ return tuple(results)
diff --git a/mmaction/models/localizers/drn/drn_utils/fcos.py b/mmaction/models/localizers/drn/drn_utils/fcos.py
new file mode 100644
index 0000000000..33b30c4cb1
--- /dev/null
+++ b/mmaction/models/localizers/drn/drn_utils/fcos.py
@@ -0,0 +1,192 @@
+# Copyright (c) OpenMMLab. All rights reserved.
+import math
+
+import torch
+from torch import nn
+
+from .inference import make_fcos_postprocessor
+from .loss import make_fcos_loss_evaluator
+
+
+class Scale(nn.Module):
+
+ def __init__(self, init_value=1.0):
+ super(Scale, self).__init__()
+ self.scale = nn.Parameter(torch.FloatTensor([init_value]))
+
+ def forward(self, x):
+ return x * self.scale
+
+
+class FCOSHead(torch.nn.Module):
+
+ def __init__(self, in_channels: int, fcos_num_class: int,
+ fcos_conv_layers: int, fcos_prior_prob: float,
+ is_second_stage: bool) -> None:
+ super(FCOSHead, self).__init__()
+ num_classes = fcos_num_class - 1
+
+ cls_tower = []
+ bbox_tower = []
+ for i in range(fcos_conv_layers):
+ cls_tower.append(
+ nn.Conv1d(
+ in_channels,
+ in_channels,
+ kernel_size=3,
+ stride=1,
+ padding=1))
+ cls_tower.append(nn.BatchNorm1d(in_channels))
+ cls_tower.append(nn.ReLU())
+ bbox_tower.append(
+ nn.Conv1d(
+ in_channels,
+ in_channels,
+ kernel_size=3,
+ stride=1,
+ padding=1))
+ bbox_tower.append(nn.BatchNorm1d(in_channels))
+ bbox_tower.append(nn.ReLU())
+
+ self.cls_tower = nn.Sequential(*cls_tower)
+ self.bbox_tower = nn.Sequential(*bbox_tower)
+ self.cls_logits = nn.Conv1d(
+ in_channels, num_classes, kernel_size=3, stride=1, padding=1)
+
+ self.bbox_pred = nn.Conv1d(
+ in_channels, 2, kernel_size=3, stride=1, padding=1)
+
+ self.mix_fc = nn.Sequential(
+ nn.Conv1d(2 * in_channels, in_channels, kernel_size=1, stride=1),
+ nn.BatchNorm1d(in_channels), nn.ReLU())
+
+ self.iou_scores = nn.Sequential(
+ nn.Conv1d(
+ in_channels,
+ in_channels // 2,
+ kernel_size=3,
+ stride=1,
+ padding=1),
+ nn.BatchNorm1d(in_channels // 2),
+ nn.ReLU(),
+ nn.Conv1d(in_channels // 2, 1, kernel_size=1, stride=1),
+ )
+
+ # initialization
+ for module in self.modules():
+ if isinstance(module, nn.Conv1d):
+ torch.nn.init.normal_(module.weight, std=0.01)
+ torch.nn.init.constant_(module.bias, 0)
+
+ # initialize the bias for focal loss
+ bias_value = -math.log((1 - fcos_prior_prob) / fcos_prior_prob)
+ torch.nn.init.constant_(self.cls_logits.bias, bias_value)
+
+ self.scales = nn.ModuleList([Scale(init_value=1.0) for _ in range(3)])
+ self.is_second_stage = is_second_stage
+
+ def forward(self, x):
+ logits = []
+ bbox_reg = []
+ iou_scores = []
+ for idx, feature in enumerate(x):
+ cls_tower = self.cls_tower(feature)
+ box_tower = self.bbox_tower(feature)
+ logits.append(self.cls_logits(cls_tower))
+
+ bbox_reg_ = torch.exp(self.scales[idx](self.bbox_pred(box_tower)))
+ if self.is_second_stage:
+ bbox_reg_ = bbox_reg_.detach()
+ bbox_reg.append(bbox_reg_)
+
+ mix_feature = torch.cat([cls_tower, box_tower], dim=1)
+ if self.is_second_stage:
+ mix_feature = mix_feature.detach()
+ mix_feature = self.mix_fc(mix_feature)
+ iou_scores.append(self.iou_scores(mix_feature))
+ return logits, bbox_reg, iou_scores
+
+
+class FCOSModule(torch.nn.Module):
+
+ def __init__(self, in_channels: int, fcos_num_class: int,
+ fcos_conv_layers: int, fcos_prior_prob: float,
+ fcos_inference_thr: float, fcos_pre_nms_top_n: int,
+ fcos_nms_thr: float, test_detections_per_img: int,
+ fpn_stride: int, focal_alpha: float, focal_gamma: float,
+ is_first_stage: bool, is_second_stage: bool) -> None:
+ super(FCOSModule, self).__init__()
+
+ head = FCOSHead(
+ in_channels=in_channels,
+ fcos_num_class=fcos_num_class,
+ fcos_conv_layers=fcos_conv_layers,
+ fcos_prior_prob=fcos_prior_prob,
+ is_second_stage=is_second_stage)
+
+ self.is_first_stage = is_first_stage
+ self.is_second_stage = is_second_stage
+ box_selector_test = make_fcos_postprocessor(fcos_num_class,
+ fcos_inference_thr,
+ fcos_pre_nms_top_n,
+ fcos_nms_thr,
+ test_detections_per_img,
+ is_first_stage)
+ loss_evaluator = make_fcos_loss_evaluator(focal_alpha, focal_gamma)
+ self.head = head
+ self.box_selector_test = box_selector_test
+ self.loss_evaluator = loss_evaluator
+ self.fpn_strides = fpn_stride
+
+ def forward(self, features, targets=None):
+ box_cls, box_regression, iou_scores = self.head(features)
+ locations = self.compute_locations(features)
+
+ if self.training:
+ return self._forward_train(locations, box_cls, box_regression,
+ targets, iou_scores)
+ else:
+ return self._forward_test(locations, box_cls, box_regression,
+ targets, iou_scores)
+
+ def _forward_train(self, locations, box_cls, box_regression, targets,
+ iou_scores):
+ loss_box_cls, loss_box_reg, loss_iou = self.loss_evaluator(
+ locations, box_cls, box_regression, targets, iou_scores,
+ self.is_first_stage)
+
+ if self.is_second_stage:
+ loss_box_cls = loss_box_cls.detach()
+ loss_box_reg = loss_box_reg.detach()
+ if self.is_first_stage:
+ loss_iou = loss_iou.detach()
+
+ losses = {
+ 'loss_cls': loss_box_cls,
+ 'loss_reg': loss_box_reg,
+ 'loss_iou': loss_iou
+ }
+ return None, losses
+
+ def _forward_test(self, locations, box_cls, box_regression, targets,
+ iou_scores):
+ boxes = self.box_selector_test(locations, box_cls, box_regression,
+ iou_scores)
+ losses = None
+ return boxes, losses
+
+ def compute_locations(self, features):
+ locations = []
+ for level, feature in enumerate(features):
+ t = feature.size(-1)
+ locations_per_level = self.compute_locations_per_level(
+ t, self.fpn_strides[level], feature.device)
+ locations.append(locations_per_level)
+ return locations
+
+ def compute_locations_per_level(self, t, stride, device):
+ shifts_t = torch.arange(
+ 0, t * stride, step=stride, dtype=torch.float32, device=device)
+ shifts_t = shifts_t.reshape(-1)
+ locations = shifts_t + stride / 2
+ return locations
diff --git a/mmaction/models/localizers/drn/drn_utils/inference.py b/mmaction/models/localizers/drn/drn_utils/inference.py
new file mode 100644
index 0000000000..09cc7ef989
--- /dev/null
+++ b/mmaction/models/localizers/drn/drn_utils/inference.py
@@ -0,0 +1,212 @@
+# Copyright (c) OpenMMLab. All rights reserved.
+"""Copied from https://github.com/Alvin-Zeng/DRN/"""
+
+import torch
+
+
+class FCOSPostProcessor(torch.nn.Module):
+ """Performs post-processing on the outputs of the RetinaNet boxes.
+
+ This is only used in the testing.
+ """
+
+ def __init__(self, pre_nms_thresh, pre_nms_top_n, nms_thresh,
+ fpn_post_nms_top_n, min_size, num_classes, is_first_stage):
+ """
+ Arguments:
+ pre_nms_thresh (float)
+ pre_nms_top_n (int)
+ nms_thresh (float)
+ fpn_post_nms_top_n (int)
+ min_size (int)
+ num_classes (int)
+ box_coder (BoxCoder)
+ """
+ super(FCOSPostProcessor, self).__init__()
+ self.pre_nms_thresh = pre_nms_thresh
+ self.pre_nms_top_n = pre_nms_top_n
+ self.nms_thresh = nms_thresh
+ self.fpn_post_nms_top_n = fpn_post_nms_top_n
+ self.min_size = min_size
+ self.num_classes = num_classes
+ self.innerness_threshold = 0.15
+ self.downsample_scale = 32
+ self.is_first_stage = is_first_stage
+
+ def forward_for_single_feature_map(self, locations, box_cls,
+ box_regression, level, iou_scores):
+ """
+ Arguments:
+ anchors: list[BoxList]
+ box_cls: tensor of size N, A * C, H, W
+ box_regression: tensor of size N, A * 4, H, W
+ """
+ N, C, T = box_cls.shape
+
+ # put in the same format as locations
+ box_cls = box_cls.permute(0, 2, 1).contiguous().sigmoid()
+ iou_scores = iou_scores.permute(0, 2, 1).contiguous().sigmoid()
+ box_regression = box_regression.permute(0, 2, 1)
+
+ # centerness = centerness.permute(0, 2, 1)
+ # centerness = centerness.reshape(N, -1).sigmoid()
+ # inner = inner.squeeze().sigmoid()
+
+ candidate_inds = (box_cls > self.pre_nms_thresh)
+ pre_nms_top_n = candidate_inds.view(N, -1).sum(1)
+ pre_nms_top_n = pre_nms_top_n.clamp(max=self.pre_nms_top_n)
+
+ # multiply the classification scores with centerness scores
+ # box_cls = box_cls * centerness[:, :, None]
+ # box_cls = box_cls + centerness[:, :, None]
+ if not self.is_first_stage:
+ box_cls = box_cls * iou_scores
+
+ results = []
+ for i in range(N):
+
+ # per_centerness = centerness[i]
+
+ per_box_cls = box_cls[i]
+ per_candidate_inds = candidate_inds[i]
+ per_box_cls = per_box_cls[per_candidate_inds]
+
+ per_candidate_nonzeros = per_candidate_inds.nonzero()
+ per_box_loc = per_candidate_nonzeros[:, 0]
+ per_class = per_candidate_nonzeros[:, 1] + 1
+
+ per_box_regression = box_regression[i]
+ per_box_regression = per_box_regression[per_box_loc]
+ per_locations = locations[per_box_loc]
+
+ # per_centerness = per_centerness[per_box_loc]
+
+ per_pre_nms_top_n = pre_nms_top_n[i]
+
+ if per_candidate_inds.sum().item() > per_pre_nms_top_n.item():
+ per_box_cls, top_k_indices = \
+ per_box_cls.topk(per_pre_nms_top_n, sorted=False)
+ per_class = per_class[top_k_indices]
+ per_box_regression = per_box_regression[top_k_indices]
+ per_locations = per_locations[top_k_indices]
+
+ # per_centerness = per_centerness[top_k_indices]
+
+ detections = torch.stack([
+ per_locations - per_box_regression[:, 0],
+ per_locations + per_box_regression[:, 1],
+ ],
+ dim=1) / self.downsample_scale
+
+ detections[:, 0].clamp_(min=0, max=1)
+ detections[:, 1].clamp_(min=0, max=1)
+
+ # remove small boxes
+ p_start, p_end = detections.unbind(dim=1)
+ duration = p_end - p_start
+ keep = (duration >= self.min_size).nonzero().squeeze(1)
+ detections = detections[keep]
+
+ temp_dict = {}
+ temp_dict['detections'] = detections
+ temp_dict['labels'] = per_class
+ temp_dict['scores'] = torch.sqrt(per_box_cls)
+ temp_dict['level'] = [level]
+ # temp_dict['centerness'] = per_centerness
+ temp_dict['locations'] = per_locations / 32
+
+ results.append(temp_dict)
+
+ return results
+
+ def forward(self, locations, box_cls, box_regression, iou_scores):
+ """
+ Arguments:
+ anchors: list[list[BoxList]]
+ box_cls: list[tensor]
+ box_regression: list[tensor]
+ image_sizes: list[(h, w)]
+ Returns:
+ boxlists (list[BoxList]): the post-processed anchors, after
+ applying box decoding and NMS
+ """
+ sampled_boxes = []
+ for i, (l, o, b, iou_s) in enumerate(
+ zip(locations, box_cls, box_regression, iou_scores)):
+ sampled_boxes.append(
+ self.forward_for_single_feature_map(l, o, b, i, iou_s))
+
+ boxlists = list(zip(*sampled_boxes))
+ # boxlists = [cat_boxlist(boxlist) for boxlist in boxlists]
+ boxlists = self.select_over_all_levels(boxlists)
+
+ return boxlists
+
+ # TODO very similar to filter_results from PostProcessor
+ # but filter_results is per image
+ # TODO Yang: solve this issue in the future. No good solution
+ # right now.
+ def select_over_all_levels(self, boxlists):
+ num_images = len(boxlists)
+ results = []
+ for i in range(num_images):
+ dicts = boxlists[i]
+ per_vid_scores = []
+ per_vid_detections = []
+ per_vid_labels = []
+ # add level number
+ per_vid_level = []
+ per_vid_locations = []
+ # per_vid_centerness = []
+ for per_scale_dict in dicts:
+ if len(per_scale_dict['detections']) != 0:
+ per_vid_detections.append(per_scale_dict['detections'])
+ if len(per_scale_dict['scores']) != 0:
+ per_vid_scores.append(per_scale_dict['scores'])
+ if len(per_scale_dict['level']) != 0:
+ per_vid_level.append(per_scale_dict['level'] *
+ len(per_scale_dict['detections']))
+
+ if len(per_scale_dict['locations']) != 0:
+ per_vid_locations.append(per_scale_dict['locations'])
+
+ # if len(per_scale_dict['centerness']) != 0:
+ # per_vid_centerness.append(per_scale_dict['centerness'])
+ if len(per_vid_detections) == 0:
+ per_vid_detections = torch.Tensor([0, 1]).unsqueeze(0)
+ per_vid_scores = torch.Tensor([1])
+ per_vid_level = [[-1]]
+ per_vid_locations = torch.Tensor([0.5])
+ # per_vid_centerness = torch.Tensor([0.5]).cuda()
+ else:
+ per_vid_detections = torch.cat(per_vid_detections, dim=0)
+ per_vid_scores = torch.cat(per_vid_scores, dim=0)
+ per_vid_level = per_vid_level
+ per_vid_locations = torch.cat(per_vid_locations, dim=0)
+ # per_vid_centerness = torch.cat(per_vid_centerness, dim=0)
+
+ temp_dict = {}
+ temp_dict['detections'] = per_vid_detections
+ temp_dict['labels'] = per_vid_labels
+ temp_dict['scores'] = per_vid_scores
+ temp_dict['level'] = per_vid_level
+ # temp_dict['centerness'] = per_vid_centerness
+ temp_dict['locations'] = per_vid_locations
+ results.append(temp_dict)
+
+ return results
+
+
+def make_fcos_postprocessor(fcos_num_class, fcos_inference_thr,
+ fcos_pre_nms_top_n, fcos_nms_thr,
+ test_detections_per_img, is_first_stage):
+ box_selector = FCOSPostProcessor(
+ pre_nms_thresh=fcos_inference_thr,
+ pre_nms_top_n=fcos_pre_nms_top_n,
+ nms_thresh=fcos_nms_thr,
+ fpn_post_nms_top_n=test_detections_per_img,
+ min_size=0,
+ num_classes=fcos_num_class,
+ is_first_stage=is_first_stage)
+
+ return box_selector
diff --git a/mmaction/models/localizers/drn/drn_utils/language_module.py b/mmaction/models/localizers/drn/drn_utils/language_module.py
new file mode 100644
index 0000000000..135652a5eb
--- /dev/null
+++ b/mmaction/models/localizers/drn/drn_utils/language_module.py
@@ -0,0 +1,92 @@
+# Copyright (c) OpenMMLab. All rights reserved.
+from typing import List
+
+import torch
+from torch import Tensor, nn
+from torch.nn.utils.rnn import pack_padded_sequence, pad_packed_sequence
+
+
+class QueryEncoder(nn.Module):
+
+ def __init__(self,
+ vocab_size: int,
+ hidden_dim: int = 512,
+ embed_dim: int = 300,
+ num_layers: int = 1,
+ bidirection: bool = True) -> None:
+ super(QueryEncoder, self).__init__()
+ self.hidden_dim = hidden_dim
+ self.embed_dim = embed_dim
+ self.embedding = nn.Embedding(
+ num_embeddings=vocab_size + 1,
+ embedding_dim=embed_dim,
+ padding_idx=0)
+ # self.embedding.weight.data.copy_(torch.load('glove_weights'))
+ self.biLSTM = nn.LSTM(
+ input_size=embed_dim,
+ hidden_size=self.hidden_dim,
+ num_layers=num_layers,
+ dropout=0.0,
+ batch_first=True,
+ bidirectional=bidirection)
+
+ self.W3 = nn.Linear(hidden_dim * 4, hidden_dim)
+ self.W2 = nn.ModuleList(
+ [nn.Linear(hidden_dim, hidden_dim * 2) for _ in range(3)])
+ self.W1 = nn.Linear(hidden_dim * 2, 1)
+
+ def extract_textual(self, q_encoding: Tensor, lstm_outputs: Tensor,
+ q_length: Tensor, t: int):
+ q_cmd = self.W3(q_encoding).relu()
+ q_cmd = self.W2[t](q_cmd)
+ q_cmd = q_cmd[:, None, :] * lstm_outputs
+ raw_att = self.W1(q_cmd).squeeze(-1)
+
+ raw_att = apply_mask1d(raw_att, q_length)
+ att = raw_att.softmax(dim=-1)
+ cmd = torch.bmm(att[:, None, :], lstm_outputs).squeeze(1)
+ return cmd
+
+ def forward(self, query_tokens: Tensor,
+ query_length: Tensor) -> List[Tensor]:
+ self.biLSTM.flatten_parameters()
+
+ query_embedding = self.embedding(query_tokens)
+
+ # output denotes the forward and backward hidden states in Eq 2.
+ query_embedding = pack_padded_sequence(
+ query_embedding, query_length.cpu(), batch_first=True)
+ output, _ = self.biLSTM(query_embedding)
+ output, _ = pad_packed_sequence(output, batch_first=True)
+
+ # q_vector denotes the global representation `g` in Eq 2.
+ q_vector_list = []
+
+ for i, length in enumerate(query_length):
+ h1 = output[i][0]
+ hs = output[i][length - 1]
+ q_vector = torch.cat((h1, hs), dim=-1)
+ q_vector_list.append(q_vector)
+ q_vector = torch.stack(q_vector_list)
+ # outputs denotes the query feature in Eq3 in 3 levels.
+ outputs = []
+ for cmd_t in range(3):
+ query_feat = self.extract_textual(q_vector, output, query_length,
+ cmd_t)
+ outputs.append(query_feat)
+
+ # Note: the output here is zero-padded
+ # we need slice the non-zero items for the following operations.
+ return outputs
+
+
+def apply_mask1d(attention: Tensor, image_locs: Tensor) -> Tensor:
+ batch_size, num_loc = attention.size()
+ tmp1 = torch.arange(
+ num_loc, dtype=attention.dtype, device=attention.device)
+ tmp1 = tmp1.expand(batch_size, num_loc)
+
+ tmp2 = image_locs.unsqueeze(dim=1).expand(batch_size, num_loc)
+ mask = tmp1 >= tmp2.to(tmp1.dtype)
+ attention = attention.masked_fill(mask, -1e30)
+ return attention
diff --git a/mmaction/models/localizers/drn/drn_utils/loss.py b/mmaction/models/localizers/drn/drn_utils/loss.py
new file mode 100644
index 0000000000..920ebac0b3
--- /dev/null
+++ b/mmaction/models/localizers/drn/drn_utils/loss.py
@@ -0,0 +1,240 @@
+# Copyright (c) OpenMMLab. All rights reserved.
+"""Adapted from https://github.com/Alvin-Zeng/DRN/"""
+
+import torch
+import torchvision
+from torch import nn
+
+INF = 100000000
+
+
+def SigmoidFocalLoss(alpha, gamma):
+
+ def loss_fn(inputs, targets):
+ loss = torchvision.ops.sigmoid_focal_loss(
+ inputs=inputs,
+ targets=targets,
+ alpha=alpha,
+ gamma=gamma,
+ reduction='sum')
+ return loss
+
+ return loss_fn
+
+
+def IOULoss():
+
+ def loss_fn(pred, target):
+ pred_left = pred[:, 0]
+ pred_right = pred[:, 1]
+
+ target_left = target[:, 0]
+ target_right = target[:, 1]
+
+ intersect = torch.min(pred_right, target_right) + torch.min(
+ pred_left, target_left)
+ target_area = target_left + target_right
+ pred_area = pred_left + pred_right
+ union = target_area + pred_area - intersect
+
+ losses = -torch.log((intersect + 1e-8) / (union + 1e-8))
+ return losses.mean()
+
+ return loss_fn
+
+
+class FCOSLossComputation(object):
+ """This class computes the FCOS losses."""
+
+ def __init__(self, focal_alpha, focal_gamma):
+ self.cls_loss_fn = SigmoidFocalLoss(focal_alpha, focal_gamma)
+ self.box_reg_loss_fn = IOULoss()
+ self.centerness_loss_fn = nn.BCEWithLogitsLoss()
+ self.iou_loss_fn = nn.SmoothL1Loss()
+
+ def prepare_targets(self, points, targets):
+ object_sizes_of_interest = [
+ [-1, 6],
+ [5.6, 11],
+ [11, INF],
+ ]
+ expanded_object_sizes_of_interest = []
+ for idx, points_per_level in enumerate(points):
+ object_sizes_of_interest_per_level = \
+ points_per_level.new_tensor(object_sizes_of_interest[idx])
+ expanded_object_sizes_of_interest.append(
+ object_sizes_of_interest_per_level[None].expand(
+ len(points_per_level), -1))
+
+ expanded_object_sizes_of_interest = torch.cat(
+ expanded_object_sizes_of_interest, dim=0)
+ num_points_per_level = [
+ len(points_per_level) for points_per_level in points
+ ]
+ points_all_level = torch.cat(points, dim=0)
+ labels, reg_targets = self.compute_targets_for_locations(
+ points_all_level, targets, expanded_object_sizes_of_interest)
+
+ for i in range(len(labels)):
+ labels[i] = torch.split(labels[i], num_points_per_level, dim=0)
+ reg_targets[i] = torch.split(
+ reg_targets[i], num_points_per_level, dim=0)
+
+ labels_level_first = []
+ reg_targets_level_first = []
+ for level in range(len(points)):
+ labels_level_first.append(
+ torch.cat([labels_per_im[level] for labels_per_im in labels],
+ dim=0))
+ reg_targets_level_first.append(
+ torch.cat([
+ reg_targets_per_im[level]
+ for reg_targets_per_im in reg_targets
+ ],
+ dim=0))
+
+ return labels_level_first, reg_targets_level_first
+
+ def compute_targets_for_locations(self, locations, targets,
+ object_sizes_of_interest):
+ labels = []
+ reg_targets = []
+ ts = locations
+
+ for im_i in range(len(targets)):
+ targets_per_im = targets[im_i]
+ bboxes = targets_per_im * 32
+
+ left = ts[:, None] - bboxes[None, 0]
+ right = bboxes[None, 1] - ts[:, None]
+ reg_targets_per_im = torch.cat([left, right], dim=1)
+
+ is_in_boxes = reg_targets_per_im.min(dim=1)[0] > 0
+ max_reg_targets_per_im = reg_targets_per_im.max(dim=1)[0]
+ is_cared_in_the_level = \
+ (max_reg_targets_per_im >= object_sizes_of_interest[:, 0]) & \
+ (max_reg_targets_per_im <= object_sizes_of_interest[:, 1])
+
+ locations_to_gt_area = bboxes[1] - bboxes[0]
+ locations_to_gt_area = locations_to_gt_area.repeat(
+ len(locations), 1)
+ locations_to_gt_area[is_in_boxes == 0] = INF
+ locations_to_gt_area[is_cared_in_the_level == 0] = INF
+
+ _ = locations_to_gt_area.min(dim=1)
+ locations_to_min_area, locations_to_gt_inds = _
+
+ labels_per_im = reg_targets_per_im.new_ones(
+ len(reg_targets_per_im))
+ labels_per_im[locations_to_min_area == INF] = 0
+
+ labels.append(labels_per_im)
+ reg_targets.append(reg_targets_per_im)
+
+ return labels, reg_targets
+
+ def __call__(self,
+ locations,
+ box_cls,
+ box_regression,
+ targets,
+ iou_scores,
+ is_first_stage=True):
+ N = box_cls[0].size(0)
+ num_classes = box_cls[0].size(1)
+ labels, reg_targets = self.prepare_targets(locations, targets)
+
+ box_cls_flatten = []
+ box_regression_flatten = []
+ # centerness_flatten = []
+ labels_flatten = []
+ reg_targets_flatten = []
+
+ for idx in range(len(labels)):
+ box_cls_flatten.append(box_cls[idx].permute(0, 2, 1).reshape(
+ -1, num_classes))
+ box_regression_flatten.append(box_regression[idx].permute(
+ 0, 2, 1).reshape(-1, 2))
+ labels_flatten.append(labels[idx].reshape(-1))
+ reg_targets_flatten.append(reg_targets[idx].reshape(-1, 2))
+
+ if not is_first_stage:
+ # [batch, 56, 2]
+ merged_box_regression = torch.cat(
+ box_regression, dim=-1).transpose(2, 1)
+ # [56]
+ merged_locations = torch.cat(locations, dim=0)
+ # [batch, 56]
+ full_locations = merged_locations[None, :].expand(
+ merged_box_regression.size(0), -1).contiguous()
+ pred_start = full_locations - merged_box_regression[:, :, 0]
+ pred_end = full_locations + merged_box_regression[:, :, 1]
+ # [batch, 56, 2]
+ predictions = torch.cat(
+ [pred_start.unsqueeze(-1),
+ pred_end.unsqueeze(-1)], dim=-1) / 32
+ # TODO: make sure the predictions are legal. (e.g. start < end)
+ predictions.clamp_(min=0, max=1)
+ # gt: [batch, 2]
+ gt_box = targets[:, None, :]
+
+ iou_target = segment_tiou(predictions, gt_box)
+ iou_pred = torch.cat(iou_scores, dim=-1).squeeze().sigmoid()
+ iou_pos_ind = iou_target > 0.9
+ pos_iou_target = iou_target[iou_pos_ind]
+
+ pos_iou_pred = iou_pred[iou_pos_ind]
+
+ if iou_pos_ind.sum().item() == 0:
+ iou_loss = torch.tensor([0.]).to(iou_pos_ind.device)
+ else:
+ iou_loss = self.iou_loss_fn(pos_iou_pred, pos_iou_target)
+
+ box_cls_flatten = torch.cat(box_cls_flatten, dim=0)
+ box_regression_flatten = torch.cat(box_regression_flatten, dim=0)
+ labels_flatten = torch.cat(labels_flatten, dim=0)
+ reg_targets_flatten = torch.cat(reg_targets_flatten, dim=0)
+
+ pos_inds = torch.nonzero(labels_flatten > 0).squeeze(1)
+ cls_loss = self.cls_loss_fn(
+ box_cls_flatten, labels_flatten.unsqueeze(1)) / (
+ pos_inds.numel() + N) # add N to avoid dividing by a zero
+
+ box_regression_flatten = box_regression_flatten[pos_inds]
+ reg_targets_flatten = reg_targets_flatten[pos_inds]
+
+ if pos_inds.numel() > 0:
+ reg_loss = self.box_reg_loss_fn(
+ box_regression_flatten,
+ reg_targets_flatten,
+ )
+ else:
+ reg_loss = box_regression_flatten.sum()
+
+ if not is_first_stage:
+ return cls_loss, reg_loss, iou_loss
+
+ return cls_loss, reg_loss, torch.tensor([0.]).to(cls_loss.device)
+
+
+def segment_tiou(box_a, box_b):
+
+ # gt: [batch, 1, 2], detections: [batch, 56, 2]
+ # calculate interaction
+ inter_max_xy = torch.min(box_a[:, :, -1], box_b[:, :, -1])
+ inter_min_xy = torch.max(box_a[:, :, 0], box_b[:, :, 0])
+ inter = torch.clamp((inter_max_xy - inter_min_xy), min=0)
+
+ # calculate union
+ union_max_xy = torch.max(box_a[:, :, -1], box_b[:, :, -1])
+ union_min_xy = torch.min(box_a[:, :, 0], box_b[:, :, 0])
+ union = torch.clamp((union_max_xy - union_min_xy), min=0)
+
+ iou = inter / (union + 1e-6)
+
+ return iou
+
+
+def make_fcos_loss_evaluator(focal_alpha, focal_gamma):
+ loss_evaluator = FCOSLossComputation(focal_alpha, focal_gamma)
+ return loss_evaluator
diff --git a/mmaction/models/losses/hvu_loss.py b/mmaction/models/losses/hvu_loss.py
index 38be482ab2..d3f7aaa274 100644
--- a/mmaction/models/losses/hvu_loss.py
+++ b/mmaction/models/losses/hvu_loss.py
@@ -1,6 +1,7 @@
# Copyright (c) OpenMMLab. All rights reserved.
import torch
import torch.nn.functional as F
+from mmengine.device import get_device
from mmaction.registry import MODELS
from .base import BaseWeightedLoss
@@ -111,7 +112,8 @@ def _forward(self, cls_score, label, mask, category_mask):
# there should be at least one sample which contains tags
# in this category
if torch.sum(category_mask_i) < 0.5:
- losses[f'{name}_LOSS'] = torch.tensor(.0).cuda()
+ losses[f'{name}_LOSS'] = torch.tensor(
+ .0, device=get_device())
loss_weights[f'{name}_LOSS'] = .0
continue
category_loss = torch.sum(category_loss * category_mask_i)
diff --git a/mmaction/models/multimodal/__init__.py b/mmaction/models/multimodal/__init__.py
new file mode 100644
index 0000000000..9a5f2a99df
--- /dev/null
+++ b/mmaction/models/multimodal/__init__.py
@@ -0,0 +1,12 @@
+# Copyright (c) OpenMMLab. All rights reserved.
+from mmaction.utils.dependency import WITH_MULTIMODAL
+
+if WITH_MULTIMODAL:
+ from .vindlu import * # noqa: F401,F403
+
+else:
+ from mmaction.registry import MODELS
+ from mmaction.utils.dependency import register_multimodal_placeholder
+
+ register_multimodal_placeholder(
+ ['VindLUVQA', 'VindLURetrievalMC', 'VindLURetrieval'], MODELS)
diff --git a/mmaction/models/multimodal/vindlu/__init__.py b/mmaction/models/multimodal/vindlu/__init__.py
new file mode 100644
index 0000000000..e17c193246
--- /dev/null
+++ b/mmaction/models/multimodal/vindlu/__init__.py
@@ -0,0 +1,12 @@
+# Copyright (c) OpenMMLab. All rights reserved.
+from .beit3d import BeitModel3D
+from .tokenizer import VindLUTokenizer
+from .vindlu_ret import VindLURetrieval
+from .vindlu_ret_mc import VindLURetrievalMC
+from .vindlu_vqa import VindLUVQA
+from .xbert import BertDecoder, BertModel
+
+__all__ = [
+ 'VindLUVQA', 'VindLURetrievalMC', 'VindLURetrieval', 'VindLUTokenizer',
+ 'BeitModel3D', 'BertDecoder', 'BertModel'
+]
diff --git a/mmaction/models/multimodal/vindlu/beit3d.py b/mmaction/models/multimodal/vindlu/beit3d.py
new file mode 100644
index 0000000000..8e0d6f2fc3
--- /dev/null
+++ b/mmaction/models/multimodal/vindlu/beit3d.py
@@ -0,0 +1,350 @@
+# Copyright (c) OpenMMLab. All rights reserved.
+import importlib
+from typing import Dict, Optional, Tuple, Union
+
+import einops
+import torch
+import torch.nn as nn
+import torch.nn.functional as F
+from transformers.models.beit import BeitConfig, BeitModel
+from transformers.models.beit.modeling_beit import BeitAttention, BeitDropPath
+from transformers.models.beit.modeling_beit import \
+ BeitEmbeddings as BeitEmbeddings2D
+from transformers.models.beit.modeling_beit import BeitLayer as BeitLayer2D
+from transformers.models.beit.modeling_beit import BeitRelativePositionBias
+from transformers.models.beit.modeling_beit import \
+ BeitRelativePositionBias as BeitRelativePositionBias2D
+
+from mmaction.registry import MODELS
+from .temporal_model import (X_CLIP, STAdapter, TemporalAttention,
+ WindowTemporalAttention)
+
+
+def interpolate_temporal_pos_embed(temp_embed_old, num_frames_new):
+ """
+ temp_embed_old: (1, num_frames_old, 1, d)
+ Returns:
+ temp_embed_new: (1, num_frames_new, 1, d)
+ """
+ temp_embed_old = temp_embed_old.squeeze(2).permute(
+ 0, 2, 1) # (1, d, num_frames_old)
+ temp_embed_new = F.interpolate(
+ temp_embed_old, num_frames_new,
+ mode='linear') # (1, d, num_frames_new)
+ temp_embed_new = temp_embed_new.permute(0, 2, 1).unsqueeze(
+ 2) # (1, num_frames_new, 1, d)
+ return temp_embed_new
+
+
+class TemporalAttentionBeit(nn.Module):
+ """temporal attention using BeitAttention."""
+
+ def __init__(self, config: BeitConfig):
+ """TODO: to be defined."""
+ super().__init__()
+
+ self.layernorm_before = nn.LayerNorm(
+ config.hidden_size, eps=config.layer_norm_eps)
+ self.attention = BeitAttention(config, window_size=None)
+ self.scale = nn.Parameter(
+ config.temporal_model_init_value * torch.ones(
+ (config.hidden_size)),
+ requires_grad=True,
+ )
+ self.drop_path = BeitDropPath(config.drop_path_rate)
+
+ def forward(self, hidden_states: torch.Tensor):
+ """forward function.
+
+ Args:
+ hidden_states (torch.Tensor): The input. Shape: [b,t,l,c]
+
+ Returns: TODO
+ """
+ b = hidden_states.shape[0]
+ output = einops.rearrange(hidden_states, 'b t l c -> (b l) t c')
+ output = self.layernorm_before(output)
+ output = self.attention(output)
+ output = einops.rearrange(output[0], '(b l) t c -> b t l c', b=b)
+ return hidden_states + self.drop_path(output[0]) * self.scale
+
+
+class BeitPooler3D(nn.Module):
+
+ def __init__(self, config: BeitConfig) -> None:
+ super().__init__()
+ self.num_prompts = config.add_k_prompts
+ self.layernorm = (
+ nn.LayerNorm(config.hidden_size, eps=config.layer_norm_eps)
+ if config.use_mean_pooling else None)
+
+ def forward(self, hidden_states: torch.Tensor) -> torch.Tensor:
+ """
+ Args:
+ hidden_states (torch.Tensor): Shape: [B,T,L,C]
+ """
+ if self.layernorm is not None:
+ # Mean pool the final hidden states of the patch tokens
+ # patch_tokens = hidden_states[:, 1 + self.num_prompts :, :]
+ if self.num_prompts > 0:
+ patch_tokens = hidden_states[:, :, 1:-self.num_prompts, :]
+ else:
+ patch_tokens = hidden_states[:, :, 1:, :]
+ pooled_output = self.layernorm(patch_tokens.mean(2))
+ else:
+ # Pool by simply taking the final hidden state of the [CLS] token
+ pooled_output = hidden_states[:, :, 0]
+
+ return pooled_output
+
+
+class BeitRelativePositionBias3D(BeitRelativePositionBias2D):
+
+ def __init__(self, config: BeitConfig, window_size: tuple) -> None:
+ super().__init__(config, window_size)
+
+ # add bias for prompts
+ self.k = config.add_k_prompts
+ if self.k > 0:
+ self.prompt_bias_table = nn.parameter.Parameter(
+ torch.zeros((2 + self.k) * self.k, config.num_attention_heads)
+ ) # k prompt-to-token, k token-to-prompt, k*k prompt-to-promt
+ else:
+ self.prompt_bias_table = None
+
+ def forward(self) -> torch.Tensor:
+ # relative position bias 2d
+ relative_position_bias = self.relative_position_bias_table[
+ self.relative_position_index.view(-1)].view(
+ self.window_size[0] * self.window_size[1] + 1,
+ self.window_size[0] * self.window_size[1] + 1,
+ -1,
+ ) # Wh*Ww,Wh*Ww,nH
+
+ # add bias for prompts
+ k = self.k
+ if k > 0:
+ l = self.window_size[0] * self.window_size[1] + 1 # noqa: E741
+ bias = torch.zeros(l + k, l + k,
+ relative_position_bias.shape[-1]).to(
+ relative_position_bias.device)
+ bias[:l, :l] = relative_position_bias
+ bias[l:, :l] = self.prompt_bias_table[:k].view(
+ k, 1, -1) # prompt to token
+ bias[:l,
+ l:] = self.prompt_bias_table[k:2 *
+ k].view(1, k,
+ -1) # token to prompt
+ bias[l:, l:] = self.prompt_bias_table[2 * k, :].view(
+ k, k, -1) # prompt to prompt
+ else:
+ bias = relative_position_bias
+
+ return bias.permute(2, 0, 1).contiguous() # nH, Wh*Ww, Wh*Ww
+
+
+class BeitEmbeddings3D(BeitEmbeddings2D):
+ """Construct the CLS token, position and patch embeddings.
+
+ Optionally, also the mask token.
+ """
+
+ def __init__(self, config: BeitConfig) -> None:
+ super().__init__(config)
+
+ if config.use_temporal_position_embedding:
+ self.temporal_position_embeddings = nn.parameter.Parameter(
+ torch.zeros(1, config.num_frames, 1, config.hidden_size))
+ else:
+ self.temporal_position_embeddings = None
+
+ if config.add_k_prompts > 0:
+ self.prompt_tokens = nn.parameter.Parameter(
+ torch.zeros(1, config.add_k_prompts, config.hidden_size))
+ else:
+ self.prompt_tokens = None
+
+ def forward(self,
+ pixel_values: torch.Tensor,
+ bool_masked_pos: Optional[torch.BoolTensor] = None
+ ) -> torch.Tensor:
+ """
+ Args:
+ pixel_values (torch.Tensor): The input image patches.
+ Shape: [B, T, C, H, W].
+
+
+ """
+ t = pixel_values.shape[1]
+ pixel_values = einops.rearrange(pixel_values,
+ 'b t c h w -> (b t) c h w')
+
+ embeddings = self.patch_embeddings(pixel_values)
+ batch_size, seq_len, _ = embeddings.size() # [(b t) l c]
+
+ cls_tokens = self.cls_token.expand(batch_size, -1, -1)
+ if bool_masked_pos is not None:
+ mask_tokens = self.mask_token.expand(batch_size, seq_len, -1)
+ # replace the masked visual tokens by mask_tokens
+ w = bool_masked_pos.unsqueeze(-1).type_as(mask_tokens)
+ embeddings = embeddings * (1 - w) + mask_tokens * w
+
+ if self.prompt_tokens is not None:
+ prompt_tokens = self.prompt_tokens.expand(batch_size, -1, -1)
+ embeddings = torch.cat((cls_tokens, embeddings, prompt_tokens),
+ dim=1)
+ else:
+ embeddings = torch.cat((cls_tokens, embeddings),
+ dim=1) # [B*T, L, C]
+ if self.position_embeddings is not None:
+ embeddings = embeddings + self.position_embeddings
+
+ embeddings = einops.rearrange(embeddings, '(b t) l c -> b t l c', t=t)
+ if self.temporal_position_embeddings is not None:
+ if t <= self.temporal_position_embeddings.shape[1]:
+ embeddings = embeddings + \
+ self.temporal_position_embeddings[:, :t]
+ else:
+ tpe = interpolate_temporal_pos_embed(
+ self.temporal_position_embeddings, t)
+ embeddings = embeddings + tpe
+
+ embeddings = self.dropout(embeddings)
+
+ return embeddings
+
+
+class BeitLayer3D(BeitLayer2D):
+
+ def __init__(self,
+ config: BeitConfig,
+ window_size: Optional[tuple] = None,
+ drop_path_rate: float = 0.0) -> None:
+ super().__init__(config, window_size, drop_path_rate)
+
+ self.temporal_model_position = config.temporal_model_position
+ if config.temporal_model_block == 'st_adapter':
+ self.temp_model = STAdapter(**config.temporal_model_config)
+ elif config.temporal_model_block == 'timesformer':
+ self.temp_model = TemporalAttention(**config.temporal_model_config)
+ elif config.temporal_model_block == 'ta_beit':
+ self.temp_model = TemporalAttentionBeit(config)
+ elif config.temporal_model_block == 'window_attention':
+ self.temp_model = WindowTemporalAttention(
+ **config.temporal_model_config)
+ elif config.temporal_model_block == 'xclip':
+ self.temp_model = X_CLIP(**config.temporal_model_config)
+ elif config.temporal_model_block == 'none':
+ self.temp_model = None
+ else:
+ raise ValueError(
+ f'not accepted temporal model: {config.temporal_model_block}')
+
+ self.temporal_model_block = config.temporal_model_block
+
+ def forward(
+ self,
+ hidden_states: torch.Tensor,
+ head_mask: Optional[torch.Tensor] = None,
+ output_attentions: bool = False,
+ relative_position_bias: Optional['BeitRelativePositionBias'] = None,
+ ) -> Union[Tuple[torch.Tensor], Tuple[torch.Tensor, torch.Tensor]]:
+
+ b, t, l, c = hidden_states.shape
+
+ if self.temporal_model_block == 'xclip':
+ assert (self.temporal_model_position == 'first'
+ and self.config.add_k_prompts
+ == 1), ('xclip must be put before the attention and'
+ 'add_k_prompts must be 1.')
+
+ if self.temp_model is not None and \
+ self.temporal_model_position == 'first':
+ hidden_states = self.temp_model(hidden_states)
+
+ hidden_states = einops.rearrange(hidden_states, 'b t l c -> (b t) l c')
+
+ self_attention_outputs = self.attention(
+ self.layernorm_before(
+ hidden_states
+ ), # in BEiT, layernorm is applied before self-attention
+ head_mask,
+ output_attentions=output_attentions,
+ relative_position_bias=relative_position_bias,
+ )
+ attention_output = self_attention_outputs[0]
+
+ # add self attentions if we output attention weights
+ outputs = self_attention_outputs[1:]
+
+ # apply lambda_1 if present
+ if self.lambda_1 is not None:
+ attention_output = self.lambda_1 * attention_output
+
+ # first residual connection
+ hidden_states = self.drop_path(attention_output) + hidden_states
+
+ # in BEiT, layernorm is also applied after self-attention
+ layer_output = self.layernorm_after(hidden_states)
+
+ layer_output = self.intermediate(layer_output)
+ layer_output = self.output(layer_output)
+
+ if self.lambda_2 is not None:
+ layer_output = self.lambda_2 * layer_output
+
+ # second residual connection
+ layer_output = self.drop_path(layer_output) + hidden_states
+
+ layer_output = einops.rearrange(
+ layer_output, '(b t) l c -> b t l c', b=b)
+
+ # apply temporal modeling block
+ if self.temp_model is not None and \
+ self.temporal_model_position == 'last':
+ layer_output = self.temp_model(layer_output)
+
+ outputs = (layer_output, ) + outputs
+
+ return outputs
+
+
+class BeitConfig3D(BeitConfig):
+
+ def __init__(self,
+ num_frames=1,
+ temporal_model_block='none',
+ temporal_model_position='last',
+ temporal_model_init_value=0.0,
+ temporal_model_config={},
+ use_temporal_position_embedding=False,
+ add_k_prompts=0,
+ **kwargs) -> None:
+
+ super().__init__(**kwargs)
+ self.temporal_model_block = temporal_model_block
+ self.temporal_model_config = temporal_model_config
+ self.temporal_model_position = temporal_model_position
+ self.temporal_model_init_value = temporal_model_init_value
+ self.use_temporal_position_embedding = use_temporal_position_embedding
+ self.add_k_prompts = add_k_prompts
+ self.num_frames = num_frames
+
+
+@MODELS.register_module()
+class BeitModel3D(BeitModel):
+
+ def __init__(self,
+ config: BeitConfig,
+ tem_config: Dict,
+ add_pooling_layer: bool = True) -> None:
+ # hack to replace original 2D modules with 3D modules
+ beit_package = importlib.import_module(
+ 'transformers.models.beit.modeling_beit')
+ beit_package.BeitEmbeddings = BeitEmbeddings3D
+ beit_package.BeitPooler = BeitPooler3D
+ beit_package.BeitLayer = BeitLayer3D
+ beit_package.BeitRelativePositionBias = BeitRelativePositionBias3D
+
+ config = BeitConfig3D.from_pretrained(config, **tem_config)
+ super().__init__(config, add_pooling_layer)
diff --git a/mmaction/models/multimodal/vindlu/modeling_bert.py b/mmaction/models/multimodal/vindlu/modeling_bert.py
new file mode 100644
index 0000000000..5ffba79bdc
--- /dev/null
+++ b/mmaction/models/multimodal/vindlu/modeling_bert.py
@@ -0,0 +1,1740 @@
+# flake8: noqa
+# Copyright 2018 The Google AI Language Team Authors and The HuggingFace Inc. team.
+# Copyright (c) 2018, NVIDIA CORPORATION. All rights reserved.
+#
+# Licensed under the Apache License, Version 2.0 (the "License");
+# you may not use this file except in compliance with the License.
+# You may obtain a copy of the License at
+#
+# http://www.apache.org/licenses/LICENSE-2.0
+#
+# Unless required by applicable law or agreed to in writing, software
+# distributed under the License is distributed on an "AS IS" BASIS,
+# WITHOUT WARRANTIES OR CONDITIONS OF ANY KIND, either express or implied.
+# See the License for the specific language governing permissions and
+# limitations under the License.
+"""PyTorch BERT model."""
+
+import math
+import os
+import warnings
+from dataclasses import dataclass
+from typing import Optional, Tuple
+
+import torch
+import torch.nn.functional as F
+import torch.utils.checkpoint
+import transformers
+from mmengine.logging import MMLogger
+from torch import Tensor, device, dtype, nn
+from torch.nn import CrossEntropyLoss, MSELoss
+from transformers.activations import ACT2FN
+# from transformers.models.bert.configuration_bert import BertConfig
+from transformers.configuration_utils import PretrainedConfig
+from transformers.file_utils import (ModelOutput, add_start_docstrings,
+ add_start_docstrings_to_model_forward,
+ replace_return_docstrings)
+from transformers.modeling_outputs import (
+ BaseModelOutputWithPastAndCrossAttentions,
+ BaseModelOutputWithPoolingAndCrossAttentions,
+ CausalLMOutputWithCrossAttentions, MaskedLMOutput,
+ MultipleChoiceModelOutput, NextSentencePredictorOutput,
+ QuestionAnsweringModelOutput, SequenceClassifierOutput,
+ TokenClassifierOutput)
+from transformers.modeling_utils import (PreTrainedModel,
+ apply_chunking_to_forward,
+ find_pruneable_heads_and_indices,
+ prune_linear_layer)
+
+transformers.logging.set_verbosity_error()
+
+_CONFIG_FOR_DOC = 'BertConfig'
+_TOKENIZER_FOR_DOC = 'BertTokenizer'
+
+BERT_PRETRAINED_MODEL_ARCHIVE_LIST = [
+ 'bert-base-uncased',
+ 'bert-large-uncased',
+ 'bert-base-cased',
+ 'bert-large-cased',
+ 'bert-base-multilingual-uncased',
+ 'bert-base-multilingual-cased',
+ 'bert-base-chinese',
+ 'bert-base-german-cased',
+ 'bert-large-uncased-whole-word-masking',
+ 'bert-large-cased-whole-word-masking',
+ 'bert-large-uncased-whole-word-masking-finetuned-squad',
+ 'bert-large-cased-whole-word-masking-finetuned-squad',
+ 'bert-base-cased-finetuned-mrpc',
+ 'bert-base-german-dbmdz-cased',
+ 'bert-base-german-dbmdz-uncased',
+ 'cl-tohoku/bert-base-japanese',
+ 'cl-tohoku/bert-base-japanese-whole-word-masking',
+ 'cl-tohoku/bert-base-japanese-char',
+ 'cl-tohoku/bert-base-japanese-char-whole-word-masking',
+ 'TurkuNLP/bert-base-finnish-cased-v1',
+ 'TurkuNLP/bert-base-finnish-uncased-v1',
+ 'wietsedv/bert-base-dutch-cased',
+ # See all BERT models at https://huggingface.co/models?filter=bert
+]
+
+
+class BertConfig(PretrainedConfig):
+ r"""
+ This is the configuration class to store the configuration of a [`BertModel`] or a [`TFBertModel`]. It is used to
+ instantiate a BERT model according to the specified arguments, defining the model architecture. Instantiating a
+ configuration with the defaults will yield a similar configuration to that of the BERT
+ [bert-base-uncased](https://huggingface.co/bert-base-uncased) architecture.
+
+ Configuration objects inherit from [`PretrainedConfig`] and can be used to control the model outputs. Read the
+ documentation from [`PretrainedConfig`] for more information.
+
+
+ Args:
+ vocab_size (`int`, *optional*, defaults to 30522):
+ Vocabulary size of the BERT model. Defines the number of different tokens that can be represented by the
+ `inputs_ids` passed when calling [`BertModel`] or [`TFBertModel`].
+ hidden_size (`int`, *optional*, defaults to 768):
+ Dimensionality of the encoder layers and the pooler layer.
+ num_hidden_layers (`int`, *optional*, defaults to 12):
+ Number of hidden layers in the Transformer encoder.
+ num_attention_heads (`int`, *optional*, defaults to 12):
+ Number of attention heads for each attention layer in the Transformer encoder.
+ intermediate_size (`int`, *optional*, defaults to 3072):
+ Dimensionality of the "intermediate" (often named feed-forward) layer in the Transformer encoder.
+ hidden_act (`str` or `Callable`, *optional*, defaults to `"gelu"`):
+ The non-linear activation function (function or string) in the encoder and pooler. If string, `"gelu"`,
+ `"relu"`, `"silu"` and `"gelu_new"` are supported.
+ hidden_dropout_prob (`float`, *optional*, defaults to 0.1):
+ The dropout probability for all fully connected layers in the embeddings, encoder, and pooler.
+ attention_probs_dropout_prob (`float`, *optional*, defaults to 0.1):
+ The dropout ratio for the attention probabilities.
+ max_position_embeddings (`int`, *optional*, defaults to 512):
+ The maximum sequence length that this model might ever be used with. Typically set this to something large
+ just in case (e.g., 512 or 1024 or 2048).
+ type_vocab_size (`int`, *optional*, defaults to 2):
+ The vocabulary size of the `token_type_ids` passed when calling [`BertModel`] or [`TFBertModel`].
+ initializer_range (`float`, *optional*, defaults to 0.02):
+ The standard deviation of the truncated_normal_initializer for initializing all weight matrices.
+ layer_norm_eps (`float`, *optional*, defaults to 1e-12):
+ The epsilon used by the layer normalization layers.
+ position_embedding_type (`str`, *optional*, defaults to `"absolute"`):
+ Type of position embedding. Choose one of `"absolute"`, `"relative_key"`, `"relative_key_query"`. For
+ positional embeddings use `"absolute"`. For more information on `"relative_key"`, please refer to
+ [Self-Attention with Relative Position Representations (Shaw et al.)](https://arxiv.org/abs/1803.02155).
+ For more information on `"relative_key_query"`, please refer to *Method 4* in [Improve Transformer Models
+ with Better Relative Position Embeddings (Huang et al.)](https://arxiv.org/abs/2009.13658).
+ use_cache (`bool`, *optional*, defaults to `True`):
+ Whether or not the model should return the last key/values attentions (not used by all models). Only
+ relevant if `config.is_decoder=True`.
+ classifier_dropout (`float`, *optional*):
+ The dropout ratio for the classification head.
+
+ Examples:
+
+ ```python
+ >>> from transformers import BertModel, BertConfig
+
+ >>> # Initializing a BERT bert-base-uncased style configuration
+ >>> configuration = BertConfig()
+
+ >>> # Initializing a model from the bert-base-uncased style configuration
+ >>> model = BertModel(configuration)
+
+ >>> # Accessing the model configuration
+ >>> configuration = model.config
+ ```"""
+ model_type = 'bert'
+
+ def __init__(
+ self,
+ vocab_size=30522,
+ hidden_size=768,
+ num_hidden_layers=12,
+ num_attention_heads=12,
+ intermediate_size=3072,
+ hidden_act='gelu',
+ hidden_dropout_prob=0.1,
+ attention_probs_dropout_prob=0.1,
+ max_position_embeddings=512,
+ type_vocab_size=2,
+ initializer_range=0.02,
+ layer_norm_eps=1e-12,
+ pad_token_id=0,
+ position_embedding_type='absolute',
+ use_cache=True,
+ classifier_dropout=None,
+ cross_module='ca',
+ encoder_width=768,
+ **kwargs,
+ ):
+ super().__init__(pad_token_id=pad_token_id, **kwargs)
+
+ self.vocab_size = vocab_size
+ self.hidden_size = hidden_size
+ self.num_hidden_layers = num_hidden_layers
+ self.num_attention_heads = num_attention_heads
+ self.hidden_act = hidden_act
+ self.intermediate_size = intermediate_size
+ self.hidden_dropout_prob = hidden_dropout_prob
+ self.attention_probs_dropout_prob = attention_probs_dropout_prob
+ self.max_position_embeddings = max_position_embeddings
+ self.type_vocab_size = type_vocab_size
+ self.initializer_range = initializer_range
+ self.layer_norm_eps = layer_norm_eps
+ self.position_embedding_type = position_embedding_type
+ self.use_cache = use_cache
+ self.classifier_dropout = classifier_dropout
+ self.cross_module = cross_module
+ self.encoder_width = encoder_width
+
+
+def load_tf_weights_in_bert(model, config, tf_checkpoint_path):
+ """Load tf checkpoints in a pytorch model."""
+ logger = MMLogger.get_current_instance()
+ try:
+ import re
+
+ import numpy as np
+ import tensorflow as tf
+ except ImportError:
+ logger.error(
+ 'Loading a TensorFlow model in PyTorch, requires TensorFlow to be installed. Please see '
+ 'https://www.tensorflow.org/install/ for installation instructions.'
+ )
+ raise
+ tf_path = os.path.abspath(tf_checkpoint_path)
+ logger.info('Converting TensorFlow checkpoint from {}'.format(tf_path))
+ # Load weights from TF model
+ init_vars = tf.train.list_variables(tf_path)
+ names = []
+ arrays = []
+ for name, shape in init_vars:
+ logger.info('Loading TF weight {} with shape {}'.format(name, shape))
+ array = tf.train.load_variable(tf_path, name)
+ names.append(name)
+ arrays.append(array)
+
+ for name, array in zip(names, arrays):
+ name = name.split('/')
+ # adam_v and adam_m are variables used in AdamWeightDecayOptimizer to calculated m and v
+ # which are not required for using pretrained model
+ if any(n in [
+ 'adam_v',
+ 'adam_m',
+ 'AdamWeightDecayOptimizer',
+ 'AdamWeightDecayOptimizer_1',
+ 'global_step',
+ ] for n in name):
+ logger.info('Skipping {}'.format('/'.join(name)))
+ continue
+ pointer = model
+ for m_name in name:
+ if re.fullmatch(r'[A-Za-z]+_\d+', m_name):
+ scope_names = re.split(r'_(\d+)', m_name)
+ else:
+ scope_names = [m_name]
+ if scope_names[0] == 'kernel' or scope_names[0] == 'gamma':
+ pointer = getattr(pointer, 'weight')
+ elif scope_names[0] == 'output_bias' or scope_names[0] == 'beta':
+ pointer = getattr(pointer, 'bias')
+ elif scope_names[0] == 'output_weights':
+ pointer = getattr(pointer, 'weight')
+ elif scope_names[0] == 'squad':
+ pointer = getattr(pointer, 'classifier')
+ else:
+ try:
+ pointer = getattr(pointer, scope_names[0])
+ except AttributeError:
+ logger.info('Skipping {}'.format('/'.join(name)))
+ continue
+ if len(scope_names) >= 2:
+ num = int(scope_names[1])
+ pointer = pointer[num]
+ if m_name[-11:] == '_embeddings':
+ pointer = getattr(pointer, 'weight')
+ elif m_name == 'kernel':
+ array = np.transpose(array)
+ try:
+ assert (
+ pointer.shape == array.shape
+ ), f'Pointer shape {pointer.shape} and array shape {array.shape} mismatched'
+ except AssertionError as e:
+ e.args += (pointer.shape, array.shape)
+ raise
+
+ logger.info('Initialize PyTorch weight {}'.format(name))
+ pointer.data = torch.from_numpy(array)
+ return model
+
+
+class BertEmbeddings(nn.Module):
+ """Construct the embeddings from word, position and token_type
+ embeddings."""
+
+ def __init__(self, config):
+ super().__init__()
+ self.word_embeddings = nn.Embedding(
+ config.vocab_size,
+ config.hidden_size,
+ padding_idx=config.pad_token_id)
+ self.position_embeddings = nn.Embedding(config.max_position_embeddings,
+ config.hidden_size)
+ self.token_type_embeddings = nn.Embedding(config.type_vocab_size,
+ config.hidden_size)
+
+ # self.LayerNorm is not snake-cased to stick with TensorFlow model variable name and be able to load
+ # any TensorFlow checkpoint file
+ self.LayerNorm = nn.LayerNorm(
+ config.hidden_size, eps=config.layer_norm_eps)
+ self.dropout = nn.Dropout(config.hidden_dropout_prob)
+
+ # position_ids (1, len position emb) is contiguous in memory and exported when serialized
+ self.register_buffer(
+ 'position_ids',
+ torch.arange(config.max_position_embeddings).expand((1, -1)))
+ self.position_embedding_type = getattr(config,
+ 'position_embedding_type',
+ 'absolute')
+
+ self.config = config
+
+ def forward(
+ self,
+ input_ids=None,
+ token_type_ids=None,
+ position_ids=None,
+ inputs_embeds=None,
+ past_key_values_length=0,
+ ):
+ if input_ids is not None:
+ input_shape = input_ids.size()
+ else:
+ input_shape = inputs_embeds.size()[:-1]
+
+ seq_length = input_shape[1]
+
+ if position_ids is None:
+ position_ids = self.position_ids[:, past_key_values_length:
+ seq_length +
+ past_key_values_length]
+
+ if token_type_ids is None:
+ token_type_ids = torch.zeros(
+ input_shape, dtype=torch.long, device=self.position_ids.device)
+
+ if inputs_embeds is None:
+ inputs_embeds = self.word_embeddings(input_ids)
+
+ token_type_embeddings = self.token_type_embeddings(token_type_ids)
+
+ embeddings = inputs_embeds + token_type_embeddings
+ if self.position_embedding_type == 'absolute':
+ position_embeddings = self.position_embeddings(position_ids)
+ embeddings += position_embeddings
+ embeddings = self.LayerNorm(embeddings)
+ embeddings = self.dropout(embeddings)
+ return embeddings
+
+
+class BertSelfAttention(nn.Module):
+
+ def __init__(self, config, is_cross_attention):
+ super().__init__()
+ self.config = config
+ if config.hidden_size % config.num_attention_heads != 0 and not hasattr(
+ config, 'embedding_size'):
+ raise ValueError(
+ 'The hidden size (%d) is not a multiple of the number of attention '
+ 'heads (%d)' %
+ (config.hidden_size, config.num_attention_heads))
+
+ self.num_attention_heads = config.num_attention_heads
+ self.attention_head_size = int(config.hidden_size /
+ config.num_attention_heads)
+ self.all_head_size = self.num_attention_heads * self.attention_head_size
+
+ self.query = nn.Linear(config.hidden_size, self.all_head_size)
+ if is_cross_attention:
+ self.key = nn.Linear(config.encoder_width, self.all_head_size)
+ self.value = nn.Linear(config.encoder_width, self.all_head_size)
+ else:
+ self.key = nn.Linear(config.hidden_size, self.all_head_size)
+ self.value = nn.Linear(config.hidden_size, self.all_head_size)
+
+ self.dropout = nn.Dropout(config.attention_probs_dropout_prob)
+ self.position_embedding_type = getattr(config,
+ 'position_embedding_type',
+ 'absolute')
+ if (self.position_embedding_type == 'relative_key'
+ or self.position_embedding_type == 'relative_key_query'):
+ self.max_position_embeddings = config.max_position_embeddings
+ self.distance_embedding = nn.Embedding(
+ 2 * config.max_position_embeddings - 1,
+ self.attention_head_size)
+ self.save_attention = False
+
+ def save_attn_gradients(self, attn_gradients):
+ self.attn_gradients = attn_gradients
+
+ def get_attn_gradients(self):
+ return self.attn_gradients
+
+ def save_attention_map(self, attention_map):
+ self.attention_map = attention_map
+
+ def get_attention_map(self):
+ return self.attention_map
+
+ def transpose_for_scores(self, x):
+ new_x_shape = x.size()[:-1] + (self.num_attention_heads,
+ self.attention_head_size)
+ x = x.view(*new_x_shape)
+ return x.permute(0, 2, 1, 3)
+
+ def forward(
+ self,
+ hidden_states,
+ attention_mask=None,
+ head_mask=None,
+ encoder_hidden_states=None,
+ encoder_attention_mask=None,
+ past_key_value=None,
+ output_attentions=False,
+ ):
+ mixed_query_layer = self.query(hidden_states)
+
+ # If this is instantiated as a cross-attention module, the keys
+ # and values come from an encoder; the attention mask needs to be
+ # such that the encoder's padding tokens are not attended to.
+ is_cross_attention = encoder_hidden_states is not None
+
+ if is_cross_attention:
+ key_layer = self.transpose_for_scores(
+ self.key(encoder_hidden_states))
+ value_layer = self.transpose_for_scores(
+ self.value(encoder_hidden_states))
+ attention_mask = encoder_attention_mask
+ elif past_key_value is not None:
+ key_layer = self.transpose_for_scores(self.key(hidden_states))
+ value_layer = self.transpose_for_scores(self.value(hidden_states))
+ key_layer = torch.cat([past_key_value[0], key_layer], dim=2)
+ value_layer = torch.cat([past_key_value[1], value_layer], dim=2)
+ else:
+ key_layer = self.transpose_for_scores(self.key(hidden_states))
+ value_layer = self.transpose_for_scores(self.value(hidden_states))
+
+ query_layer = self.transpose_for_scores(mixed_query_layer)
+
+ past_key_value = (key_layer, value_layer)
+
+ # Take the dot product between "query" and "key" to get the raw attention scores.
+ attention_scores = torch.matmul(query_layer,
+ key_layer.transpose(-1, -2))
+
+ if (self.position_embedding_type == 'relative_key'
+ or self.position_embedding_type == 'relative_key_query'):
+ seq_length = hidden_states.size()[1]
+ position_ids_l = torch.arange(
+ seq_length, dtype=torch.long,
+ device=hidden_states.device).view(-1, 1)
+ position_ids_r = torch.arange(
+ seq_length, dtype=torch.long,
+ device=hidden_states.device).view(1, -1)
+ distance = position_ids_l - position_ids_r
+ positional_embedding = self.distance_embedding(
+ distance + self.max_position_embeddings - 1)
+ positional_embedding = positional_embedding.to(
+ dtype=query_layer.dtype) # fp16 compatibility
+
+ if self.position_embedding_type == 'relative_key':
+ relative_position_scores = torch.einsum(
+ 'bhld,lrd->bhlr', query_layer, positional_embedding)
+ attention_scores = attention_scores + relative_position_scores
+ elif self.position_embedding_type == 'relative_key_query':
+ relative_position_scores_query = torch.einsum(
+ 'bhld,lrd->bhlr', query_layer, positional_embedding)
+ relative_position_scores_key = torch.einsum(
+ 'bhrd,lrd->bhlr', key_layer, positional_embedding)
+ attention_scores = (
+ attention_scores + relative_position_scores_query +
+ relative_position_scores_key)
+
+ attention_scores = attention_scores / math.sqrt(
+ self.attention_head_size)
+ if attention_mask is not None:
+ # Apply the attention mask is (precomputed for all layers in BertModel forward() function)
+ attention_scores = attention_scores + attention_mask
+
+ # Normalize the attention scores to probabilities.
+ attention_probs = nn.Softmax(dim=-1)(attention_scores)
+
+ if is_cross_attention and self.save_attention:
+ self.save_attention_map(attention_probs)
+ attention_probs.register_hook(self.save_attn_gradients)
+
+ # This is actually dropping out entire tokens to attend to, which might
+ # seem a bit unusual, but is taken from the original Transformer paper.
+ attention_probs_dropped = self.dropout(attention_probs)
+
+ # Mask heads if we want to
+ if head_mask is not None:
+ attention_probs_dropped = attention_probs_dropped * head_mask
+
+ context_layer = torch.matmul(attention_probs_dropped, value_layer)
+
+ context_layer = context_layer.permute(0, 2, 1, 3).contiguous()
+ new_context_layer_shape = context_layer.size()[:-2] + (
+ self.all_head_size, )
+ context_layer = context_layer.view(*new_context_layer_shape)
+
+ # added `attention_scores` to return tuple
+ outputs = ((context_layer, attention_probs,
+ attention_scores) if output_attentions else
+ (context_layer, ))
+
+ outputs = outputs + (past_key_value, )
+ return outputs
+
+
+class BertSelfOutput(nn.Module):
+
+ def __init__(self, config):
+ super().__init__()
+ self.dense = nn.Linear(config.hidden_size, config.hidden_size)
+ self.LayerNorm = nn.LayerNorm(
+ config.hidden_size, eps=config.layer_norm_eps)
+ self.dropout = nn.Dropout(config.hidden_dropout_prob)
+
+ def forward(self, hidden_states, input_tensor):
+ hidden_states = self.dense(hidden_states)
+ hidden_states = self.dropout(hidden_states)
+ hidden_states = self.LayerNorm(hidden_states + input_tensor)
+ return hidden_states
+
+
+class BertAttention(nn.Module):
+
+ def __init__(self, config, is_cross_attention=False):
+ super().__init__()
+
+ self.self = BertSelfAttention(config, is_cross_attention)
+
+ self.output = BertSelfOutput(config)
+ self.pruned_heads = set()
+
+ def prune_heads(self, heads):
+ if len(heads) == 0:
+ return
+ heads, index = find_pruneable_heads_and_indices(
+ heads,
+ self.self.num_attention_heads,
+ self.self.attention_head_size,
+ self.pruned_heads,
+ )
+
+ # Prune linear layers
+ self.self.query = prune_linear_layer(self.self.query, index)
+ self.self.key = prune_linear_layer(self.self.key, index)
+ self.self.value = prune_linear_layer(self.self.value, index)
+ self.output.dense = prune_linear_layer(self.output.dense, index, dim=1)
+
+ # Update hyper params and store pruned heads
+ self.self.num_attention_heads = self.self.num_attention_heads - len(
+ heads)
+ self.self.all_head_size = self.self.attention_head_size * self.self.num_attention_heads
+ self.pruned_heads = self.pruned_heads.union(heads)
+
+ def forward(
+ self,
+ hidden_states,
+ attention_mask=None,
+ head_mask=None,
+ encoder_hidden_states=None,
+ encoder_attention_mask=None,
+ past_key_value=None,
+ output_attentions=False,
+ ):
+ self_outputs = self.self(
+ hidden_states,
+ attention_mask,
+ head_mask,
+ encoder_hidden_states,
+ encoder_attention_mask,
+ past_key_value,
+ output_attentions,
+ )
+ attention_output = self.output(self_outputs[0], hidden_states)
+ # add attentions if we output them
+ outputs = (attention_output, ) + self_outputs[1:]
+ return outputs # (context_layer, attention_probs, attention_scores, past_key_value,)
+
+
+class BertIntermediate(nn.Module):
+
+ def __init__(self, config):
+ super().__init__()
+ self.dense = nn.Linear(config.hidden_size, config.intermediate_size)
+ if isinstance(config.hidden_act, str):
+ self.intermediate_act_fn = ACT2FN[config.hidden_act]
+ else:
+ self.intermediate_act_fn = config.hidden_act
+
+ def forward(self, hidden_states):
+ hidden_states = self.dense(hidden_states)
+ hidden_states = self.intermediate_act_fn(hidden_states)
+ return hidden_states
+
+
+class BertOutput(nn.Module):
+
+ def __init__(self, config):
+ super().__init__()
+ self.dense = nn.Linear(config.intermediate_size, config.hidden_size)
+ self.LayerNorm = nn.LayerNorm(
+ config.hidden_size, eps=config.layer_norm_eps)
+ self.dropout = nn.Dropout(config.hidden_dropout_prob)
+
+ def forward(self, hidden_states, input_tensor):
+ hidden_states = self.dense(hidden_states)
+ hidden_states = self.dropout(hidden_states)
+ hidden_states = self.LayerNorm(hidden_states + input_tensor)
+ return hidden_states
+
+
+class BertLayer(nn.Module):
+
+ def __init__(self, config, layer_num):
+ super().__init__()
+ self.config = config
+ self.chunk_size_feed_forward = config.chunk_size_feed_forward
+ self.seq_len_dim = 1
+ self.attention = BertAttention(config)
+
+ self.has_cross_attention = layer_num >= config.fusion_layer
+ if self.has_cross_attention:
+ self.crossattention = BertAttention(
+ config, is_cross_attention=True)
+ self.intermediate = BertIntermediate(config)
+ self.output = BertOutput(config)
+
+ def forward(
+ self,
+ hidden_states,
+ attention_mask=None,
+ head_mask=None,
+ encoder_hidden_states=None,
+ encoder_attention_mask=None,
+ past_key_value=None,
+ output_attentions=False,
+ ):
+ # decoder uni-directional self-attention cached key/values tuple is at positions 1,2
+ self_attn_past_key_value = past_key_value[:
+ 2] if past_key_value is not None else None
+ self_attention_outputs = self.attention(
+ hidden_states,
+ attention_mask,
+ head_mask,
+ output_attentions=output_attentions,
+ past_key_value=self_attn_past_key_value,
+ ) # (context_layer, attention_probs, attention_scores, past_key_value,)
+ attention_output = self_attention_outputs[0]
+
+ outputs = self_attention_outputs[1:-1]
+ present_key_value = self_attention_outputs[-1]
+
+ if self.has_cross_attention:
+ assert (
+ encoder_hidden_states is not None
+ ), 'encoder_hidden_states must be given for cross-attention layers'
+
+ if type(encoder_hidden_states) == list:
+ cross_attention_outputs = self.crossattention(
+ attention_output,
+ attention_mask,
+ head_mask,
+ encoder_hidden_states[(self.layer_num -
+ self.config.fusion_layer) %
+ len(encoder_hidden_states)],
+ encoder_attention_mask[(self.layer_num -
+ self.config.fusion_layer) %
+ len(encoder_hidden_states)],
+ output_attentions=output_attentions,
+ )
+ attention_output = cross_attention_outputs[0]
+ outputs = outputs + cross_attention_outputs[1:-1]
+
+ else:
+ cross_attention_outputs = self.crossattention(
+ attention_output,
+ attention_mask,
+ head_mask,
+ encoder_hidden_states,
+ encoder_attention_mask,
+ output_attentions=output_attentions,
+ ) # (context_layer, attention_probs, attention_scores, past_key_value,)
+ attention_output = cross_attention_outputs[0]
+ # add cross attentions if we output attention weights
+ outputs = outputs + cross_attention_outputs[1:-1]
+ layer_output = apply_chunking_to_forward(
+ self.feed_forward_chunk,
+ self.chunk_size_feed_forward,
+ self.seq_len_dim,
+ attention_output,
+ )
+ outputs = (layer_output, ) + outputs
+
+ outputs = outputs + (present_key_value, )
+
+ return outputs
+
+ def feed_forward_chunk(self, attention_output):
+ intermediate_output = self.intermediate(attention_output)
+ layer_output = self.output(intermediate_output, attention_output)
+ return layer_output
+
+
+class BertEncoder(nn.Module):
+
+ def __init__(self, config):
+ super().__init__()
+ self.config = config
+ self.layer = nn.ModuleList(
+ [BertLayer(config, i) for i in range(config.num_hidden_layers)])
+ logger = MMLogger.get_current_instance()
+ logger.info(f'build bert with cross_module: {config.cross_module}')
+
+ def forward(
+ self,
+ hidden_states,
+ attention_mask=None,
+ head_mask=None,
+ encoder_hidden_states=None,
+ encoder_attention_mask=None,
+ past_key_values=None,
+ use_cache=None,
+ output_attentions=False,
+ output_hidden_states=False,
+ return_dict=True,
+ mode='multi_modal',
+ normalize_attention=True,
+ ):
+ all_hidden_states = () if output_hidden_states else None
+ all_self_attentions = () if output_attentions else None
+ # all_cross_attentions = () if output_attentions and self.config.add_cross_attention else None
+ all_cross_attentions = () if output_attentions else None
+
+ next_decoder_cache = () if use_cache else None
+
+ if (mode == 'text' or mode == 'temporal'
+ ): # temporal is added and used for temporal att module.
+ start_layer = 0
+ output_layer = self.config.fusion_layer
+
+ elif mode == 'fusion':
+ start_layer = self.config.fusion_layer
+ output_layer = self.config.num_hidden_layers
+
+ elif mode == 'multi_modal':
+ start_layer = 0
+ output_layer = self.config.num_hidden_layers
+
+ for i in range(start_layer, output_layer):
+ layer_module = self.layer[i]
+ if output_hidden_states:
+ all_hidden_states = all_hidden_states + (hidden_states, )
+
+ layer_head_mask = head_mask[i] if head_mask is not None else None
+ past_key_value = past_key_values[
+ i] if past_key_values is not None else None
+
+ if getattr(self.config, 'gradient_checkpointing',
+ False) and self.training:
+
+ if use_cache:
+ logger = MMLogger.get_current_instance()
+ logger.warn(
+ '`use_cache=True` is incompatible with `config.gradient_checkpointing=True`. Setting '
+ '`use_cache=False`...')
+ use_cache = False
+
+ def create_custom_forward(module):
+
+ def custom_forward(*inputs):
+ return module(*inputs, past_key_value,
+ output_attentions)
+
+ return custom_forward
+
+ layer_outputs = torch.utils.checkpoint.checkpoint(
+ create_custom_forward(layer_module),
+ hidden_states,
+ attention_mask,
+ layer_head_mask,
+ encoder_hidden_states,
+ encoder_attention_mask,
+ use_reentrant=False,
+ )
+ else:
+ layer_outputs = layer_module(
+ hidden_states,
+ attention_mask,
+ layer_head_mask,
+ encoder_hidden_states,
+ encoder_attention_mask,
+ past_key_value,
+ output_attentions,
+ ) # (context_layer, attention_probs, attention_scores, past_key_value,)
+ hidden_states = layer_outputs[0]
+ if use_cache:
+ next_decoder_cache += (layer_outputs[-1], )
+ if output_attentions:
+ # whether to output normalized attention,
+ # note for unnormalized attention, there is a mask added
+ offset = int(normalize_attention)
+ # all_self_attentions = all_self_attentions + (layer_outputs[1], )
+ all_self_attentions = all_self_attentions + (
+ layer_outputs[2 - offset], )
+ if hasattr(layer_module, 'crossattention'):
+ # all_cross_attentions = all_cross_attentions + (layer_outputs[3], )
+ all_cross_attentions = all_cross_attentions + (
+ layer_outputs[4 - offset], )
+
+ if output_hidden_states:
+ all_hidden_states = all_hidden_states + (hidden_states, )
+
+ if not return_dict:
+ return tuple(v for v in [
+ hidden_states,
+ next_decoder_cache,
+ all_hidden_states,
+ all_self_attentions,
+ all_cross_attentions,
+ ] if v is not None)
+ return BaseModelOutputWithPastAndCrossAttentions(
+ last_hidden_state=hidden_states,
+ past_key_values=next_decoder_cache,
+ hidden_states=all_hidden_states,
+ attentions=all_self_attentions,
+ cross_attentions=all_cross_attentions,
+ )
+
+
+class BertPooler(nn.Module):
+
+ def __init__(self, config):
+ super().__init__()
+ self.dense = nn.Linear(config.hidden_size, config.hidden_size)
+ self.activation = nn.Tanh()
+
+ def forward(self, hidden_states):
+ # We "pool" the model by simply taking the hidden state corresponding
+ # to the first token.
+ first_token_tensor = hidden_states[:, 0]
+ pooled_output = self.dense(first_token_tensor)
+ pooled_output = self.activation(pooled_output)
+ return pooled_output
+
+
+class BertPredictionHeadTransform(nn.Module):
+
+ def __init__(self, config):
+ super().__init__()
+ self.dense = nn.Linear(config.hidden_size, config.hidden_size)
+ if isinstance(config.hidden_act, str):
+ self.transform_act_fn = ACT2FN[config.hidden_act]
+ else:
+ self.transform_act_fn = config.hidden_act
+ self.LayerNorm = nn.LayerNorm(
+ config.hidden_size, eps=config.layer_norm_eps)
+
+ def forward(self, hidden_states):
+ hidden_states = self.dense(hidden_states)
+ hidden_states = self.transform_act_fn(hidden_states)
+ hidden_states = self.LayerNorm(hidden_states)
+ return hidden_states
+
+
+class BertLMPredictionHead(nn.Module):
+
+ def __init__(self, config):
+ super().__init__()
+ self.transform = BertPredictionHeadTransform(config)
+
+ # The output weights are the same as the input embeddings, but there is
+ # an output-only bias for each token.
+ self.decoder = nn.Linear(
+ config.hidden_size, config.vocab_size, bias=False)
+
+ self.bias = nn.Parameter(torch.zeros(config.vocab_size))
+
+ # Need a link between the two variables so that the bias is correctly resized with `resize_token_embeddings`
+ self.decoder.bias = self.bias
+
+ def forward(self, hidden_states):
+ hidden_states = self.transform(hidden_states)
+ hidden_states = self.decoder(hidden_states)
+ return hidden_states
+
+
+class BertOnlyMLMHead(nn.Module):
+
+ def __init__(self, config):
+ super().__init__()
+ self.predictions = BertLMPredictionHead(config)
+
+ def forward(self, sequence_output):
+ prediction_scores = self.predictions(sequence_output)
+ return prediction_scores
+
+
+class BertOnlyNSPHead(nn.Module):
+
+ def __init__(self, config):
+ super().__init__()
+ self.seq_relationship = nn.Linear(config.hidden_size, 2)
+
+ def forward(self, pooled_output):
+ seq_relationship_score = self.seq_relationship(pooled_output)
+ return seq_relationship_score
+
+
+class BertPreTrainingHeads(nn.Module):
+
+ def __init__(self, config):
+ super().__init__()
+ self.predictions = BertLMPredictionHead(config)
+ self.seq_relationship = nn.Linear(config.hidden_size, 2)
+
+ def forward(self, sequence_output, pooled_output):
+ prediction_scores = self.predictions(sequence_output)
+ seq_relationship_score = self.seq_relationship(pooled_output)
+ return prediction_scores, seq_relationship_score
+
+
+class BertPreTrainedModel(PreTrainedModel):
+ """An abstract class to handle weights initialization and a simple
+ interface for downloading and loading pretrained models."""
+
+ config_class = BertConfig
+ load_tf_weights = load_tf_weights_in_bert
+ base_model_prefix = 'bert'
+ _keys_to_ignore_on_load_missing = [r'position_ids']
+
+ def _init_weights(self, module):
+ """Initialize the weights."""
+ if isinstance(module, (nn.Linear, nn.Embedding)):
+ # Slightly different from the TF version which uses truncated_normal for initialization
+ # cf https://github.com/pytorch/pytorch/pull/5617
+ module.weight.data.normal_(
+ mean=0.0, std=self.config.initializer_range)
+ elif isinstance(module, nn.LayerNorm):
+ module.bias.data.zero_()
+ module.weight.data.fill_(1.0)
+ if isinstance(module, nn.Linear) and module.bias is not None:
+ module.bias.data.zero_()
+
+
+@dataclass
+class BertForPreTrainingOutput(ModelOutput):
+ """Output type of :class:`~transformers.BertForPreTraining`.
+
+ Args:
+ loss (`optional`, returned when ``labels`` is provided, ``torch.FloatTensor`` of shape :obj:`(1,)`):
+ Total loss as the sum of the masked language modeling loss and the next sequence prediction
+ (classification) loss.
+ prediction_logits (:obj:`torch.FloatTensor` of shape :obj:`(batch_size, sequence_length, config.vocab_size)`):
+ Prediction scores of the language modeling head (scores for each vocabulary token before SoftMax).
+ seq_relationship_logits (:obj:`torch.FloatTensor` of shape :obj:`(batch_size, 2)`):
+ Prediction scores of the next sequence prediction (classification) head (scores of True/False continuation
+ before SoftMax).
+ hidden_states (:obj:`tuple(torch.FloatTensor)`, `optional`, returned when ``output_hidden_states=True`` is passed or when ``config.output_hidden_states=True``):
+ Tuple of :obj:`torch.FloatTensor` (one for the output of the embeddings + one for the output of each layer)
+ of shape :obj:`(batch_size, sequence_length, hidden_size)`.
+ Hidden-states of the model at the output of each layer plus the initial embedding outputs.
+ attentions (:obj:`tuple(torch.FloatTensor)`, `optional`, returned when ``output_attentions=True`` is passed or when ``config.output_attentions=True``):
+ Tuple of :obj:`torch.FloatTensor` (one for each layer) of shape :obj:`(batch_size, num_heads,
+ sequence_length, sequence_length)`.
+ Attentions weights after the attention softmax, used to compute the weighted average in the self-attention
+ heads.
+ """
+
+ loss: Optional[torch.FloatTensor] = None
+ prediction_logits: torch.FloatTensor = None
+ seq_relationship_logits: torch.FloatTensor = None
+ hidden_states: Optional[Tuple[torch.FloatTensor]] = None
+ attentions: Optional[Tuple[torch.FloatTensor]] = None
+
+
+BERT_START_DOCSTRING = r"""
+ This model inherits from :class:`~transformers.PreTrainedModel`. Check the superclass documentation for the generic
+ methods the library implements for all its model (such as downloading or saving, resizing the input embeddings,
+ pruning heads etc.)
+ This model is also a PyTorch `torch.nn.Module `__
+ subclass. Use it as a regular PyTorch Module and refer to the PyTorch documentation for all matter related to
+ general usage and behavior.
+ Parameters:
+ config (:class:`~transformers.BertConfig`): Model configuration class with all the parameters of the model.
+ Initializing with a config file does not load the weights associated with the model, only the
+ configuration. Check out the :meth:`~transformers.PreTrainedModel.from_pretrained` method to load the model
+ weights.
+"""
+
+BERT_INPUTS_DOCSTRING = r"""
+ Args:
+ input_ids (:obj:`torch.LongTensor` of shape :obj:`({0})`):
+ Indices of input sequence tokens in the vocabulary.
+ Indices can be obtained using :class:`~transformers.BertTokenizer`. See
+ :meth:`transformers.PreTrainedTokenizer.encode` and :meth:`transformers.PreTrainedTokenizer.__call__` for
+ details.
+ `What are input IDs? <../glossary.html#input-ids>`__
+ attention_mask (:obj:`torch.FloatTensor` of shape :obj:`({0})`, `optional`):
+ Mask to avoid performing attention on padding token indices. Mask values selected in ``[0, 1]``:
+ - 1 for tokens that are **not masked**,
+ - 0 for tokens that are **masked**.
+ `What are attention masks? <../glossary.html#attention-mask>`__
+ token_type_ids (:obj:`torch.LongTensor` of shape :obj:`({0})`, `optional`):
+ Segment token indices to indicate first and second portions of the inputs. Indices are selected in ``[0,
+ 1]``:
+ - 0 corresponds to a `sentence A` token,
+ - 1 corresponds to a `sentence B` token.
+ `What are token type IDs? <../glossary.html#token-type-ids>`_
+ position_ids (:obj:`torch.LongTensor` of shape :obj:`({0})`, `optional`):
+ Indices of positions of each input sequence tokens in the position embeddings. Selected in the range ``[0,
+ config.max_position_embeddings - 1]``.
+ `What are position IDs? <../glossary.html#position-ids>`_
+ head_mask (:obj:`torch.FloatTensor` of shape :obj:`(num_heads,)` or :obj:`(num_layers, num_heads)`, `optional`):
+ Mask to nullify selected heads of the self-attention modules. Mask values selected in ``[0, 1]``:
+ - 1 indicates the head is **not masked**,
+ - 0 indicates the head is **masked**.
+ inputs_embeds (:obj:`torch.FloatTensor` of shape :obj:`({0}, hidden_size)`, `optional`):
+ Optionally, instead of passing :obj:`input_ids` you can choose to directly pass an embedded representation.
+ This is useful if you want more control over how to convert :obj:`input_ids` indices into associated
+ vectors than the model's internal embedding lookup matrix.
+ output_attentions (:obj:`bool`, `optional`):
+ Whether or not to return the attentions tensors of all attention layers. See ``attentions`` under returned
+ tensors for more detail.
+ output_hidden_states (:obj:`bool`, `optional`):
+ Whether or not to return the hidden states of all layers. See ``hidden_states`` under returned tensors for
+ more detail.
+ return_dict (:obj:`bool`, `optional`):
+ Whether or not to return a :class:`~transformers.file_utils.ModelOutput` instead of a plain tuple.
+"""
+
+
+@add_start_docstrings(
+ 'The bare Bert Model transformer outputting raw hidden-states without any specific head on top.',
+ BERT_START_DOCSTRING,
+)
+class BertModel(BertPreTrainedModel):
+ """The model can behave as an encoder (with only self-attention) as well as
+ a decoder, in which case a layer of cross-attention is added between the
+ self-attention layers, following the architecture described in `Attention
+ is all you need `__ by Ashish Vaswani,
+ Noam Shazeer, Niki Parmar, Jakob Uszkoreit, Llion Jones, Aidan N.
+
+ Gomez, Lukasz Kaiser and Illia Polosukhin. argument and
+ :obj:`add_cross_attention` set to :obj:`True`; an
+ :obj:`encoder_hidden_states` is then expected as an input to the forward
+ pass.
+ """
+
+ def __init__(self, config, add_pooling_layer=True):
+ super().__init__(config)
+ self.config = config
+
+ self.embeddings = BertEmbeddings(config)
+
+ self.encoder = BertEncoder(config)
+
+ self.pooler = BertPooler(config) if add_pooling_layer else None
+
+ self.init_weights()
+
+ def get_input_embeddings(self):
+ return self.embeddings.word_embeddings
+
+ def set_input_embeddings(self, value):
+ self.embeddings.word_embeddings = value
+
+ def _prune_heads(self, heads_to_prune):
+ """Prunes heads of the model.
+
+ heads_to_prune: dict of {layer_num: list of heads to prune in this layer} See base
+ class PreTrainedModel
+ """
+ for layer, heads in heads_to_prune.items():
+ self.encoder.layer[layer].attention.prune_heads(heads)
+
+ def get_extended_attention_mask(self, attention_mask: Tensor,
+ input_shape: Tuple[int], device: device,
+ is_decoder: bool) -> Tensor:
+ """Makes broadcastable attention and causal masks so that future and
+ masked tokens are ignored.
+
+ Arguments:
+ attention_mask (:obj:`torch.Tensor`):
+ Mask with ones indicating tokens to attend to, zeros for tokens to ignore.
+ input_shape (:obj:`Tuple[int]`):
+ The shape of the input to the model.
+ device: (:obj:`torch.device`):
+ The device of the input to the model.
+
+ Returns:
+ :obj:`torch.Tensor` The extended attention mask, with a the same dtype as :obj:`attention_mask.dtype`.
+ """
+ # We can provide a self-attention mask of dimensions [batch_size, from_seq_length, to_seq_length]
+ # ourselves in which case we just need to make it broadcastable to all heads.
+ if attention_mask.dim() == 3:
+ extended_attention_mask = attention_mask[:, None, :, :]
+ elif attention_mask.dim() == 2:
+ # Provided a padding mask of dimensions [batch_size, seq_length]
+ # - if the model is a decoder, apply a causal mask in addition to the padding mask
+ # - if the model is an encoder, make the mask broadcastable to [batch_size, num_heads, seq_length, seq_length]
+ if is_decoder:
+ batch_size, seq_length = input_shape
+ seq_ids = torch.arange(seq_length, device=device)
+ causal_mask = (
+ seq_ids[None, None, :].repeat(batch_size, seq_length, 1) <=
+ seq_ids[None, :, None])
+ # in case past_key_values are used we need to add a prefix ones mask to the causal mask
+ # causal and attention masks must have same type with pytorch version < 1.3
+ causal_mask = causal_mask.to(attention_mask.dtype)
+
+ if causal_mask.shape[1] < attention_mask.shape[1]:
+ prefix_seq_len = attention_mask.shape[
+ 1] - causal_mask.shape[1]
+ causal_mask = torch.cat(
+ [
+ torch.ones(
+ (batch_size, seq_length, prefix_seq_len),
+ device=device,
+ dtype=causal_mask.dtype,
+ ),
+ causal_mask,
+ ],
+ axis=-1,
+ )
+
+ extended_attention_mask = (
+ causal_mask[:, None, :, :] *
+ attention_mask[:, None, None, :])
+ else:
+ extended_attention_mask = attention_mask[:, None, None, :]
+ else:
+ raise ValueError(
+ 'Wrong shape for input_ids (shape {}) or attention_mask (shape {})'
+ .format(input_shape, attention_mask.shape))
+
+ # Since attention_mask is 1.0 for positions we want to attend and 0.0 for
+ # masked positions, this operation will create a tensor which is 0.0 for
+ # positions we want to attend and -10000.0 for masked positions.
+ # Since we are adding it to the raw scores before the softmax, this is
+ # effectively the same as removing these entirely.
+ extended_attention_mask = extended_attention_mask.to(
+ dtype=self.dtype) # fp16 compatibility
+ extended_attention_mask = (1.0 - extended_attention_mask) * -10000.0
+ return extended_attention_mask
+
+ def forward(
+ self,
+ input_ids=None,
+ attention_mask=None,
+ token_type_ids=None,
+ position_ids=None,
+ head_mask=None,
+ inputs_embeds=None,
+ encoder_embeds=None,
+ encoder_hidden_states=None,
+ encoder_attention_mask=None,
+ past_key_values=None,
+ use_cache=None,
+ output_attentions=None,
+ output_hidden_states=None,
+ return_dict=None,
+ is_decoder=False,
+ mode='multi_modal',
+ normalize_attention=True,
+ ):
+ r"""
+ encoder_hidden_states (:obj:`torch.FloatTensor` of shape :obj:`(batch_size, sequence_length, hidden_size)`, `optional`):
+ Sequence of hidden-states at the output of the last layer of the encoder. Used in the cross-attention if
+ the model is configured as a decoder.
+ encoder_attention_mask (:obj:`torch.FloatTensor` of shape :obj:`(batch_size, sequence_length)`, `optional`):
+ Mask to avoid performing attention on the padding token indices of the encoder input. This mask is used in
+ the cross-attention if the model is configured as a decoder. Mask values selected in ``[0, 1]``:
+ - 1 for tokens that are **not masked**,
+ - 0 for tokens that are **masked**.
+ past_key_values (:obj:`tuple(tuple(torch.FloatTensor))` of length :obj:`config.n_layers` with each tuple having 4 tensors of shape :obj:`(batch_size, num_heads, sequence_length - 1, embed_size_per_head)`):
+ Contains precomputed key and value hidden states of the attention blocks. Can be used to speed up decoding.
+ If :obj:`past_key_values` are used, the user can optionally input only the last :obj:`decoder_input_ids`
+ (those that don't have their past key value states given to this model) of shape :obj:`(batch_size, 1)`
+ instead of all :obj:`decoder_input_ids` of shape :obj:`(batch_size, sequence_length)`.
+ use_cache (:obj:`bool`, `optional`):
+ If set to :obj:`True`, :obj:`past_key_values` key value states are returned and can be used to speed up
+ decoding (see :obj:`past_key_values`).
+ """
+ output_attentions = (
+ output_attentions if output_attentions is not None else
+ self.config.output_attentions)
+ output_hidden_states = (
+ output_hidden_states if output_hidden_states is not None else
+ self.config.output_hidden_states)
+ return_dict = return_dict if return_dict is not None else self.config.use_return_dict
+
+ if is_decoder:
+ use_cache = use_cache if use_cache is not None else self.config.use_cache
+ else:
+ use_cache = False
+
+ if input_ids is not None and inputs_embeds is not None:
+ raise ValueError(
+ 'You cannot specify both input_ids and inputs_embeds at the same time'
+ )
+ elif input_ids is not None:
+ input_shape = input_ids.size()
+ batch_size, seq_length = input_shape
+ device = input_ids.device
+ elif inputs_embeds is not None:
+ input_shape = inputs_embeds.size()[:-1]
+ batch_size, seq_length = input_shape
+ device = inputs_embeds.device
+ elif encoder_embeds is not None:
+ input_shape = encoder_embeds.size()[:-1]
+ batch_size, seq_length = input_shape
+ device = encoder_embeds.device
+ else:
+ raise ValueError(
+ 'You have to specify either input_ids or inputs_embeds or encoder_embeds'
+ )
+
+ # past_key_values_length
+ past_key_values_length = (
+ past_key_values[0][0].shape[2]
+ if past_key_values is not None else 0)
+
+ if attention_mask is None:
+ attention_mask = torch.ones(
+ ((batch_size, seq_length + past_key_values_length)),
+ device=device)
+ if token_type_ids is None:
+ token_type_ids = torch.zeros(
+ input_shape, dtype=torch.long, device=device)
+
+ # We can provide a self-attention mask of dimensions [batch_size, from_seq_length, to_seq_length]
+ # ourselves in which case we just need to make it broadcastable to all heads.
+ extended_attention_mask: torch.Tensor = self.get_extended_attention_mask(
+ attention_mask, input_shape, device, is_decoder)
+
+ # If a 2D or 3D attention mask is provided for the cross-attention
+ # we need to make broadcastable to [batch_size, num_heads, seq_length, seq_length]
+ if encoder_hidden_states is not None:
+ if type(encoder_hidden_states) == list:
+ encoder_batch_size, encoder_sequence_length, _ = encoder_hidden_states[
+ 0].size()
+ else:
+ encoder_batch_size, encoder_sequence_length, _ = encoder_hidden_states.size(
+ )
+ encoder_hidden_shape = (encoder_batch_size,
+ encoder_sequence_length)
+
+ if type(encoder_attention_mask) == list:
+ encoder_extended_attention_mask = [
+ self.invert_attention_mask(mask)
+ for mask in encoder_attention_mask
+ ]
+ elif encoder_attention_mask is None:
+ encoder_attention_mask = torch.ones(
+ encoder_hidden_shape, device=device)
+ encoder_extended_attention_mask = self.invert_attention_mask(
+ encoder_attention_mask)
+ else:
+ encoder_extended_attention_mask = self.invert_attention_mask(
+ encoder_attention_mask)
+ else:
+ encoder_extended_attention_mask = None
+
+ # Prepare head mask if needed
+ # 1.0 in head_mask indicate we keep the head
+ # attention_probs has shape bsz x n_heads x N x N
+ # input head_mask has shape [num_heads] or [num_hidden_layers x num_heads]
+ # and head_mask is converted to shape [num_hidden_layers x batch x num_heads x seq_length x seq_length]
+ head_mask = self.get_head_mask(head_mask,
+ self.config.num_hidden_layers)
+
+ if encoder_embeds is None:
+ embedding_output = self.embeddings(
+ input_ids=input_ids,
+ position_ids=position_ids,
+ token_type_ids=token_type_ids,
+ inputs_embeds=inputs_embeds,
+ past_key_values_length=past_key_values_length,
+ )
+ else:
+ embedding_output = encoder_embeds
+
+ encoder_outputs = self.encoder(
+ embedding_output,
+ attention_mask=extended_attention_mask,
+ head_mask=head_mask,
+ encoder_hidden_states=encoder_hidden_states,
+ encoder_attention_mask=encoder_extended_attention_mask,
+ past_key_values=past_key_values,
+ use_cache=use_cache,
+ output_attentions=output_attentions,
+ output_hidden_states=output_hidden_states,
+ return_dict=return_dict,
+ mode=mode,
+ normalize_attention=normalize_attention,
+ )
+ sequence_output = encoder_outputs[0]
+ pooled_output = self.pooler(
+ sequence_output) if self.pooler is not None else None
+
+ if not return_dict:
+ return (sequence_output, pooled_output) + encoder_outputs[1:]
+
+ return BaseModelOutputWithPoolingAndCrossAttentions(
+ last_hidden_state=sequence_output,
+ pooler_output=pooled_output,
+ past_key_values=encoder_outputs.past_key_values,
+ hidden_states=encoder_outputs.hidden_states,
+ attentions=encoder_outputs.attentions,
+ cross_attentions=encoder_outputs.cross_attentions,
+ )
+
+
+@add_start_docstrings(
+ """
+ Bert Model with two heads on top as done during the pretraining: a `masked language modeling` head and a `next
+ sentence prediction (classification)` head.
+ """,
+ BERT_START_DOCSTRING,
+)
+class BertForPreTraining(BertPreTrainedModel):
+
+ def __init__(self, config):
+ super().__init__(config)
+
+ self.bert = BertModel(config)
+ self.cls = BertPreTrainingHeads(config)
+
+ self.init_weights()
+
+ def get_output_embeddings(self):
+ return self.cls.predictions.decoder
+
+ def set_output_embeddings(self, new_embeddings):
+ self.cls.predictions.decoder = new_embeddings
+
+ @add_start_docstrings_to_model_forward(
+ BERT_INPUTS_DOCSTRING.format('batch_size, sequence_length'))
+ @replace_return_docstrings(
+ output_type=BertForPreTrainingOutput, config_class=_CONFIG_FOR_DOC)
+ def forward(
+ self,
+ input_ids=None,
+ attention_mask=None,
+ token_type_ids=None,
+ position_ids=None,
+ head_mask=None,
+ inputs_embeds=None,
+ labels=None,
+ next_sentence_label=None,
+ output_attentions=None,
+ output_hidden_states=None,
+ return_dict=None,
+ ):
+ r"""
+ labels (:obj:`torch.LongTensor` of shape ``(batch_size, sequence_length)``, `optional`):
+ Labels for computing the masked language modeling loss. Indices should be in ``[-100, 0, ...,
+ config.vocab_size]`` (see ``input_ids`` docstring) Tokens with indices set to ``-100`` are ignored
+ (masked), the loss is only computed for the tokens with labels in ``[0, ..., config.vocab_size]``
+ next_sentence_label (``torch.LongTensor`` of shape ``(batch_size,)``, `optional`):
+ Labels for computing the next sequence prediction (classification) loss. Input should be a sequence pair
+ (see :obj:`input_ids` docstring) Indices should be in ``[0, 1]``:
+ - 0 indicates sequence B is a continuation of sequence A,
+ - 1 indicates sequence B is a random sequence.
+ kwargs (:obj:`Dict[str, any]`, optional, defaults to `{}`):
+ Used to hide legacy arguments that have been deprecated.
+ Returns:
+ Example::
+ >>> from transformers import BertTokenizer, BertForPreTraining
+ >>> import torch
+ >>> tokenizer = BertTokenizer.from_pretrained('bert-base-uncased')
+ >>> model = BertForPreTraining.from_pretrained('bert-base-uncased')
+ >>> inputs = tokenizer("Hello, my dog is cute", return_tensors="pt")
+ >>> outputs = model(**inputs)
+ >>> prediction_logits = outputs.prediction_logits
+ >>> seq_relationship_logits = outputs.seq_relationship_logits
+ """
+ return_dict = return_dict if return_dict is not None else self.config.use_return_dict
+
+ outputs = self.bert(
+ input_ids,
+ attention_mask=attention_mask,
+ token_type_ids=token_type_ids,
+ position_ids=position_ids,
+ head_mask=head_mask,
+ inputs_embeds=inputs_embeds,
+ output_attentions=output_attentions,
+ output_hidden_states=output_hidden_states,
+ return_dict=return_dict,
+ )
+
+ sequence_output, pooled_output = outputs[:2]
+ prediction_scores, seq_relationship_score = self.cls(
+ sequence_output, pooled_output)
+
+ total_loss = None
+ if labels is not None and next_sentence_label is not None:
+ loss_fct = CrossEntropyLoss()
+ masked_lm_loss = loss_fct(
+ prediction_scores.view(-1, self.config.vocab_size),
+ labels.view(-1))
+ next_sentence_loss = loss_fct(
+ seq_relationship_score.view(-1, 2),
+ next_sentence_label.view(-1))
+ total_loss = masked_lm_loss + next_sentence_loss
+
+ if not return_dict:
+ output = (prediction_scores, seq_relationship_score) + outputs[2:]
+ return ((total_loss, ) +
+ output) if total_loss is not None else output
+
+ return BertForPreTrainingOutput(
+ loss=total_loss,
+ prediction_logits=prediction_scores,
+ seq_relationship_logits=seq_relationship_score,
+ hidden_states=outputs.hidden_states,
+ attentions=outputs.attentions,
+ )
+
+
+@add_start_docstrings(
+ """Bert Model with a `language modeling` head on top for CLM fine-tuning. """,
+ BERT_START_DOCSTRING,
+)
+class BertLMHeadModel(BertPreTrainedModel):
+
+ _keys_to_ignore_on_load_unexpected = [r'pooler']
+ _keys_to_ignore_on_load_missing = [
+ r'position_ids', r'predictions.decoder.bias'
+ ]
+
+ def __init__(self, config):
+ super().__init__(config)
+
+ self.bert = BertModel(config, add_pooling_layer=False)
+ self.cls = BertOnlyMLMHead(config)
+
+ self.init_weights()
+
+ def get_output_embeddings(self):
+ return self.cls.predictions.decoder
+
+ def set_output_embeddings(self, new_embeddings):
+ self.cls.predictions.decoder = new_embeddings
+
+ @add_start_docstrings_to_model_forward(
+ BERT_INPUTS_DOCSTRING.format('batch_size, sequence_length'))
+ @replace_return_docstrings(
+ output_type=CausalLMOutputWithCrossAttentions,
+ config_class=_CONFIG_FOR_DOC)
+ def forward(
+ self,
+ input_ids=None,
+ attention_mask=None,
+ token_type_ids=None,
+ position_ids=None,
+ head_mask=None,
+ inputs_embeds=None,
+ encoder_hidden_states=None,
+ encoder_attention_mask=None,
+ labels=None,
+ past_key_values=None,
+ use_cache=None,
+ output_attentions=None,
+ output_hidden_states=None,
+ return_dict=None,
+ is_decoder=True,
+ reduction='mean',
+ mode='multi_modal',
+ normalize_attention=True,
+ soft_labels=None,
+ alpha=0,
+ return_logits=False,
+ ):
+ r"""
+ encoder_hidden_states (:obj:`torch.FloatTensor` of shape :obj:`(batch_size, sequence_length, hidden_size)`, `optional`):
+ Sequence of hidden-states at the output of the last layer of the encoder. Used in the cross-attention if
+ the model is configured as a decoder.
+ encoder_attention_mask (:obj:`torch.FloatTensor` of shape :obj:`(batch_size, sequence_length)`, `optional`):
+ Mask to avoid performing attention on the padding token indices of the encoder input. This mask is used in
+ the cross-attention if the model is configured as a decoder. Mask values selected in ``[0, 1]``:
+ - 1 for tokens that are **not masked**,
+ - 0 for tokens that are **masked**.
+ labels (:obj:`torch.LongTensor` of shape :obj:`(batch_size, sequence_length)`, `optional`):
+ Labels for computing the left-to-right language modeling loss (next word prediction). Indices should be in
+ ``[-100, 0, ..., config.vocab_size]`` (see ``input_ids`` docstring) Tokens with indices set to ``-100`` are
+ ignored (masked), the loss is only computed for the tokens with labels n ``[0, ..., config.vocab_size]``
+ past_key_values (:obj:`tuple(tuple(torch.FloatTensor))` of length :obj:`config.n_layers` with each tuple having 4 tensors of shape :obj:`(batch_size, num_heads, sequence_length - 1, embed_size_per_head)`):
+ Contains precomputed key and value hidden states of the attention blocks. Can be used to speed up decoding.
+ If :obj:`past_key_values` are used, the user can optionally input only the last :obj:`decoder_input_ids`
+ (those that don't have their past key value states given to this model) of shape :obj:`(batch_size, 1)`
+ instead of all :obj:`decoder_input_ids` of shape :obj:`(batch_size, sequence_length)`.
+ use_cache (:obj:`bool`, `optional`):
+ If set to :obj:`True`, :obj:`past_key_values` key value states are returned and can be used to speed up
+ decoding (see :obj:`past_key_values`).
+ Returns:
+ Example::
+ >>> from transformers import BertTokenizer, BertLMHeadModel, BertConfig
+ >>> import torch
+ >>> tokenizer = BertTokenizer.from_pretrained('bert-base-cased')
+ >>> config = BertConfig.from_pretrained("bert-base-cased")
+ >>> model = BertLMHeadModel.from_pretrained('bert-base-cased', config=config)
+ >>> inputs = tokenizer("Hello, my dog is cute", return_tensors="pt")
+ >>> outputs = model(**inputs)
+ >>> prediction_logits = outputs.logits
+ """
+ return_dict = return_dict if return_dict is not None else self.config.use_return_dict
+ if labels is not None:
+ use_cache = False
+
+ outputs = self.bert(
+ input_ids,
+ attention_mask=attention_mask,
+ token_type_ids=token_type_ids,
+ position_ids=position_ids,
+ head_mask=head_mask,
+ inputs_embeds=inputs_embeds,
+ encoder_hidden_states=encoder_hidden_states,
+ encoder_attention_mask=encoder_attention_mask,
+ past_key_values=past_key_values,
+ use_cache=use_cache,
+ output_attentions=output_attentions,
+ output_hidden_states=output_hidden_states,
+ return_dict=return_dict,
+ is_decoder=is_decoder,
+ mode=mode,
+ normalize_attention=normalize_attention,
+ )
+
+ sequence_output = outputs[0]
+ prediction_scores = self.cls(sequence_output)
+
+ if return_logits:
+ return prediction_scores[:, :-1, :].contiguous()
+
+ lm_loss = None
+ if labels is not None:
+ # we are doing next-token prediction; shift prediction scores and input ids by one
+ shifted_prediction_scores = prediction_scores[:, :
+ -1, :].contiguous()
+ labels = labels[:, 1:].contiguous()
+ loss_fct = CrossEntropyLoss(reduction=reduction)
+ lm_loss = loss_fct(
+ shifted_prediction_scores.view(-1, self.config.vocab_size),
+ labels.view(-1))
+ lm_loss = lm_loss.view(prediction_scores.size(0), -1).sum(1)
+
+ if soft_labels is not None:
+ loss_distill = -torch.sum(
+ F.log_softmax(shifted_prediction_scores, dim=1) * soft_labels,
+ dim=-1)
+ loss_distill = (loss_distill * (labels != -100)).sum(1)
+ lm_loss = (1 - alpha) * lm_loss + alpha * loss_distill
+
+ if not return_dict:
+ output = (prediction_scores, ) + outputs[2:]
+ return ((lm_loss, ) + output) if lm_loss is not None else output
+
+ return CausalLMOutputWithCrossAttentions(
+ loss=lm_loss,
+ logits=prediction_scores,
+ past_key_values=outputs.past_key_values,
+ hidden_states=outputs.hidden_states,
+ attentions=outputs.attentions,
+ cross_attentions=outputs.cross_attentions,
+ )
+
+ def prepare_inputs_for_generation(self,
+ input_ids,
+ past=None,
+ attention_mask=None,
+ **model_kwargs):
+ input_shape = input_ids.shape
+ # if model is used as a decoder in encoder-decoder model, the decoder attention mask is created on the fly
+ if attention_mask is None:
+ attention_mask = input_ids.new_ones(input_shape)
+
+ # cut decoder_input_ids if past is used
+ if past is not None:
+ input_ids = input_ids[:, -1:]
+
+ return {
+ 'input_ids':
+ input_ids,
+ 'attention_mask':
+ attention_mask,
+ 'past_key_values':
+ past,
+ 'encoder_hidden_states':
+ model_kwargs.get('encoder_hidden_states', None),
+ 'encoder_attention_mask':
+ model_kwargs.get('encoder_attention_mask', None),
+ 'is_decoder':
+ True,
+ }
+
+ def _reorder_cache(self, past, beam_idx):
+ reordered_past = ()
+ for layer_past in past:
+ reordered_past += (tuple(
+ past_state.index_select(0, beam_idx)
+ for past_state in layer_past), )
+ return reordered_past
+
+
+@dataclass
+class MaskedLMOutputWithDistill(MaskedLMOutput):
+ loss_aux: Optional[torch.FloatTensor] = None
+ loss_distill: Optional[torch.FloatTensor] = None
+
+
+@add_start_docstrings(
+ """Bert Model with a `language modeling` head on top. """,
+ BERT_START_DOCSTRING)
+class BertForMaskedLM(BertPreTrainedModel):
+
+ _keys_to_ignore_on_load_unexpected = [r'pooler']
+ _keys_to_ignore_on_load_missing = [
+ r'position_ids', r'predictions.decoder.bias'
+ ]
+
+ def __init__(self, config):
+ super().__init__(config)
+
+ self.bert = BertModel(config, add_pooling_layer=False)
+ self.cls = BertOnlyMLMHead(config)
+
+ self.init_weights()
+
+ def tie_aux_decoder_weights(self, module, aux_modules):
+ """Tie decoder weights of all `aux_modules` to `module`, (not bias)"""
+ for m in aux_modules:
+ m.predictions.decoder.weight = module.predictions.decoder.weight
+
+ def get_output_embeddings(self):
+ return self.cls.predictions.decoder
+
+ def set_output_embeddings(self, new_embeddings):
+ self.cls.predictions.decoder = new_embeddings
+
+ def forward(
+ self,
+ input_ids=None,
+ attention_mask=None,
+ token_type_ids=None,
+ position_ids=None,
+ head_mask=None,
+ inputs_embeds=None,
+ encoder_embeds=None,
+ encoder_hidden_states=None,
+ encoder_attention_mask=None,
+ labels=None,
+ output_attentions=None,
+ output_hidden_states=None,
+ return_dict=None,
+ is_decoder=False,
+ mode='multi_modal',
+ normalize_attention=True,
+ soft_labels=None,
+ alpha=0,
+ return_logits=False,
+ ):
+ r"""
+ labels (:obj:`torch.LongTensor` of shape :obj:`(batch_size, sequence_length)`, `optional`):
+ Labels for computing the masked language modeling loss. Indices should be in ``[-100, 0, ...,
+ config.vocab_size]`` (see ``input_ids`` docstring) Tokens with indices set to ``-100`` are ignored
+ (masked), the loss is only computed for the tokens with labels in ``[0, ..., config.vocab_size]``
+ """
+
+ return_dict = return_dict if return_dict is not None else self.config.use_return_dict
+
+ outputs = self.bert(
+ input_ids,
+ attention_mask=attention_mask,
+ token_type_ids=token_type_ids,
+ position_ids=position_ids,
+ head_mask=head_mask,
+ inputs_embeds=inputs_embeds,
+ encoder_embeds=encoder_embeds,
+ encoder_hidden_states=encoder_hidden_states,
+ encoder_attention_mask=encoder_attention_mask,
+ output_attentions=output_attentions,
+ output_hidden_states=output_hidden_states,
+ return_dict=return_dict,
+ is_decoder=is_decoder,
+ mode=mode,
+ normalize_attention=normalize_attention,
+ )
+
+ sequence_output = outputs[0]
+ prediction_scores = self.cls(sequence_output)
+
+ if return_logits:
+ return prediction_scores
+
+ masked_lm_loss = None
+ masked_lm_loss_aux = 0.0
+ if labels is not None:
+ loss_fct = CrossEntropyLoss() # -100 index = padding token
+ masked_lm_loss = loss_fct(
+ prediction_scores.view(-1, self.config.vocab_size),
+ labels.view(-1))
+
+ if soft_labels is not None:
+ loss_distill = -torch.sum(
+ F.log_softmax(prediction_scores, dim=1) * soft_labels, dim=-1)
+ loss_distill = loss_distill[labels != -100].mean()
+ masked_lm_loss = (1 -
+ alpha) * masked_lm_loss + alpha * loss_distill
+
+ if not return_dict:
+ output = (prediction_scores, ) + outputs[2:]
+ return ((masked_lm_loss, ) +
+ output) if masked_lm_loss is not None else output
+
+ # changed from MaskedLMOutput to MaskedLMOutputWithDistill
+ return MaskedLMOutputWithDistill(
+ loss=masked_lm_loss,
+ loss_aux=masked_lm_loss_aux,
+ logits=prediction_scores,
+ hidden_states=outputs.hidden_states,
+ attentions=outputs.attentions,
+ )
+
+ def prepare_inputs_for_generation(self,
+ input_ids,
+ attention_mask=None,
+ **model_kwargs):
+ input_shape = input_ids.shape
+ effective_batch_size = input_shape[0]
+
+ # add a dummy token
+ assert (self.config.pad_token_id
+ is not None), 'The PAD token should be defined for generation'
+ attention_mask = torch.cat([
+ attention_mask,
+ attention_mask.new_zeros((attention_mask.shape[0], 1))
+ ],
+ dim=-1)
+ dummy_token = torch.full(
+ (effective_batch_size, 1),
+ self.config.pad_token_id,
+ dtype=torch.long,
+ device=input_ids.device,
+ )
+ input_ids = torch.cat([input_ids, dummy_token], dim=1)
+
+ return {'input_ids': input_ids, 'attention_mask': attention_mask}
diff --git a/mmaction/models/multimodal/vindlu/temporal_model.py b/mmaction/models/multimodal/vindlu/temporal_model.py
new file mode 100644
index 0000000000..7271aedc8a
--- /dev/null
+++ b/mmaction/models/multimodal/vindlu/temporal_model.py
@@ -0,0 +1,213 @@
+# Copyright (c) OpenMMLab. All rights reserved.
+import math
+
+import einops
+import torch
+from einops import rearrange
+from timm.models.layers import DropPath
+from torch import nn
+from torch.nn import LayerNorm, Linear, MultiheadAttention
+
+
+class STAdapter(nn.Module):
+ """ST Adapter."""
+
+ def __init__(
+ self,
+ kernel_size=(3, 3, 3),
+ input_dim=768,
+ hidden_dim=384,
+ img_size=224,
+ patch_size=16,
+ drop_prob=0.1,
+ ):
+ super(STAdapter, self).__init__()
+ self.kernel_size = kernel_size
+ self.input_dim = input_dim
+ self.hidden_dim = hidden_dim
+
+ self.h = self.w = img_size // patch_size
+
+ self.linear1 = nn.Linear(input_dim, hidden_dim)
+ self.linear2 = nn.Linear(hidden_dim, input_dim)
+ self.act = nn.ReLU()
+ self.conv = nn.Conv3d(
+ hidden_dim,
+ hidden_dim,
+ kernel_size=kernel_size,
+ padding='same',
+ groups=hidden_dim)
+ self.droppath = DropPath(drop_prob=drop_prob)
+
+ self.scale = nn.parameter.Parameter(torch.zeros([]))
+
+ def forward(self, x: torch.Tensor):
+ """forward.
+
+ Args:
+ x (torch.Tensor): input features.
+ Shape: [bs, nframes, l, c]. l = 1 + h*w
+
+ Returns: features after adapter. The same shape as input.
+ """
+ if x.shape[1] == 1: # for single frame, return itself.
+ return x
+
+ shortcut = x
+ x = self.linear1(x)
+ cls = x[:, :, :1, :]
+ tokens = x[:, :, 1:, :]
+ tokens = einops.rearrange(
+ tokens, 'b t (h w) c -> b c t h w', h=self.h).contiguous()
+ tokens = self.conv(tokens)
+ tokens = einops.rearrange(tokens, 'b c t h w -> b t (h w) c')
+ x = torch.cat([cls, tokens], dim=2) # [b, t, 1+h*w, c]
+ x = self.act(x)
+ x = self.linear2(x)
+
+ return shortcut + self.scale * self.droppath(x)
+
+
+class TemporalAttention(nn.Module):
+ """perform temporal self-attention."""
+
+ def __init__(self, input_dim=768, droppath_rate=0.1):
+ """
+
+ Kwargs:
+ input_dim (int): The input feature dimension.
+
+
+ """
+ super().__init__()
+
+ self._input_dim = input_dim
+ self.temporal_attn = MultiheadAttention(
+ input_dim, num_heads=input_dim // 64)
+ self.norm = LayerNorm(input_dim, eps=1e-12)
+ self.linear = Linear(input_dim, input_dim)
+ self.droppath = DropPath(droppath_rate)
+ self.scale = nn.parameter.Parameter(torch.zeros([]))
+
+ def forward(self, x: torch.Tensor):
+ """forward.
+
+ Args:
+ x (torch.Tensor): input features.
+ Shape: [bs, nframes, l, c]. l = 1 + h*w
+
+ Returns: features after adapter. The same shape as input.
+ """
+ if x.shape[1] == 1: # for single frame, return itself.
+ return x
+
+ shortcut = x
+ x = einops.rearrange(x, 'b t l c -> t (b l) c')
+ x = self.norm(x)
+ x = self.temporal_attn(x, x, x)[0]
+ x = einops.rearrange(x, 't (b l) c -> b t l c', b=shortcut.shape[0])
+ return shortcut + self.scale * self.droppath(x)
+
+
+class WindowTemporalAttention(nn.Module):
+ """perform windowed temporal self-attention."""
+
+ def __init__(self, input_dim=768, droppath_rate=0.1, window_size=(2, 2)):
+ """
+
+ Kwargs:
+ input_dim (int): The input feature dimension.
+
+
+ """
+ super().__init__()
+
+ self._input_dim = input_dim
+ self.temporal_attn = MultiheadAttention(
+ input_dim, num_heads=input_dim // 64)
+ self.norm = LayerNorm(input_dim, eps=1e-12)
+ self.droppath = DropPath(droppath_rate)
+ self.scale = nn.parameter.Parameter(torch.zeros([]))
+ self.wh, self.ww = window_size
+
+ def forward(self, x: torch.Tensor):
+ """forward.
+
+ Args:
+ x (torch.Tensor): input features.
+ Shape: [bs, nframes, l, c]. l = 1 + h*w
+
+ Returns: features after adapter. The same shape as input.
+ """
+ if x.shape[1] == 1: # for single frame, return itself.
+ return x
+ shortcut = x
+
+ h = w = int(math.sqrt(x.shape[2] - 1))
+ cls_token = x[:, :, :1, :]
+ x = einops.rearrange(
+ x[:, :, 1:, :],
+ 'b t (nh wh nw ww) c -> (t wh ww) (b nh nw) c',
+ nh=h // self.wh,
+ wh=self.wh,
+ nw=w // self.ww,
+ ww=self.ww,
+ )
+ x = self.norm(x)
+ x = self.temporal_attn(x, x, x)[0]
+ x = einops.rearrange(
+ x,
+ '(t wh ww) (b nh nw) c -> b t (nh wh nw ww) c',
+ wh=self.wh,
+ ww=self.ww,
+ nh=h // self.wh,
+ nw=w // self.ww,
+ )
+ # add back cls token.
+ x = torch.concat([cls_token, x], dim=2)
+ return shortcut + self.scale * self.droppath(x)
+
+
+class X_CLIP(nn.Module):
+ """perform windowed temporal self-attention."""
+
+ def __init__(self, input_dim=768, droppath_rate=0.1, num_prompts=1):
+ """
+
+ Kwargs:
+ input_dim (int): The input feature dimension.
+
+
+ """
+ super().__init__()
+
+ d_model = input_dim
+
+ self.message_fc = nn.Linear(d_model, d_model)
+ self.message_ln = LayerNorm(d_model, eps=1e-12)
+ self.message_attn = nn.MultiheadAttention(d_model, d_model // 64)
+ self.num_prompts = num_prompts
+
+ self.droppath = DropPath(droppath_rate)
+
+ def forward(self, x: torch.Tensor):
+ """forward.
+
+ Args:
+ x (torch.Tensor): input features.
+ Shape: [bs, nframes, l, c]. l = 1 + h*w
+
+ Returns: features after adapter. The same shape as input.
+ """
+ if x.shape[1] == 1: # for single frame, return itself.
+ return x
+ msg_token = self.message_ln(self.message_fc(x[:, :,
+ 0, :])) # [b, t, c]
+ msg_token = rearrange(msg_token, 'b t c -> t b c')
+ msg_token = msg_token + self.droppath(
+ self.message_attn(msg_token, msg_token, msg_token)[0])
+ msg_token = rearrange(msg_token, 't b c -> b t c')
+ # replace the last prompt token with msg_token.
+ x = torch.cat([x[:, :, :-1, :],
+ msg_token.unsqueeze(2)], dim=2) # [b, t, l+1, c]
+ return x
diff --git a/mmaction/models/multimodal/vindlu/tokenizer.py b/mmaction/models/multimodal/vindlu/tokenizer.py
new file mode 100644
index 0000000000..92be293dff
--- /dev/null
+++ b/mmaction/models/multimodal/vindlu/tokenizer.py
@@ -0,0 +1,45 @@
+# Copyright (c) OpenMMLab. All rights reserved.
+from typing import List, Optional
+
+from transformers import BertTokenizer
+
+from mmaction.registry import TOKENIZER
+
+
+class VindLUTokenizer(BertTokenizer):
+ """VindLUTokenizer inherit BertTokenizer.
+
+ The main difference from BertTokenizer is removing the last separate token
+ for a single sequence.
+ """
+
+ def build_inputs_with_special_tokens(
+ self,
+ token_ids_0: List[int],
+ token_ids_1: Optional[List[int]] = None) -> List[int]:
+ """Build model inputs from a sequence or a pair of sequence for
+ sequence classification tasks by concatenating and adding special
+ tokens. A BERT sequence has the following format:
+
+ - single sequence: `[CLS] X`
+ - pair of sequences: `[CLS] A [SEP] B [SEP]`
+
+ Args:
+ token_ids_0 (`List[int]`):
+ List of IDs to which the special tokens will be added.
+ token_ids_1 (`List[int]`, *optional*):
+ Optional second list of IDs for sequence pairs.
+
+ Returns:
+ `List[int]`: List of [input IDs](../glossary#input-ids) with
+ the appropriate special tokens.
+ """
+ if token_ids_1 is None:
+ return [self.cls_token_id] + token_ids_0
+ cls = [self.cls_token_id]
+ sep = [self.sep_token_id]
+ return cls + token_ids_0 + sep + token_ids_1 + sep
+
+
+TOKENIZER.register_module(
+ 'VindLUTokenizer', module=VindLUTokenizer.from_pretrained)
diff --git a/mmaction/models/multimodal/vindlu/utils.py b/mmaction/models/multimodal/vindlu/utils.py
new file mode 100644
index 0000000000..8737dde9ea
--- /dev/null
+++ b/mmaction/models/multimodal/vindlu/utils.py
@@ -0,0 +1,195 @@
+# Copyright (c) OpenMMLab. All rights reserved.
+import mmengine.dist as dist
+import numpy as np
+import torch
+import torch.nn.functional as F
+from mmengine.logging import MMLogger
+from scipy import interpolate
+
+
+def all_gather_concat(data: torch.Tensor) -> torch.Tensor:
+ """Gather tensors with different first-dimension size and concat to one
+ tenosr.
+
+ Note:
+ Only the first dimension should be different.
+
+ Args:
+ data (Tensor): Tensor to be gathered.
+
+ Returns:
+ torch.Tensor: The concatenated tenosr.
+ """
+ if dist.get_world_size() == 1:
+ return data
+
+ data_size = torch.tensor(data.size(0), device=data.device)
+ sizes_list = dist.all_gather(data_size)
+
+ total_length = sum(sizes_list)
+ max_length = max(sizes_list)
+ size_diff = max_length.item() - data_size.item()
+ if size_diff:
+ padding = torch.zeros(
+ size_diff, *data.size()[1:], device=data.device, dtype=data.dtype)
+ data = torch.cat((data, padding))
+
+ gather_list = dist.all_gather(data)
+
+ # gather all data according to the default DDP sampler. For instance,
+ # 8 samples on 2 GPUs, GPU0: [0,2,4,6], GPU1: [1,3,5,7], will be gathered
+ # as [0,1,2,3,4,5,6,7]
+ all_data = []
+ for gather_batch in zip(*gather_list):
+ all_data.extend(gather_batch)
+
+ return torch.stack(all_data)[:total_length]
+
+
+def interpolate_pos_embed_beit(state_dict, new_model):
+ """interpolate the positional embeddings. The spatial pe is relative and
+ temporal pe is absolute. additional temporal pe is padded with 0.
+
+ Args:
+ state_dict (dict): The state_dict.
+ new_model (nn.Module): The created model.
+
+ Returns: dict. The state_dict with updated positional embeddings.
+ """
+ state_dict = interpolate_pos_relative_bias_beit(
+ state_dict_old=state_dict,
+ state_dict_new=new_model.state_dict(),
+ patch_shape_new=new_model.vision_encoder.embeddings.patch_embeddings.
+ patch_shape,
+ )
+ # absolute temporal pos bias
+ temporal_pe_key = 'vision_encoder.embeddings.temporal_position_embeddings'
+ if temporal_pe_key in state_dict:
+ logger = MMLogger.get_current_instance()
+ logger.info(
+ f'interpolate temporal positional embeddings: {temporal_pe_key}')
+ state_dict[temporal_pe_key] = load_temp_embed_with_mismatch(
+ temp_embed_old=state_dict[temporal_pe_key],
+ temp_embed_new=new_model.state_dict()[temporal_pe_key],
+ )
+ return state_dict
+
+
+def load_temp_embed_with_mismatch(temp_embed_old,
+ temp_embed_new,
+ add_zero=True):
+ """Add/Remove extra temporal_embeddings as needed.
+ https://arxiv.org/abs/2104.00650 shows adding zero paddings works.
+
+ temp_embed_old: (1, num_frames_old, 1, d)
+ temp_embed_new: (1, num_frames_new, 1, d)
+ add_zero: bool, if True, add zero, else, interpolate trained embeddings.
+ """
+ # TODO zero pad
+ num_frms_new = temp_embed_new.shape[1]
+ num_frms_old = temp_embed_old.shape[1]
+ logger = MMLogger.get_current_instance()
+ logger.info(
+ f'Load temporal_embeddings, lengths: {num_frms_old}-->{num_frms_new}')
+ if num_frms_new > num_frms_old:
+ if add_zero:
+ temp_embed_new[:, :num_frms_old] \
+ = temp_embed_old # untrained embeddings are zeros.
+ else:
+ temp_embed_new = interpolate_temporal_pos_embed(
+ temp_embed_old, num_frms_new)
+ elif num_frms_new < num_frms_old:
+ temp_embed_new = temp_embed_old[:, :num_frms_new]
+ else: # =
+ temp_embed_new = temp_embed_old
+ return temp_embed_new
+
+
+def interpolate_temporal_pos_embed(temp_embed_old, num_frames_new):
+ """
+ temp_embed_old: (1, num_frames_old, 1, d)
+ Returns:
+ temp_embed_new: (1, num_frames_new, 1, d)
+ """
+ temp_embed_old = temp_embed_old.squeeze(2).permute(
+ 0, 2, 1) # (1, d, num_frames_old)
+ temp_embed_new = F.interpolate(
+ temp_embed_old, num_frames_new,
+ mode='linear') # (1, d, num_frames_new)
+ temp_embed_new = temp_embed_new.permute(0, 2, 1).unsqueeze(
+ 2) # (1, num_frames_new, 1, d)
+ return temp_embed_new
+
+
+def interpolate_pos_relative_bias_beit(state_dict_old, state_dict_new,
+ patch_shape_new):
+ """
+ Args:
+ state_dict_old: loaded state dict
+ state_dict_new: state dict for model with new image size
+ patch_shape_new: new model patch_shape
+ ref: https://github.com/microsoft/unilm/blob/master/beit/run_class_finetuning.py # noqa: E501
+ """
+ all_keys = list(state_dict_old.keys())
+ for key in all_keys:
+ if 'relative_position_index' in key:
+ state_dict_old.pop(key)
+
+ if 'relative_position_bias_table' in key:
+ rel_pos_bias = state_dict_old[key]
+ src_num_pos, num_attn_heads = rel_pos_bias.size()
+ dst_num_pos, _ = state_dict_new[key].size()
+ dst_patch_shape = patch_shape_new
+ if dst_patch_shape[0] != dst_patch_shape[1]:
+ raise NotImplementedError()
+ num_extra_tokens = dst_num_pos - (dst_patch_shape[0] * 2 - 1) * (
+ dst_patch_shape[1] * 2 - 1)
+ src_size = int((src_num_pos - num_extra_tokens)**0.5)
+ dst_size = int((dst_num_pos - num_extra_tokens)**0.5)
+ if src_size != dst_size:
+ extra_tokens = rel_pos_bias[-num_extra_tokens:, :]
+ rel_pos_bias = rel_pos_bias[:-num_extra_tokens, :]
+
+ def geometric_progression(a, r, n):
+ return a * (1.0 - r**n) / (1.0 - r)
+
+ left, right = 1.01, 1.5
+ while right - left > 1e-6:
+ q = (left + right) / 2.0
+ gp = geometric_progression(1, q, src_size // 2)
+ if gp > dst_size // 2:
+ right = q
+ else:
+ left = q
+
+ dis = []
+ cur = 1
+ for i in range(src_size // 2):
+ dis.append(cur)
+ cur += q**(i + 1)
+
+ r_ids = [-_ for _ in reversed(dis)]
+
+ x = r_ids + [0] + dis
+ y = r_ids + [0] + dis
+
+ t = dst_size // 2.0
+ dx = np.arange(-t, t + 0.1, 1.0)
+ dy = np.arange(-t, t + 0.1, 1.0)
+
+ all_rel_pos_bias = []
+
+ for i in range(num_attn_heads):
+ z = rel_pos_bias[:, i].view(src_size,
+ src_size).float().numpy()
+ f = interpolate.interp2d(x, y, z, kind='cubic')
+ all_rel_pos_bias.append(
+ torch.Tensor(f(dx, dy)).contiguous().view(-1, 1).to(
+ rel_pos_bias.device))
+
+ rel_pos_bias = torch.cat(all_rel_pos_bias, dim=-1)
+
+ new_rel_pos_bias = torch.cat((rel_pos_bias, extra_tokens),
+ dim=0)
+ state_dict_old[key] = new_rel_pos_bias
+ return state_dict_old
diff --git a/mmaction/models/multimodal/vindlu/vindlu.py b/mmaction/models/multimodal/vindlu/vindlu.py
new file mode 100644
index 0000000000..1f6f9dcff2
--- /dev/null
+++ b/mmaction/models/multimodal/vindlu/vindlu.py
@@ -0,0 +1,227 @@
+# Copyright (c) OpenMMLab. All rights reserved.
+from abc import abstractmethod
+from typing import Optional
+
+import torch
+from mmengine.logging import MMLogger
+from mmengine.model import BaseModel
+from mmengine.runner.checkpoint import _load_checkpoint
+from torch import nn
+
+from mmaction.registry import MODELS, TOKENIZER
+from mmaction.utils import ForwardResults, SampleList
+from .utils import (interpolate_pos_embed_beit,
+ interpolate_pos_relative_bias_beit)
+
+
+class VindLUBase(BaseModel):
+ """VindLU base Model.
+
+ Args:
+ tokenizer: (dict): The config for tokenizer.
+ vision_encoder (dict): Backbone for extracting image features.
+ text_encoder (dict): Backbone for extracting text features.
+ temperature (float): Temperature parameter that controls the
+ concentration level of the distribution. Defaults to 0.07.
+ gradient_checkpointing (bool): Whether to do gradient_checkpointing.
+ Using checkpoint will save some memory while slowing down the
+ training speed. Defaults to False.
+ data_preprocessor (Optional[dict]): The config for preprocessing input
+ data.
+ init_cfg (Optional[dict]): the config to control the initialization.
+ Defaults to None.
+ """
+
+ def __init__(
+ self,
+ tokenizer: dict,
+ vision_encoder: dict,
+ text_encoder: dict,
+ proj_dim: int = 256,
+ temperature: float = 0.07,
+ gradient_checkpointing: bool = False,
+ pretrined_vl: bool = True,
+ data_preprocessor: Optional[dict] = None,
+ init_cfg: Optional[dict] = None,
+ ):
+ if data_preprocessor is None:
+ data_preprocessor = dict(type='ActionDataPreprocessor')
+ super().__init__(
+ init_cfg=init_cfg, data_preprocessor=data_preprocessor)
+
+ self.tokenizer = TOKENIZER.build(tokenizer)
+ self.vision_cfg = vision_encoder
+ self.text_encoder_cfg = text_encoder
+ self.gradient_checkpointing = gradient_checkpointing
+ self.text_encoder_cfg.gradient_checkpointing = gradient_checkpointing
+
+ self.vision_width = vision_encoder.pop('encoder_width')
+ self.text_width = text_encoder.encoder_width
+ self.pretrined_vl = pretrined_vl
+
+ if self.vision_cfg.pop('add_ln'):
+ self.vision_layernorm = nn.LayerNorm(self.vision_width, eps=1e-12)
+ else:
+ self.vision_layernorm = nn.Identity()
+
+ self.vision_encoder = MODELS.build(self.vision_cfg)
+
+ if gradient_checkpointing:
+ self.vision_encoder.gradient_checkpointing_enable()
+
+ self.text_encoder = MODELS.build(self.text_encoder_cfg)
+
+ self.vision_proj = nn.Linear(self.vision_width, proj_dim)
+ self.text_proj = nn.Linear(self.text_width, proj_dim)
+
+ self.temp = nn.parameter.Parameter(torch.ones([]) * temperature)
+ self.itm_head = nn.Linear(self.text_width, 2)
+
+ def extract_feat(self, inputs: torch.Tensor, **kwargs) -> ForwardResults:
+ """Extract features from raw inputs."""
+
+ @abstractmethod
+ def loss(self, inputs: torch.Tensor, data_samples: SampleList,
+ **kwargs) -> dict:
+ """Calculate losses from a batch of inputs and data samples."""
+
+ def forward(self, inputs, data_samples, mode: str = 'loss'):
+ """The unified entry for a forward process in both training and test.
+
+ The method should accept three modes:
+
+ - ``tensor``: Forward the whole network and return tensor or tuple of
+ tensor without any post-processing, same as a common nn.Module.
+ - ``predict``: Forward and return the predictions, which are fully
+ processed to a list of :obj:`ActionDataSample`.
+ - ``loss``: Forward and return a dict of losses according to the given
+ inputs and data samples.
+
+ Note that this method doesn't handle neither back propagation nor
+ optimizer updating, which are done in the :meth:`train_step`.
+
+ Args:
+ inputs (torch.Tensor): The input tensor with shape
+ (N, C, ...) in general.
+ data_samples (List[``ActionDataSample], optional): The
+ annotation data of every samples. Defaults to None.
+ mode (str): Return what kind of value. Defaults to ``tensor``.
+
+ Returns:
+ The return type depends on ``mode``.
+
+ - If ``mode="tensor"``, return a tensor or a tuple of tensor.
+ - If ``mode="predict"``, return a list of ``ActionDataSample``.
+ - If ``mode="loss"``, return a dict of tensor.
+ """
+
+ if mode == 'tensor':
+ return self.extract_feat(inputs, data_samples)
+ elif mode == 'loss':
+ return self.loss(inputs, data_samples)
+ elif mode == 'predict':
+ return self.predict(inputs, data_samples)
+ else:
+ raise RuntimeError(f'Invalid mode "{mode}".')
+
+ def encode_vision(self, image):
+ """encode image / videos as features.
+
+ Args:
+ image (torch.Tensor): The input images.
+
+ Returns: tuple.
+ - vision_embeds (torch.Tensor): The features of all patches.
+ Shape: [B,T,L,C].
+ - pooled_vision_embeds (torch.Tensor): The pooled features.
+ Shape: [B,T,C].
+ """
+ output_dict = self.vision_encoder(image)
+ vision_embeds = self.vision_layernorm(output_dict.last_hidden_state)
+ pooled_vision_embeds = output_dict.pooler_output
+
+ return vision_embeds, pooled_vision_embeds
+
+ def encode_text(self, text):
+ """encode text.
+ Args:
+ text (dict): The output of huggingface's `PreTrainedTokenizer`.
+ contains keys:
+ - input_ids (torch.Tensor): Token ids to be fed to a model.
+ Shape: [B,L].
+ - attention_mask (torch.Tensor): The mask indicate padded tokens.
+ Shape: [B,L]. 0 is padded token.
+ - other keys refer to "https://huggingface.co/docs/transformers/v4.21.2/en/main_classes/tokenizer#transformers.PreTrainedTokenizer.__call__". # noqa: E501
+ Returns: tuple.
+ - text_embeds (torch.Tensor): The features of all tokens. Shape: [B,L,C].
+ - pooled_text_embeds (torch.Tensor): The pooled features. Shape: [B,C].
+
+ """
+ text_output = self.text_encoder(
+ text.input_ids,
+ attention_mask=text.attention_mask,
+ return_dict=True,
+ mode='text',
+ )
+ text_embeds = text_output.last_hidden_state
+ pooled_text_embeds = text_embeds[:, 0]
+ return text_embeds, pooled_text_embeds
+
+ @torch.no_grad()
+ def clip_contrastive_temperature(self, min_val=0.001, max_val=0.5):
+ """Seems only used during pre-training."""
+ self.temp.clamp_(min_val, max_val)
+
+ @property
+ def device(self):
+ return next(self.parameters()).device
+
+ def preprocess_state_dict(self, state_dict):
+ """Preprocess pretrained checkpoint for text_encoder."""
+ for key in list(state_dict.keys()):
+ if 'bert' in key:
+ encoder_key = key.replace('bert.', '')
+ state_dict[encoder_key] = state_dict[key]
+ del state_dict[key]
+ return state_dict
+
+ def load_from_pretrainded_beit(self):
+ from transformers.models.beit.modeling_beit import BeitModel
+ beit2d = BeitModel.from_pretrained(
+ self.vision_cfg.pretrained_model_name_or_path)
+ ori_state_dict = beit2d.state_dict()
+ del beit2d
+ # interpolate relative pos bias
+ state_dict = interpolate_pos_relative_bias_beit(
+ state_dict_old=ori_state_dict,
+ state_dict_new=self.vision_encoder.state_dict(),
+ patch_shape_new=self.vision_encoder.embeddings.patch_embeddings.
+ patch_shape,
+ )
+
+ for k in list(state_dict.keys()):
+ if 'prompt_bias_table' in k:
+ del state_dict[k]
+
+ msg = self.vision_encoder.load_state_dict(state_dict, strict=False)
+ logger = MMLogger.get_current_instance()
+ logger.info(msg)
+
+ def init_weights(self):
+ if self.vision_cfg.get('pretrained2d', False):
+ self.load_from_pretrainded_beit()
+
+ if self.pretrined_vl:
+ assert self.init_cfg.get('type') == 'Pretrained', (
+ 'Please specify '
+ 'init_cfg to use pretrained video-language checkpoint')
+ self.pretrained = self.init_cfg.get('checkpoint')
+ checkpoint = _load_checkpoint(self.pretrained, map_location='cpu')
+ state_dict = checkpoint['model']
+ state_dict = interpolate_pos_embed_beit(state_dict, self)
+ state_dict = self.preprocess_state_dict(state_dict)
+ msg = self.load_state_dict(state_dict, strict=False)
+ logger = MMLogger.get_current_instance()
+ logger.info(msg)
+ else:
+ super().init_weights()
diff --git a/mmaction/models/multimodal/vindlu/vindlu_ret.py b/mmaction/models/multimodal/vindlu/vindlu_ret.py
new file mode 100644
index 0000000000..cc80982c39
--- /dev/null
+++ b/mmaction/models/multimodal/vindlu/vindlu_ret.py
@@ -0,0 +1,464 @@
+# Copyright (c) OpenMMLab. All rights reserved.
+from typing import Dict, List, Optional
+
+import mmengine.dist as dist
+import torch
+import torch.nn.functional as F
+from einops import rearrange
+from torch.distributed.nn import all_gather as all_gather_with_grad
+
+from mmaction.registry import MODELS
+from mmaction.structures import ActionDataSample
+from mmaction.utils import track_on_main_process
+from .utils import all_gather_concat
+from .vindlu import VindLUBase
+
+
+@MODELS.register_module()
+class VindLURetrieval(VindLUBase):
+ """VindLU retriever.
+
+ max_txt_len (int): Max text length of input text, used for retrieval
+ from multiple choices. Defaults to 32.
+ topk (int): Select topk similarity as candidates for compute matching
+ scores. Defaults to 256.
+ negative_all_rank (bool): Whether to sample negative data from all
+ ranks for image text matching in training. Defaults to False.
+ fast_match (bool): If False, select topk similarity as candidates and
+ compute the matching score. If True, return the similarity as the
+ matching score directly. Defaults to False.
+ **kwargs: Other keyword arguments to initialize the VindLU base model.
+ """
+
+ def __init__(self,
+ max_txt_len: int = 32,
+ topk: int = 128,
+ negative_all_rank: bool = False,
+ fast_match: bool = False,
+ **kwargs):
+ super().__init__(**kwargs)
+
+ self.max_txt_len = max_txt_len
+ self.topk = topk
+ self.negative_all_rank = negative_all_rank
+ self.fast_match = fast_match
+
+ def loss(
+ self,
+ inputs: torch.Tensor,
+ data_samples: Optional[List[ActionDataSample]] = None,
+ ) -> Dict[str, torch.tensor]:
+ """Calculate losses from a batch of inputs and data samples.
+
+ Args:
+ inputs (dict): A batch of inputs. The input tensor with of
+ at least one modality. For image, the value is a tensor
+ of shape (N, C, ...) in general.
+ For text, the value is a dict of tokenized text inputs.
+ data_samples (Optional[List[DataSample]]):
+ The annotation data of every samples. Defaults to None.
+
+ Returns:
+ Dict[str, torch.tensor]: a dictionary of loss components of
+ """
+ output = self.extract_feat(inputs, data_samples)
+
+ text_embeds = output['text_embeds']
+ text_attn_mask = output['text_attn_mask']
+ image_embeds = output['image_embeds']
+ image_feat = output['image_feat']
+ text_feat = output['text_feat']
+
+ image_atts = torch.ones(
+ image_embeds.size()[:-1], dtype=torch.long).to(self.device)
+
+ # ITC Loss
+ # B*world_size, D
+ image_feat_all = torch.cat(dist.all_gather(image_feat))
+ # B*world_size, D
+ text_feat_all = torch.cat(dist.all_gather(text_feat))
+
+ # image to text similarity
+ # B, B*world_size
+ sim_i2t = torch.einsum('mld,nd->mln', image_feat,
+ text_feat_all).mean(1) / self.temp
+ # text-image similarity
+ # B, B*world_size
+ sim_t2i = torch.einsum('md,nld->mln', text_feat,
+ image_feat_all).mean(1) / self.temp
+
+ rank = dist.get_rank()
+ bs = inputs.size(0)
+ itc_targets = torch.linspace(
+ rank * bs, rank * bs + bs - 1, bs, dtype=int).to(self.device)
+
+ itc_loss = (F.cross_entropy(sim_i2t, itc_targets) +
+ F.cross_entropy(sim_t2i, itc_targets)) / 2
+
+ # prepare for itm
+ output_pos = self.text_encoder(
+ encoder_embeds=text_embeds,
+ attention_mask=text_attn_mask,
+ encoder_hidden_states=image_embeds,
+ encoder_attention_mask=image_atts,
+ return_dict=True,
+ mode='fusion',
+ )
+
+ idx = torch.tensor([i.gt_video_id for i in data_samples]).view(-1, 1)
+ bs = idx.size(0)
+ if self.negative_all_rank:
+ idxs = torch.cat(dist.all_gather(idx))
+ image_feat_world = torch.cat(dist.all_gather(image_feat))
+ text_feat_world = torch.cat(dist.all_gather(text_feat))
+ att_mask_world = torch.cat(dist.all_gather(text_attn_mask))
+ text_embeds_world = torch.cat(all_gather_with_grad(text_embeds))
+ image_embeds_world = torch.cat(all_gather_with_grad(image_embeds))
+ else:
+ idxs = idx
+ image_feat_world = image_feat.detach()
+ text_feat_world = text_feat.detach()
+ image_embeds_world = image_embeds
+ text_embeds_world = text_embeds
+ att_mask_world = text_attn_mask
+
+ with torch.no_grad():
+ # compute sample similarity
+ sim_i2t = torch.einsum('mld,nd->mln', image_feat,
+ text_feat_world).mean(1) / self.temp
+ sim_t2i = torch.einsum('md,nld->mln', text_feat,
+ image_feat_world).mean(1) / self.temp
+
+ mask = torch.eq(idx, idxs.t()).to(self.device)
+ weights_i2t = F.softmax(sim_i2t + 1e-4, dim=1)
+ weights_i2t.masked_fill_(mask, 0)
+
+ weights_t2i = F.softmax(sim_t2i + 1e-4, dim=1)
+ weights_t2i.masked_fill_(mask, 0)
+
+ # select a negative image for each text
+ neg_idx = torch.multinomial(weights_t2i, 1).squeeze()
+ image_embeds_neg = image_embeds_world[neg_idx]
+
+ # select a negative text for each image
+ neg_idx = torch.multinomial(weights_i2t, 1).squeeze()
+ text_embeds_neg = text_embeds_world[neg_idx]
+ text_atts_neg = att_mask_world[neg_idx]
+
+ text_embeds_all = torch.cat([text_embeds, text_embeds_neg], dim=0)
+ text_atts_all = torch.cat([text_attn_mask, text_atts_neg], dim=0)
+
+ image_embeds_all = torch.cat([image_embeds_neg, image_embeds], dim=0)
+ image_atts_all = torch.cat([image_atts, image_atts], dim=0)
+
+ output_neg = self.text_encoder(
+ encoder_embeds=text_embeds_all,
+ attention_mask=text_atts_all,
+ encoder_hidden_states=image_embeds_all,
+ encoder_attention_mask=image_atts_all,
+ return_dict=True,
+ mode='fusion',
+ )
+
+ vl_embeddings = torch.cat(
+ [
+ output_pos.last_hidden_state[:, 0, :],
+ output_neg.last_hidden_state[:, 0, :],
+ ],
+ dim=0,
+ )
+
+ itm_targets = torch.ones((3 * bs, ),
+ dtype=torch.long,
+ device=inputs.device)
+ itm_targets[bs:] = 0
+ itm_logit = self.itm_head(vl_embeddings)
+ itm_loss = F.cross_entropy(itm_logit, itm_targets)
+
+ return dict(itc_loss=itc_loss, itm_loss=itm_loss)
+
+ def preprocess_text(self, data_samples):
+ sample_item = data_samples[0]
+
+ if sample_item is not None and 'text' in sample_item:
+ if isinstance(sample_item.get('text'), (list, tuple)):
+ texts = []
+ for sample in data_samples:
+ texts.extend(sample.get('text'))
+ elif isinstance(sample_item.get('text'), str):
+ texts = [sample.get('text') for sample in data_samples]
+ else:
+ raise TypeError('text must be a string or a list of strings')
+ else:
+ return None
+
+ # perform tokenize first if satisfied conditions
+ texts = self.tokenizer(
+ texts,
+ padding='max_length',
+ truncation=True,
+ max_length=self.max_txt_len,
+ return_tensors='pt',
+ ).to(self.device)
+
+ return texts
+
+ def extract_feat(
+ self,
+ images: torch.Tensor = None,
+ data_samples: List[ActionDataSample] = None,
+ return_texts=True,
+ ) -> Dict[str, torch.Tensor]:
+ """Extract features from the input dict.
+
+ Args:
+ images (tensor, optional): The images to extract features.
+ Defaults to None.
+ data_samples (list, optional): The data samples containing texts
+ to extract features. Defaults to None.
+ return_texts (bool): Whether to return the tokenized text and the
+ corresponding attention masks. Defaults to True.
+
+ Returns:
+ Tuple[torch.Tensor]: The output features.
+ If multimodal_backbone is not exist, tuple of torch.Tensor
+ will be returned.
+ """
+ if data_samples is not None:
+ texts = self.preprocess_text(data_samples)
+ else:
+ texts = None
+
+ assert images is not None or texts is not None, \
+ 'At least single modality should be passed as inputs.'
+
+ results = {}
+ if texts is not None and return_texts:
+ results.update({
+ 'text_ids': texts.input_ids,
+ 'text_attn_mask': texts.attention_mask,
+ })
+
+ # extract image features
+ if images is not None:
+ image_embeds, pooled_image_embeds = self.encode_vision(images)
+ # concat temporal embeds
+ image_embeds = rearrange(image_embeds,
+ 'b t l c -> b (t l) c').contiguous()
+ results['image_embeds'] = image_embeds
+ results['image_feat'] = F.normalize(
+ self.vision_proj(pooled_image_embeds), dim=-1)
+
+ # extract text features
+ if texts is not None:
+ texts_output = self.text_encoder(
+ texts.input_ids,
+ attention_mask=texts.attention_mask,
+ return_dict=True,
+ mode='text')
+
+ text_embeds = texts_output.last_hidden_state
+ pooled_text_feat = text_embeds[:, 0]
+ results['text_embeds'] = text_embeds
+ results['text_feat'] = F.normalize(
+ self.text_proj(pooled_text_feat), dim=-1)
+
+ return results
+
+ def predict(self, images, data_samples, cal_i2t=True, cal_t2i=True):
+ feats = self.extract_feat(images, data_samples)
+
+ return self.predict_all(
+ feats, data_samples, cal_i2t=cal_i2t, cal_t2i=cal_t2i)
+
+ def predict_all(self,
+ feats,
+ data_samples,
+ num_images=None,
+ num_texts=None,
+ cal_i2t=True,
+ cal_t2i=True):
+ text_attn_mask = feats['text_attn_mask']
+ image_embeds = feats.get('image_embeds', None)
+ image_feat = feats['image_feat']
+ text_embeds = feats['text_embeds']
+ text_feat = feats['text_feat']
+
+ num_images = num_images or image_feat.size(0)
+ num_texts = num_texts or text_feat.size(0)
+
+ image_embeds_all = all_gather_concat(image_embeds)[:num_images]
+ image_feat_all = all_gather_concat(image_feat)[:num_images]
+ text_feat_all = all_gather_concat(text_feat)[:num_texts]
+ text_embeds_all = all_gather_concat(text_embeds)[:num_texts]
+ text_attn_mask_all = all_gather_concat(text_attn_mask)[:num_texts]
+
+ results = []
+ if cal_i2t:
+ result_i2t = self.compute_score_matrix_i2t(
+ image_feat,
+ image_embeds,
+ text_feat_all,
+ text_embeds_all,
+ text_attn_mask_all,
+ )
+ results.append(
+ self._get_predictions(result_i2t, data_samples, mode='i2t'))
+ if cal_t2i:
+ result_t2i = self.compute_score_matrix_t2i(
+ image_feat_all,
+ image_embeds_all,
+ text_feat,
+ text_embeds,
+ text_attn_mask,
+ )
+ results.append(
+ self._get_predictions(result_t2i, data_samples, mode='t2i'))
+ return tuple(results)
+
+ def compute_score_matrix_i2t(self, img_feats, img_embeds, text_feats,
+ text_embeds, text_atts):
+ """Compare the score matrix for image-to-text retrieval. Every image
+ should compare to all the text features.
+
+ Args:
+ img_feats (torch.Tensor): The input img feats tensor with shape
+ (M, C). M stands for numbers of samples on a single GPU.
+ img_embeds (torch.Tensor): The input img embeds tensor with shape
+ (M, C). M stands for numbers of samples on a single GPU.
+ text_feats (torch.Tensor): The input text feats tensor with shape
+ (N, C). N stands for numbers of all samples on all GPUs.
+ text_embeds (torch.Tensor): The input tensor with shape (N, C).
+ text_atts (torch.Tensor): The input tensor with shape (N, C).
+
+ Returns:
+ torch.Tensor: Score matrix of image-to-text retrieval.
+ """
+ # compute i2t sim matrix
+ sim_matrix_i2t = torch.einsum('mld,nd->mln', img_feats,
+ text_feats).mean(1)
+ if self.fast_match:
+ return sim_matrix_i2t
+
+ score_matrix_i2t = torch.full((img_feats.size(0), text_feats.size(0)),
+ -100.0).to(self.device)
+ for i in track_on_main_process(
+ range(img_feats.size(0)), 'Compute I2T scores...'):
+ sims = sim_matrix_i2t[i]
+ topk_sim, topk_idx = sims.topk(k=self.topk, dim=0)
+ topk_bz = 32
+ encoder_output = img_embeds[i].repeat(topk_bz, 1, 1)
+ encoder_att = torch.ones(
+ encoder_output.size()[:-1], dtype=torch.long).to(self.device)
+ for j in range(0, self.topk // topk_bz):
+ batch_topk = topk_idx[j * topk_bz:(j + 1) * topk_bz]
+ output = self.text_encoder(
+ encoder_embeds=text_embeds[batch_topk],
+ attention_mask=text_atts[batch_topk],
+ encoder_hidden_states=encoder_output,
+ encoder_attention_mask=encoder_att,
+ return_dict=True,
+ mode='fusion')
+ score = self.itm_head(output.last_hidden_state[:, 0, :])[:, 1]
+ score_matrix_i2t[i, batch_topk] = score
+ return score_matrix_i2t
+
+ def compute_score_matrix_t2i(self, img_feats, img_embeds, text_feats,
+ text_embeds, text_atts):
+ """Compare the score matrix for text-to-image retrieval. Every text
+ should compare to all the image features.
+
+ Args:
+ img_feats (torch.Tensor): The input img feats tensor with shape
+ (M, C). M stands for numbers of samples on a single GPU.
+ img_embeds (torch.Tensor): The input img embeds tensor with shape
+ (M, C). M stands for numbers of samples on a single GPU.
+ text_feats (torch.Tensor): The input text feats tensor with shape
+ (N, C). N stands for numbers of all samples on all GPUs.
+ text_embeds (torch.Tensor): The input tensor with shape (M, C).
+ text_atts (torch.Tensor): The input tensor with shape (M, C).
+
+ Returns:
+ torch.Tensor: Score matrix of text-to-image retrieval.
+ """
+ # compute t2i sim matrix
+ sim_matrix_t2i = torch.einsum('md,nld->mln', text_feats,
+ img_feats).mean(1)
+
+ if self.fast_match:
+ return sim_matrix_t2i
+
+ score_matrix_t2i = torch.full((text_feats.size(0), img_feats.size(0)),
+ -100.0).to(self.device)
+ for i in track_on_main_process(
+ range(text_feats.size(0)), 'Compute T2I scores...'):
+ sims = sim_matrix_t2i[i]
+ topk_sim, topk_idx = sims.topk(k=self.topk, dim=0)
+ topk_bz = 32
+ for j in range(0, self.topk // topk_bz):
+ batch_topk = topk_idx[j * topk_bz:(j + 1) * topk_bz]
+ encoder_output = img_embeds[batch_topk]
+ encoder_att = torch.ones(
+ encoder_output.size()[:-1],
+ dtype=torch.long).to(self.device)
+ output = self.text_encoder(
+ encoder_embeds=text_embeds[i].repeat(topk_bz, 1, 1),
+ attention_mask=text_atts[i].repeat(topk_bz, 1),
+ encoder_hidden_states=encoder_output,
+ encoder_attention_mask=encoder_att,
+ return_dict=True,
+ mode='fusion')
+ score = self.itm_head(output.last_hidden_state[:, 0, :])[:, 1]
+ score_matrix_t2i[i, batch_topk] = score
+ return score_matrix_t2i
+
+ def _get_predictions(self,
+ result: torch.Tensor,
+ data_samples: List[ActionDataSample],
+ mode: str = 'i2t'):
+ """Post-process the output of retriever.
+
+ Args:
+ result (torch.Tensor): Score matrix of single retrieve,
+ either from image or text.
+ data_samples (List[ActionDataSample], optional): The annotation
+ data of every samples.
+ mode (str): Retrieve mode, either `i2t` for image to text, or `t2i`
+ text to image. Defaults to `i2t`.
+
+ Returns:
+ List[ActionDataSample]: the raw data_samples with
+ the predicted results.
+ """
+
+ # create data sample if not exists
+ if data_samples is None:
+ data_samples = [ActionDataSample() for _ in range(result.size(0))]
+ elif mode == 't2i':
+ # Process data samples to align with the num of texts.
+ new_data_samples = []
+ for sample in data_samples:
+ if isinstance(sample.text, (list, tuple)):
+ texts = sample.text
+ else:
+ texts = [sample.text]
+ for i, text in enumerate(texts):
+ new_sample = ActionDataSample(text=text)
+ if 'gt_video_id' in sample:
+ new_sample.gt_label = sample.gt_video_id[i]
+ new_data_samples.append(new_sample)
+ assert len(new_data_samples) == result.size(0)
+ data_samples = new_data_samples
+ elif mode == 'i2t':
+ for sample in data_samples:
+ if 'gt_text_id' in sample:
+ sample.gt_label = sample.gt_text_id
+ else:
+ raise ValueError(f'Type {mode} is not supported.')
+
+ for data_sample, score in zip(data_samples, result):
+ idx = score.argmax(keepdim=True).detach()
+
+ data_sample.set_pred_score(score)
+ data_sample.set_pred_label(idx)
+ return data_samples
diff --git a/mmaction/models/multimodal/vindlu/vindlu_ret_mc.py b/mmaction/models/multimodal/vindlu/vindlu_ret_mc.py
new file mode 100644
index 0000000000..d701438bb7
--- /dev/null
+++ b/mmaction/models/multimodal/vindlu/vindlu_ret_mc.py
@@ -0,0 +1,87 @@
+# Copyright (c) OpenMMLab. All rights reserved.
+import torch
+import torch.nn.functional as F
+from einops import rearrange
+
+from mmaction.registry import MODELS
+from .vindlu_ret import VindLURetrieval
+
+
+@MODELS.register_module()
+class VindLURetrievalMC(VindLURetrieval):
+ """VindLU VQA retrieval multiple choice.
+
+ score_weight (float): Weight coefficient for itm_head score to compute the
+ choice score. similarity_weight (float): Weight coefficient for similarity
+ score to compute the choice score.
+ """
+
+ def __init__(self, score_weight=0.7, similarity_weight=0.3, **kwargs):
+ kwargs.pop('text_decoder')
+ super().__init__(**kwargs)
+ self.score_weight = score_weight
+ self.similarity_weight = similarity_weight
+
+ def predict(self, inputs, data_samples, **kwargs):
+ """Predict captions from a batch of inputs.
+
+ Args:
+ images (torch.Tensor): The input images tensor with shape
+ (N, C, ...) in general.
+ data_samples (List[DataSample], optional): The annotation
+ data of every samples. Defaults to None.
+ **kwargs: Other keyword arguments accepted by the ``predict``
+
+ Returns:
+ List[ActionDataSample]: Return list of data samples.
+ """
+ num_options_per_q = len(data_samples[0].caption_options)
+ for sample in data_samples:
+ sample.text = sample.caption_options
+
+ output = self.extract_feat(inputs, data_samples)
+
+ text_embeds = output['text_embeds']
+ text_attn_mask = output['text_attn_mask']
+ image_embeds = output['image_embeds']
+ image_feat = output['image_feat']
+ text_feat = output['text_feat']
+
+ # compute similarity between vision feat and caption feat
+ text_feat = rearrange(
+ text_feat, '(b n) c -> b c n', n=num_options_per_q)
+ sim = torch.matmul(image_feat.mean(1, keepdim=True),
+ text_feat).squeeze(1) / self.temp
+ sim = F.softmax(sim, dim=1).flatten()
+
+ # cross-modal encode
+ encoder_output = image_embeds.repeat_interleave(
+ num_options_per_q, dim=0)
+ image_atts = torch.ones(
+ encoder_output.size()[:-1], dtype=torch.long).to(inputs.device)
+ output = self.text_encoder(
+ encoder_embeds=text_embeds,
+ attention_mask=text_attn_mask,
+ encoder_hidden_states=encoder_output,
+ encoder_attention_mask=image_atts,
+ return_dict=True,
+ mode='fusion',
+ )
+ itm_embeds = output.last_hidden_state[:, 0] # [CLS]
+
+ itm_score = F.softmax(self.itm_head(itm_embeds), dim=1)[:, 1] # [bs*5]
+ score = itm_score * self.score_weight + sim * self.similarity_weight
+
+ pred_answers = score.view(-1, num_options_per_q).max(1)[1].cpu()
+
+ # assemble predictions
+ ensemble_scores = score.view(-1, num_options_per_q).cpu() # (bsz, 5)
+
+ out_data_samples = []
+ for data_sample, ensemble_score, pred_ans in \
+ zip(data_samples, ensemble_scores, pred_answers):
+ data_sample.pred_label = pred_ans.item()
+ data_sample.score = ensemble_score.numpy()
+ out_data_samples.append(data_sample)
+
+ return out_data_samples
diff --git a/mmaction/models/multimodal/vindlu/vindlu_vqa.py b/mmaction/models/multimodal/vindlu/vindlu_vqa.py
new file mode 100644
index 0000000000..87233b9b21
--- /dev/null
+++ b/mmaction/models/multimodal/vindlu/vindlu_vqa.py
@@ -0,0 +1,266 @@
+# Copyright (c) OpenMMLab. All rights reserved.
+from typing import Optional
+
+import mmengine
+import torch
+import torch.nn.functional as F
+from einops import rearrange
+
+from mmaction.registry import MODELS
+from .vindlu import VindLUBase
+
+
+@MODELS.register_module()
+class VindLUVQA(VindLUBase):
+ """VindLU VQA.
+
+ Args:
+ text_decoder (dict): Backbone for extracting
+ multi-modal features. We apply this part as VQA fusion module.
+ answer_list_path (str, optional): Path to `answer_list.json`.
+ max_question_len (int): Max text length of question text.
+ Defaults to 25.
+ max_answer_len (int): Max text length of answer text. Defaults to 5.
+ num_ans_candidates (int): Number of answer candidates, used to filter
+ out answers with low probability. Defaults to 128.
+ **kwargs: Other keyword arguments accepted by the VindLUBase.
+ """
+
+ def __init__(self,
+ text_decoder: dict,
+ answer_list_path: Optional[str] = None,
+ max_question_len: int = 25,
+ max_answer_len: int = 5,
+ num_ans_candidates: int = 128,
+ **kwargs):
+ super().__init__(**kwargs)
+
+ self.max_question_len = max_question_len
+ self.max_answer_len = max_answer_len
+ self.num_ans_candidates = num_ans_candidates
+ self.answer_list_path = answer_list_path
+ self.text_decoder_cfg = text_decoder
+
+ # for inference only
+ if answer_list_path:
+ self.answer_list = mmengine.load(answer_list_path)
+
+ # delete extra/unnecessary modules inherited from VindLUBase
+ extra_attributes = ['vision_proj', 'text_proj', 'temp', 'itm_head']
+ for attr in extra_attributes:
+ delattr(self, attr)
+
+ self.text_decoder_cfg.gradient_checkpointing = \
+ self.gradient_checkpointing
+ self.text_decoder = MODELS.build(self.text_decoder_cfg)
+
+ def forward_encoder(self, inputs, data_samples):
+ # forward vision encoder
+ image_embeds, _ = self.encode_vision(inputs)
+ image_embeds = rearrange(image_embeds, 'b t l c -> b (t l) c')
+ image_atts = torch.ones(
+ image_embeds.size()[:-1], dtype=torch.long).to(inputs.device)
+
+ # forward text encoder
+ questions = [sample.question for sample in data_samples]
+ questions = self.tokenizer(
+ questions,
+ padding='max_length',
+ truncation=True,
+ max_length=self.max_question_len,
+ return_tensors='pt').to(inputs.device)
+
+ question_output = self.text_encoder(
+ questions.input_ids,
+ attention_mask=questions.attention_mask,
+ encoder_hidden_states=image_embeds,
+ encoder_attention_mask=image_atts,
+ return_dict=True)
+
+ return questions, question_output
+
+ def loss(self, inputs, data_samples):
+ """Calculate losses from a batch of inputs and data samples.
+
+ Args:
+ inputs (dict): A batch of inputs. The input tensor with of
+ at least one modality. For image, the value is a tensor
+ of shape (N, C, ...) in general.
+ For text, the value is a dict of tokenized text inputs.
+ data_samples (Optional[List[DataSample]]):
+ The annotation data of every samples. Defaults to None.
+
+ Returns:
+ Dict[str, torch.tensor]: a dictionary of loss components of
+ """
+
+ questions, question_output = self.forward_encoder(inputs, data_samples)
+
+ weights = torch.cat(
+ [torch.tensor(sample.gt_answer_weight) for sample in data_samples],
+ dim=0).to(inputs.device)
+ raw_answers = []
+ for sample in data_samples:
+ raw_answers.extend(sample.gt_answer)
+ answer_count = torch.tensor([
+ len(sample.gt_answer) for sample in data_samples
+ ]).to(inputs.device)
+ answers = [a + ' ' + '[SEP]' for a in raw_answers]
+ answers = self.tokenizer(
+ answers,
+ padding='max_length',
+ truncation=True,
+ max_length=self.max_answer_len,
+ return_tensors='pt').to(inputs.device)
+
+ answer_targets = answers.input_ids.masked_fill(
+ answers.input_ids == self.tokenizer.pad_token_id, -100)
+
+ question_states = []
+ question_atts = []
+ for b, n in enumerate(answer_count):
+ question_states += [question_output.last_hidden_state[b]] * n
+ question_atts += [questions.attention_mask[b]] * n
+ question_states = torch.stack(question_states, 0).to(inputs.device)
+ question_atts = torch.stack(question_atts, 0).to(inputs.device)
+
+ answer_output = self.text_decoder(
+ answers.input_ids,
+ attention_mask=answers.attention_mask,
+ encoder_hidden_states=question_states,
+ encoder_attention_mask=question_atts,
+ labels=answer_targets,
+ return_dict=True,
+ reduction='none',
+ )
+ loss = weights * answer_output.loss
+ loss = loss.sum() / inputs.size(0)
+
+ return dict(loss=loss)
+
+ def predict(self, inputs, data_samples, **kwargs):
+
+ questions, question_output = self.forward_encoder(inputs, data_samples)
+
+ raw_answers = self.answer_list
+ answers = [a + ' ' + '[SEP]' for a in raw_answers]
+ answers = self.tokenizer(
+ answers,
+ padding='max_length',
+ truncation=True,
+ max_length=self.max_answer_len,
+ return_tensors='pt',
+ ).to(inputs.device)
+
+ topk_ids, topk_probs = self.rank_answer(
+ question_output.last_hidden_state, questions.attention_mask,
+ answers.input_ids, answers.attention_mask, self.num_ans_candidates)
+
+ out_data_samples = []
+ for data_sample, topk_id, topk_prob in zip(data_samples, topk_ids,
+ topk_probs):
+ _, pred = topk_prob.max(dim=0)
+ data_sample.pred_answer = raw_answers[topk_id[pred]]
+ out_data_samples.append(data_sample)
+
+ return out_data_samples
+
+ def rank_answer(self, question_states, question_atts, answer_ids,
+ answer_atts, k):
+ """
+ question_states: (bsz, Lq, d)
+ answer_ids: answer input id after tokenization, (#answers, La)
+ """
+ num_ques = question_states.size(0)
+ start_ids = answer_ids[0, 0].repeat(num_ques, 1) # bos token
+
+ start_output = self.text_decoder(
+ start_ids,
+ encoder_hidden_states=question_states,
+ encoder_attention_mask=question_atts,
+ return_dict=True,
+ reduction='none',
+ )
+ logits = start_output.logits[:, 0, :] # first token's logit
+
+ # topk_probs: top-k probability
+ # topk_ids: [num_question, k]
+ answer_first_token = answer_ids[:, 1]
+ prob_first_token = F.softmax(
+ logits, dim=1).index_select(
+ dim=1, index=answer_first_token)
+ topk_probs, topk_ids = prob_first_token.topk(k, dim=1)
+
+ # answer input: [num_question*k, answer_len]
+ input_ids = []
+ input_atts = []
+ for b, topk_id in enumerate(topk_ids):
+ input_ids.append(answer_ids.index_select(dim=0, index=topk_id))
+ input_atts.append(answer_atts.index_select(dim=0, index=topk_id))
+ input_ids = torch.cat(input_ids, dim=0)
+ input_atts = torch.cat(input_atts, dim=0)
+
+ targets_ids = input_ids.masked_fill(
+ input_ids == self.tokenizer.pad_token_id, -100)
+
+ question_states = question_states.repeat_interleave(k, dim=0)
+ question_atts = question_atts.repeat_interleave(k, dim=0)
+
+ output = self.text_decoder(
+ input_ids,
+ attention_mask=input_atts,
+ encoder_hidden_states=question_states,
+ encoder_attention_mask=question_atts,
+ labels=targets_ids,
+ return_dict=True,
+ reduction='none',
+ )
+
+ answer_loss = output.loss
+ answer_loss = answer_loss.view(input_ids.size(0), -1)
+
+ # topk_prob: first token probability
+ topk_probs = topk_probs.view(-1, 1)
+ log_probs = torch.cat([topk_probs.log(), -answer_loss], dim=1)
+
+ # re-calculate log probabilities for the answer sequences
+ # using chain rule
+ log_probs_sum = log_probs.sum(1)
+ log_probs_sum = log_probs_sum.view(num_ques, k)
+
+ topk_probs = F.softmax(log_probs_sum, dim=-1)
+ # get top-k after re-ranking
+ topk_probs, rerank_id = topk_probs.topk(k, dim=1)
+ topk_ids = torch.gather(topk_ids, 1, rerank_id)
+
+ return topk_ids, topk_probs
+
+ def preprocess_state_dict(self, state_dict):
+ """Preprocess pretrained checkpoint for text_encoder and
+ text_decoder."""
+ for key in list(state_dict.keys()):
+ if 'bert' in key:
+ encoder_key = key.replace('bert.', '')
+ state_dict[encoder_key] = state_dict[key]
+
+ # init text decoder as multimodal encoder
+ # (last 6 layers of model.text_encoder)
+ # only for generation tasks like VQA
+ if self.text_decoder_cfg and 'text_encoder' in key:
+ if 'layer' in key:
+ encoder_keys = key.split('.')
+ layer_num = int(encoder_keys[4])
+ if layer_num < self.text_encoder_cfg.fusion_layer:
+ del state_dict[key]
+ continue
+ else:
+ decoder_layer_num = layer_num - 9
+ encoder_keys[4] = str(decoder_layer_num)
+ encoder_key = '.'.join(encoder_keys)
+ else:
+ encoder_key = key
+ decoder_key = encoder_key.replace('text_encoder',
+ 'text_decoder')
+ state_dict[decoder_key] = state_dict[key]
+ del state_dict[key]
+ return state_dict
diff --git a/mmaction/models/multimodal/vindlu/xbert.py b/mmaction/models/multimodal/vindlu/xbert.py
new file mode 100644
index 0000000000..df020ce535
--- /dev/null
+++ b/mmaction/models/multimodal/vindlu/xbert.py
@@ -0,0 +1,40 @@
+# Copyright (c) OpenMMLab. All rights reserved.
+from mmaction.registry import MODELS
+from .modeling_bert import (BertConfig, BertForMaskedLM, BertLMHeadModel,
+ BertModel)
+
+
+@MODELS.register_module()
+class XBertForMaskedLM(BertForMaskedLM):
+
+ def __init__(self, pretrained_model_name_or_path, fusion_layer,
+ encoder_width, **kwargs):
+ config = BertConfig.from_pretrained(pretrained_model_name_or_path)
+ config.fusion_layer = fusion_layer
+ config.encoder_width = encoder_width
+ config.update(kwargs)
+ super().__init__(config)
+
+
+@MODELS.register_module()
+class XBertModel(BertModel):
+
+ def __init__(self, pretrained_model_name_or_path, fusion_layer,
+ encoder_width, add_pooling_layer, **kwargs):
+ config = BertConfig.from_pretrained(pretrained_model_name_or_path)
+ config.fusion_layer = fusion_layer
+ config.encoder_width = encoder_width
+ config.update(kwargs)
+ super().__init__(config, add_pooling_layer)
+
+
+@MODELS.register_module()
+class BertDecoder(BertLMHeadModel):
+
+ def __init__(self, pretrained_model_name_or_path, fusion_layer,
+ encoder_width, **kwargs):
+ config = BertConfig.from_pretrained(pretrained_model_name_or_path)
+ config.fusion_layer = fusion_layer
+ config.encoder_width = encoder_width
+ config.update(kwargs)
+ super().__init__(config)
diff --git a/mmaction/models/necks/tpn.py b/mmaction/models/necks/tpn.py
index b3cdc92ff9..c04dde4123 100644
--- a/mmaction/models/necks/tpn.py
+++ b/mmaction/models/necks/tpn.py
@@ -254,7 +254,7 @@ def loss(self, x: torch.Tensor,
data_samples: Optional[SampleList]) -> dict:
"""Calculate auxiliary loss."""
x = self(x)
- labels = [x.gt_labels.item for x in data_samples]
+ labels = [x.gt_label for x in data_samples]
labels = torch.stack(labels).to(x.device)
labels = labels.squeeze()
if labels.shape == torch.Size([]):
diff --git a/mmaction/models/recognizers/base.py b/mmaction/models/recognizers/base.py
index 7ce2a51b1f..ced45380cf 100644
--- a/mmaction/models/recognizers/base.py
+++ b/mmaction/models/recognizers/base.py
@@ -162,7 +162,7 @@ def loss(self, inputs: torch.Tensor, data_samples: SampleList,
These should usually be mean centered and std scaled.
data_samples (List[``ActionDataSample``]): The batch
data samples. It usually includes information such
- as ``gt_labels``.
+ as ``gt_label``.
Returns:
dict: A dictionary of loss components.
@@ -187,7 +187,7 @@ def predict(self, inputs: torch.Tensor, data_samples: SampleList,
These should usually be mean centered and std scaled.
data_samples (List[``ActionDataSample``]): The batch
data samples. It usually includes information such
- as ``gt_labels``.
+ as ``gt_label``.
Returns:
List[``ActionDataSample``]: Return the recognition results.
diff --git a/mmaction/models/roi_heads/__init__.py b/mmaction/models/roi_heads/__init__.py
index 6ff62a1929..d7d413bb79 100644
--- a/mmaction/models/roi_heads/__init__.py
+++ b/mmaction/models/roi_heads/__init__.py
@@ -1,10 +1,23 @@
# Copyright (c) OpenMMLab. All rights reserved.
-from .bbox_heads import BBoxHeadAVA
-from .roi_extractors import SingleRoIExtractor3D
-from .roi_head import AVARoIHead
-from .shared_heads import ACRNHead, FBOHead, LFBInferHead
-
-__all__ = [
- 'AVARoIHead', 'BBoxHeadAVA', 'SingleRoIExtractor3D', 'ACRNHead', 'FBOHead',
- 'LFBInferHead'
-]
+try:
+ from mmdet.registry import MODELS as MMDET_MODELS
+
+ from .bbox_heads import BBoxHeadAVA
+ from .roi_extractors import SingleRoIExtractor3D
+ from .roi_head import AVARoIHead
+ from .shared_heads import ACRNHead, FBOHead, LFBInferHead
+
+ for module in [
+ AVARoIHead, BBoxHeadAVA, SingleRoIExtractor3D, ACRNHead, FBOHead,
+ LFBInferHead
+ ]:
+
+ MMDET_MODELS.register_module()(module)
+
+ __all__ = [
+ 'AVARoIHead', 'BBoxHeadAVA', 'SingleRoIExtractor3D', 'ACRNHead',
+ 'FBOHead', 'LFBInferHead'
+ ]
+
+except (ImportError, ModuleNotFoundError):
+ pass
diff --git a/mmaction/models/roi_heads/bbox_heads/bbox_head.py b/mmaction/models/roi_heads/bbox_heads/bbox_head.py
index 3fad373cf2..7faa632b18 100644
--- a/mmaction/models/roi_heads/bbox_heads/bbox_head.py
+++ b/mmaction/models/roi_heads/bbox_heads/bbox_head.py
@@ -5,25 +5,17 @@
import torch
import torch.nn as nn
import torch.nn.functional as F
+from mmdet.models.task_modules.samplers import SamplingResult
from mmengine.config import ConfigDict
from mmengine.structures import InstanceData
-from torch import Tensor
-
-from mmaction.structures.bbox import bbox_target
-from mmaction.utils import InstanceList
-
-try:
- from mmdet.models.task_modules.samplers import SamplingResult
- from mmdet.registry import MODELS as MMDET_MODELS
- mmdet_imported = True
-except (ImportError, ModuleNotFoundError):
- from mmaction.utils import SamplingResult
- mmdet_imported = False
-
# Resolve cross-entropy function to support multi-target in Torch < 1.10
# This is a very basic 'hack', with minimal functionality to support the
# procedure under prior torch versions
from packaging import version as pv
+from torch import Tensor
+
+from mmaction.structures.bbox import bbox_target
+from mmaction.utils import InstanceList
if pv.parse(torch.__version__) < pv.parse('1.10'):
@@ -44,6 +36,8 @@ class BBoxHeadAVA(nn.Module):
"""Simplest RoI head, with only one fc layer for classification.
Args:
+ background_class (bool): Whether set class 0 as background class and
+ ignore it when calculate loss.
temporal_pool_type (str): The temporal pool type. Choices are ``avg``
or ``max``. Defaults to ``avg``.
spatial_pool_type (str): The spatial pool type. Choices are ``avg`` or
@@ -70,6 +64,7 @@ class BBoxHeadAVA(nn.Module):
def __init__(
self,
+ background_class: bool,
temporal_pool_type: str = 'avg',
spatial_pool_type: str = 'max',
in_channels: int = 2048,
@@ -98,6 +93,8 @@ def __init__(
self.focal_gamma = focal_gamma
self.focal_alpha = focal_alpha
+ self.background_class = background_class
+
if topk is None:
self.topk = ()
elif isinstance(topk, int):
@@ -251,9 +248,11 @@ def loss_and_target(self, cls_score: Tensor, rois: Tensor,
losses = dict()
# Only use the cls_score
if cls_score is not None:
- labels = labels[:, 1:] # Get valid labels (ignore first one)
+ if self.background_class:
+ labels = labels[:, 1:] # Get valid labels (ignore first one)
+ cls_score = cls_score[:, 1:]
pos_inds = torch.sum(labels, dim=-1) > 0
- cls_score = cls_score[pos_inds, 1:]
+ cls_score = cls_score[pos_inds]
labels = labels[pos_inds]
# Compute First Recall/Precisions
@@ -268,7 +267,7 @@ def loss_and_target(self, cls_score: Tensor, rois: Tensor,
# If Single-label, need to ensure that target labels sum to 1: ie
# that they are valid probabilities.
- if not self.multilabel:
+ if not self.multilabel and self.background_class:
labels = labels / labels.sum(dim=1, keepdim=True)
# Select Loss function based on single/multi-label
@@ -414,7 +413,3 @@ def _bbox_crop_undo(bboxes, crop_quadruple):
results.scores = scores
return results
-
-
-if mmdet_imported:
- MMDET_MODELS.register_module()(BBoxHeadAVA)
diff --git a/mmaction/models/roi_heads/roi_extractors/single_straight3d.py b/mmaction/models/roi_heads/roi_extractors/single_straight3d.py
index 6a1044cd74..242b1a5d13 100644
--- a/mmaction/models/roi_heads/roi_extractors/single_straight3d.py
+++ b/mmaction/models/roi_heads/roi_extractors/single_straight3d.py
@@ -6,12 +6,6 @@
import torch.nn.functional as F
from torch import Tensor
-try:
- from mmdet.registry import MODELS as MMDET_MODELS
- mmdet_imported = True
-except (ImportError, ModuleNotFoundError):
- mmdet_imported = False
-
class SingleRoIExtractor3D(nn.Module):
"""Extract RoI features from a single level feature map.
@@ -130,7 +124,3 @@ def forward(self, feat: Union[Tensor, Tuple[Tensor]],
roi_feats = torch.stack(roi_feats, dim=2)
return roi_feats, feat
-
-
-if mmdet_imported:
- MMDET_MODELS.register_module()(SingleRoIExtractor3D)
diff --git a/mmaction/models/roi_heads/roi_head.py b/mmaction/models/roi_heads/roi_head.py
index baa1b42e77..f98d5fe39c 100644
--- a/mmaction/models/roi_heads/roi_head.py
+++ b/mmaction/models/roi_heads/roi_head.py
@@ -1,227 +1,206 @@
# Copyright (c) OpenMMLab. All rights reserved.
from typing import List, Tuple, Union
+from mmdet.models.roi_heads import StandardRoIHead
+from mmdet.models.task_modules.samplers import SamplingResult
+from mmdet.structures.bbox import bbox2roi
from torch import Tensor
from mmaction.utils import ConfigType, InstanceList, SampleList
-try:
- from mmdet.models.roi_heads import StandardRoIHead
- from mmdet.models.task_modules.samplers import SamplingResult
- from mmdet.registry import MODELS as MMDET_MODELS
- from mmdet.structures.bbox import bbox2roi
- mmdet_imported = True
-except (ImportError, ModuleNotFoundError):
- from mmaction.utils import SamplingResult
- mmdet_imported = False
-
-if mmdet_imported:
-
- @MMDET_MODELS.register_module()
- class AVARoIHead(StandardRoIHead):
-
- def loss(self, x: Union[Tensor,
- Tuple[Tensor]], rpn_results_list: InstanceList,
- data_samples: SampleList, **kwargs) -> dict:
- """Perform forward propagation and loss calculation of the
- detection roi on the features of the upstream network.
-
- Args:
- x (Tensor or Tuple[Tensor]): The image features extracted by
- the upstream network.
- rpn_results_list (List[:obj:`InstanceData`]): List of region
- proposals.
- data_samples (List[:obj:`ActionDataSample`]): The batch
- data samples.
-
- Returns:
- Dict[str, Tensor]: A dictionary of loss components.
- """
- assert len(rpn_results_list) == len(data_samples)
- batch_gt_instances = []
- for data_sample in data_samples:
- batch_gt_instances.append(data_sample.gt_instances)
-
- # assign gts and sample proposals
- num_imgs = len(data_samples)
- sampling_results = []
- for i in range(num_imgs):
- # rename rpn_results.bboxes to rpn_results.priors
- rpn_results = rpn_results_list[i]
- rpn_results.priors = rpn_results.pop('bboxes')
-
- assign_result = self.bbox_assigner.assign(
- rpn_results, batch_gt_instances[i], None)
- sampling_result = self.bbox_sampler.sample(
- assign_result, rpn_results, batch_gt_instances[i])
- sampling_results.append(sampling_result)
-
- # LFB needs meta_info: 'img_key'
- batch_img_metas = [
- data_samples.metainfo for data_samples in data_samples
- ]
-
- losses = dict()
- # bbox head forward and loss
- bbox_results = self.bbox_loss(x, sampling_results, batch_img_metas)
- losses.update(bbox_results['loss_bbox'])
-
- return losses
-
- def _bbox_forward(self, x: Union[Tensor, Tuple[Tensor]], rois: Tensor,
- batch_img_metas: List[dict], **kwargs) -> dict:
- """Box head forward function used in both training and testing.
-
- Args:
- x (Tensor or Tuple[Tensor]): The image features extracted by
- the upstream network.
- rois (Tensor): RoIs with the shape (n, 5) where the first
- column indicates batch id of each RoI.
- batch_img_metas (List[dict]): List of image information.
-
- Returns:
- dict[str, Tensor]: Usually returns a dictionary with keys:
-
- - `cls_score` (Tensor): Classification scores.
- - `bbox_pred` (Tensor): Box energies / deltas.
- - `bbox_feats` (Tensor): Extract bbox RoI features.
- """
- bbox_feats, global_feat = self.bbox_roi_extractor(x, rois)
-
- if self.with_shared_head:
- bbox_feats = self.shared_head(
- bbox_feats,
- feat=global_feat,
- rois=rois,
- img_metas=batch_img_metas)
-
- cls_score = self.bbox_head(bbox_feats)
-
- bbox_results = dict(cls_score=cls_score, bbox_feats=bbox_feats)
- return bbox_results
-
- def bbox_loss(self, x: Union[Tensor, Tuple[Tensor]],
- sampling_results: List[SamplingResult],
+
+class AVARoIHead(StandardRoIHead):
+
+ def loss(self, x: Union[Tensor,
+ Tuple[Tensor]], rpn_results_list: InstanceList,
+ data_samples: SampleList, **kwargs) -> dict:
+ """Perform forward propagation and loss calculation of the detection
+ roi on the features of the upstream network.
+
+ Args:
+ x (Tensor or Tuple[Tensor]): The image features extracted by
+ the upstream network.
+ rpn_results_list (List[:obj:`InstanceData`]): List of region
+ proposals.
+ data_samples (List[:obj:`ActionDataSample`]): The batch
+ data samples.
+
+ Returns:
+ Dict[str, Tensor]: A dictionary of loss components.
+ """
+ assert len(rpn_results_list) == len(data_samples)
+ batch_gt_instances = []
+ for data_sample in data_samples:
+ batch_gt_instances.append(data_sample.gt_instances)
+
+ # assign gts and sample proposals
+ num_imgs = len(data_samples)
+ sampling_results = []
+ for i in range(num_imgs):
+ # rename rpn_results.bboxes to rpn_results.priors
+ rpn_results = rpn_results_list[i]
+ rpn_results.priors = rpn_results.pop('bboxes')
+
+ assign_result = self.bbox_assigner.assign(rpn_results,
+ batch_gt_instances[i],
+ None)
+ sampling_result = self.bbox_sampler.sample(assign_result,
+ rpn_results,
+ batch_gt_instances[i])
+ sampling_results.append(sampling_result)
+
+ # LFB needs meta_info: 'img_key'
+ batch_img_metas = [
+ data_samples.metainfo for data_samples in data_samples
+ ]
+
+ losses = dict()
+ # bbox head forward and loss
+ bbox_results = self.bbox_loss(x, sampling_results, batch_img_metas)
+ losses.update(bbox_results['loss_bbox'])
+
+ return losses
+
+ def _bbox_forward(self, x: Union[Tensor, Tuple[Tensor]], rois: Tensor,
batch_img_metas: List[dict], **kwargs) -> dict:
- """Perform forward propagation and loss calculation of the bbox
- head on the features of the upstream network.
+ """Box head forward function used in both training and testing.
- Args:
- x (Tensor or Tuple[Tensor]): The image features extracted by
- the upstream network.
- sampling_results (List[SamplingResult]): Sampling results.
- batch_img_metas (List[dict]): List of image information.
+ Args:
+ x (Tensor or Tuple[Tensor]): The image features extracted by
+ the upstream network.
+ rois (Tensor): RoIs with the shape (n, 5) where the first
+ column indicates batch id of each RoI.
+ batch_img_metas (List[dict]): List of image information.
- Returns:
+ Returns:
dict[str, Tensor]: Usually returns a dictionary with keys:
- - `cls_score` (Tensor): Classification scores.
- - `bbox_pred` (Tensor): Box energies / deltas.
- - `bbox_feats` (Tensor): Extract bbox RoI features.
- - `loss_bbox` (dict): A dictionary of bbox loss components.
- """
- rois = bbox2roi([res.priors for res in sampling_results])
- bbox_results = self._bbox_forward(x, rois, batch_img_metas)
+ - `cls_score` (Tensor): Classification scores.
+ - `bbox_pred` (Tensor): Box energies / deltas.
+ - `bbox_feats` (Tensor): Extract bbox RoI features.
+ """
+ bbox_feats, global_feat = self.bbox_roi_extractor(x, rois)
- bbox_loss_and_target = self.bbox_head.loss_and_target(
- cls_score=bbox_results['cls_score'],
- rois=rois,
- sampling_results=sampling_results,
- rcnn_train_cfg=self.train_cfg)
-
- bbox_results.update(loss_bbox=bbox_loss_and_target['loss_bbox'])
- return bbox_results
-
- def predict(self, x: Union[Tensor, Tuple[Tensor]],
- rpn_results_list: InstanceList, data_samples: SampleList,
- **kwargs) -> InstanceList:
- """Perform forward propagation of the roi head and predict
- detection results on the features of the upstream network.
-
- Args:
- x (Tensor or Tuple[Tensor]): The image features extracted by
- the upstream network.
- rpn_results_list (List[:obj:`InstanceData`]): list of region
- proposals.
- data_samples (List[:obj:`ActionDataSample`]): The batch
- data samples.
-
- Returns:
- List[obj:`InstanceData`]: Detection results of each image.
- Each item usually contains following keys.
-
- - scores (Tensor): Classification scores, has a shape
- (num_instance, )
- - labels (Tensor): Labels of bboxes, has a shape
- (num_instances, ).
- """
- assert self.with_bbox, 'Bbox head must be implemented.'
- batch_img_metas = [
- data_samples.metainfo for data_samples in data_samples
- ]
- if isinstance(x, tuple):
- x_shape = x[0].shape
- else:
- x_shape = x.shape
-
- assert x_shape[0] == 1, 'only accept 1 sample at test mode'
- assert x_shape[0] == len(batch_img_metas) == len(rpn_results_list)
-
- results_list = self.predict_bbox(
- x,
- batch_img_metas,
- rpn_results_list,
- rcnn_test_cfg=self.test_cfg)
-
- return results_list
-
- def predict_bbox(self, x: Tuple[Tensor], batch_img_metas: List[dict],
- rpn_results_list: InstanceList,
- rcnn_test_cfg: ConfigType) -> InstanceList:
- """Perform forward propagation of the bbox head and predict
- detection results on the features of the upstream network.
-
- Args:
- x (tuple[Tensor]): Feature maps of all scale level.
- batch_img_metas (list[dict]): List of image information.
- rpn_results_list (list[:obj:`InstanceData`]): List of region
- proposals.
- rcnn_test_cfg (obj:`ConfigDict`): `test_cfg` of R-CNN.
-
- Returns:
- list[:obj:`InstanceData`]: Detection results of each image
- after the post process. Each item usually contains following
- keys:
- - scores (Tensor): Classification scores, has a shape
- (num_instance, )
- - labels (Tensor): Labels of bboxes, has a shape
- (num_instances, ).
- """
- proposals = [res.bboxes for res in rpn_results_list]
- rois = bbox2roi(proposals)
- bbox_results = self._bbox_forward(x, rois, batch_img_metas)
-
- # split batch bbox prediction back to each image
- cls_scores = bbox_results['cls_score']
- num_proposals_per_img = tuple(len(p) for p in proposals)
- rois = rois.split(num_proposals_per_img, 0)
- cls_scores = cls_scores.split(num_proposals_per_img, 0)
-
- result_list = self.bbox_head.predict_by_feat(
+ if self.with_shared_head:
+ bbox_feats = self.shared_head(
+ bbox_feats,
+ feat=global_feat,
rois=rois,
- cls_scores=cls_scores,
- batch_img_metas=batch_img_metas,
- rcnn_test_cfg=rcnn_test_cfg)
-
- return result_list
-else:
- # Just define an empty class, so that __init__ can import it.
- class AVARoIHead:
-
- def __init__(self, *args, **kwargs):
- raise ImportError(
- 'Failed to import `bbox2roi` from `mmdet.core.bbox`, '
- 'or failed to import `MODELS` from `mmdet.registry`, '
- 'or failed to import `StandardRoIHead` from '
- '`mmdet.models.roi_heads`. You will be unable to use '
- '`AVARoIHead`. ')
+ img_metas=batch_img_metas)
+
+ cls_score = self.bbox_head(bbox_feats)
+
+ bbox_results = dict(cls_score=cls_score, bbox_feats=bbox_feats)
+ return bbox_results
+
+ def bbox_loss(self, x: Union[Tensor, Tuple[Tensor]],
+ sampling_results: List[SamplingResult],
+ batch_img_metas: List[dict], **kwargs) -> dict:
+ """Perform forward propagation and loss calculation of the bbox head on
+ the features of the upstream network.
+
+ Args:
+ x (Tensor or Tuple[Tensor]): The image features extracted by
+ the upstream network.
+ sampling_results (List[SamplingResult]): Sampling results.
+ batch_img_metas (List[dict]): List of image information.
+
+ Returns:
+ dict[str, Tensor]: Usually returns a dictionary with keys:
+
+ - `cls_score` (Tensor): Classification scores.
+ - `bbox_pred` (Tensor): Box energies / deltas.
+ - `bbox_feats` (Tensor): Extract bbox RoI features.
+ - `loss_bbox` (dict): A dictionary of bbox loss components.
+ """
+ rois = bbox2roi([res.priors for res in sampling_results])
+ bbox_results = self._bbox_forward(x, rois, batch_img_metas)
+
+ bbox_loss_and_target = self.bbox_head.loss_and_target(
+ cls_score=bbox_results['cls_score'],
+ rois=rois,
+ sampling_results=sampling_results,
+ rcnn_train_cfg=self.train_cfg)
+
+ bbox_results.update(loss_bbox=bbox_loss_and_target['loss_bbox'])
+ return bbox_results
+
+ def predict(self, x: Union[Tensor,
+ Tuple[Tensor]], rpn_results_list: InstanceList,
+ data_samples: SampleList, **kwargs) -> InstanceList:
+ """Perform forward propagation of the roi head and predict detection
+ results on the features of the upstream network.
+
+ Args:
+ x (Tensor or Tuple[Tensor]): The image features extracted by
+ the upstream network.
+ rpn_results_list (List[:obj:`InstanceData`]): list of region
+ proposals.
+ data_samples (List[:obj:`ActionDataSample`]): The batch
+ data samples.
+
+ Returns:
+ List[obj:`InstanceData`]: Detection results of each image.
+ Each item usually contains following keys.
+
+ - scores (Tensor): Classification scores, has a shape
+ (num_instance, )
+ - labels (Tensor): Labels of bboxes, has a shape
+ (num_instances, ).
+ """
+ assert self.with_bbox, 'Bbox head must be implemented.'
+ batch_img_metas = [
+ data_samples.metainfo for data_samples in data_samples
+ ]
+ if isinstance(x, tuple):
+ x_shape = x[0].shape
+ else:
+ x_shape = x.shape
+
+ assert x_shape[0] == 1, 'only accept 1 sample at test mode'
+ assert x_shape[0] == len(batch_img_metas) == len(rpn_results_list)
+
+ results_list = self.predict_bbox(
+ x, batch_img_metas, rpn_results_list, rcnn_test_cfg=self.test_cfg)
+
+ return results_list
+
+ def predict_bbox(self, x: Tuple[Tensor], batch_img_metas: List[dict],
+ rpn_results_list: InstanceList,
+ rcnn_test_cfg: ConfigType) -> InstanceList:
+ """Perform forward propagation of the bbox head and predict detection
+ results on the features of the upstream network.
+
+ Args:
+ x (tuple[Tensor]): Feature maps of all scale level.
+ batch_img_metas (list[dict]): List of image information.
+ rpn_results_list (list[:obj:`InstanceData`]): List of region
+ proposals.
+ rcnn_test_cfg (obj:`ConfigDict`): `test_cfg` of R-CNN.
+
+ Returns:
+ list[:obj:`InstanceData`]: Detection results of each image
+ after the post process. Each item usually contains following
+ keys:
+ - scores (Tensor): Classification scores, has a shape
+ (num_instance, )
+ - labels (Tensor): Labels of bboxes, has a shape
+ (num_instances, ).
+ """
+ proposals = [res.bboxes for res in rpn_results_list]
+ rois = bbox2roi(proposals)
+ bbox_results = self._bbox_forward(x, rois, batch_img_metas)
+
+ # split batch bbox prediction back to each image
+ cls_scores = bbox_results['cls_score']
+ num_proposals_per_img = tuple(len(p) for p in proposals)
+ rois = rois.split(num_proposals_per_img, 0)
+ cls_scores = cls_scores.split(num_proposals_per_img, 0)
+
+ result_list = self.bbox_head.predict_by_feat(
+ rois=rois,
+ cls_scores=cls_scores,
+ batch_img_metas=batch_img_metas,
+ rcnn_test_cfg=rcnn_test_cfg)
+
+ return result_list
diff --git a/mmaction/models/roi_heads/shared_heads/acrn_head.py b/mmaction/models/roi_heads/shared_heads/acrn_head.py
index 3271c74bbe..5c37e2c1ec 100644
--- a/mmaction/models/roi_heads/shared_heads/acrn_head.py
+++ b/mmaction/models/roi_heads/shared_heads/acrn_head.py
@@ -5,18 +5,9 @@
from mmengine.model.weight_init import constant_init, kaiming_init
from mmengine.utils.dl_utils.parrots_wrapper import _BatchNorm
-from mmaction.registry import MODELS
-
-try:
- from mmdet.registry import MODELS as MMDET_MODELS
- mmdet_imported = True
-except (ImportError, ModuleNotFoundError):
- mmdet_imported = False
-
# Note: All these heads take 5D Tensors as input (N, C, T, H, W)
-@MODELS.register_module()
class ACRNHead(nn.Module):
"""ACRN Head: Tile + 1x1 convolution + 3x3 convolution.
@@ -132,7 +123,3 @@ def forward(self, x, feat, rois, **kwargs):
new_feat = conv(new_feat)
return new_feat
-
-
-if mmdet_imported:
- MMDET_MODELS.register_module()(ACRNHead)
diff --git a/mmaction/models/roi_heads/shared_heads/fbo_head.py b/mmaction/models/roi_heads/shared_heads/fbo_head.py
index aeb9c28514..8f4cba20ac 100644
--- a/mmaction/models/roi_heads/shared_heads/fbo_head.py
+++ b/mmaction/models/roi_heads/shared_heads/fbo_head.py
@@ -10,15 +10,8 @@
from mmengine.runner import load_checkpoint
from mmengine.utils.dl_utils.parrots_wrapper import _BatchNorm
-from mmaction.registry import MODELS
from .lfb import LFB
-try:
- from mmdet.registry import MODELS as MMDET_MODELS
- mmdet_imported = True
-except (ImportError, ModuleNotFoundError):
- mmdet_imported = False
-
class NonLocalLayer(nn.Module):
"""Non-local layer used in `FBONonLocal` is a variation of the vanilla non-
@@ -322,7 +315,6 @@ def forward(self, st_feat, lt_feat):
return out
-@MODELS.register_module()
class FBOHead(nn.Module):
"""Feature Bank Operator Head.
@@ -403,7 +395,3 @@ def forward(self, x, rois, img_metas, **kwargs):
out = torch.cat([identity, fbo_feat], dim=1)
return out
-
-
-if mmdet_imported:
- MMDET_MODELS.register_module()(FBOHead)
diff --git a/mmaction/models/roi_heads/shared_heads/lfb_infer_head.py b/mmaction/models/roi_heads/shared_heads/lfb_infer_head.py
index fdf71092a1..d19fc36203 100644
--- a/mmaction/models/roi_heads/shared_heads/lfb_infer_head.py
+++ b/mmaction/models/roi_heads/shared_heads/lfb_infer_head.py
@@ -6,18 +6,9 @@
import torch.distributed as dist
import torch.nn as nn
-from mmaction.registry import MODELS
-
-try:
- from mmdet.registry import MODELS as MMDET_MODELS
- mmdet_imported = True
-except (ImportError, ModuleNotFoundError):
- mmdet_imported = False
-
# Note: All these heads take 5D Tensors as input (N, C, T, H, W)
-@MODELS.register_module()
class LFBInferHead(nn.Module):
"""Long-Term Feature Bank Infer Head.
@@ -155,7 +146,3 @@ def __del__(self):
osp.join(self.lfb_prefix_path, f'lfb_{self.dataset_mode}.pkl'))
torch.save(lfb, lfb_file_path)
print(f'LFB has been constructed in {lfb_file_path}!')
-
-
-if mmdet_imported:
- MMDET_MODELS.register_module()(LFBInferHead)
diff --git a/mmaction/models/task_modules/__init__.py b/mmaction/models/task_modules/__init__.py
index 9a6d4e76de..7fc1d7769e 100644
--- a/mmaction/models/task_modules/__init__.py
+++ b/mmaction/models/task_modules/__init__.py
@@ -1,4 +1,12 @@
# Copyright (c) OpenMMLab. All rights reserved.
-from .assigners import MaxIoUAssignerAVA
+try:
+ from mmdet.registry import TASK_UTILS as MMDET_TASK_UTILS
-__all__ = ['MaxIoUAssignerAVA']
+ from .assigners import MaxIoUAssignerAVA
+
+ MMDET_TASK_UTILS.register_module()(MaxIoUAssignerAVA)
+
+ __all__ = ['MaxIoUAssignerAVA']
+
+except (ImportError, ModuleNotFoundError):
+ pass
diff --git a/mmaction/models/task_modules/assigners/max_iou_assigner_ava.py b/mmaction/models/task_modules/assigners/max_iou_assigner_ava.py
index 89fb6044ac..604065829f 100644
--- a/mmaction/models/task_modules/assigners/max_iou_assigner_ava.py
+++ b/mmaction/models/task_modules/assigners/max_iou_assigner_ava.py
@@ -1,138 +1,119 @@
# Copyright (c) OpenMMLab. All rights reserved.
import torch
+from mmdet.models.task_modules import AssignResult, MaxIoUAssigner
from torch import Tensor
-try:
- from mmdet.models.task_modules import AssignResult, MaxIoUAssigner
- from mmdet.registry import TASK_UTILS as MMDET_TASK_UTILS
- mmdet_imported = True
-except (ImportError, ModuleNotFoundError):
- mmdet_imported = False
-if mmdet_imported:
+class MaxIoUAssignerAVA(MaxIoUAssigner):
+ """Assign a corresponding gt bbox or background to each bbox.
- @MMDET_TASK_UTILS.register_module()
- class MaxIoUAssignerAVA(MaxIoUAssigner):
- """Assign a corresponding gt bbox or background to each bbox.
+ Each proposals will be assigned with `-1`, `0`, or a positive integer
+ indicating the ground truth index.
- Each proposals will be assigned with `-1`, `0`, or a positive integer
- indicating the ground truth index.
+ - -1: don't care
+ - 0: negative sample, no assigned gt
+ - positive integer: positive sample, index (1-based) of assigned gt
- - -1: don't care
- - 0: negative sample, no assigned gt
- - positive integer: positive sample, index (1-based) of assigned gt
+ Args:
+ pos_iou_thr (float): IoU threshold for positive bboxes.
+ neg_iou_thr (float | tuple): IoU threshold for negative bboxes.
+ min_pos_iou (float): Minimum iou for a bbox to be considered as a
+ positive bbox. Positive samples can have smaller IoU than
+ pos_iou_thr due to the 4th step (assign max IoU sample to each
+ gt). Defaults to 0.
+ gt_max_assign_all (bool): Whether to assign all bboxes with the
+ same highest overlap with some gt to that gt. Defaults to True.
+ """
- Args:
- pos_iou_thr (float): IoU threshold for positive bboxes.
- neg_iou_thr (float | tuple): IoU threshold for negative bboxes.
- min_pos_iou (float): Minimum iou for a bbox to be considered as a
- positive bbox. Positive samples can have smaller IoU than
- pos_iou_thr due to the 4th step (assign max IoU sample to each
- gt). Defaults to 0.
- gt_max_assign_all (bool): Whether to assign all bboxes with the
- same highest overlap with some gt to that gt. Defaults to True.
- """
+ # The function is overridden, to handle the case that gt_label is not
+ # int
+ def assign_wrt_overlaps(self, overlaps: Tensor,
+ gt_labels: Tensor) -> AssignResult:
+ """Assign w.r.t. the overlaps of bboxes with gts.
- # The function is overridden, to handle the case that gt_label is not
- # int
- def assign_wrt_overlaps(self, overlaps: Tensor,
- gt_labels: Tensor) -> AssignResult:
- """Assign w.r.t. the overlaps of bboxes with gts.
-
- Args:
- overlaps (Tensor): Overlaps between k gt_bboxes and n bboxes,
- shape(k, n).
- gt_labels (Tensor): Labels of k gt_bboxes, shape
- (k, num_classes).
-
- Returns:
- :obj:`AssignResult`: The assign result.
- """
- num_gts, num_bboxes = overlaps.size(0), overlaps.size(1)
-
- # 1. assign -1 by default
- assigned_gt_inds = overlaps.new_full((num_bboxes, ),
- -1,
- dtype=torch.long)
-
- if num_gts == 0 or num_bboxes == 0:
- # No ground truth or boxes, return empty assignment
- max_overlaps = overlaps.new_zeros((num_bboxes, ))
- assigned_labels = overlaps.new_full((num_bboxes, ),
- -1,
- dtype=torch.long)
- if num_gts == 0:
- # No truth, assign everything to background
- assigned_gt_inds[:] = 0
- return AssignResult(
- num_gts=num_gts,
- gt_inds=assigned_gt_inds,
- max_overlaps=max_overlaps,
- labels=assigned_labels)
-
- # for each anchor, which gt best overlaps with it
- # for each anchor, the max iou of all gts
- max_overlaps, argmax_overlaps = overlaps.max(dim=0)
- # for each gt, which anchor best overlaps with it
- # for each gt, the max iou of all proposals
- gt_max_overlaps, gt_argmax_overlaps = overlaps.max(dim=1)
-
- # 2. assign negative: below
- # the negative inds are set to be 0
- if isinstance(self.neg_iou_thr, float):
- assigned_gt_inds[(max_overlaps >= 0)
- & (max_overlaps < self.neg_iou_thr)] = 0
- elif isinstance(self.neg_iou_thr, tuple):
- assert len(self.neg_iou_thr) == 2
- assigned_gt_inds[(max_overlaps >= self.neg_iou_thr[0])
- & (max_overlaps < self.neg_iou_thr[1])] = 0
-
- # 3. assign positive: above positive IoU threshold
- pos_inds = max_overlaps >= self.pos_iou_thr
- assigned_gt_inds[pos_inds] = argmax_overlaps[pos_inds] + 1
-
- if self.match_low_quality:
- # Low-quality matching will overwrite the assigned_gt_inds
- # assigned in Step 3. Thus, the assigned gt might not be the
- # best one for prediction.
- # For example, if bbox A has 0.9 and 0.8 iou with GT bbox
- # 1 & 2, bbox 1 will be assigned as the best target for bbox A
- # in step 3. However, if GT bbox 2's gt_argmax_overlaps = A,
- # bbox A's assigned_gt_inds will be overwritten to be bbox B.
- # This might be the reason that it is not used in ROI Heads.
- for i in range(num_gts):
- if gt_max_overlaps[i] >= self.min_pos_iou:
- if self.gt_max_assign_all:
- max_iou_inds = overlaps[i, :] == gt_max_overlaps[i]
- assigned_gt_inds[max_iou_inds] = i + 1
- else:
- assigned_gt_inds[gt_argmax_overlaps[i]] = i + 1
-
- # consider multi-class case (AVA)
- assert len(gt_labels[0]) > 1
- assigned_labels = assigned_gt_inds.new_zeros(
- (num_bboxes, len(gt_labels[0])), dtype=torch.float32)
-
- # If not assigned, labels will be all 0
- pos_inds = torch.nonzero(
- assigned_gt_inds > 0, as_tuple=False).squeeze()
- if pos_inds.numel() > 0:
- assigned_labels[pos_inds] = gt_labels[
- assigned_gt_inds[pos_inds] - 1]
+ Args:
+ overlaps (Tensor): Overlaps between k gt_bboxes and n bboxes,
+ shape(k, n).
+ gt_labels (Tensor): Labels of k gt_bboxes, shape
+ (k, num_classes).
+ Returns:
+ :obj:`AssignResult`: The assign result.
+ """
+ num_gts, num_bboxes = overlaps.size(0), overlaps.size(1)
+
+ # 1. assign -1 by default
+ assigned_gt_inds = overlaps.new_full((num_bboxes, ),
+ -1,
+ dtype=torch.long)
+
+ if num_gts == 0 or num_bboxes == 0:
+ # No ground truth or boxes, return empty assignment
+ max_overlaps = overlaps.new_zeros((num_bboxes, ))
+ assigned_labels = overlaps.new_full((num_bboxes, ),
+ -1,
+ dtype=torch.long)
+ if num_gts == 0:
+ # No truth, assign everything to background
+ assigned_gt_inds[:] = 0
return AssignResult(
num_gts=num_gts,
gt_inds=assigned_gt_inds,
max_overlaps=max_overlaps,
labels=assigned_labels)
-else:
- # define an empty class, so that can be imported
- class MaxIoUAssignerAVA:
-
- def __init__(self, *args, **kwargs):
- raise ImportError(
- 'Failed to import `AssignResult`, `MaxIoUAssigner` from '
- '`mmdet.core.bbox` or failed to import `TASK_UTILS` from '
- '`mmdet.registry`. The class `MaxIoUAssignerAVA` is '
- 'invalid. ')
+ # for each anchor, which gt best overlaps with it
+ # for each anchor, the max iou of all gts
+ max_overlaps, argmax_overlaps = overlaps.max(dim=0)
+ # for each gt, which anchor best overlaps with it
+ # for each gt, the max iou of all proposals
+ gt_max_overlaps, gt_argmax_overlaps = overlaps.max(dim=1)
+
+ # 2. assign negative: below
+ # the negative inds are set to be 0
+ if isinstance(self.neg_iou_thr, float):
+ assigned_gt_inds[(max_overlaps >= 0)
+ & (max_overlaps < self.neg_iou_thr)] = 0
+ elif isinstance(self.neg_iou_thr, tuple):
+ assert len(self.neg_iou_thr) == 2
+ assigned_gt_inds[(max_overlaps >= self.neg_iou_thr[0])
+ & (max_overlaps < self.neg_iou_thr[1])] = 0
+
+ # 3. assign positive: above positive IoU threshold
+ pos_inds = max_overlaps >= self.pos_iou_thr
+ assigned_gt_inds[pos_inds] = argmax_overlaps[pos_inds] + 1
+
+ if self.match_low_quality:
+ # Low-quality matching will overwrite the assigned_gt_inds
+ # assigned in Step 3. Thus, the assigned gt might not be the
+ # best one for prediction.
+ # For example, if bbox A has 0.9 and 0.8 iou with GT bbox
+ # 1 & 2, bbox 1 will be assigned as the best target for bbox A
+ # in step 3. However, if GT bbox 2's gt_argmax_overlaps = A,
+ # bbox A's assigned_gt_inds will be overwritten to be bbox B.
+ # This might be the reason that it is not used in ROI Heads.
+ for i in range(num_gts):
+ if gt_max_overlaps[i] >= self.min_pos_iou:
+ if self.gt_max_assign_all:
+ max_iou_inds = overlaps[i, :] == gt_max_overlaps[i]
+ assigned_gt_inds[max_iou_inds] = i + 1
+ else:
+ assigned_gt_inds[gt_argmax_overlaps[i]] = i + 1
+
+ # consider multi-class case (AVA)
+ assert len(gt_labels[0]) > 1
+ assigned_labels = assigned_gt_inds.new_zeros(
+ (num_bboxes, len(gt_labels[0])), dtype=torch.float32)
+
+ # If not assigned, labels will be all 0
+ pos_inds = torch.nonzero(
+ assigned_gt_inds > 0, as_tuple=False).squeeze()
+ if pos_inds.numel() > 0:
+ assigned_labels[pos_inds] = gt_labels[assigned_gt_inds[pos_inds] -
+ 1]
+
+ return AssignResult(
+ num_gts=num_gts,
+ gt_inds=assigned_gt_inds,
+ max_overlaps=max_overlaps,
+ labels=assigned_labels)
diff --git a/mmaction/models/utils/blending_utils.py b/mmaction/models/utils/blending_utils.py
index 2d3732eeb1..855ca226b1 100644
--- a/mmaction/models/utils/blending_utils.py
+++ b/mmaction/models/utils/blending_utils.py
@@ -55,18 +55,18 @@ def __call__(self, imgs: torch.Tensor, batch_data_samples: SampleList,
shape of (B, N, C, H, W) or (B, N, C, T, H, W).
batch_data_samples (List[:obj:`ActionDataSample`]): The batch
data samples. It usually includes information such
- as `gt_labels`.
+ as `gt_label`.
Returns:
mixed_imgs (torch.Tensor): Blending images, float tensor with the
same shape of the input imgs.
batch_data_samples (List[:obj:`ActionDataSample`]): The modified
- batch data samples. ``gt_labels`` in each data sample are
+ batch data samples. ``gt_label`` in each data sample are
converted from a hard label to a blended soft label, float
tensor with the shape of (num_classes, ) and all elements are
in range [0, 1].
"""
- label = [x.gt_labels.item for x in batch_data_samples]
+ label = [x.gt_label for x in batch_data_samples]
# single-label classification
if label[0].size(0) == 1:
label = torch.tensor(label, dtype=torch.long).to(imgs.device)
@@ -79,7 +79,7 @@ def __call__(self, imgs: torch.Tensor, batch_data_samples: SampleList,
**kwargs)
for label_item, sample in zip(mixed_label, batch_data_samples):
- sample.gt_labels.item = label_item
+ sample.set_gt_label(label_item)
return mixed_imgs, batch_data_samples
diff --git a/mmaction/registry.py b/mmaction/registry.py
index 6d7d831db1..f214d514e5 100644
--- a/mmaction/registry.py
+++ b/mmaction/registry.py
@@ -54,7 +54,7 @@
DATA_SAMPLERS = Registry(
'data sampler',
parent=MMENGINE_DATA_SAMPLERS,
- locations=['mmaction.engine'])
+ locations=['mmaction.datasets'])
TRANSFORMS = Registry(
'transform',
parent=MMENGINE_TRANSFORMS,
@@ -132,3 +132,9 @@
# manage function
FUNCTION = Registry(
'function', parent=MMENGINE_FUNCTION, locations=['mmaction.mmengine'])
+
+# Tokenizer to encode sequence
+TOKENIZER = Registry(
+ 'tokenizer',
+ locations=['mmaction.models'],
+)
diff --git a/mmaction/structures/action_data_sample.py b/mmaction/structures/action_data_sample.py
index 6ea146cba2..79bec540a0 100644
--- a/mmaction/structures/action_data_sample.py
+++ b/mmaction/structures/action_data_sample.py
@@ -1,15 +1,16 @@
# Copyright (c) OpenMMLab. All rights reserved.
-from numbers import Number
-from typing import Sequence, Union
+from typing import Dict, Sequence, Union
import numpy as np
import torch
-from mmengine.structures import BaseDataElement, InstanceData, LabelData
+from mmengine.structures import BaseDataElement, InstanceData
from mmengine.utils import is_str
+LABEL_TYPE = Union[torch.Tensor, np.ndarray, Sequence, int]
+SCORE_TYPE = Union[torch.Tensor, np.ndarray, Sequence, Dict]
-def format_label(value: Union[torch.Tensor, np.ndarray, Sequence,
- int]) -> torch.Tensor:
+
+def format_label(value: LABEL_TYPE) -> torch.Tensor:
"""Convert various python types to label-format tensor.
Supported types are: :class:`numpy.ndarray`, :class:`torch.Tensor`,
@@ -19,7 +20,7 @@ def format_label(value: Union[torch.Tensor, np.ndarray, Sequence,
value (torch.Tensor | numpy.ndarray | Sequence | int): Label value.
Returns:
- :obj:`torch.Tensor`: The foramtted label tensor.
+ :obj:`torch.Tensor`: The formatted label tensor.
"""
# Handle single number
@@ -34,119 +35,62 @@ def format_label(value: Union[torch.Tensor, np.ndarray, Sequence,
value = torch.LongTensor([value])
elif not isinstance(value, torch.Tensor):
raise TypeError(f'Type {type(value)} is not an available label type.')
- assert value.ndim == 1, \
- f'The dims of value should be 1, but got {value.ndim}.'
return value
-def format_score(value: Union[torch.Tensor, np.ndarray,
- Sequence]) -> torch.Tensor:
+def format_score(value: SCORE_TYPE) -> Union[torch.Tensor, Dict]:
"""Convert various python types to score-format tensor.
Supported types are: :class:`numpy.ndarray`, :class:`torch.Tensor`,
:class:`Sequence`.
Args:
- value (torch.Tensor | numpy.ndarray | Sequence): Score values.
+ value (torch.Tensor | numpy.ndarray | Sequence | dict):
+ Score values or dict of scores values.
Returns:
- :obj:`torch.Tensor`: The foramtted score tensor.
+ :obj:`torch.Tensor` | dict: The formatted scores.
"""
if isinstance(value, np.ndarray):
value = torch.from_numpy(value).float()
elif isinstance(value, Sequence) and not is_str(value):
value = torch.tensor(value).float()
+ elif isinstance(value, dict):
+ for k, v in value.items():
+ value[k] = format_score(v)
elif not isinstance(value, torch.Tensor):
raise TypeError(f'Type {type(value)} is not an available label type.')
- assert value.ndim == 1, \
- f'The dims of value should be 1, but got {value.ndim}.'
return value
class ActionDataSample(BaseDataElement):
- def set_gt_labels(
- self, value: Union[np.ndarray, torch.Tensor, Sequence[Number], Number]
- ) -> 'ActionDataSample':
- """Set label of ``gt_labels``."""
- label_data = getattr(self, '_gt_label', LabelData())
- label_data.item = format_label(value)
- self.gt_labels = label_data
+ def set_gt_label(self, value: LABEL_TYPE) -> 'ActionDataSample':
+ """Set `gt_label``."""
+ self.set_field(format_label(value), 'gt_label', dtype=torch.Tensor)
return self
- def set_pred_label(
- self, value: Union[np.ndarray, torch.Tensor, Sequence[Number], Number]
- ) -> 'ActionDataSample':
- """Set label of ``pred_label``."""
- label_data = getattr(self, '_pred_label', LabelData())
- label_data.item = format_label(value)
- self.pred_labels = label_data
+ def set_pred_label(self, value: LABEL_TYPE) -> 'ActionDataSample':
+ """Set ``pred_label``."""
+ self.set_field(format_label(value), 'pred_label', dtype=torch.Tensor)
return self
- def set_pred_score(self, value: torch.Tensor) -> 'ActionDataSample':
+ def set_pred_score(self, value: SCORE_TYPE) -> 'ActionDataSample':
"""Set score of ``pred_label``."""
- label_data = getattr(self, '_pred_label', LabelData())
- label_data.item = format_score(value)
+ score = format_score(value)
+ self.set_field(score, 'pred_score')
if hasattr(self, 'num_classes'):
- assert len(label_data.item) == self.num_classes, \
- f'The length of score {len(label_data.item)} should be '\
+ assert len(score) == self.num_classes, \
+ f'The length of score {len(score)} should be '\
f'equal to the num_classes {self.num_classes}.'
else:
self.set_field(
- name='num_classes',
- value=len(label_data.item),
- field_type='metainfo')
- self.pred_scores = label_data
+ name='num_classes', value=len(score), field_type='metainfo')
return self
- @property
- def gt_labels(self):
- """Property of `gt_labels`"""
- return self._gt_labels
-
- @gt_labels.setter
- def gt_labels(self, value):
- """Setter of `gt_labels`"""
- self.set_field(value, '_gt_labels', LabelData)
-
- @gt_labels.deleter
- def gt_labels(self):
- """Deleter of `gt_labels`"""
- del self._gt_labels
-
- @property
- def pred_scores(self):
- """Property of `pred_scores`"""
- return self._pred_scores
-
- @pred_scores.setter
- def pred_scores(self, value):
- """Setter of `pred_scores`"""
- self.set_field(value, '_pred_scores', LabelData)
-
- @pred_scores.deleter
- def pred_scores(self):
- """Deleter of `pred_scores`"""
- del self._pred_scores
-
- @property
- def pred_labels(self):
- """Property of `pred_labels`"""
- return self._pred_labels
-
- @pred_labels.setter
- def pred_labels(self, value):
- """Setter of `pred_labels`"""
- self.set_field(value, '_pred_labels', LabelData)
-
- @pred_labels.deleter
- def pred_labels(self):
- """Deleter of `pred_labels`"""
- del self._pred_labels
-
@property
def proposals(self):
"""Property of `proposals`"""
diff --git a/mmaction/utils/__init__.py b/mmaction/utils/__init__.py
index af91d382c4..54e78dd2b6 100644
--- a/mmaction/utils/__init__.py
+++ b/mmaction/utils/__init__.py
@@ -3,17 +3,12 @@
from .gradcam_utils import GradCAM
from .misc import (VideoWriter, frame_extract, get_random_string, get_shm_dir,
get_str_type, get_thread_id)
+from .progress import track, track_on_main_process
from .setup_env import register_all_modules
from .typing_utils import * # noqa: F401,F403
__all__ = [
- 'collect_env',
- 'get_random_string',
- 'get_thread_id',
- 'get_shm_dir',
- 'frame_extract',
- 'GradCAM',
- 'register_all_modules',
- 'VideoWriter',
- 'get_str_type',
+ 'collect_env', 'get_random_string', 'get_thread_id', 'get_shm_dir',
+ 'frame_extract', 'GradCAM', 'register_all_modules', 'VideoWriter',
+ 'get_str_type', 'track', 'track_on_main_process'
]
diff --git a/mmaction/utils/dependency.py b/mmaction/utils/dependency.py
new file mode 100644
index 0000000000..dd8df115ec
--- /dev/null
+++ b/mmaction/utils/dependency.py
@@ -0,0 +1,81 @@
+# Copyright (c) OpenMMLab. All rights reserved.
+import re
+from functools import wraps
+from inspect import isfunction
+
+from importlib_metadata import PackageNotFoundError, distribution
+from mmengine.utils import digit_version
+
+
+def satisfy_requirement(dep):
+ pat = '(' + '|'.join(['>=', '==', '>']) + ')'
+ parts = re.split(pat, dep, maxsplit=1)
+ parts = [p.strip() for p in parts]
+ package = parts[0]
+ if len(parts) > 1:
+ op, version = parts[1:]
+ op = {
+ '>=': '__ge__',
+ '==': '__eq__',
+ '>': '__gt__',
+ '<': '__lt__',
+ '<=': '__le__'
+ }[op]
+ else:
+ op, version = None, None
+
+ try:
+ dist = distribution(package)
+ if op is None or getattr(digit_version(dist.version), op)(
+ digit_version(version)):
+ return True
+ except PackageNotFoundError:
+ pass
+
+ return False
+
+
+def require(dep, install=None):
+ """A wrapper of function for extra package requirements.
+
+ Args:
+ dep (str): The dependency package name, like ``transformers``
+ or ``transformers>=4.28.0``.
+ install (str, optional): The installation command hint. Defaults
+ to None, which means to use "pip install dep".
+ """
+
+ def wrapper(fn):
+ assert isfunction(fn)
+
+ @wraps(fn)
+ def ask_install(*args, **kwargs):
+ name = fn.__qualname__.replace('.__init__', '')
+ ins = install or f'pip install "{dep}"'
+ raise ImportError(
+ f'{name} requires {dep}, please install it by `{ins}`.')
+
+ if satisfy_requirement(dep):
+ fn._verify_require = getattr(fn, '_verify_require', lambda: None)
+ return fn
+
+ ask_install._verify_require = ask_install
+ return ask_install
+
+ return wrapper
+
+
+WITH_MULTIMODAL = all(
+ satisfy_requirement(item) for item in ['transformers>=4.28.0'])
+
+
+def register_multimodal_placeholder(names, registry):
+ for name in names:
+
+ def ask_install(*args, **kwargs):
+ raise ImportError(
+ f'{name} requires extra multi-modal dependencies, please '
+ 'install it by `pip install "mmaction2[multimodal]"` '
+ 'or `pip install -e ".[multimodal]"`.')
+
+ registry.register_module(name=name, module=ask_install)
diff --git a/mmaction/utils/gradcam_utils.py b/mmaction/utils/gradcam_utils.py
index 23f124f554..3d1a7f8f47 100644
--- a/mmaction/utils/gradcam_utils.py
+++ b/mmaction/utils/gradcam_utils.py
@@ -94,11 +94,11 @@ def _calculate_localization_map(self,
self.model.cls_head.average_clips = 'score'
# model forward & backward
results = self.model.test_step(data)
- preds = [result.pred_scores.item for result in results]
+ preds = [result.pred_score for result in results]
preds = torch.stack(preds)
if use_labels:
- labels = [result.gt_labels.item for result in results]
+ labels = [result.gt_label for result in results]
labels = torch.stack(labels)
score = torch.gather(preds, dim=1, index=labels)
else:
diff --git a/mmaction/utils/progress.py b/mmaction/utils/progress.py
new file mode 100644
index 0000000000..b23f976a42
--- /dev/null
+++ b/mmaction/utils/progress.py
@@ -0,0 +1,40 @@
+# Copyright (c) OpenMMLab. All rights reserved.
+from typing import Optional
+
+import mmengine.dist as dist
+import rich.progress as progress
+from rich.live import Live
+
+disable_progress_bar = False
+global_progress = progress.Progress(
+ '{task.description}',
+ progress.BarColumn(),
+ progress.TaskProgressColumn(show_speed=True),
+ progress.TimeRemainingColumn(),
+)
+global_live = Live(global_progress, refresh_per_second=10)
+
+
+def track(sequence, description: str = '', total: Optional[float] = None):
+ if disable_progress_bar:
+ yield from sequence
+ else:
+ global_live.start()
+ task_id = global_progress.add_task(description, total=total)
+ task = global_progress._tasks[task_id]
+ try:
+ yield from global_progress.track(sequence, task_id=task_id)
+ finally:
+ if task.total is None:
+ global_progress.update(task_id, total=task.completed)
+ if all(task.finished for task in global_progress.tasks):
+ global_live.stop()
+ for task_id in global_progress.task_ids:
+ global_progress.remove_task(task_id)
+
+
+def track_on_main_process(sequence, description='', total=None):
+ if not dist.is_main_process() or disable_progress_bar:
+ yield from sequence
+ else:
+ yield from track(sequence, total=total, description=description)
diff --git a/mmaction/version.py b/mmaction/version.py
index acae488d8a..94905dc04e 100644
--- a/mmaction/version.py
+++ b/mmaction/version.py
@@ -1,6 +1,6 @@
# Copyright (c) Open-MMLab. All rights reserved.
-__version__ = '1.1.0'
+__version__ = '1.2.0'
def parse_version_info(version_str: str):
diff --git a/mmaction/visualization/action_visualizer.py b/mmaction/visualization/action_visualizer.py
index 5924669c83..7a3bfab85e 100644
--- a/mmaction/visualization/action_visualizer.py
+++ b/mmaction/visualization/action_visualizer.py
@@ -63,7 +63,7 @@ class ActionVisualizer(Visualizer):
>>> video = video.get_batch(range(32)).asnumpy()
>>> # Example annotation
>>> data_sample = ActionDataSample()
- >>> data_sample.gt_labels = LabelData(item=torch.tensor([2]))
+ >>> data_sample.gt_label = LabelData(item=torch.tensor([2]))
>>> # Setup the visualizer
>>> vis = ActionVisualizer(
... save_dir="./outputs",
@@ -215,8 +215,8 @@ def add_datasample(self,
self.set_image(frame)
if draw_gt and 'gt_labels' in data_sample:
- gt_labels = data_sample.gt_labels
- idx = gt_labels.item.tolist()
+ gt_labels = data_sample.gt_label
+ idx = gt_labels.tolist()
class_labels = [''] * len(idx)
if classes is not None:
class_labels = [f' ({classes[i]})' for i in idx]
diff --git a/projects/actionclip/README.md b/projects/actionclip/README.md
index a16b44e249..ffe14a4cae 100644
--- a/projects/actionclip/README.md
+++ b/projects/actionclip/README.md
@@ -46,24 +46,45 @@ Create a symbolic link from `$MMACTION2/data` to `./data` in the current directo
ln -s ../../data ./data
```
+### Training commands
+
+**To train with single GPU:**
+
+```bash
+mim train mmaction configs/actionclip_vit-base-p32-res224-clip-pre_g8xb16_1x1x8_k400-rgb.py
+```
+
+**To train with multiple GPUs:**
+
+```bash
+mim train mmaction configs/actionclip_vit-base-p32-res224-clip-pre_g8xb16_1x1x8_k400-rgb.py --launcher pytorch --gpus 8
+```
+
+**To train with multiple GPUs by slurm:**
+
+```bash
+mim train mmaction configs/actionclip_vit-base-p32-res224-clip-pre_g8xb16_1x1x8_k400-rgb.py --launcher slurm \
+ --gpus 8 --gpus-per-node 8 --partition $PARTITION
+```
+
### Testing commands
**To test with single GPU:**
```bash
-mim test mmaction configs/actionclip_vit-base-p32-res224-clip-pre_1x1x8_k400-rgb.py --checkpoint $CHECKPOINT
+mim test mmaction configs/actionclip_vit-base-p32-res224-clip-pre_g8xb16_1x1x8_k400-rgb.py --checkpoint $CHECKPOINT
```
**To test with multiple GPUs:**
```bash
-mim test mmaction configs/actionclip_vit-base-p32-res224-clip-pre_1x1x8_k400-rgb.py --checkpoint $CHECKPOINT --launcher pytorch --gpus 8
+mim test mmaction configs/actionclip_vit-base-p32-res224-clip-pre_g8xb16_1x1x8_k400-rgb.py --checkpoint $CHECKPOINT --launcher pytorch --gpus 8
```
**To test with multiple GPUs by slurm:**
```bash
-mim test mmaction configs/actionclip_vit-base-p32-res224-clip-pre_1x1x8_k400-rgb.py --checkpoint $CHECKPOINT --launcher slurm \
+mim test mmaction configs/actionclip_vit-base-p32-res224-clip-pre_g8xb16_1x1x8_k400-rgb.py --checkpoint $CHECKPOINT --launcher slurm \
--gpus 8 --gpus-per-node 8 --partition $PARTITION
```
@@ -80,6 +101,13 @@ mim test mmaction configs/actionclip_vit-base-p32-res224-clip-pre_1x1x8_k400-rgb
\[1\] The models are ported from the repo [ActionCLIP](https://github.com/sallymmx/ActionCLIP) and tested on our data. Currently, we only support the testing of ActionCLIP models. Due to the variation in testing data, our reported test accuracy differs from that of the original repository (on average, it is lower by one point). Please refer to this [issue](https://github.com/sallymmx/ActionCLIP/issues/14) for more details.
+### Kinetics400 (Trained on Our K400 dataset)
+
+| frame sampling strategy | gpus | backbone | top1 acc | top5 acc | testing protocol | config | ckpt | log |
+| :---------------------: | :--: | :------: | :------: | :------: | :---------------: | :-------------------------------------------: | :------------------------------------------: | :-----------------------------------------: |
+| 1x1x8 | 8 | ViT-B/32 | 77.5 | 93.2 | 8 clips x 1 crop | [config](./configs/actionclip_vit-base-p32-res224-clip-pre_g8xb16_1x1x8_k400-rgb.py) | [ckpt](https://download.openmmlab.com/mmaction/v1.0/projects/actionclip/actionclip_vit-base-p32-res224-clip-pre_g8xb16_1x1x8_k400-rgb/actionclip_vit-base-p32-res224-clip-pre_g8xb16_1x1x8_k400-rgb_20230801-8535b794.pth) | [log](https://download.openmmlab.com/mmaction/v1.0/projects/actionclip/actionclip_vit-base-p32-res224-clip-pre_g8xb16_1x1x8_k400-rgb/actionclip_vit-base-p32-res224-clip-pre_g8xb16_1x1x8_k400-rgb.log) |
+| 1x1x8 | 8 | ViT-B/16 | 81.3 | 95.2 | 8 clips x 1 crop | [config](./configs/actionclip_vit-base-p16-res224-clip-pre_g8xb16_1x1x8_k400-rgb.py) | [ckpt](https://download.openmmlab.com/mmaction/v1.0/projects/actionclip/actionclip_vit-base-p16-res224-clip-pre_g8xb16_1x1x8_k400-rgb/actionclip_vit-base-p16-res224-clip-pre_g8xb16_1x1x8_k400-rgb_20230801-b307a0cd.pth) | [log](https://download.openmmlab.com/mmaction/v1.0/projects/actionclip/actionclip_vit-base-p16-res224-clip-pre_g8xb16_1x1x8_k400-rgb/actionclip_vit-base-p16-res224-clip-pre_g8xb16_1x1x8_k400-rgb.log) |
+
## Zero-Shot Prediction
We offer two methods for zero-shot prediction as follows. The `test.mp4` can be downloaded from [here](https://github-production-user-asset-6210df.s3.amazonaws.com/58767402/237333525-89ebee9a-573e-4e27-9047-0ad6422fa82f.mp4).
@@ -120,6 +148,7 @@ print("Label probs:", probs) # [[9.995e-01 5.364e-07 6.666e-04]]
```python
import mmengine
+import torch
from mmaction.utils import register_all_modules
from mmaction.apis import inference_recognizer, init_recognizer
@@ -139,7 +168,7 @@ device = "cuda" if torch.cuda.is_available() else "cpu"
model = init_recognizer(config=config, checkpoint=checkpoint_path, device=device)
pred_result = inference_recognizer(model, 'test.mp4')
-probs = pred_result.pred_scores.item.cpu().numpy()
+probs = pred_result.pred_score.cpu().numpy()
print("Label probs:", probs) # [9.995e-01 5.364e-07 6.666e-04]
```
diff --git a/projects/actionclip/configs/actionclip_vit-base-p16-res224-clip-pre_g8xb16_1x1x8_k400-rgb.py b/projects/actionclip/configs/actionclip_vit-base-p16-res224-clip-pre_g8xb16_1x1x8_k400-rgb.py
new file mode 100644
index 0000000000..732fd6fac0
--- /dev/null
+++ b/projects/actionclip/configs/actionclip_vit-base-p16-res224-clip-pre_g8xb16_1x1x8_k400-rgb.py
@@ -0,0 +1,162 @@
+custom_imports = dict(imports='models')
+
+num_segs = 8
+
+model = dict(
+ type='ActionClip',
+ clip_arch='ViT-B/16',
+ num_adapter_segs=num_segs,
+ num_adapter_layers=6,
+ to_float32=True,
+ labels_or_label_file='configs/label_map_k400.txt',
+ data_preprocessor=dict(
+ type='ActionDataPreprocessor',
+ mean=[122.771, 116.746, 104.093],
+ std=[68.500, 66.632, 70.323],
+ format_shape='NCHW'))
+
+dataset_type = 'VideoDataset'
+data_root = 'data/kinetics400/videos_train'
+data_root_val = 'data/kinetics400/videos_val'
+ann_file_train = 'data/kinetics400/kinetics400_train_list_videos.txt'
+ann_file_val = 'data/kinetics400/kinetics400_val_list_videos.txt'
+ann_file_test = 'data/kinetics400/kinetics400_val_list_videos.txt'
+
+file_client_args = dict(io_backend='disk')
+file_client_args = dict(
+ io_backend='petrel',
+ path_mapping=dict(
+ {'data/kinetics400/': 's3://openmmlab/datasets/action/Kinetics400/'}))
+
+train_pipeline = [
+ dict(type='DecordInit', **file_client_args),
+ dict(
+ type='SampleFrames', clip_len=1, frame_interval=1, num_clips=num_segs),
+ dict(type='DecordDecode'),
+ dict(type='Resize', scale=(-1, 256)),
+ dict(type='RandomResizedCrop'),
+ dict(
+ type='MultiScaleCrop',
+ input_size=224,
+ scales=(1, .875, .75, .66),
+ random_crop=False,
+ num_fixed_crops=13,
+ max_wh_scale_gap=1),
+ dict(type='Resize', scale=(224, 224), keep_ratio=False),
+ dict(type='Flip', flip_ratio=0.5),
+ dict(type='FormatShape', input_format='NCHW'),
+ dict(type='PackActionInputs')
+]
+
+val_pipeline = [
+ dict(type='DecordInit', **file_client_args),
+ dict(
+ type='SampleFrames',
+ clip_len=1,
+ frame_interval=1,
+ num_clips=num_segs,
+ test_mode=True),
+ dict(type='DecordDecode'),
+ dict(type='Resize', scale=(-1, 256)),
+ dict(type='CenterCrop', crop_size=224),
+ dict(type='FormatShape', input_format='NCHW'),
+ dict(type='PackActionInputs')
+]
+
+test_pipeline = val_pipeline
+
+train_dataloader = dict(
+ batch_size=16,
+ num_workers=16,
+ persistent_workers=True,
+ sampler=dict(type='DefaultSampler', shuffle=True),
+ dataset=dict(
+ type=dataset_type,
+ ann_file=ann_file_train,
+ data_prefix=dict(video=data_root),
+ pipeline=train_pipeline))
+val_dataloader = dict(
+ batch_size=16,
+ num_workers=16,
+ persistent_workers=True,
+ sampler=dict(type='DefaultSampler', shuffle=False),
+ dataset=dict(
+ type=dataset_type,
+ ann_file=ann_file_val,
+ data_prefix=dict(video=data_root_val),
+ pipeline=val_pipeline,
+ test_mode=True))
+test_dataloader = dict(
+ batch_size=1,
+ num_workers=16,
+ persistent_workers=True,
+ sampler=dict(type='DefaultSampler', shuffle=False),
+ dataset=dict(
+ type=dataset_type,
+ ann_file=ann_file_test,
+ data_prefix=dict(video=data_root_val),
+ pipeline=test_pipeline,
+ test_mode=True))
+
+val_evaluator = dict(type='AccMetric')
+test_evaluator = val_evaluator
+
+train_cfg = dict(
+ type='EpochBasedTrainLoop', max_epochs=50, val_begin=1, val_interval=1)
+val_cfg = dict(type='ValLoop')
+test_cfg = dict(type='TestLoop')
+
+optim_wrapper = dict(
+ optimizer=dict(
+ type='AdamW', lr=5e-6, betas=(0.9, 0.98), eps=1e-08, weight_decay=0.2),
+ paramwise_cfg=dict(custom_keys=dict(adapter=dict(lr_mult=10))))
+
+param_scheduler = [
+ dict(
+ type='LinearLR',
+ start_factor=0.01,
+ by_epoch=True,
+ begin=0,
+ end=5,
+ convert_to_iter_based=True),
+ dict(
+ type='CosineAnnealingLR',
+ T_max=45,
+ eta_min=0,
+ by_epoch=True,
+ begin=5,
+ end=50,
+ convert_to_iter_based=True)
+]
+
+# Default setting for scaling LR automatically
+# - `enable` means enable scaling LR automatically
+# or not by default.
+# - `base_batch_size` = (8 GPUs) x (16 samples per GPU).
+auto_scale_lr = dict(enable=False, base_batch_size=128)
+
+default_scope = 'mmaction'
+
+default_hooks = dict(
+ runtime_info=dict(type='RuntimeInfoHook'),
+ timer=dict(type='IterTimerHook'),
+ logger=dict(type='LoggerHook', interval=100, ignore_last=False),
+ param_scheduler=dict(type='ParamSchedulerHook'),
+ checkpoint=dict(
+ type='CheckpointHook', interval=1, save_best='auto', max_keep_ckpts=5),
+ sampler_seed=dict(type='DistSamplerSeedHook'),
+ sync_buffers=dict(type='SyncBuffersHook'))
+
+env_cfg = dict(
+ cudnn_benchmark=False,
+ mp_cfg=dict(mp_start_method='fork', opencv_num_threads=0),
+ dist_cfg=dict(backend='nccl'))
+
+log_processor = dict(type='LogProcessor', window_size=20, by_epoch=True)
+
+vis_backends = [dict(type='LocalVisBackend')]
+visualizer = dict(type='ActionVisualizer', vis_backends=vis_backends)
+
+log_level = 'INFO'
+load_from = None
+resume = False
diff --git a/projects/actionclip/configs/actionclip_vit-base-p32-res224-clip-pre_g8xb16_1x1x8_k400-rgb.py b/projects/actionclip/configs/actionclip_vit-base-p32-res224-clip-pre_g8xb16_1x1x8_k400-rgb.py
new file mode 100644
index 0000000000..0991730c71
--- /dev/null
+++ b/projects/actionclip/configs/actionclip_vit-base-p32-res224-clip-pre_g8xb16_1x1x8_k400-rgb.py
@@ -0,0 +1,162 @@
+custom_imports = dict(imports='models')
+
+num_segs = 8
+
+model = dict(
+ type='ActionClip',
+ clip_arch='ViT-B/32',
+ num_adapter_segs=num_segs,
+ num_adapter_layers=6,
+ to_float32=True,
+ labels_or_label_file='configs/label_map_k400.txt',
+ data_preprocessor=dict(
+ type='ActionDataPreprocessor',
+ mean=[122.771, 116.746, 104.093],
+ std=[68.500, 66.632, 70.323],
+ format_shape='NCHW'))
+
+dataset_type = 'VideoDataset'
+data_root = 'data/kinetics400/videos_train'
+data_root_val = 'data/kinetics400/videos_val'
+ann_file_train = 'data/kinetics400/kinetics400_train_list_videos.txt'
+ann_file_val = 'data/kinetics400/kinetics400_val_list_videos.txt'
+ann_file_test = 'data/kinetics400/kinetics400_val_list_videos.txt'
+
+file_client_args = dict(io_backend='disk')
+file_client_args = dict(
+ io_backend='petrel',
+ path_mapping=dict(
+ {'data/kinetics400/': 's3://openmmlab/datasets/action/Kinetics400/'}))
+
+train_pipeline = [
+ dict(type='DecordInit', **file_client_args),
+ dict(
+ type='SampleFrames', clip_len=1, frame_interval=1, num_clips=num_segs),
+ dict(type='DecordDecode'),
+ dict(type='Resize', scale=(-1, 256)),
+ dict(type='RandomResizedCrop'),
+ dict(
+ type='MultiScaleCrop',
+ input_size=224,
+ scales=(1, .875, .75, .66),
+ random_crop=False,
+ num_fixed_crops=13,
+ max_wh_scale_gap=1),
+ dict(type='Resize', scale=(224, 224), keep_ratio=False),
+ dict(type='Flip', flip_ratio=0.5),
+ dict(type='FormatShape', input_format='NCHW'),
+ dict(type='PackActionInputs')
+]
+
+val_pipeline = [
+ dict(type='DecordInit', **file_client_args),
+ dict(
+ type='SampleFrames',
+ clip_len=1,
+ frame_interval=1,
+ num_clips=num_segs,
+ test_mode=True),
+ dict(type='DecordDecode'),
+ dict(type='Resize', scale=(-1, 256)),
+ dict(type='CenterCrop', crop_size=224),
+ dict(type='FormatShape', input_format='NCHW'),
+ dict(type='PackActionInputs')
+]
+
+test_pipeline = val_pipeline
+
+train_dataloader = dict(
+ batch_size=16,
+ num_workers=16,
+ persistent_workers=True,
+ sampler=dict(type='DefaultSampler', shuffle=True),
+ dataset=dict(
+ type=dataset_type,
+ ann_file=ann_file_train,
+ data_prefix=dict(video=data_root),
+ pipeline=train_pipeline))
+val_dataloader = dict(
+ batch_size=16,
+ num_workers=16,
+ persistent_workers=True,
+ sampler=dict(type='DefaultSampler', shuffle=False),
+ dataset=dict(
+ type=dataset_type,
+ ann_file=ann_file_val,
+ data_prefix=dict(video=data_root_val),
+ pipeline=val_pipeline,
+ test_mode=True))
+test_dataloader = dict(
+ batch_size=1,
+ num_workers=16,
+ persistent_workers=True,
+ sampler=dict(type='DefaultSampler', shuffle=False),
+ dataset=dict(
+ type=dataset_type,
+ ann_file=ann_file_test,
+ data_prefix=dict(video=data_root_val),
+ pipeline=test_pipeline,
+ test_mode=True))
+
+val_evaluator = dict(type='AccMetric')
+test_evaluator = val_evaluator
+
+train_cfg = dict(
+ type='EpochBasedTrainLoop', max_epochs=50, val_begin=1, val_interval=1)
+val_cfg = dict(type='ValLoop')
+test_cfg = dict(type='TestLoop')
+
+optim_wrapper = dict(
+ optimizer=dict(
+ type='AdamW', lr=5e-6, betas=(0.9, 0.98), eps=1e-08, weight_decay=0.2),
+ paramwise_cfg=dict(custom_keys=dict(adapter=dict(lr_mult=10))))
+
+param_scheduler = [
+ dict(
+ type='LinearLR',
+ start_factor=0.01,
+ by_epoch=True,
+ begin=0,
+ end=5,
+ convert_to_iter_based=True),
+ dict(
+ type='CosineAnnealingLR',
+ T_max=45,
+ eta_min=0,
+ by_epoch=True,
+ begin=5,
+ end=50,
+ convert_to_iter_based=True)
+]
+
+# Default setting for scaling LR automatically
+# - `enable` means enable scaling LR automatically
+# or not by default.
+# - `base_batch_size` = (8 GPUs) x (16 samples per GPU).
+auto_scale_lr = dict(enable=False, base_batch_size=128)
+
+default_scope = 'mmaction'
+
+default_hooks = dict(
+ runtime_info=dict(type='RuntimeInfoHook'),
+ timer=dict(type='IterTimerHook'),
+ logger=dict(type='LoggerHook', interval=100, ignore_last=False),
+ param_scheduler=dict(type='ParamSchedulerHook'),
+ checkpoint=dict(
+ type='CheckpointHook', interval=1, save_best='auto', max_keep_ckpts=5),
+ sampler_seed=dict(type='DistSamplerSeedHook'),
+ sync_buffers=dict(type='SyncBuffersHook'))
+
+env_cfg = dict(
+ cudnn_benchmark=False,
+ mp_cfg=dict(mp_start_method='fork', opencv_num_threads=0),
+ dist_cfg=dict(backend='nccl'))
+
+log_processor = dict(type='LogProcessor', window_size=20, by_epoch=True)
+
+vis_backends = [dict(type='LocalVisBackend')]
+visualizer = dict(type='ActionVisualizer', vis_backends=vis_backends)
+
+log_level = 'INFO'
+load_from = None
+resume = False
diff --git a/projects/actionclip/models/actionclip.py b/projects/actionclip/models/actionclip.py
index 923b78c68f..6b125b40b2 100644
--- a/projects/actionclip/models/actionclip.py
+++ b/projects/actionclip/models/actionclip.py
@@ -1,9 +1,11 @@
-from typing import Dict, List, Optional, Union
+from typing import Any, Dict, List, Optional, Tuple, Union
import clip
import mmengine
+import numpy as np
import torch
import torch.nn.functional as F
+from mmengine.dist import all_gather, get_rank
from mmengine.model import BaseModel
from mmengine.structures import LabelData
@@ -11,7 +13,23 @@
from .adapter import TransformerAdapter
-def text_prompt(labels_or_label_file, template=None):
+class GatherLayer(torch.autograd.Function):
+
+ @staticmethod
+ def forward(ctx: Any, input: torch.Tensor) -> Tuple[List]:
+ ctx.save_for_backward(input)
+ output = all_gather(input)
+ return tuple(output)
+
+ @staticmethod
+ def backward(ctx: Any, *grads: torch.Tensor) -> torch.Tensor:
+ input, = ctx.saved_tensors
+ grad_out = torch.zeros_like(input)
+ grad_out[:] = grads[get_rank()]
+ return grad_out
+
+
+def text_prompt(labels_or_label_file, templates_or_template_file=None):
if isinstance(labels_or_label_file, str):
labels = mmengine.list_from_file(labels_or_label_file)
elif isinstance(labels_or_label_file, list):
@@ -20,8 +38,8 @@ def text_prompt(labels_or_label_file, template=None):
raise ValueError(f'`labels_or_label_file` must be `list` or `str`, '
f'but got {type(labels_or_label_file)}')
- if template is None:
- template = [
+ if templates_or_template_file is None:
+ templates = [
'a photo of action {}', 'a picture of action {}',
'Human action of {}', '{}, an action', '{} this is an action',
'{}, a video of action', 'Playing action of {}', '{}',
@@ -30,15 +48,15 @@ def text_prompt(labels_or_label_file, template=None):
'Video classification of {}', 'A video of {}', 'The man is {}',
'The woman is {}'
]
- elif isinstance(template, str):
- template = [template]
- elif not mmengine.is_seq_of(template, str):
+ elif isinstance(templates_or_template_file, str):
+ templates = mmengine.list_from_file(templates_or_template_file)
+ elif not mmengine.is_seq_of(templates_or_template_file, str):
raise ValueError(f'`template` must be list of `str`, `str` or `None`, '
- f'but got {type(template)}')
+ f'but got {type(templates_or_template_file)}')
- num_prompt = len(template)
+ num_prompt = len(templates)
prompt = torch.cat(
- [clip.tokenize(t.format(c)) for t in template for c in labels])
+ [clip.tokenize(t.format(c)) for t in templates for c in labels])
return prompt, num_prompt
@@ -49,18 +67,25 @@ def __init__(self,
clip_arch: str,
num_adapter_segs: int,
num_adapter_layers: int = 6,
+ to_float32: bool = False,
labels_or_label_file: Optional[Union[List[str], str]] = None,
- template: Optional[Union[List[str], str]] = None,
- data_preprocessor: Optional[Dict] = None):
+ templates_or_template_file: Optional[Union[List[str],
+ str]] = None,
+ data_preprocessor: Optional[Dict] = None,
+ loss: Dict = dict(type='CrossEntropyLoss', loss_weight=0.5)):
super(ActionClip, self).__init__(data_preprocessor=data_preprocessor)
- self.clip = clip.load(clip_arch)[0]
+ self.clip = clip.load(clip_arch, device='cpu')[0]
+ if to_float32:
+ self.clip.float()
+
self.adapter = TransformerAdapter(self.clip, num_adapter_segs,
num_adapter_layers)
+ self.loss = MODELS.build(loss)
+
if labels_or_label_file is not None:
- self.prompt, self.num_prompt = text_prompt(labels_or_label_file,
- template)
- self.text_features = None
+ self.prompt, self.num_prompt = text_prompt(
+ labels_or_label_file, templates_or_template_file)
def encode_video(self, video):
b, n, c, h, w = video.shape
@@ -95,14 +120,13 @@ def forward(self,
bsz = len(data_samples)
num_views = video_features.shape[0] // bsz
- if self.text_features is None:
- text_features = self.encode_text(self.prompt.to(inputs.device))
- self.text_features = text_features / text_features.norm(
- dim=-1, keepdim=True)
+ text_features = self.encode_text(self.prompt.to(inputs.device))
+ text_features = text_features / text_features.norm(
+ dim=-1, keepdim=True)
# (bsz*num_views, num_prompt, num_classes) ->
# (bsz, num_views*num_prompt, num_classes)
- similarity = (100.0 * video_features @ self.text_features.T). \
+ similarity = (100.0 * video_features @ text_features.T). \
view(bsz, num_views * self.num_prompt, -1)
cls_scores = F.softmax(similarity, dim=2).mean(dim=1)
@@ -112,6 +136,41 @@ def forward(self,
return data_samples
+ elif mode == 'loss':
+ video_features = self.encode_video(inputs)
+ video_features = video_features / video_features.norm(
+ dim=-1, keepdim=True)
+
+ text_id = np.random.randint(
+ self.num_prompt, size=len(data_samples))
+ real_labels = [x.gt_labels.item.item() for x in data_samples]
+ selected_prompt = self.prompt.view(
+ self.num_prompt, -1,
+ self.prompt.shape[-1])[text_id, real_labels].to(inputs.device)
+
+ text_features = self.encode_text(selected_prompt)
+ text_features = text_features / text_features.norm(
+ dim=-1, keepdim=True)
+
+ video_features = torch.cat(
+ GatherLayer.apply(video_features), dim=0)
+ text_features = torch.cat(GatherLayer.apply(text_features), dim=0)
+
+ logit_scale = self.clip.logit_scale.exp()
+ logits_per_video = logit_scale * video_features @ text_features.t()
+ logits_per_text = logits_per_video.t()
+ labels = torch.arange(logits_per_video.shape[0]).to(
+ logit_scale.device)
+
+ sim_loss_v2t = self.loss(logits_per_video, labels)
+ sim_loss_t2v = self.loss(logits_per_text, labels)
+
+ losses = dict()
+ losses['sim_loss_v2t'] = sim_loss_v2t
+ losses['sim_loss_t2v'] = sim_loss_t2v
+ return losses
+
else:
- raise RuntimeError(f'Invalid mode "{mode}". '
- 'Only supports `predict` and `tensor` mode. ')
+ raise RuntimeError(
+ f'Invalid mode "{mode}". '
+ 'Only supports `predict`, `loss` and `tensor` mode. ')
diff --git a/projects/umt/README.md b/projects/umt/README.md
new file mode 100644
index 0000000000..1d2db487fd
--- /dev/null
+++ b/projects/umt/README.md
@@ -0,0 +1,93 @@
+# UMT Project
+
+[Unmasked Teacher: Towards Training-Efficient Video Foundation Models](https://arxiv.org/abs/2303.16058)
+
+
+
+## Abstract
+
+
+
+Video Foundation Models (VFMs) have received limited exploration due to high computational costs and data scarcity. Previous VFMs rely on Image Foundation Models (IFMs), which face challenges in transferring to the video domain. Although VideoMAE has trained a robust ViT from limited data, its low-level reconstruction poses convergence difficulties and conflicts with high-level cross-modal alignment. This paper proposes a training-efficient method for temporal-sensitive VFMs that integrates the benefits of existing methods. To increase data efficiency, we mask out most of the low-semantics video tokens, but selectively align the unmasked tokens with IFM, which serves as the UnMasked Teacher (UMT). By providing semantic guidance, our method enables faster convergence and multimodal friendliness. With a progressive pre-training framework, our model can handle various tasks including scene-related, temporal-related, and complex video-language understanding. Using only public sources for pre-training in 6 days on 32 A100 GPUs, our scratch-built ViT-L/16 achieves state-of-the-art performances on various video tasks.
+
+
+
+
+
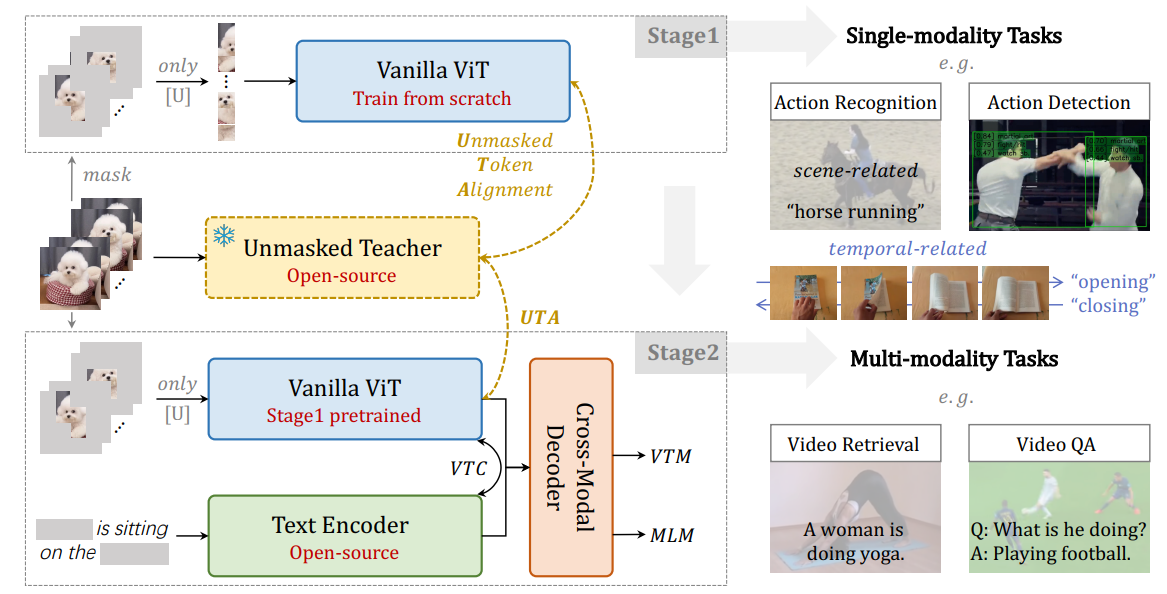
+
+
+## Usage
+
+### Setup Environment
+
+Please refer to [Installation](https://mmaction2.readthedocs.io/en/latest/get_started/installation.html) to install MMAction2.
+
+Assume that you are located at `$MMACTION2/projects/umt`.
+
+Add the current folder to `PYTHONPATH`, so that Python can find your code. Run the following command in the current directory to add it.
+
+> Please run it every time after you opened a new shell.
+
+```shell
+export PYTHONPATH=`pwd`:$PYTHONPATH
+```
+
+### Data Preparation
+
+Prepare the Kinetics dataset according to the [instruction](https://github.com/open-mmlab/mmaction2/tree/main/tools/data/kinetics#readme).
+
+Create a symbolic link from `$MMACTION2/data` to `./data` in the current directory, so that Python can locate your data. Run the following command in the current directory to create the symbolic link.
+
+```shell
+ln -s ../../data ./data
+```
+
+### Testing commands
+
+**To test with single GPU:**
+
+```bash
+mim test mmaction configs/umt-base-p16-res224_kinetics710-pre-ft_u8_k400-rgb.py --checkpoint $CHECKPOINT
+```
+
+**To test with multiple GPUs:**
+
+```bash
+mim test mmaction configs/umt-base-p16-res224_kinetics710-pre-ft_u8_k400-rgb.py --checkpoint $CHECKPOINT --launcher pytorch --gpus 8
+```
+
+**To test with multiple GPUs by slurm:**
+
+```bash
+mim test mmaction configs/umt-base-p16-res224_kinetics710-pre-ft_u8_k400-rgb.py --checkpoint $CHECKPOINT --launcher slurm \
+ --gpus 8 --gpus-per-node 8 --partition $PARTITION
+```
+
+## Results
+
+### Kinetics400
+
+| frame sampling strategy | resolution | backbone | pretrain | top1 acc | testing protocol | config | ckpt |
+| :---------------------: | :--------: | :------: | :---------: | :------: | :--------------: | :-------------------------------------------------------------: | :-----------------------------------------------------------: |
+| uniform 8 | 224x224 | UMT-B | Kinetics710 | 87.33 | 4 clips x 3 crop | [config](./configs/umt-base-p16-res224_kinetics710-pre-ft_u8_k400-rgb.py) | [ckpt](https://download.openmmlab.com/mmaction/v1.0/projects/umt/umt-base-p16-res224_kinetics710-pre-ft_u8_k400-rgb/umt-base-p16-res224_kinetics710-pre-ft_u8_k400-rgb.pth) |
+| uniform 8 | 224x224 | UMT-L | Kinetics710 | 90.21 | 4 clips x 3 crop | [config](./configs/umt-large-p16-res224_kinetics710-pre-ft_u8_k400-rgb.py) | [ckpt](https://download.openmmlab.com/mmaction/v1.0/projects/umt/umt-large-p16-res224_kinetics710-pre-ft_u8_k400-rgb/umt-large-p16-res224_kinetics710-pre-ft_u8_k400-rgb.pth) |
+
+### Kinetics700
+
+| frame sampling strategy | resolution | backbone | pretrain | top1 acc | testing protocol | config | ckpt |
+| :---------------------: | :--------: | :------: | :---------: | :------: | :--------------: | :-------------------------------------------------------------: | :-----------------------------------------------------------: |
+| uniform 8 | 224x224 | UMT-B | Kinetics710 | 77.95 | 4 clips x 3 crop | [config](./configs/umt-base-p16-res224_kinetics710-pre-ft_u8_k700-rgb.py) | [ckpt](https://download.openmmlab.com/mmaction/v1.0/projects/umt/umt-base-p16-res224_kinetics710-pre-ft_u8_k700-rgb/umt-base-p16-res224_kinetics710-pre-ft_u8_k700-rgb.pth) |
+| uniform 8 | 224x224 | UMT-L | Kinetics710 | 82.79 | 4 clips x 3 crop | [config](./configs/umt-large-p16-res224_kinetics710-pre-ft_u8_k700-rgb.py) | [ckpt](https://download.openmmlab.com/mmaction/v1.0/projects/umt/umt-large-p16-res224_kinetics710-pre-ft_u8_k700-rgb/umt-large-p16-res224_kinetics710-pre-ft_u8_k700-rgb.pth) |
+
+## Citation
+
+
+
+```bibtex
+@article{li2023unmasked,
+ title={Unmasked teacher: Towards training-efficient video foundation models},
+ author={Li, Kunchang and Wang, Yali and Li, Yizhuo and Wang, Yi and He, Yinan and Wang, Limin and Qiao, Yu},
+ journal={arXiv preprint arXiv:2303.16058},
+ year={2023}
+}
+```
diff --git a/projects/umt/configs/umt-base-p16-res224_kinetics710-pre-ft_u8_k400-rgb.py b/projects/umt/configs/umt-base-p16-res224_kinetics710-pre-ft_u8_k400-rgb.py
new file mode 100644
index 0000000000..e4077abcb4
--- /dev/null
+++ b/projects/umt/configs/umt-base-p16-res224_kinetics710-pre-ft_u8_k400-rgb.py
@@ -0,0 +1,82 @@
+custom_imports = dict(imports='models')
+
+# model settings
+model = dict(
+ type='Recognizer3D',
+ backbone=dict(
+ type='UMTViT',
+ patch_size=16,
+ embed_dim=768,
+ depth=12,
+ num_heads=12,
+ mlp_ratio=4,
+ all_frames=8,
+ qkv_bias=True),
+ cls_head=dict(
+ type='TimeSformerHead',
+ num_classes=400,
+ in_channels=768,
+ average_clips='prob'),
+ data_preprocessor=dict(
+ type='ActionDataPreprocessor',
+ mean=[114.75, 114.75, 114.75],
+ std=[57.375, 57.375, 57.375],
+ format_shape='NCTHW'))
+
+# dataset settings
+dataset_type = 'VideoDataset'
+data_root_val = 'data/kinetics400/videos_val'
+ann_file_test = 'data/kinetics400/kinetics400_val_list_videos.txt'
+
+file_client_args = dict(io_backend='disk')
+
+test_pipeline = [
+ dict(type='DecordInit', **file_client_args),
+ dict(type='UniformSample', clip_len=8, num_clips=4, test_mode=True),
+ dict(type='DecordDecode'),
+ dict(type='Resize', scale=(-1, 224)),
+ dict(type='ThreeCrop', crop_size=224),
+ dict(type='FormatShape', input_format='NCTHW'),
+ dict(type='PackActionInputs')
+]
+
+test_dataloader = dict(
+ batch_size=8,
+ num_workers=16,
+ persistent_workers=True,
+ sampler=dict(type='DefaultSampler', shuffle=False),
+ dataset=dict(
+ type=dataset_type,
+ ann_file=ann_file_test,
+ data_prefix=dict(video=data_root_val),
+ pipeline=test_pipeline,
+ test_mode=True))
+
+test_evaluator = dict(type='AccMetric')
+test_cfg = dict(type='TestLoop')
+
+default_scope = 'mmaction'
+
+default_hooks = dict(
+ runtime_info=dict(type='RuntimeInfoHook'),
+ timer=dict(type='IterTimerHook'),
+ logger=dict(type='LoggerHook', interval=20, ignore_last=False),
+ param_scheduler=dict(type='ParamSchedulerHook'),
+ checkpoint=dict(
+ type='CheckpointHook', interval=1, save_best='auto', max_keep_ckpts=5),
+ sampler_seed=dict(type='DistSamplerSeedHook'),
+ sync_buffers=dict(type='SyncBuffersHook'))
+
+env_cfg = dict(
+ cudnn_benchmark=False,
+ mp_cfg=dict(mp_start_method='fork', opencv_num_threads=0),
+ dist_cfg=dict(backend='nccl'))
+
+log_processor = dict(type='LogProcessor', window_size=20, by_epoch=True)
+
+vis_backends = [dict(type='LocalVisBackend')]
+visualizer = dict(type='ActionVisualizer', vis_backends=vis_backends)
+
+log_level = 'INFO'
+load_from = None
+resume = False
diff --git a/projects/umt/configs/umt-base-p16-res224_kinetics710-pre-ft_u8_k700-rgb.py b/projects/umt/configs/umt-base-p16-res224_kinetics710-pre-ft_u8_k700-rgb.py
new file mode 100644
index 0000000000..29bf3f002d
--- /dev/null
+++ b/projects/umt/configs/umt-base-p16-res224_kinetics710-pre-ft_u8_k700-rgb.py
@@ -0,0 +1,82 @@
+custom_imports = dict(imports='models')
+
+# model settings
+model = dict(
+ type='Recognizer3D',
+ backbone=dict(
+ type='UMTViT',
+ patch_size=16,
+ embed_dim=768,
+ depth=12,
+ num_heads=12,
+ mlp_ratio=4,
+ all_frames=8,
+ qkv_bias=True),
+ cls_head=dict(
+ type='TimeSformerHead',
+ num_classes=700,
+ in_channels=768,
+ average_clips='prob'),
+ data_preprocessor=dict(
+ type='ActionDataPreprocessor',
+ mean=[114.75, 114.75, 114.75],
+ std=[57.375, 57.375, 57.375],
+ format_shape='NCTHW'))
+
+# dataset settings
+dataset_type = 'VideoDataset'
+data_root_val = 'data/kinetics700/videos_val'
+ann_file_test = 'data/kinetics700/kinetics700_val_list_videos.txt'
+
+file_client_args = dict(io_backend='disk')
+
+test_pipeline = [
+ dict(type='DecordInit', **file_client_args),
+ dict(type='UniformSample', clip_len=8, num_clips=4, test_mode=True),
+ dict(type='DecordDecode'),
+ dict(type='Resize', scale=(-1, 224)),
+ dict(type='ThreeCrop', crop_size=224),
+ dict(type='FormatShape', input_format='NCTHW'),
+ dict(type='PackActionInputs')
+]
+
+test_dataloader = dict(
+ batch_size=8,
+ num_workers=16,
+ persistent_workers=True,
+ sampler=dict(type='DefaultSampler', shuffle=False),
+ dataset=dict(
+ type=dataset_type,
+ ann_file=ann_file_test,
+ data_prefix=dict(video=data_root_val),
+ pipeline=test_pipeline,
+ test_mode=True))
+
+test_evaluator = dict(type='AccMetric')
+test_cfg = dict(type='TestLoop')
+
+default_scope = 'mmaction'
+
+default_hooks = dict(
+ runtime_info=dict(type='RuntimeInfoHook'),
+ timer=dict(type='IterTimerHook'),
+ logger=dict(type='LoggerHook', interval=20, ignore_last=False),
+ param_scheduler=dict(type='ParamSchedulerHook'),
+ checkpoint=dict(
+ type='CheckpointHook', interval=1, save_best='auto', max_keep_ckpts=5),
+ sampler_seed=dict(type='DistSamplerSeedHook'),
+ sync_buffers=dict(type='SyncBuffersHook'))
+
+env_cfg = dict(
+ cudnn_benchmark=False,
+ mp_cfg=dict(mp_start_method='fork', opencv_num_threads=0),
+ dist_cfg=dict(backend='nccl'))
+
+log_processor = dict(type='LogProcessor', window_size=20, by_epoch=True)
+
+vis_backends = [dict(type='LocalVisBackend')]
+visualizer = dict(type='ActionVisualizer', vis_backends=vis_backends)
+
+log_level = 'INFO'
+load_from = None
+resume = False
diff --git a/projects/umt/configs/umt-large-p16-res224_kinetics710-pre-ft_u8_k400-rgb.py b/projects/umt/configs/umt-large-p16-res224_kinetics710-pre-ft_u8_k400-rgb.py
new file mode 100644
index 0000000000..e243744e8a
--- /dev/null
+++ b/projects/umt/configs/umt-large-p16-res224_kinetics710-pre-ft_u8_k400-rgb.py
@@ -0,0 +1,82 @@
+custom_imports = dict(imports='models')
+
+# model settings
+model = dict(
+ type='Recognizer3D',
+ backbone=dict(
+ type='UMTViT',
+ patch_size=16,
+ embed_dim=1024,
+ depth=24,
+ num_heads=16,
+ mlp_ratio=4,
+ all_frames=8,
+ qkv_bias=True),
+ cls_head=dict(
+ type='TimeSformerHead',
+ num_classes=400,
+ in_channels=1024,
+ average_clips='prob'),
+ data_preprocessor=dict(
+ type='ActionDataPreprocessor',
+ mean=[114.75, 114.75, 114.75],
+ std=[57.375, 57.375, 57.375],
+ format_shape='NCTHW'))
+
+# dataset settings
+dataset_type = 'VideoDataset'
+data_root_val = 'data/kinetics400/videos_val'
+ann_file_test = 'data/kinetics400/kinetics400_val_list_videos.txt'
+
+file_client_args = dict(io_backend='disk')
+
+test_pipeline = [
+ dict(type='DecordInit', **file_client_args),
+ dict(type='UniformSample', clip_len=8, num_clips=4, test_mode=True),
+ dict(type='DecordDecode'),
+ dict(type='Resize', scale=(-1, 224)),
+ dict(type='ThreeCrop', crop_size=224),
+ dict(type='FormatShape', input_format='NCTHW'),
+ dict(type='PackActionInputs')
+]
+
+test_dataloader = dict(
+ batch_size=8,
+ num_workers=16,
+ persistent_workers=True,
+ sampler=dict(type='DefaultSampler', shuffle=False),
+ dataset=dict(
+ type=dataset_type,
+ ann_file=ann_file_test,
+ data_prefix=dict(video=data_root_val),
+ pipeline=test_pipeline,
+ test_mode=True))
+
+test_evaluator = dict(type='AccMetric')
+test_cfg = dict(type='TestLoop')
+
+default_scope = 'mmaction'
+
+default_hooks = dict(
+ runtime_info=dict(type='RuntimeInfoHook'),
+ timer=dict(type='IterTimerHook'),
+ logger=dict(type='LoggerHook', interval=20, ignore_last=False),
+ param_scheduler=dict(type='ParamSchedulerHook'),
+ checkpoint=dict(
+ type='CheckpointHook', interval=1, save_best='auto', max_keep_ckpts=5),
+ sampler_seed=dict(type='DistSamplerSeedHook'),
+ sync_buffers=dict(type='SyncBuffersHook'))
+
+env_cfg = dict(
+ cudnn_benchmark=False,
+ mp_cfg=dict(mp_start_method='fork', opencv_num_threads=0),
+ dist_cfg=dict(backend='nccl'))
+
+log_processor = dict(type='LogProcessor', window_size=20, by_epoch=True)
+
+vis_backends = [dict(type='LocalVisBackend')]
+visualizer = dict(type='ActionVisualizer', vis_backends=vis_backends)
+
+log_level = 'INFO'
+load_from = None
+resume = False
diff --git a/projects/umt/configs/umt-large-p16-res224_kinetics710-pre-ft_u8_k700-rgb.py b/projects/umt/configs/umt-large-p16-res224_kinetics710-pre-ft_u8_k700-rgb.py
new file mode 100644
index 0000000000..e75747b946
--- /dev/null
+++ b/projects/umt/configs/umt-large-p16-res224_kinetics710-pre-ft_u8_k700-rgb.py
@@ -0,0 +1,82 @@
+custom_imports = dict(imports='models')
+
+# model settings
+model = dict(
+ type='Recognizer3D',
+ backbone=dict(
+ type='UMTViT',
+ patch_size=16,
+ embed_dim=1024,
+ depth=24,
+ num_heads=16,
+ mlp_ratio=4,
+ all_frames=8,
+ qkv_bias=True),
+ cls_head=dict(
+ type='TimeSformerHead',
+ num_classes=700,
+ in_channels=1024,
+ average_clips='prob'),
+ data_preprocessor=dict(
+ type='ActionDataPreprocessor',
+ mean=[114.75, 114.75, 114.75],
+ std=[57.375, 57.375, 57.375],
+ format_shape='NCTHW'))
+
+# dataset settings
+dataset_type = 'VideoDataset'
+data_root_val = 'data/kinetics700/videos_val'
+ann_file_test = 'data/kinetics700/kinetics700_val_list_videos.txt'
+
+file_client_args = dict(io_backend='disk')
+
+test_pipeline = [
+ dict(type='DecordInit', **file_client_args),
+ dict(type='UniformSample', clip_len=8, num_clips=4, test_mode=True),
+ dict(type='DecordDecode'),
+ dict(type='Resize', scale=(-1, 224)),
+ dict(type='ThreeCrop', crop_size=224),
+ dict(type='FormatShape', input_format='NCTHW'),
+ dict(type='PackActionInputs')
+]
+
+test_dataloader = dict(
+ batch_size=8,
+ num_workers=16,
+ persistent_workers=True,
+ sampler=dict(type='DefaultSampler', shuffle=False),
+ dataset=dict(
+ type=dataset_type,
+ ann_file=ann_file_test,
+ data_prefix=dict(video=data_root_val),
+ pipeline=test_pipeline,
+ test_mode=True))
+
+test_evaluator = dict(type='AccMetric')
+test_cfg = dict(type='TestLoop')
+
+default_scope = 'mmaction'
+
+default_hooks = dict(
+ runtime_info=dict(type='RuntimeInfoHook'),
+ timer=dict(type='IterTimerHook'),
+ logger=dict(type='LoggerHook', interval=20, ignore_last=False),
+ param_scheduler=dict(type='ParamSchedulerHook'),
+ checkpoint=dict(
+ type='CheckpointHook', interval=1, save_best='auto', max_keep_ckpts=5),
+ sampler_seed=dict(type='DistSamplerSeedHook'),
+ sync_buffers=dict(type='SyncBuffersHook'))
+
+env_cfg = dict(
+ cudnn_benchmark=False,
+ mp_cfg=dict(mp_start_method='fork', opencv_num_threads=0),
+ dist_cfg=dict(backend='nccl'))
+
+log_processor = dict(type='LogProcessor', window_size=20, by_epoch=True)
+
+vis_backends = [dict(type='LocalVisBackend')]
+visualizer = dict(type='ActionVisualizer', vis_backends=vis_backends)
+
+log_level = 'INFO'
+load_from = None
+resume = False
diff --git a/projects/umt/models/__init__.py b/projects/umt/models/__init__.py
new file mode 100644
index 0000000000..5c0c77a862
--- /dev/null
+++ b/projects/umt/models/__init__.py
@@ -0,0 +1,3 @@
+from .vit import UMTViT
+
+__all__ = ['UMTViT']
diff --git a/projects/umt/models/vit.py b/projects/umt/models/vit.py
new file mode 100644
index 0000000000..00cebb128e
--- /dev/null
+++ b/projects/umt/models/vit.py
@@ -0,0 +1,344 @@
+from functools import partial
+
+import numpy as np
+import torch
+import torch.nn as nn
+import torch.nn.functional as F
+import torch.utils.checkpoint as checkpoint
+from mmcv.cnn.bricks import DropPath
+from mmengine import to_2tuple
+
+from mmaction.registry import MODELS
+
+
+class Mlp(nn.Module):
+
+ def __init__(self,
+ in_features,
+ hidden_features=None,
+ out_features=None,
+ act_layer=nn.GELU,
+ drop=0.):
+ super().__init__()
+ out_features = out_features or in_features
+ hidden_features = hidden_features or in_features
+ self.fc1 = nn.Linear(in_features, hidden_features)
+ self.act = act_layer()
+ self.fc2 = nn.Linear(hidden_features, out_features)
+ self.drop = nn.Dropout(drop)
+
+ def forward(self, x):
+ x = self.fc1(x)
+ x = self.act(x)
+ # x = self.drop(x)
+ # commit this for the original BERT implement
+ x = self.fc2(x)
+ x = self.drop(x)
+ return x
+
+
+class Attention(nn.Module):
+
+ def __init__(self,
+ dim,
+ num_heads=8,
+ qkv_bias=False,
+ qk_scale=None,
+ attn_drop=0.,
+ proj_drop=0.,
+ attn_head_dim=None):
+ super().__init__()
+ self.num_heads = num_heads
+ head_dim = dim // num_heads
+ if attn_head_dim is not None:
+ head_dim = attn_head_dim
+ all_head_dim = head_dim * self.num_heads
+ self.scale = qk_scale or head_dim**-0.5
+
+ self.qkv = nn.Linear(dim, all_head_dim * 3, bias=False)
+ if qkv_bias:
+ self.q_bias = nn.Parameter(torch.zeros(all_head_dim))
+ self.v_bias = nn.Parameter(torch.zeros(all_head_dim))
+ else:
+ self.q_bias = None
+ self.v_bias = None
+
+ self.attn_drop = nn.Dropout(attn_drop)
+ self.proj = nn.Linear(all_head_dim, dim)
+ self.proj_drop = nn.Dropout(proj_drop)
+
+ def forward(self, x):
+ B, N, C = x.shape
+ qkv_bias = None
+ if self.q_bias is not None:
+ qkv_bias = torch.cat(
+ (self.q_bias,
+ torch.zeros_like(self.v_bias,
+ requires_grad=False), self.v_bias))
+ qkv = F.linear(input=x, weight=self.qkv.weight, bias=qkv_bias)
+ qkv = qkv.reshape(B, N, 3, self.num_heads, -1).permute(2, 0, 3, 1, 4)
+ q, k, v = qkv[0], qkv[1], qkv[2]
+
+ q = q * self.scale
+ attn = (q @ k.transpose(-2, -1))
+
+ attn = attn.softmax(dim=-1)
+ attn = self.attn_drop(attn)
+
+ x = (attn @ v).transpose(1, 2).reshape(B, N, -1)
+ x = self.proj(x)
+ x = self.proj_drop(x)
+ return x
+
+
+class Block(nn.Module):
+
+ def __init__(self,
+ dim,
+ num_heads,
+ mlp_ratio=4.,
+ qkv_bias=False,
+ qk_scale=None,
+ drop=0.,
+ attn_drop=0.,
+ drop_path=0.,
+ init_values=None,
+ act_layer=nn.GELU,
+ norm_layer=nn.LayerNorm,
+ attn_head_dim=None):
+ super().__init__()
+ self.norm1 = norm_layer(dim)
+ self.attn = Attention(
+ dim,
+ num_heads=num_heads,
+ qkv_bias=qkv_bias,
+ qk_scale=qk_scale,
+ attn_drop=attn_drop,
+ proj_drop=drop,
+ attn_head_dim=attn_head_dim)
+ self.drop_path = DropPath(
+ drop_path) if drop_path > 0. else nn.Identity()
+ self.norm2 = norm_layer(dim)
+ mlp_hidden_dim = int(dim * mlp_ratio)
+ self.mlp = Mlp(
+ in_features=dim,
+ hidden_features=mlp_hidden_dim,
+ act_layer=act_layer,
+ drop=drop)
+
+ if init_values > 0:
+ self.gamma_1 = nn.Parameter(
+ init_values * torch.ones((dim)), requires_grad=True)
+ self.gamma_2 = nn.Parameter(
+ init_values * torch.ones((dim)), requires_grad=True)
+ else:
+ self.gamma_1, self.gamma_2 = None, None
+
+ def forward(self, x):
+ if self.gamma_1 is None:
+ x = x + self.drop_path(self.attn(self.norm1(x)))
+ x = x + self.drop_path(self.mlp(self.norm2(x)))
+ else:
+ x = x + self.drop_path(self.gamma_1 * self.attn(self.norm1(x)))
+ x = x + self.drop_path(self.gamma_2 * self.mlp(self.norm2(x)))
+ return x
+
+
+class PatchEmbed(nn.Module):
+
+ def __init__(self,
+ img_size=224,
+ patch_size=16,
+ in_chans=3,
+ embed_dim=768,
+ num_frames=16,
+ tubelet_size=2):
+ super().__init__()
+ img_size = to_2tuple(img_size)
+ patch_size = to_2tuple(patch_size)
+ self.tubelet_size = int(tubelet_size)
+ num_patches = (img_size[1] //
+ patch_size[1]) * (img_size[0] // patch_size[0]) * (
+ num_frames // self.tubelet_size)
+ self.img_size = img_size
+ self.patch_size = patch_size
+ self.num_patches = num_patches
+ self.proj = nn.Conv3d(
+ in_channels=in_chans,
+ out_channels=embed_dim,
+ kernel_size=(self.tubelet_size, patch_size[0], patch_size[1]),
+ stride=(self.tubelet_size, patch_size[0], patch_size[1]))
+
+ def forward(self, x):
+ B, C, T, H, W = x.shape
+ assert H == self.img_size[0] and W == self.img_size[1], \
+ f"Input image size ({H}*{W}) doesn't match model " \
+ f'({self.img_size[0]}*{self.img_size[1]}).'
+ x = self.proj(x).flatten(2).transpose(1, 2)
+ return x
+
+
+# sin-cos position encoding
+def get_sinusoid_encoding_table(n_position,
+ d_hid,
+ cur_frame=-1,
+ pre_n_position=1568):
+ """Sinusoid position encoding table."""
+
+ def get_position_angle_vec(position):
+ return [
+ position / np.power(10000, 2 * (hid_j // 2) / d_hid)
+ for hid_j in range(d_hid)
+ ]
+
+ sinusoid_table = np.array(
+ [get_position_angle_vec(pos_i) for pos_i in range(pre_n_position)])
+ sinusoid_table[:, 0::2] = np.sin(sinusoid_table[:, 0::2]) # dim 2i
+ sinusoid_table[:, 1::2] = np.cos(sinusoid_table[:, 1::2]) # dim 2i+1
+ sinusoid_table = torch.tensor(
+ sinusoid_table, dtype=torch.float, requires_grad=False).unsqueeze(0)
+ print(f'n_position: {n_position}')
+ print(f'pre_n_position: {pre_n_position}')
+ if n_position // cur_frame * 8 != pre_n_position and cur_frame != -1:
+ T = 8 # checkpoint frame
+ P = 14 # checkpoint size
+ C = d_hid
+ new_P = int((n_position // cur_frame)**0.5) # testing size
+ print(
+ f'Pretraining uses 14x14, but current version is {new_P}x{new_P}')
+ print('Interpolate the position embedding')
+ sinusoid_table = sinusoid_table.reshape(-1, T, P, P, C)
+ sinusoid_table = sinusoid_table.reshape(-1, P, P,
+ C).permute(0, 3, 1, 2)
+ sinusoid_table = torch.nn.functional.interpolate(
+ sinusoid_table,
+ size=(new_P, new_P),
+ mode='bicubic',
+ align_corners=False)
+ # BT, C, H, W -> BT, H, W, C -> B, T, H, W, C
+ sinusoid_table = sinusoid_table.permute(0, 2, 3, 1).reshape(
+ -1, T, new_P, new_P, C)
+ sinusoid_table = sinusoid_table.flatten(1, 3)
+ if cur_frame != -1 and cur_frame != 8:
+ print(f'Pretraining uses 8 frames, but current frame is {cur_frame}')
+ print('Interpolate the position embedding')
+ T = 8 # checkpoint frame
+ new_T = cur_frame # testing frame
+ # interpolate
+ P = int((n_position // cur_frame)**0.5) # testing size
+ C = d_hid
+ sinusoid_table = sinusoid_table.reshape(-1, T, P, P, C)
+ sinusoid_table = sinusoid_table.permute(0, 2, 3, 4,
+ 1).reshape(-1, C,
+ T) # BHW, C, T
+ sinusoid_table = torch.nn.functional.interpolate(
+ sinusoid_table, size=new_T, mode='linear')
+ sinusoid_table = sinusoid_table.reshape(1, P, P, C, new_T).permute(
+ 0, 4, 1, 2, 3) # B, T, H, W, C
+ sinusoid_table = sinusoid_table.flatten(1, 3)
+ if n_position == pre_n_position:
+ return sinusoid_table
+ else:
+ print('Use learnable position embedding')
+ return nn.Parameter(sinusoid_table, requires_grad=True)
+
+
+@MODELS.register_module()
+class UMTViT(nn.Module):
+
+ def __init__(self,
+ img_size=224,
+ patch_size=16,
+ in_chans=3,
+ embed_dim=768,
+ depth=12,
+ num_heads=12,
+ mlp_ratio=4.,
+ qkv_bias=False,
+ qk_scale=None,
+ drop_rate=0.,
+ attn_drop_rate=0.,
+ drop_path_rate=0.,
+ norm_layer=partial(nn.LayerNorm, eps=1e-6),
+ init_values=0.,
+ use_learnable_pos_emb=False,
+ all_frames=16,
+ tubelet_size=1,
+ use_checkpoint=False,
+ checkpoint_num=0,
+ use_mean_pooling=True):
+ super().__init__()
+ self.num_features = self.embed_dim = embed_dim
+ self.tubelet_size = tubelet_size
+ self.patch_embed = PatchEmbed(
+ img_size=img_size,
+ patch_size=patch_size,
+ in_chans=in_chans,
+ embed_dim=embed_dim,
+ num_frames=all_frames,
+ tubelet_size=self.tubelet_size)
+ num_patches = self.patch_embed.num_patches
+ self.use_checkpoint = use_checkpoint
+ self.checkpoint_num = checkpoint_num
+ print(f'Use checkpoint: {use_checkpoint}')
+ print(f'Checkpoint number: {checkpoint_num}')
+
+ if use_learnable_pos_emb:
+ self.pos_embed = nn.Parameter(
+ torch.zeros(1, num_patches, embed_dim))
+ else:
+ # sine-cosine positional embeddings is on the way
+ if patch_size == 14:
+ pre_n_position = 2048
+ else:
+ pre_n_position = 1568
+ self.pos_embed = get_sinusoid_encoding_table(
+ num_patches,
+ embed_dim,
+ all_frames // tubelet_size,
+ pre_n_position=pre_n_position)
+
+ self.pos_drop = nn.Dropout(p=drop_rate)
+
+ dpr = [x.item() for x in torch.linspace(0, drop_path_rate, depth)]
+ self.blocks = nn.ModuleList([
+ Block(
+ dim=embed_dim,
+ num_heads=num_heads,
+ mlp_ratio=mlp_ratio,
+ qkv_bias=qkv_bias,
+ qk_scale=qk_scale,
+ drop=drop_rate,
+ attn_drop=attn_drop_rate,
+ drop_path=dpr[i],
+ norm_layer=norm_layer,
+ init_values=init_values) for i in range(depth)
+ ])
+ self.norm = nn.Identity() if use_mean_pooling else norm_layer(
+ embed_dim)
+ self.fc_norm = norm_layer(embed_dim) if use_mean_pooling else None
+
+ def forward_features(self, x):
+ x = self.patch_embed(x)
+ B, _, _ = x.size()
+
+ if self.pos_embed is not None:
+ x = x + self.pos_embed.expand(B, -1, -1).type_as(x).to(
+ x.device).clone().detach()
+ x = self.pos_drop(x)
+
+ for idx, blk in enumerate(self.blocks):
+ if self.use_checkpoint and idx < self.checkpoint_num:
+ x = checkpoint.checkpoint(blk, x)
+ else:
+ x = blk(x)
+
+ x = self.norm(x)
+ if self.fc_norm is not None:
+ return self.fc_norm(x.mean(1))
+ else:
+ return x[:, 0]
+
+ def forward(self, x):
+ x = self.forward_features(x)
+ return x
diff --git a/requirements/mminstall.txt b/requirements/mminstall.txt
index 8381c8c000..386fc55696 100644
--- a/requirements/mminstall.txt
+++ b/requirements/mminstall.txt
@@ -1,2 +1,2 @@
-mmcv>=2.0.0rc0,<2.1.0
-mmengine>=0.5.0,<1.0.0
+mmcv>=2.0.0rc4,<2.2.0
+mmengine>=0.7.1,<1.0.0
diff --git a/requirements/multimodal.txt b/requirements/multimodal.txt
new file mode 100644
index 0000000000..c3503a0875
--- /dev/null
+++ b/requirements/multimodal.txt
@@ -0,0 +1 @@
+transformers>=4.28.0
diff --git a/resources/miaomiao_qrcode.jpg b/resources/miaomiao_qrcode.jpg
new file mode 100644
index 0000000000..d34cbae6fd
Binary files /dev/null and b/resources/miaomiao_qrcode.jpg differ
diff --git a/setup.py b/setup.py
index 4776e54145..94471e5220 100644
--- a/setup.py
+++ b/setup.py
@@ -191,5 +191,6 @@ def add_mim_extension():
'tests': parse_requirements('requirements/tests.txt'),
'optional': parse_requirements('requirements/optional.txt'),
'mim': parse_requirements('requirements/mminstall.txt'),
+ 'multimodal': parse_requirements('requirements/multimodal.txt'),
},
zip_safe=False)
diff --git a/tests/apis/test_inference.py b/tests/apis/test_inference.py
index 1b004943f7..749c3af01b 100644
--- a/tests/apis/test_inference.py
+++ b/tests/apis/test_inference.py
@@ -66,7 +66,7 @@ def test_inference_recognizer(self, config, video_path, devices):
result = inference_recognizer(model, video_path)
self.assertIsInstance(result, ActionDataSample)
- self.assertTrue(result.pred_scores.item.shape, (400, ))
+ self.assertTrue(result.pred_score.shape, (400, ))
def test_detection_inference(self):
from mmdet.apis import init_detector
diff --git a/tests/data/annotations/sample.pkl b/tests/data/annotations/sample.pkl
index ee61c71252..63a3834b7a 100644
Binary files a/tests/data/annotations/sample.pkl and b/tests/data/annotations/sample.pkl differ
diff --git a/tests/datasets/test_pose_dataset.py b/tests/datasets/test_pose_dataset.py
index 83a19e0398..e97d0180a8 100644
--- a/tests/datasets/test_pose_dataset.py
+++ b/tests/datasets/test_pose_dataset.py
@@ -1,4 +1,7 @@
# Copyright (c) OpenMMLab. All rights reserved.
+import numpy as np
+import pytest
+
from mmaction.datasets import PoseDataset
from .base import BaseTestDataset
@@ -7,9 +10,39 @@ class TestPoseDataset(BaseTestDataset):
def test_pose_dataset(self):
ann_file = self.pose_ann_file
-
+ data_prefix = dict(video='root')
dataset = PoseDataset(
ann_file=ann_file,
pipeline=[],
- )
+ split='train',
+ box_thr=0.5,
+ data_prefix=data_prefix)
assert len(dataset) == 100
+ item = dataset[0]
+ assert item['frame_dir'].startswith(data_prefix['video'])
+
+ dataset = PoseDataset(
+ ann_file=ann_file,
+ pipeline=[],
+ split='train',
+ valid_ratio=0.2,
+ box_thr=0.9)
+ assert len(dataset) == 84
+ for item in dataset:
+ assert np.all(item['box_score'][item['anno_inds']] >= 0.9)
+ assert item['valid'][0.9] / item['total_frames'] >= 0.2
+
+ dataset = PoseDataset(
+ ann_file=ann_file,
+ pipeline=[],
+ split='train',
+ valid_ratio=0.3,
+ box_thr=0.7)
+ assert len(dataset) == 87
+ for item in dataset:
+ assert np.all(item['box_score'][item['anno_inds']] >= 0.7)
+ assert item['valid'][0.7] / item['total_frames'] >= 0.3
+
+ with pytest.raises(AssertionError):
+ dataset = PoseDataset(
+ ann_file=ann_file, pipeline=[], valid_ratio=0.2, box_thr=0.55)
diff --git a/tests/datasets/transforms/test_formating.py b/tests/datasets/transforms/test_formating.py
index 4668732746..93e32249b5 100644
--- a/tests/datasets/transforms/test_formating.py
+++ b/tests/datasets/transforms/test_formating.py
@@ -34,7 +34,7 @@ def test_transform(self):
self.assertIn('data_samples', results)
self.assertIsInstance(results['inputs'], torch.Tensor)
self.assertEqual(results['inputs'].shape, (2, 300, 17, 3))
- self.assertEqual(results['data_samples'].gt_labels.item,
+ self.assertEqual(results['data_samples'].gt_label,
torch.LongTensor([1]))
# heatmap_imgs input
@@ -45,7 +45,7 @@ def test_transform(self):
self.assertIn('data_samples', results)
self.assertIsInstance(results['inputs'], torch.Tensor)
self.assertEqual(results['inputs'].shape, (2, 17, 56, 56))
- self.assertEqual(results['data_samples'].gt_labels.item,
+ self.assertEqual(results['data_samples'].gt_label,
torch.LongTensor([1]))
# audios input
@@ -82,7 +82,7 @@ def test_transform(self):
self.assertIsInstance(results['inputs'], torch.Tensor)
self.assertIsInstance(results['data_samples'], ActionDataSample)
self.assertEqual(results['data_samples'].img_shape, (256, 256, 3))
- self.assertEqual(results['data_samples'].gt_labels.item,
+ self.assertEqual(results['data_samples'].gt_label,
torch.LongTensor([1]))
# Test grayscale image
@@ -191,12 +191,21 @@ def test_format_shape():
# invalid input format
FormatShape('NHWC')
- # 'NCHW' input format
+ # 'NCHW' input format (RGB Modality)
results = dict(
imgs=np.random.randn(3, 224, 224, 3), num_clips=1, clip_len=3)
format_shape = FormatShape('NCHW')
assert format_shape(results)['input_shape'] == (3, 3, 224, 224)
+ # `NCHW` input format (Flow Modality)
+ results = dict(
+ imgs=np.random.randn(3, 224, 224, 2),
+ num_clips=1,
+ clip_len=3,
+ modality='Flow')
+ format_shape = FormatShape('NCHW')
+ assert format_shape(results)['input_shape'] == (1, 6, 224, 224)
+
# `NCTHW` input format with num_clips=1, clip_len=3
results = dict(
imgs=np.random.randn(3, 224, 224, 3), num_clips=1, clip_len=3)
@@ -229,11 +238,6 @@ def test_format_shape():
format_shape = FormatShape('NCTHW_Heatmap')
assert format_shape(results)['input_shape'] == (2, 17, 6, 56, 56)
- # `NCHW_Flow` input format
- results = dict(imgs=np.random.randn(6, 224, 224), num_clips=1, clip_len=3)
- format_shape = FormatShape('NCHW_Flow')
- assert format_shape(results)['input_shape'] == (1, 6, 224, 224)
-
# `NPTCHW` input format
results = dict(
imgs=np.random.randn(72, 224, 224, 3),
diff --git a/tests/datasets/transforms/test_loading.py b/tests/datasets/transforms/test_loading.py
index 035a2213cc..888c993fd5 100644
--- a/tests/datasets/transforms/test_loading.py
+++ b/tests/datasets/transforms/test_loading.py
@@ -10,8 +10,7 @@
from mmengine.testing import assert_dict_has_keys
from numpy.testing import assert_array_almost_equal
-from mmaction.datasets.transforms import (AudioDecode, AudioDecodeInit,
- DecordDecode, DecordInit,
+from mmaction.datasets.transforms import (DecordDecode, DecordInit,
GenerateLocalizationLabels,
LoadAudioFeature, LoadHVULabel,
LoadLocalizationFeature,
@@ -487,8 +486,8 @@ def test_rawframe_decode(self):
frame_selector = RawFrameDecode(io_backend='disk')
results = frame_selector(inputs)
assert assert_dict_has_keys(results, target_keys)
- assert np.shape(results['imgs']) == (len(inputs['frame_inds']) * 2,
- 240, 320)
+ assert np.shape(results['imgs']) == (len(inputs['frame_inds']), 240,
+ 320, 2)
assert results['original_shape'] == (240, 320)
# test frame selector with 1 dim input for flow images
@@ -497,8 +496,8 @@ def test_rawframe_decode(self):
frame_selector = RawFrameDecode(io_backend='disk')
results = frame_selector(inputs)
assert assert_dict_has_keys(results, target_keys)
- assert np.shape(results['imgs']) == (len(inputs['frame_inds']) * 2,
- 240, 320)
+ assert np.shape(results['imgs']) == (len(inputs['frame_inds']), 240,
+ 320, 2)
assert results['original_shape'] == (240, 320)
return
@@ -533,42 +532,6 @@ def test_rawframe_decode(self):
f'{frame_selector.__class__.__name__}(io_backend=disk, '
f'decoding_backend=turbojpeg)')
- def test_audio_decode_init(self):
- try:
- import soundfile as sf # noqa: F401
- except (OSError, ImportError):
- return
- target_keys = ['audios', 'length', 'sample_rate']
- inputs = copy.deepcopy(self.audio_results)
- audio_decode_init = AudioDecodeInit()
- results = audio_decode_init(inputs)
- assert assert_dict_has_keys(results, target_keys)
-
- # test when no audio file exists
- inputs = copy.deepcopy(self.audio_results)
- inputs['audio_path'] = 'foo/foo/bar.wav'
- audio_decode_init = AudioDecodeInit()
- results = audio_decode_init(inputs)
- assert assert_dict_has_keys(results, target_keys)
- assert results['audios'].shape == (10.0 *
- audio_decode_init.sample_rate, )
- assert repr(audio_decode_init) == (
- f'{audio_decode_init.__class__.__name__}('
- f'io_backend=disk, '
- f'sample_rate=16000, '
- f'pad_method=zero)')
-
- def test_audio_decode(self):
- target_keys = ['frame_inds', 'audios']
- inputs = copy.deepcopy(self.audio_results)
- inputs['frame_inds'] = np.arange(0, self.audio_total_frames,
- 2)[:, np.newaxis]
- inputs['num_clips'] = 1
- inputs['length'] = 1280
- audio_selector = AudioDecode()
- results = audio_selector(inputs)
- assert assert_dict_has_keys(results, target_keys)
-
def test_pyav_decode_motion_vector(self):
pyav_init = PyAVInit()
pyav = PyAVDecodeMotionVector()
diff --git a/tests/datasets/transforms/test_pose_transforms.py b/tests/datasets/transforms/test_pose_transforms.py
index 913447f938..7383a5380c 100644
--- a/tests/datasets/transforms/test_pose_transforms.py
+++ b/tests/datasets/transforms/test_pose_transforms.py
@@ -2,17 +2,15 @@
import copy
import copy as cp
import os.path as osp
-import tempfile
from collections import defaultdict
import numpy as np
import pytest
-from mmengine import dump
from mmengine.testing import assert_dict_has_keys
from numpy.testing import assert_array_almost_equal, assert_array_equal
-from mmaction.datasets.transforms import (GeneratePoseTarget, GenSkeFeat,
- JointToBone, LoadKineticsPose,
+from mmaction.datasets.transforms import (DecompressPose, GeneratePoseTarget,
+ GenSkeFeat, JointToBone,
MergeSkeFeat, MMCompact, MMDecode,
MMUniformSampleFrames, PadTo,
PoseCompact, PoseDecode,
@@ -23,7 +21,7 @@
class TestPoseTransforms:
@staticmethod
- def test_load_kinetics_pose():
+ def test_decompress_pose():
def get_mode(arr):
cnt = defaultdict(lambda: 0)
@@ -32,86 +30,68 @@ def get_mode(arr):
max_val = max(cnt.values())
return [k for k in cnt if cnt[k] == max_val], max_val
- with tempfile.TemporaryDirectory() as tmpdir:
- filename = osp.join(tmpdir, 'tmp.pkl')
- total_frames = 100
- img_shape = (224, 224)
- frame_inds = np.random.choice(range(100), size=120)
- frame_inds.sort()
- anno_flag = np.random.random(120) > 0.1
- anno_inds = np.array([i for i, f in enumerate(anno_flag) if f])
- kp = np.random.random([120, 17, 3])
- dump(kp, filename)
- results = dict(
- filename=filename,
- total_frames=total_frames,
- img_shape=img_shape,
- frame_inds=frame_inds)
-
- inp = cp.deepcopy(results)
-
- with pytest.raises(NotImplementedError):
- LoadKineticsPose(squeeze=True, max_person=100, source='xxx')
-
- load_kinetics_pose = LoadKineticsPose(
- squeeze=True, max_person=100, source='openpose-18')
-
- assert str(load_kinetics_pose) == (
- 'LoadKineticsPose(io_backend=disk, '
- 'squeeze=True, max_person=100, '
- "keypoint_weight={'face': 1, "
- "'torso': 2, 'limb': 3}, "
- 'source=openpose-18, kwargs={})')
- return_results = load_kinetics_pose(inp)
- assert return_results['keypoint'].shape[:-1] == \
- return_results['keypoint_score'].shape
-
- num_person = return_results['keypoint'].shape[0]
- num_frame = return_results['keypoint'].shape[1]
- assert num_person == get_mode(frame_inds)[1]
- assert np.max(return_results['keypoint']) > 1
- assert num_frame == len(set(frame_inds))
-
- inp = cp.deepcopy(results)
- load_kinetics_pose = LoadKineticsPose(
- squeeze=False, max_person=100, source='openpose-18')
- return_results = load_kinetics_pose(inp)
- assert return_results['keypoint'].shape[:-1] == \
- return_results['keypoint_score'].shape
-
- num_person = return_results['keypoint'].shape[0]
- num_frame = return_results['keypoint'].shape[1]
- assert num_person == get_mode(frame_inds)[1]
- assert np.max(return_results['keypoint']) > 1
- assert num_frame == total_frames
-
- inp = cp.deepcopy(results)
- inp['anno_inds'] = anno_inds
- load_kinetics_pose = LoadKineticsPose(
- squeeze=True, max_person=100, source='mmpose')
- return_results = load_kinetics_pose(inp)
- assert return_results['keypoint'].shape[:-1] == \
- return_results['keypoint_score'].shape
-
- num_person = return_results['keypoint'].shape[0]
- num_frame = return_results['keypoint'].shape[1]
- assert num_person == get_mode(frame_inds[anno_inds])[1]
- assert np.max(return_results['keypoint']) <= 1
- assert num_frame == len(set(frame_inds[anno_inds]))
-
- inp = cp.deepcopy(results)
- inp['anno_inds'] = anno_inds
- load_kinetics_pose = LoadKineticsPose(
- squeeze=True, max_person=2, source='mmpose')
- return_results = load_kinetics_pose(inp)
- assert return_results['keypoint'].shape[:-1] == \
- return_results['keypoint_score'].shape
-
- num_person = return_results['keypoint'].shape[0]
- num_frame = return_results['keypoint'].shape[1]
- assert num_person <= 2
- assert np.max(return_results['keypoint']) <= 1
- assert num_frame == len(set(frame_inds[anno_inds]))
+ total_frames = 100
+ img_shape = (224, 224)
+ frame_inds = np.random.choice(range(100), size=120)
+ frame_inds.sort()
+ anno_flag = np.random.random(120) > 0.1
+ anno_inds = np.array([i for i, f in enumerate(anno_flag) if f])
+ kp = np.random.random([120, 17, 3])
+ results = dict(
+ frame_inds=frame_inds,
+ keypoint=kp,
+ total_frames=total_frames,
+ img_shape=img_shape)
+
+ inp = cp.deepcopy(results)
+
+ decompress_pose = DecompressPose(squeeze=True, max_person=100)
+
+ assert str(decompress_pose) == (
+ 'DecompressPose(squeeze=True, max_person=100)')
+ return_results = decompress_pose(inp)
+ assert return_results['keypoint'].shape[:-1] == \
+ return_results['keypoint_score'].shape
+
+ num_person = return_results['keypoint'].shape[0]
+ num_frame = return_results['keypoint'].shape[1]
+ assert num_person == get_mode(frame_inds)[1]
+ assert num_frame == len(set(frame_inds))
+
+ inp = cp.deepcopy(results)
+ decompress_pose = DecompressPose(squeeze=False, max_person=100)
+ return_results = decompress_pose(inp)
+ assert return_results['keypoint'].shape[:-1] == \
+ return_results['keypoint_score'].shape
+
+ num_person = return_results['keypoint'].shape[0]
+ num_frame = return_results['keypoint'].shape[1]
+ assert num_person == get_mode(frame_inds)[1]
+ assert num_frame == total_frames
+
+ inp = cp.deepcopy(results)
+ inp['anno_inds'] = anno_inds
+ decompress_pose = DecompressPose(squeeze=True, max_person=100)
+ return_results = decompress_pose(inp)
+ assert return_results['keypoint'].shape[:-1] == \
+ return_results['keypoint_score'].shape
+
+ num_person = return_results['keypoint'].shape[0]
+ num_frame = return_results['keypoint'].shape[1]
+ assert num_person == get_mode(frame_inds[anno_inds])[1]
+ assert num_frame == len(set(frame_inds[anno_inds]))
+
+ inp = cp.deepcopy(results)
+ inp['anno_inds'] = anno_inds
+ decompress_pose = DecompressPose(squeeze=True, max_person=2)
+ return_results = decompress_pose(inp)
+ assert return_results['keypoint'].shape[:-1] == \
+ return_results['keypoint_score'].shape
+
+ num_person = return_results['keypoint'].shape[0]
+ num_frame = return_results['keypoint'].shape[1]
+ assert num_person <= 2
+ assert num_frame == len(set(frame_inds[anno_inds]))
@staticmethod
def test_generate_pose_target():
diff --git a/tests/datasets/transforms/test_processing.py b/tests/datasets/transforms/test_processing.py
index 028f5d7129..cc7c18add2 100644
--- a/tests/datasets/transforms/test_processing.py
+++ b/tests/datasets/transforms/test_processing.py
@@ -7,11 +7,10 @@
from mmengine.testing import assert_dict_has_keys
from numpy.testing import assert_array_almost_equal
-from mmaction.datasets.transforms import (AudioAmplify, CenterCrop,
- ColorJitter, Flip, Fuse,
- MelSpectrogram, MultiScaleCrop,
- RandomCrop, RandomResizedCrop,
- Resize, TenCrop, ThreeCrop)
+from mmaction.datasets.transforms import (CenterCrop, ColorJitter, Flip, Fuse,
+ MultiScaleCrop, RandomCrop,
+ RandomResizedCrop, Resize, TenCrop,
+ ThreeCrop)
def check_crop(origin_imgs, result_imgs, result_bbox, num_crops=1):
@@ -70,59 +69,6 @@ def check_flip(origin_imgs, result_imgs, flip_type):
return True
-class TestAudio:
-
- @staticmethod
- def test_audio_amplify():
- target_keys = ['audios', 'amplify_ratio']
- with pytest.raises(TypeError):
- # ratio should be float
- AudioAmplify(1)
-
- audio = (np.random.rand(8, ))
- results = dict(audios=audio)
- amplifier = AudioAmplify(1.5)
- results = amplifier(results)
- assert assert_dict_has_keys(results, target_keys)
- assert repr(amplifier) == (f'{amplifier.__class__.__name__}'
- f'(ratio={amplifier.ratio})')
-
- @staticmethod
- def test_melspectrogram():
- target_keys = ['audios']
- with pytest.raises(TypeError):
- # ratio should be float
- MelSpectrogram(window_size=12.5)
- audio = (np.random.rand(1, 160000))
-
- # test padding
- results = dict(audios=audio, sample_rate=16000)
- results['num_clips'] = 1
- results['sample_rate'] = 16000
- mel = MelSpectrogram()
- try:
- import soundfile as sf # noqa: F401
- except (OSError, ImportError):
- return
-
- results = mel(results)
- assert assert_dict_has_keys(results, target_keys)
-
- # test truncating
- audio = (np.random.rand(1, 160000))
- results = dict(audios=audio, sample_rate=16000)
- results['num_clips'] = 1
- results['sample_rate'] = 16000
- mel = MelSpectrogram(fixed_length=1)
- results = mel(results)
- assert assert_dict_has_keys(results, target_keys)
- assert repr(mel) == (f'{mel.__class__.__name__}'
- f'(window_size={mel.window_size}), '
- f'step_size={mel.step_size}, '
- f'n_mels={mel.n_mels}, '
- f'fixed_length={mel.fixed_length})')
-
-
class TestColor:
@staticmethod
diff --git a/tests/evaluation/metrics/test_acc_metric.py b/tests/evaluation/metrics/test_acc_metric.py
index aeb6fb2cb0..b0e966933e 100644
--- a/tests/evaluation/metrics/test_acc_metric.py
+++ b/tests/evaluation/metrics/test_acc_metric.py
@@ -26,8 +26,7 @@ def generate_data(num_classes=5, random_label=False, multi_label=False):
label = torch.randint(num_classes, size=[1])
else:
label = torch.LongTensor([scores.argmax().item()])
- data_sample = dict(
- pred_scores=dict(item=scores), gt_labels=dict(item=label))
+ data_sample = dict(pred_score=scores, gt_label=label)
data_samples.append(data_sample)
return data_batch, data_samples
@@ -97,7 +96,7 @@ def test_evaluate(self):
"""Test using the metric in the same way as Evalutor."""
pred = [
ActionDataSample().set_pred_score(i).set_pred_label(
- j).set_gt_labels(k).to_dict() for i, j, k in zip([
+ j).set_gt_label(k).to_dict() for i, j, k in zip([
torch.tensor([0.7, 0.0, 0.3]),
torch.tensor([0.5, 0.2, 0.3]),
torch.tensor([0.4, 0.5, 0.1]),
@@ -122,7 +121,7 @@ def test_evaluate(self):
# Test with label
for sample in pred:
- del sample['pred_scores']
+ del sample['pred_score']
metric = METRICS.build(dict(type='ConfusionMatrix'))
metric.process(None, pred)
with self.assertRaisesRegex(AssertionError,
diff --git a/tests/evaluation/metrics/test_retrieval_metric.py b/tests/evaluation/metrics/test_retrieval_metric.py
index cb1f1c72ba..fffc0dbacc 100644
--- a/tests/evaluation/metrics/test_retrieval_metric.py
+++ b/tests/evaluation/metrics/test_retrieval_metric.py
@@ -1,8 +1,13 @@
# Copyright (c) OpenMMLab. All rights reserved.
+from unittest import TestCase
+
+import numpy as np
import pytest
import torch
-from mmaction.evaluation.metrics import RetrievalMetric
+from mmaction.evaluation.metrics import RetrievalMetric, RetrievalRecall
+from mmaction.registry import METRICS
+from mmaction.structures import ActionDataSample
def generate_data(num_samples=5, feat_dim=10, random_label=False):
@@ -47,3 +52,114 @@ def test_acc_metric():
assert eval_results['R1'] == eval_results['R5'] == eval_results[
'R10'] == 100.0
assert eval_results['MdR'] == eval_results['MnR'] == 1.0
+
+
+class TestRetrievalRecall(TestCase):
+
+ def test_evaluate(self):
+ """Test using the metric in the same way as Evalutor."""
+ pred = [
+ ActionDataSample().set_pred_score(i).set_gt_label(k).to_dict()
+ for i, k in zip([
+ torch.tensor([0.7, 0.0, 0.3]),
+ torch.tensor([0.5, 0.2, 0.3]),
+ torch.tensor([0.4, 0.5, 0.1]),
+ torch.tensor([0.0, 0.0, 1.0]),
+ torch.tensor([0.0, 0.0, 1.0]),
+ torch.tensor([0.0, 0.0, 1.0]),
+ ], [[0], [0], [1], [2], [2], [0]])
+ ]
+
+ # Test with score (use score instead of label if score exists)
+ metric = METRICS.build(dict(type='RetrievalRecall', topk=1))
+ metric.process(None, pred)
+ recall = metric.evaluate(6)
+ self.assertIsInstance(recall, dict)
+ self.assertAlmostEqual(
+ recall['retrieval/Recall@1'], 5 / 6 * 100, places=4)
+
+ # Test with invalid topk
+ with self.assertRaisesRegex(RuntimeError, 'selected index k'):
+ metric = METRICS.build(dict(type='RetrievalRecall', topk=10))
+ metric.process(None, pred)
+ metric.evaluate(6)
+
+ with self.assertRaisesRegex(ValueError, '`topk` must be a'):
+ METRICS.build(dict(type='RetrievalRecall', topk=-1))
+
+ # Test initialization
+ metric = METRICS.build(dict(type='RetrievalRecall', topk=5))
+ self.assertEqual(metric.topk, (5, ))
+
+ # Test initialization
+ metric = METRICS.build(dict(type='RetrievalRecall', topk=(1, 2, 5)))
+ self.assertEqual(metric.topk, (1, 2, 5))
+
+ def test_calculate(self):
+ """Test using the metric from static method."""
+
+ # seq of indices format
+ y_true = [[0, 2, 5, 8, 9], [1, 4, 6]]
+ y_pred = [np.arange(10)] * 2
+
+ # test with average is 'macro'
+ recall_score = RetrievalRecall.calculate(
+ y_pred, y_true, topk=1, pred_indices=True, target_indices=True)
+ expect_recall = 50.
+ self.assertEqual(recall_score[0].item(), expect_recall)
+
+ # test with tensor input
+ y_true = torch.Tensor([[1, 0, 1, 0, 0, 1, 0, 0, 1, 1],
+ [0, 1, 0, 0, 1, 0, 1, 0, 0, 0]])
+ y_pred = np.array([np.linspace(0.95, 0.05, 10)] * 2)
+ recall_score = RetrievalRecall.calculate(y_pred, y_true, topk=1)
+ expect_recall = 50.
+ self.assertEqual(recall_score[0].item(), expect_recall)
+
+ # test with topk is 5
+ y_pred = np.array([np.linspace(0.95, 0.05, 10)] * 2)
+ recall_score = RetrievalRecall.calculate(y_pred, y_true, topk=2)
+ expect_recall = 100.
+ self.assertEqual(recall_score[0].item(), expect_recall)
+
+ # test with topk is (1, 5)
+ y_pred = np.array([np.linspace(0.95, 0.05, 10)] * 2)
+ recall_score = RetrievalRecall.calculate(y_pred, y_true, topk=(1, 5))
+ expect_recalls = [50., 100.]
+ self.assertEqual(len(recall_score), len(expect_recalls))
+ for i in range(len(expect_recalls)):
+ self.assertEqual(recall_score[i].item(), expect_recalls[i])
+
+ # Test with invalid pred
+ y_pred = dict()
+ y_true = [[0, 2, 5, 8, 9], [1, 4, 6]]
+ with self.assertRaisesRegex(AssertionError, '`pred` must be Seq'):
+ RetrievalRecall.calculate(y_pred, y_true, True, True)
+
+ # Test with invalid target
+ y_true = dict()
+ y_pred = [np.arange(10)] * 2
+ with self.assertRaisesRegex(AssertionError, '`target` must be Seq'):
+ RetrievalRecall.calculate(
+ y_pred, y_true, topk=1, pred_indices=True, target_indices=True)
+
+ # Test with different length `pred` with `target`
+ y_true = [[0, 2, 5, 8, 9], [1, 4, 6]]
+ y_pred = [np.arange(10)] * 3
+ with self.assertRaisesRegex(AssertionError, 'Length of `pred`'):
+ RetrievalRecall.calculate(
+ y_pred, y_true, topk=1, pred_indices=True, target_indices=True)
+
+ # Test with invalid pred
+ y_true = [[0, 2, 5, 8, 9], dict()]
+ y_pred = [np.arange(10)] * 2
+ with self.assertRaisesRegex(AssertionError, '`target` should be'):
+ RetrievalRecall.calculate(
+ y_pred, y_true, topk=1, pred_indices=True, target_indices=True)
+
+ # Test with invalid target
+ y_true = [[0, 2, 5, 8, 9], [1, 4, 6]]
+ y_pred = [np.arange(10), dict()]
+ with self.assertRaisesRegex(AssertionError, '`pred` should be'):
+ RetrievalRecall.calculate(
+ y_pred, y_true, topk=1, pred_indices=True, target_indices=True)
diff --git a/tests/models/backbones/test_mobileone_tsm.py b/tests/models/backbones/test_mobileone_tsm.py
new file mode 100644
index 0000000000..b018e9f5a2
--- /dev/null
+++ b/tests/models/backbones/test_mobileone_tsm.py
@@ -0,0 +1,85 @@
+# Copyright (c) OpenMMLab. All rights reserved.
+import os
+import tempfile
+
+import torch
+from mmengine.runner import load_checkpoint, save_checkpoint
+from mmengine.runner.checkpoint import _load_checkpoint_with_prefix
+
+from mmaction.models.backbones.mobileone_tsm import MobileOneTSM
+from mmaction.testing import generate_backbone_demo_inputs
+
+
+def test_mobileone_tsm_backbone():
+ """Test MobileOne TSM backbone."""
+
+ from mmpretrain.models.backbones.mobileone import MobileOneBlock
+
+ from mmaction.models.backbones.resnet_tsm import TemporalShift
+
+ model = MobileOneTSM('s0', pretrained2d=False)
+ model.init_weights()
+ for cur_module in model.modules():
+ if isinstance(cur_module, TemporalShift):
+ # TemporalShift is a wrapper of MobileOneBlock
+ assert isinstance(cur_module.net, MobileOneBlock)
+ assert cur_module.num_segments == model.num_segments
+ assert cur_module.shift_div == model.shift_div
+
+ inputs = generate_backbone_demo_inputs((8, 3, 64, 64))
+
+ feat = model(inputs)
+ assert feat.shape == torch.Size([8, 1024, 2, 2])
+
+ model = MobileOneTSM('s1', pretrained2d=False)
+ feat = model(inputs)
+ assert feat.shape == torch.Size([8, 1280, 2, 2])
+
+ model = MobileOneTSM('s2', pretrained2d=False)
+ feat = model(inputs)
+ assert feat.shape == torch.Size([8, 2048, 2, 2])
+
+ model = MobileOneTSM('s3', pretrained2d=False)
+ feat = model(inputs)
+ assert feat.shape == torch.Size([8, 2048, 2, 2])
+
+ model = MobileOneTSM('s4', pretrained2d=False)
+ feat = model(inputs)
+ assert feat.shape == torch.Size([8, 2048, 2, 2])
+
+
+def test_mobileone_init_weight():
+ checkpoint = ('https://download.openmmlab.com/mmclassification/v0'
+ '/mobileone/mobileone-s0_8xb32_in1k_20221110-0bc94952.pth')
+ # ckpt = torch.load(checkpoint)['state_dict']
+ model = MobileOneTSM(
+ arch='s0',
+ init_cfg=dict(
+ type='Pretrained', checkpoint=checkpoint, prefix='backbone'))
+ model.init_weights()
+ ori_ckpt = _load_checkpoint_with_prefix(
+ 'backbone', model.init_cfg['checkpoint'], map_location='cpu')
+ for name, param in model.named_parameters():
+ ori_name = name.replace('.net', '')
+ assert torch.allclose(param, ori_ckpt[ori_name]), \
+ f'layer {name} fail to load from pretrained checkpoint'
+
+
+def test_load_deploy_mobileone():
+ # Test output before and load from deploy checkpoint
+ model = MobileOneTSM('s0', pretrained2d=False)
+ inputs = generate_backbone_demo_inputs((8, 3, 64, 64))
+ tmpdir = tempfile.gettempdir()
+ ckpt_path = os.path.join(tmpdir, 'ckpt.pth')
+ model.switch_to_deploy()
+ model.eval()
+ outputs = model(inputs)
+
+ model_deploy = MobileOneTSM('s0', pretrained2d=False, deploy=True)
+ save_checkpoint(model.state_dict(), ckpt_path)
+ load_checkpoint(model_deploy, ckpt_path)
+
+ outputs_load = model_deploy(inputs)
+ for feat, feat_load in zip(outputs, outputs_load):
+ assert torch.allclose(feat, feat_load)
+ os.remove(ckpt_path)
diff --git a/tests/models/backbones/test_resnet_audio.py b/tests/models/backbones/test_resnet_audio.py
index 6c22bd137a..48af1744f5 100644
--- a/tests/models/backbones/test_resnet_audio.py
+++ b/tests/models/backbones/test_resnet_audio.py
@@ -3,6 +3,7 @@
from mmaction.models import ResNetAudio
from mmaction.testing import generate_backbone_demo_inputs
+from mmaction.utils import register_all_modules
def test_resnet_audio_backbone():
@@ -10,6 +11,7 @@ def test_resnet_audio_backbone():
input_shape = (1, 1, 16, 16)
spec = generate_backbone_demo_inputs(input_shape)
# inference
+ register_all_modules()
audioonly = ResNetAudio(50, None)
audioonly.init_weights()
audioonly.train()
diff --git a/tests/models/data_preprocessors/test_data_preprocessor.py b/tests/models/data_preprocessors/test_data_preprocessor.py
index 5fe3e8f663..9591305691 100644
--- a/tests/models/data_preprocessors/test_data_preprocessor.py
+++ b/tests/models/data_preprocessors/test_data_preprocessor.py
@@ -15,7 +15,7 @@ def generate_dummy_data(batch_size, input_shape):
'inputs':
[torch.randint(0, 255, input_shape) for _ in range(batch_size)],
'data_samples':
- [ActionDataSample().set_gt_labels(2) for _ in range(batch_size)]
+ [ActionDataSample().set_gt_label(2) for _ in range(batch_size)]
}
return data
@@ -53,8 +53,8 @@ def test_data_preprocessor():
format_shape='NCTHW',
blending=dict(type='MixupBlending', num_classes=5))
data = psr(deepcopy(raw_data), training=True)
- assert data['data_samples'][0].gt_labels.item.shape == (5, )
- assert data['data_samples'][1].gt_labels.item.shape == (5, )
+ assert data['data_samples'][0].gt_label.shape == (5, )
+ assert data['data_samples'][1].gt_label.shape == (5, )
raw_data = generate_dummy_data(2, (1, 3, 224, 224))
psr = ActionDataPreprocessor(
diff --git a/tests/models/data_preprocessors/test_multimodal_data_preprocessor.py b/tests/models/data_preprocessors/test_multimodal_data_preprocessor.py
index 35483bd5d9..671d2c1c96 100644
--- a/tests/models/data_preprocessors/test_multimodal_data_preprocessor.py
+++ b/tests/models/data_preprocessors/test_multimodal_data_preprocessor.py
@@ -13,7 +13,7 @@
def generate_dummy_data(batch_size, input_keys, input_shapes):
data = dict()
data['data_samples'] = [
- ActionDataSample().set_gt_labels(2) for _ in range(batch_size)
+ ActionDataSample().set_gt_label(2) for _ in range(batch_size)
]
data['inputs'] = dict()
for key, shape in zip(input_keys, input_shapes):
diff --git a/tests/models/heads/test_feature_head.py b/tests/models/heads/test_feature_head.py
index 932ed87133..424016bc8d 100644
--- a/tests/models/heads/test_feature_head.py
+++ b/tests/models/heads/test_feature_head.py
@@ -27,7 +27,7 @@ def test_2d_recognizer(self):
input_shape = [3, 3, 32, 32]
data_batch = {
'inputs': [torch.randint(0, 256, input_shape)],
- 'data_samples': [ActionDataSample().set_gt_labels(2)]
+ 'data_samples': [ActionDataSample().set_gt_label(2)]
}
feat = recognizer.test_step(data_batch)
assert isinstance(feat, torch.Tensor)
@@ -46,7 +46,7 @@ def test_3d_recognizer(self):
input_shape = [1, 3, 4, 32, 32]
data_batch = {
'inputs': [torch.randint(0, 256, input_shape)],
- 'data_samples': [ActionDataSample().set_gt_labels(2)]
+ 'data_samples': [ActionDataSample().set_gt_label(2)]
}
feat = recognizer.test_step(data_batch)
assert isinstance(feat, torch.Tensor)
diff --git a/tests/models/heads/test_omni_head.py b/tests/models/heads/test_omni_head.py
index f9181893af..2724830353 100644
--- a/tests/models/heads/test_omni_head.py
+++ b/tests/models/heads/test_omni_head.py
@@ -31,9 +31,7 @@ def testOmniHead():
video_feat = torch.randn(2, 400, 8, 8, 8)
video_score = head(video_feat)
assert video_score.shape == torch.Size([2, 200])
- data_samples = [
- obj('gt_label', obj('label', torch.tensor(1))) for _ in range(2)
- ]
+ data_samples = [obj('gt_label', torch.tensor(1)) for _ in range(2)]
losses = head.loss_by_feat(video_score, data_samples)
assert 'loss_cls' in losses
@@ -41,6 +39,6 @@ def testOmniHead():
head.eval()
image_score = head(image_feat)
assert image_score.shape == torch.Size([1, 100])
- data_samples = [obj('gt_labels', obj('item', torch.tensor(1)))]
+ data_samples = [obj('gt_label', torch.tensor(1))]
losses = head.loss_by_feat(image_score, data_samples)
assert 'loss_cls' in losses
diff --git a/tests/models/necks/test_tpn.py b/tests/models/necks/test_tpn.py
index 1e9387aa39..08cc17dedc 100644
--- a/tests/models/necks/test_tpn.py
+++ b/tests/models/necks/test_tpn.py
@@ -3,7 +3,6 @@
import pytest
import torch
-from mmengine.structures import LabelData
from mmaction.models import TPN
from mmaction.structures import ActionDataSample
@@ -14,7 +13,7 @@ def get_label(label_):
label = []
for idx, one_label in enumerate(label_):
data_sample = ActionDataSample()
- data_sample.gt_labels = LabelData(item=label_[idx])
+ data_sample.set_gt_label(label_[idx])
label.append(data_sample)
return label
diff --git a/tests/models/recognizers/recognizer_omni.py b/tests/models/recognizers/recognizer_omni.py
index 23c58748de..e06cd5c03f 100644
--- a/tests/models/recognizers/recognizer_omni.py
+++ b/tests/models/recognizers/recognizer_omni.py
@@ -12,8 +12,7 @@
def test_omni_resnet():
register_all_modules()
config = get_recognizer_cfg(
- 'omnisource/slowonly_r50_16xb16-8x8x1-256e_imagenet-kinetics400-rgb.py'
- )
+ 'omnisource/slowonly_r50_8xb16-8x8x1-256e_imagenet-kinetics400-rgb.py')
recognizer = MODELS.build(config.model)
# test train_step
@@ -24,8 +23,8 @@ def test_omni_resnet():
torch.randint(0, 255, (1, 3, 8, 224, 224))
],
'data_samples': [
- ActionDataSample().set_gt_labels(2),
- ActionDataSample().set_gt_labels(2)
+ ActionDataSample().set_gt_label(2),
+ ActionDataSample().set_gt_label(2)
]
}
@@ -35,8 +34,8 @@ def test_omni_resnet():
torch.randint(0, 255, (1, 3, 224, 224))
],
'data_samples': [
- ActionDataSample().set_gt_labels(2),
- ActionDataSample().set_gt_labels(2)
+ ActionDataSample().set_gt_label(2),
+ ActionDataSample().set_gt_label(2)
]
}
@@ -54,7 +53,7 @@ def test_omni_resnet():
# test test_step
with torch.no_grad():
predictions = recognizer.test_step(video_sample)
- score = predictions[0].pred_scores.item
- assert len(predictions) == 1
+ score = predictions[0].pred_score
+ assert len(predictions) == 2
assert torch.min(score) >= 0
assert torch.max(score) <= 1
diff --git a/tests/models/recognizers/test_recognizer2d.py b/tests/models/recognizers/test_recognizer2d.py
index b40398755b..9c48877204 100644
--- a/tests/models/recognizers/test_recognizer2d.py
+++ b/tests/models/recognizers/test_recognizer2d.py
@@ -21,7 +21,7 @@ def train_test_step(cfg, input_shape):
'inputs':
[torch.randint(0, 256, input_shape) for i in range(batch_size)],
'data_samples':
- [ActionDataSample().set_gt_labels(2) for i in range(batch_size)]
+ [ActionDataSample().set_gt_label(2) for i in range(batch_size)]
}
# test train_step
@@ -34,7 +34,7 @@ def train_test_step(cfg, input_shape):
# test test_step
with torch.no_grad():
predictions = recognizer.test_step(data_batch)
- score = predictions[0].pred_scores.item
+ score = predictions[0].pred_score
assert len(predictions) == batch_size
assert score.shape == torch.Size([num_classes])
assert torch.min(score) >= 0
@@ -46,7 +46,7 @@ def train_test_step(cfg, input_shape):
data_batch['inputs'] = [torch.randint(0, 256, input_shape)]
with torch.no_grad():
predictions = recognizer.test_step(data_batch)
- score = predictions[0].pred_scores.item
+ score = predictions[0].pred_score
assert len(predictions) == batch_size
assert score.shape == torch.Size([num_classes])
@@ -90,6 +90,16 @@ def test_tsn_mmcls_backbone():
train_test_step(config, input_shape)
+def test_tsn_mobileone():
+ register_all_modules()
+ config = get_recognizer_cfg(
+ 'tsn/custom_backbones/tsn_imagenet-pretrained-mobileone-s4_8xb32-1x1x8-100e_kinetics400-rgb.py' # noqa: E501
+ )
+ config.model['backbone']['init_cfg'] = None
+ input_shape = (1, 3, 3, 32, 32)
+ train_test_step(config, input_shape)
+
+
def test_tsn_timm_backbone():
# test tsn from timm
register_all_modules()
@@ -142,6 +152,7 @@ def test_tsn_tv_backbone():
def test_tsm():
register_all_modules()
+ # test tsm-mobilenetv2
config = get_recognizer_cfg(
'tsm/tsm_imagenet-pretrained-mobilenetv2_8xb16-1x1x8-100e_kinetics400-rgb.py' # noqa: E501
)
@@ -151,6 +162,7 @@ def test_tsm():
input_shape = (1, 8, 3, 32, 32)
train_test_step(config, input_shape)
+ # test tsm-res50
config = get_recognizer_cfg(
'tsm/tsm_imagenet-pretrained-r50_8xb16-1x1x8-50e_kinetics400-rgb.py')
config.model['backbone']['pretrained'] = None
@@ -159,6 +171,16 @@ def test_tsm():
input_shape = (1, 8, 3, 32, 32)
train_test_step(config, input_shape)
+ # test tsm-mobileone
+ config = get_recognizer_cfg(
+ 'tsm/tsm_imagenet-pretrained-mobileone-s4_8xb16-1x1x16-50e_kinetics400-rgb.py' # noqa: E501
+ )
+ config.model['backbone']['init_cfg'] = None
+ config.model['backbone']['pretrained2d'] = None
+
+ input_shape = (1, 16, 3, 32, 32)
+ train_test_step(config, input_shape)
+
def test_trn():
register_all_modules()
diff --git a/tests/models/recognizers/test_recognizer3d.py b/tests/models/recognizers/test_recognizer3d.py
index 7d80de00fb..c9f73d1a10 100644
--- a/tests/models/recognizers/test_recognizer3d.py
+++ b/tests/models/recognizers/test_recognizer3d.py
@@ -14,7 +14,7 @@ def train_test_step(cfg, input_shape):
num_classes = cfg.model.cls_head.num_classes
data_batch = {
'inputs': [torch.randint(0, 256, input_shape)],
- 'data_samples': [ActionDataSample().set_gt_labels(2)]
+ 'data_samples': [ActionDataSample().set_gt_label(2)]
}
# test train_step
@@ -27,7 +27,7 @@ def train_test_step(cfg, input_shape):
# test test_step
with torch.no_grad():
predictions = recognizer.test_step(data_batch)
- score = predictions[0].pred_scores.item
+ score = predictions[0].pred_score
assert len(predictions) == 1
assert score.shape == torch.Size([num_classes])
assert torch.min(score) >= 0
@@ -40,7 +40,7 @@ def train_test_step(cfg, input_shape):
data_batch['inputs'] = [torch.randint(0, 256, input_shape)]
with torch.no_grad():
predictions = recognizer.test_step(data_batch)
- score = predictions[0].pred_scores.item
+ score = predictions[0].pred_score
assert len(predictions) == 1
assert score.shape == torch.Size([num_views, num_classes])
diff --git a/tests/models/recognizers/test_recognizer_gcn.py b/tests/models/recognizers/test_recognizer_gcn.py
index 7ae1441a6b..723c77d595 100644
--- a/tests/models/recognizers/test_recognizer_gcn.py
+++ b/tests/models/recognizers/test_recognizer_gcn.py
@@ -14,7 +14,7 @@ def train_test_step(cfg, input_shape):
num_classes = cfg.model.cls_head.num_classes
data_batch = {
'inputs': [torch.randn(input_shape)],
- 'data_samples': [ActionDataSample().set_gt_labels(2)]
+ 'data_samples': [ActionDataSample().set_gt_label(2)]
}
# test train_step
@@ -27,7 +27,7 @@ def train_test_step(cfg, input_shape):
# test test_step
with torch.no_grad():
predictions = recognizer.test_step(data_batch)
- score = predictions[0].pred_scores.item
+ score = predictions[0].pred_score
assert len(predictions) == 1
assert score.shape == torch.Size([num_classes])
assert torch.min(score) >= 0
@@ -40,7 +40,7 @@ def train_test_step(cfg, input_shape):
data_batch['inputs'] = [torch.randn(input_shape)]
with torch.no_grad():
predictions = recognizer.test_step(data_batch)
- score = predictions[0].pred_scores.item
+ score = predictions[0].pred_score
assert len(predictions) == 1
assert score.shape == torch.Size([num_clips, num_classes])
diff --git a/tests/models/roi_heads/test_bbox_heads.py b/tests/models/roi_heads/test_bbox_heads.py
index 3b756051b1..8f04e8c8ff 100644
--- a/tests/models/roi_heads/test_bbox_heads.py
+++ b/tests/models/roi_heads/test_bbox_heads.py
@@ -10,13 +10,14 @@ def test_bbox_head_ava():
bbox head."""
with pytest.raises(TypeError):
# topk must be None, int or tuple[int]
- BBoxHeadAVA(topk=0.1)
+ BBoxHeadAVA(background_class=True, topk=0.1)
with pytest.raises(AssertionError):
# topk should be smaller than num_classes
- BBoxHeadAVA(num_classes=5, topk=(3, 5))
+ BBoxHeadAVA(background_class=True, num_classes=5, topk=(3, 5))
- bbox_head = BBoxHeadAVA(in_channels=10, num_classes=4, topk=1)
+ bbox_head = BBoxHeadAVA(
+ background_class=True, in_channels=10, num_classes=4, topk=1)
input = torch.randn([3, 10, 2, 2, 2])
ret = bbox_head(input)
assert ret.shape == (3, 4)
@@ -48,10 +49,16 @@ def test_bbox_head_ava():
torch.ones([4, 6], dtype=bool))
# Test Multi-Label Loss
- bbox_head = BBoxHeadAVA() # Why is this here? isn't this redundant?
+ bbox_head = BBoxHeadAVA(
+ background_class=True) # Why is this here? isn't this redundant?
bbox_head.init_weights()
- bbox_head = BBoxHeadAVA(temporal_pool_type='max', spatial_pool_type='avg')
+ bbox_head = BBoxHeadAVA(
+ background_class=True,
+ temporal_pool_type='max',
+ spatial_pool_type='avg')
bbox_head.init_weights()
+
+ # test without background class
"""
losses = bbox_head.loss(
cls_score=cls_score,
diff --git a/tests/models/similarity/test_clip_similarity.py b/tests/models/similarity/test_clip_similarity.py
index 9afa158243..6838cf812e 100644
--- a/tests/models/similarity/test_clip_similarity.py
+++ b/tests/models/similarity/test_clip_similarity.py
@@ -1,6 +1,8 @@
# Copyright (c) OpenMMLab. All rights reserved.
+import platform
from unittest.mock import MagicMock
+import pytest
import torch
from mmaction.registry import MODELS
@@ -9,6 +11,7 @@
from mmaction.utils import register_all_modules
+@pytest.mark.skipif(platform.system() == 'Windows', reason='Windows mem limit')
def test_clip_similarity():
register_all_modules()
cfg = get_similarity_cfg(
diff --git a/tests/models/utils/test_blending_utils.py b/tests/models/utils/test_blending_utils.py
index 993b331093..e2eba9de47 100644
--- a/tests/models/utils/test_blending_utils.py
+++ b/tests/models/utils/test_blending_utils.py
@@ -4,7 +4,6 @@
import torch
import torch.nn.functional as F
from mmcv.transforms import to_tensor
-from mmengine.structures import LabelData
from mmaction.models import CutmixBlending, MixupBlending, RandomBatchAugment
from mmaction.structures import ActionDataSample
@@ -14,7 +13,7 @@ def get_label(label_):
label = []
for idx, one_label in enumerate(label_):
data_sample = ActionDataSample()
- data_sample.gt_labels = LabelData(item=label_[idx])
+ data_sample.set_gt_label(label_[idx])
label.append(data_sample)
return label
diff --git a/tests/models/utils/test_gradcam.py b/tests/models/utils/test_gradcam.py
index e9568531c5..3982907bcb 100644
--- a/tests/models/utils/test_gradcam.py
+++ b/tests/models/utils/test_gradcam.py
@@ -41,7 +41,7 @@ def _do_test_2D_models(recognizer,
device='cpu'):
demo_data = {
'inputs': [torch.randint(0, 256, input_shape[1:])],
- 'data_samples': [ActionDataSample().set_gt_labels(2)]
+ 'data_samples': [ActionDataSample().set_gt_label(2)]
}
recognizer = recognizer.to(device)
@@ -67,7 +67,7 @@ def _do_test_3D_models(recognizer,
input_shape, num_classes=num_classes, model_type='3D')
demo_data = {
'inputs': [torch.randint(0, 256, input_shape[1:])],
- 'data_samples': [ActionDataSample().set_gt_labels(2)]
+ 'data_samples': [ActionDataSample().set_gt_label(2)]
}
gradcam = GradCAM(recognizer, target_layer_name)
diff --git a/tests/visualization/test_action_visualizer.py b/tests/visualization/test_action_visualizer.py
index c86b324af9..298b59a842 100644
--- a/tests/visualization/test_action_visualizer.py
+++ b/tests/visualization/test_action_visualizer.py
@@ -3,8 +3,6 @@
import decord
import pytest
-import torch
-from mmengine.structures import LabelData
from mmaction.structures import ActionDataSample
from mmaction.visualization import ActionVisualizer
@@ -16,7 +14,7 @@ def test_visualizer():
video = video.get_batch(range(32)).asnumpy()
data_sample = ActionDataSample()
- data_sample.gt_labels = LabelData(item=torch.tensor([2]))
+ data_sample.set_gt_label(2)
vis = ActionVisualizer()
vis.add_datasample('demo', video)
diff --git a/tests/visualization/test_video_backend.py b/tests/visualization/test_video_backend.py
index c5153d812d..591646eb7a 100644
--- a/tests/visualization/test_video_backend.py
+++ b/tests/visualization/test_video_backend.py
@@ -8,8 +8,6 @@
import decord
import pytest
-import torch
-from mmengine.structures import LabelData
from mmaction.structures import ActionDataSample
from mmaction.utils import register_all_modules
@@ -24,7 +22,7 @@ def test_local_visbackend():
video = video.get_batch(range(32)).asnumpy()
data_sample = ActionDataSample()
- data_sample.gt_labels = LabelData(item=torch.tensor([2]))
+ data_sample.set_gt_label(2)
with TemporaryDirectory() as tmp_dir:
vis = ActionVisualizer(
save_dir=tmp_dir, vis_backends=[dict(type='LocalVisBackend')])
@@ -46,7 +44,7 @@ def test_tensorboard_visbackend():
video = video.get_batch(range(32)).asnumpy()
data_sample = ActionDataSample()
- data_sample.gt_labels = LabelData(item=torch.tensor([2]))
+ data_sample.set_gt_label(2)
with TemporaryDirectory() as tmp_dir:
vis = ActionVisualizer(
save_dir=tmp_dir,
@@ -63,29 +61,3 @@ def test_tensorboard_visbackend():
# wait tensorboard store asynchronously
time.sleep(1)
return
-
-
-"""
-def test_wandb_visbackend():
- video = decord.VideoReader('./demo/demo.mp4')
- video = video.get_batch(range(32)).asnumpy()
-
- data_sample = ActionDataSample()
- data_sample.gt_labels = LabelData(item=torch.tensor([2]))
-
- vis = ActionVisualizer(
- save_dir='./outputs', vis_backends=[dict(type='WandbVisBackend')])
- vis.add_datasample('demo', video, data_sample, step=1)
-
- wandb_dir = 'outputs/vis_data/wandb/'
- assert Path(wandb_dir).exists()
-
- flag = False
- for item in os.listdir(wandb_dir):
- if item.startswith('run-') and os.path.isdir('%s/%s' %
- (wandb_dir, item)):
- flag = True
- break
- assert flag, 'Cannot find wandb folder!'
- return
-"""
diff --git a/tools/analysis_tools/report_accuracy.py b/tools/analysis_tools/report_accuracy.py
index c361f644de..d5c529dfe1 100644
--- a/tools/analysis_tools/report_accuracy.py
+++ b/tools/analysis_tools/report_accuracy.py
@@ -39,20 +39,13 @@ def main():
data_sample_list = [load(f) for f in args.preds]
score_list = []
for data_samples in data_sample_list:
- scores = [
- sample['pred_scores']['item'].numpy() for sample in data_samples
- ]
+ scores = [sample['pred_score'].numpy() for sample in data_samples]
score_list.append(scores)
if args.multi_label:
- labels = [
- sample['gt_labels']['item'] for sample in data_sample_list[0]
- ]
+ labels = [sample['gt_label'] for sample in data_sample_list[0]]
else:
- labels = [
- sample['gt_labels']['item'].item()
- for sample in data_sample_list[0]
- ]
+ labels = [sample['gt_label'].item() for sample in data_sample_list[0]]
if args.apply_softmax:
diff --git a/tools/convert/reparameterize_model.py b/tools/convert/reparameterize_model.py
new file mode 100644
index 0000000000..6220e092fc
--- /dev/null
+++ b/tools/convert/reparameterize_model.py
@@ -0,0 +1,57 @@
+# Copyright (c) OpenMMLab. All rights reserved.
+import argparse
+from pathlib import Path
+
+import torch
+
+from mmaction.apis import init_recognizer
+from mmaction.models.recognizers import BaseRecognizer
+
+
+def convert_recoginzer_to_deploy(model, checkpoint, save_path):
+ print('Converting...')
+ assert hasattr(model, 'backbone') and \
+ hasattr(model.backbone, 'switch_to_deploy'), \
+ '`model.backbone` must has method of "switch_to_deploy".' \
+ f' But {model.backbone.__class__} does not have.'
+
+ model.backbone.switch_to_deploy()
+ checkpoint['state_dict'] = model.state_dict()
+ torch.save(checkpoint, save_path)
+
+ print('Done! Save at path "{}"'.format(save_path))
+
+
+def main():
+ parser = argparse.ArgumentParser(
+ description='Convert the parameters of the repvgg block '
+ 'from training mode to deployment mode.')
+ parser.add_argument(
+ 'config_path',
+ help='The path to the configuration file of the network '
+ 'containing the repvgg block.')
+ parser.add_argument(
+ 'checkpoint_path',
+ help='The path to the checkpoint file corresponding to the model.')
+ parser.add_argument(
+ 'save_path',
+ help='The path where the converted checkpoint file is stored.')
+ args = parser.parse_args()
+
+ save_path = Path(args.save_path)
+ if save_path.suffix != '.pth' and save_path.suffix != '.tar':
+ print('The path should contain the name of the pth format file.')
+ exit()
+ save_path.parent.mkdir(parents=True, exist_ok=True)
+
+ model = init_recognizer(
+ args.config_path, checkpoint=args.checkpoint_path, device='cpu')
+ assert isinstance(model, BaseRecognizer), \
+ '`model` must be a `mmpretrain.classifiers.ImageClassifier` instance.'
+
+ checkpoint = torch.load(args.checkpoint_path)
+ convert_recoginzer_to_deploy(model, checkpoint, args.save_path)
+
+
+if __name__ == '__main__':
+ main()
diff --git a/tools/data/build_audio_features.py b/tools/data/build_audio_features.py
index 28356a0e64..cd3070bace 100644
--- a/tools/data/build_audio_features.py
+++ b/tools/data/build_audio_features.py
@@ -38,11 +38,16 @@ class AudioTools:
`_.
Args:
- frame_rate (int): The frame rate per second of the video. Default: 30.
- sample_rate (int): The sample rate for audio sampling. Default: 16000.
- num_mels (int): Number of channels of the melspectrogram. Default: 80.
- fft_size (int): fft_size / sample_rate is window size. Default: 1280.
- hop_size (int): hop_size / sample_rate is step size. Default: 320.
+ frame_rate (int): The frame rate per second of the video.
+ Defaults to 30.
+ sample_rate (int): The sample rate for audio sampling.
+ Defaults to 16000.
+ num_mels (int): Number of channels of the melspectrogram.
+ Defaults to 80.
+ fft_size (int): fft_size / sample_rate is window size.
+ Defaults to 1280.
+ hop_size (int): hop_size / sample_rate is step size.
+ Defaults to 320.
"""
def __init__(self,
@@ -290,15 +295,15 @@ def extract_audio_feature(wav_path, audio_tools, mel_out_dir):
parser.add_argument('audio_home_path', type=str)
parser.add_argument('spectrogram_save_path', type=str)
parser.add_argument('--level', type=int, default=1)
- parser.add_argument('--ext', default='.m4a')
+ parser.add_argument('--ext', default='m4a')
parser.add_argument('--num-workers', type=int, default=4)
parser.add_argument('--part', type=str, default='1/1')
args = parser.parse_args()
mmengine.mkdir_or_exist(args.spectrogram_save_path)
- files = glob.glob(
- osp.join(args.audio_home_path, '*/' * args.level, '*' + args.ext))
+ files = glob.glob(args.audio_home_path + '/*' * args.level + '.' +
+ args.ext)
print(f'found {len(files)} files.')
files = sorted(files)
if args.part is not None:
diff --git a/tools/data/charades-sta/README.md b/tools/data/charades-sta/README.md
new file mode 100644
index 0000000000..b2bea83d2b
--- /dev/null
+++ b/tools/data/charades-sta/README.md
@@ -0,0 +1,59 @@
+# Preparing AVA
+
+## Introduction
+
+
+
+```BibTeX
+@inproceedings{gao2017tall,
+ title={Tall: Temporal activity localization via language query},
+ author={Gao, Jiyang and Sun, Chen and Yang, Zhenheng and Nevatia, Ram},
+ booktitle={Proceedings of the IEEE international conference on computer vision},
+ pages={5267--5275},
+ year={2017}
+}
+
+@inproceedings{DRN2020CVPR,
+ author = {Runhao, Zeng and Haoming, Xu and Wenbing, Huang and Peihao, Chen and Mingkui, Tan and Chuang Gan},
+ title = {Dense Regression Network for Video Grounding},
+ booktitle = {CVPR},
+ year = {2020},
+}
+```
+
+Charades-STA is a new dataset built on top of Charades by adding sentence temporal annotations. It is introduced by Gao et al. in `TALL: Temporal Activity Localization via Language Query`. Currently, we only support C3D features from `Dense Regression Network for Video Grounding`.
+
+## Step 1. Prepare Annotations
+
+First of all, you can run the following script to prepare annotations from the official repository of DRN:
+
+```shell
+bash download_annotations.sh
+```
+
+## Step 2. Prepare C3D features
+
+After the first step, you should be at `${MMACTION2}/data/CharadesSTA/`. Download the C3D features following the [official command](https://github.com/Alvin-Zeng/DRN/tree/master#download-features) to the current directory `${MMACTION2}/data/CharadesSTA/`.
+
+After finishing the two steps, the folder structure will look like:
+
+```
+mmaction2
+โโโ mmaction
+โโโ tools
+โโโ configs
+โโโ data
+โ โโโ CharadesSTA
+โ โ โโโ C3D_unit16_overlap0.5_merged
+โ โ | โโโ 001YG.pt
+โ โ | โโโ 003WS.pt
+โ โ | โโโ 004QE.pt
+โ โ | โโโ 00607.pt
+โ โ | โโโ ...
+โ โ โโโ Charades_duration.json
+โ โ โโโ Charades_fps_dict.json
+โ โ โโโ Charades_frames_info.json
+โ โ โโโ Charades_sta_test.txt
+โ โ โโโ Charades_sta_train.txt
+โ โ โโโ Charades_word2id.json
+```
diff --git a/tools/data/charades-sta/download_annotations.sh b/tools/data/charades-sta/download_annotations.sh
new file mode 100644
index 0000000000..85bdb7d1a8
--- /dev/null
+++ b/tools/data/charades-sta/download_annotations.sh
@@ -0,0 +1,18 @@
+#!/usr/bin/env bash
+
+DATA_DIR="../../../data/CharadesSTA/"
+
+if [[ ! -d "${DATA_DIR}" ]]; then
+ echo "${DATA_DIR} does not exist. Creating";
+ mkdir -p ${DATA_DIR}
+fi
+
+cd ${DATA_DIR}
+
+URL="https://raw.githubusercontent.com/Alvin-Zeng/DRN/master/data/dataset/Charades"
+wget ${URL}/Charades_frames_info.json
+wget ${URL}/Charades_duration.json
+wget ${URL}/Charades_fps_dict.json
+wget ${URL}/Charades_sta_test.txt
+wget ${URL}/Charades_sta_train.txt
+wget ${URL}/Charades_word2id.json
diff --git a/tools/data/msrvtt/README.md b/tools/data/msrvtt/README.md
new file mode 100644
index 0000000000..e9e72ad6b4
--- /dev/null
+++ b/tools/data/msrvtt/README.md
@@ -0,0 +1,68 @@
+# Preparing MSR-VTT Retrieval/ Video Question-Answering Dataset
+
+## Introduction
+
+
+
+```BibTeX
+@inproceedings{xu2016msr,
+ title={Msr-vtt: A large video description dataset for bridging video and language},
+ author={Xu, Jun and Mei, Tao and Yao, Ting and Rui, Yong},
+ booktitle={CVPR},
+ pages={5288--5296},
+ year={2016}
+}
+```
+
+Before preparing the dataset, please make sure that the directory is located at `$MMACTION2/tools/data/msrvtt/`.
+
+## Step 1. Download Annotation Files
+
+You can directly download the following annotation files related to MSR-VTT from the [Google Drive link](https://drive.google.com/drive/folders/12cr94wT8j7pR09AR2nmQg6o26Y1arI50) provided by [VindLU](https://github.com/klauscc) and place them in the `$MMACTION2/tools/data/msrvtt/annotations` directory:
+
+- [msrvtt_qa_train.json](https://drive.google.com/file/d/12dJq5_7v8FytrJwrPB_f22tET1MmGCNh/view?usp=drive_link)
+- [msrvtt_qa_val.json](https://drive.google.com/file/d/138q-A-V8fCC2nBYJgqkQa3gBfXVNbNNd/view?usp=drive_link)
+- [msrvtt_qa_test.json](https://drive.google.com/file/d/13IiEcUMHiNppWhGwVY1eAaip6iSJM35A/view?usp=drive_link)
+- [msrvtt_qa_answer_list.json](https://drive.google.com/file/d/131euz_dssRkDTk3-ioAS5ZsvIxS_Tt4M/view?usp=drive_link)
+- [msrvtt_mc_test.json](https://drive.google.com/file/d/13FrUQ2ZDsNDraP7lfnKvTArPIgdtHuLC/view?usp=drive_link)
+- [msrvtt_ret_train9k.json](https://drive.google.com/file/d/13OVo0XRdVWTHlFFxbKg3daYCHsMbJxyd/view?usp=drive_link)
+- [msrvtt_ret_train7k.json](https://drive.google.com/file/d/13ID97BX4ExO6mWPIUMp-GzXcPBkviSLx/view?usp=drive_link)
+- [msrvtt_ret_test1k.json](https://drive.google.com/file/d/13FLrjI-aleKeU7LbJMDrYgktX7MbTbzu/view?usp=drive_link)
+- [msrvtt_test1k.json](https://drive.google.com/file/d/12z6y-DNwIfICSzOhekbJwSbf7z2hlibE/view?usp=drive_link)
+
+## Step 2. Prepare Video Data
+
+You can refer to the [official website](https://www.microsoft.com/en-us/research/publication/msr-vtt-a-large-video-description-dataset-for-bridging-video-and-language/) of this dataset for basic information. Run the following commands to prepare the MSRVTT video files:
+
+```shell
+# Download original videos
+bash download_msrvtt.sh
+# Preprocess videos to lower FPS and dimensions
+bash compress_msrvtt.sh
+```
+
+After completing the above preparation steps, the directory structure will be as follows:
+
+```
+mmaction2
+โโโ mmaction
+โโโ tools
+โโโ configs
+โโโ data
+โ โโโ msrvtt
+โ โ โโโ annotations
+โ โ โ โโโ msrvtt_qa_train.json
+โ โ โ โโโ msrvtt_qa_val.json
+โ โ โ โโโ msrvtt_qa_test.json
+โ โ โ โโโ msrvtt_qa_answer_list.json
+โ โ โ โโโ msrvtt_mc_test.json
+โ โ โ โโโ msrvtt_ret_train9k.json
+โ โ โ โโโ msrvtt_ret_train7k.json
+โ โ โ โโโ msrvtt_ret_test1k.json
+โ โ โ โโโ msrvtt_test1k.json
+โ โ โโโ videos_2fps_224
+โ โ โโโ video0.mp4
+โ โ โโโ video1.mp4
+โ โ โโโ ...
+โ โ โโโ video9999.mp4
+```
diff --git a/tools/data/msrvtt/README_zh-CN.md b/tools/data/msrvtt/README_zh-CN.md
new file mode 100644
index 0000000000..bbd3a009c4
--- /dev/null
+++ b/tools/data/msrvtt/README_zh-CN.md
@@ -0,0 +1,68 @@
+# ๅๅค MSR-VTT ๆฃ็ดข/่ง้ข้ฎ็ญๆฐๆฎ้
+
+## ็ฎไป
+
+
+
+```BibTeX
+@inproceedings{xu2016msr,
+ title={Msr-vtt: A large video description dataset for bridging video and language},
+ author={Xu, Jun and Mei, Tao and Yao, Ting and Rui, Yong},
+ booktitle={CVPR},
+ pages={5288--5296},
+ year={2016}
+}
+```
+
+ๅจๆฐๆฎ้ๅๅคๅ๏ผ่ฏท็กฎไฟๅฝไปค่กๅฝๅ่ทฏๅพไธบ `$MMACTION2/tools/data/msrvtt/`ใ
+
+## ๆญฅ้ชค 1. ไธ่ฝฝๆ ๆณจๆไปถ
+
+็จๆทๅฏไป [VindLU](https://github.com/klauscc/VindLU) ๆไพ็ [Google Drive ้พๆฅ](https://drive.google.com/drive/folders/12cr94wT8j7pR09AR2nmQg6o26Y1arI50)ไธญ็ดๆฅไธ่ฝฝไปฅไธไธ MSR-VTT ็ธๅ
ณ็ๆ ๆณจๆไปถ, ๅนถๆพ็ฝฎๅฐ `$MMACTION2/tools/data/msrvtt/annotations` ่ทฏๅพไธ:
+
+- [msrvtt_qa_train.json](https://drive.google.com/file/d/12dJq5_7v8FytrJwrPB_f22tET1MmGCNh/view?usp=drive_link)
+- [msrvtt_qa_val.json](https://drive.google.com/file/d/138q-A-V8fCC2nBYJgqkQa3gBfXVNbNNd/view?usp=drive_link)
+- [msrvtt_qa_test.json](https://drive.google.com/file/d/13IiEcUMHiNppWhGwVY1eAaip6iSJM35A/view?usp=drive_link)
+- [msrvtt_qa_answer_list.json](https://drive.google.com/file/d/131euz_dssRkDTk3-ioAS5ZsvIxS_Tt4M/view?usp=drive_link)
+- [msrvtt_mc_test.json](https://drive.google.com/file/d/13FrUQ2ZDsNDraP7lfnKvTArPIgdtHuLC/view?usp=drive_link)
+- [msrvtt_ret_train9k.json](https://drive.google.com/file/d/13OVo0XRdVWTHlFFxbKg3daYCHsMbJxyd/view?usp=drive_link)
+- [msrvtt_ret_train7k.json](https://drive.google.com/file/d/13ID97BX4ExO6mWPIUMp-GzXcPBkviSLx/view?usp=drive_link)
+- [msrvtt_ret_test1k.json](https://drive.google.com/file/d/13FLrjI-aleKeU7LbJMDrYgktX7MbTbzu/view?usp=drive_link)
+- [msrvtt_test1k.json](https://drive.google.com/file/d/12z6y-DNwIfICSzOhekbJwSbf7z2hlibE/view?usp=drive_link)
+
+## ๆญฅ้ชค 2. ๅๅค่ง้ขๆฐๆฎ
+
+็จๆทๅฏๅ่่ฏฅๆฐๆฎ้็[ๅฎ็ฝ](https://www.microsoft.com/en-us/research/publication/msr-vtt-a-large-video-description-dataset-for-bridging-video-and-language/)๏ผไปฅ่ทๅๆฐๆฎ้็ธๅ
ณ็ๅบๆฌไฟกๆฏใ่ฟ่กไธ้ข็ๅฝไปคๅๅค MSRVTT ่ง้ขๆไปถ:
+
+```shell
+# download original videos
+bash download_msrvtt.sh
+# preprocess videos to lower FPS and dimension
+bash compress_msrvtt.sh
+```
+
+ๅฎๆไธ่ฟฐๅๅคๆญฅ้ชคๅ๏ผๆไปถ็ฎๅฝๅฆไธ๏ผ
+
+```
+mmaction2
+โโโ mmaction
+โโโ tools
+โโโ configs
+โโโ data
+โ โโโ msrvtt
+โ โ โโโ annotations
+โ โ โ โโโ msrvtt_qa_train.json
+โ โ โ โโโ msrvtt_qa_val.json
+โ โ โ โโโ msrvtt_qa_test.json
+โ โ โ โโโ msrvtt_qa_answer_list.json
+โ โ โ โโโ msrvtt_mc_test.json
+โ โ โ โโโ msrvtt_ret_train9k.json
+โ โ โ โโโ msrvtt_ret_train7k.json
+โ โ โ โโโ msrvtt_ret_test1k.json
+โ โ โ โโโ msrvtt_test1k.json
+โ โ โโโ videos_2fps_224
+โ โ โโโ video0.mp4
+โ โ โโโ video1.mp4
+โ โ โโโ ...
+โ โ โโโ video9999.mp4
+```
diff --git a/tools/data/msrvtt/compress.py b/tools/data/msrvtt/compress.py
new file mode 100644
index 0000000000..48f022ddba
--- /dev/null
+++ b/tools/data/msrvtt/compress.py
@@ -0,0 +1,192 @@
+# Copyright (c) OpenMMLab. All rights reserved.
+"""Used to compress videos (FPS and dimensions) in the Singularity project.
+
+copied from https://github.com/klauscc/VindLU
+"""
+import argparse
+import os
+import shutil
+import subprocess
+from multiprocessing import Pool
+from os.path import exists, join
+from pathlib import Path
+
+try:
+ from psutil import cpu_count
+except ImportError:
+ from multiprocessing import cpu_count
+
+from functools import partial
+
+from PIL import Image
+from tqdm import tqdm
+
+
+def resize_image(input_path, output_path, size=224):
+ with Image.open(input_path) as img:
+ w, h = img.width, img.height
+ r = 1. * w / h
+ if w > h:
+ h = size
+ w = r * size
+ else:
+ h = size / r
+ w = size
+
+ img_resized = img.resize((int(w), int(h)))
+ img_resized.save(output_path)
+
+
+def _compress_images(input_output_pair, size=224):
+ """Scale and downsample an input image to a given fps and size (shorter
+ side size).
+
+ This also removes the audio from the image.
+ """
+ input_image_path, output_image_path = input_output_pair
+ try:
+ resize_image(input_image_path, output_image_path, size)
+ except Exception as e:
+ print(f'Caught Exception {e}')
+
+
+def _compress_videos(input_output_pair, size=224, fps=3):
+ """Scale and downsample an input video to a given fps and size (shorter
+ side size).
+
+ This also removes the audio from the video.
+ """
+ input_file_path, output_file_path = input_output_pair
+ try:
+ command = [
+ 'ffmpeg',
+ '-y', # (optional) overwrite output file if it exists
+ '-i',
+ input_file_path,
+ '-filter:v', # no audio
+ f"scale='if(gt(a,1),trunc(oh*a/2)*2,{size})':'if(gt(a,1),{size},trunc(ow*a/2)*2)'", # noqa: E501
+ '-map',
+ '0:v', # no audio
+ '-r',
+ str(fps), # frames per second
+ # '-g', str(16),
+ output_file_path,
+ ]
+ subprocess.run(
+ command, stdout=subprocess.PIPE, stderr=subprocess.STDOUT)
+ except Exception as e:
+ raise e
+
+
+def _compress(input_output_pair, fps=3, size=224, file_type='image'):
+ if file_type == 'image':
+ _compress_images(input_output_pair, size)
+ elif file_type == 'video':
+ _compress_videos(input_output_pair, size, fps)
+
+
+def prepare_input_output_pairs(input_root,
+ output_root,
+ input_file_list_path=None):
+ # filename list in `input_file_list_path` can be created very fast using `ls -U . >> ../video_filenames.txt` # noqa: E501
+ if input_file_list_path:
+ with open(input_file_list_path, 'r') as f:
+ filenames = [s.strip() for s in f.readlines()]
+ else:
+ filenames = [
+ video_path.name for video_path in Path(input_root).glob('*.mp4')
+ ]
+ print(f'There are {len(filenames)} video/images files loaded from list.')
+ input_file_path_list = []
+ output_file_path_list = []
+ for e in tqdm(filenames, desc='find un-processed videos/images'):
+ input_file_path = join(input_root, e)
+ output_file_path = join(output_root, e)
+ if not exists(output_file_path):
+ input_file_path_list.append(input_file_path)
+ output_file_path_list.append(output_file_path)
+ return input_file_path_list, output_file_path_list
+
+
+def run_compress():
+ parser = argparse.ArgumentParser(
+ description='Compress videos/images for speed-up')
+ parser.add_argument(
+ '--input_root', type=str, help='input root', required=True)
+ parser.add_argument(
+ '--input_file_list_path',
+ type=str,
+ default=None,
+ help='list of video filenames under args.input_root, it can be '
+ 'created efficiently with `ls -U /path/to/video >> /path/to/video_filenames.txt`' # noqa: E501
+ )
+ parser.add_argument(
+ '--output_root', type=str, help='output root', required=True)
+ parser.add_argument(
+ '--size',
+ type=int,
+ default=224,
+ help='shorter side size, aspect ratio is kept')
+ parser.add_argument('--num_workers', type=int, default=24, help='#workers')
+ parser.add_argument(
+ '--fps',
+ type=int,
+ default=3,
+ help='fps for output video, ignored if file_type == image')
+ parser.add_argument(
+ '--file_type',
+ type=str,
+ choices=['image', 'video'],
+ help='input file type')
+ args = parser.parse_args()
+
+ # set paths
+ input_root = args.input_root
+ output_root = args.output_root
+ assert input_root != output_root
+ if not exists(output_root):
+ os.makedirs(output_root, exist_ok=True)
+
+ # prepare and find un-processed
+ input_file_path_list, output_file_path_list = prepare_input_output_pairs(
+ input_root,
+ output_root,
+ input_file_list_path=args.input_file_list_path,
+ )
+ print(f'input_file_path_list[:3] {input_file_path_list[:3]}')
+ print(f'output_file_path_list[:3] {output_file_path_list[:3]}')
+ print('Total videos/images need to process: {}'.format(
+ len(input_file_path_list)))
+
+ # start parallel jobs
+ num_cores = cpu_count()
+ num_workers = args.num_workers
+ print(
+ f'Begin with {num_cores}-core logical processor, {num_workers} workers'
+ )
+ compress = partial(
+ _compress, fps=args.fps, size=args.size, file_type=args.file_type)
+ input_pairs = list(zip(input_file_path_list, output_file_path_list))
+ with Pool(num_workers) as pool, tqdm(
+ total=len(input_file_path_list),
+ desc='re-encoding videos/images') as pbar:
+ for idx, _ in enumerate(
+ pool.imap_unordered(compress, input_pairs, chunksize=32)):
+ pbar.update(1)
+
+ # copy-paste failed files
+ print('Compress finished, copy-paste failed files...')
+ copy_count = 0
+ for input_file_path, output_file_path in zip(input_file_path_list,
+ output_file_path_list):
+ if exists(input_file_path):
+ if exists(output_file_path) is False or os.path.getsize(
+ output_file_path) < 1.:
+ copy_count += 1
+ shutil.copyfile(input_file_path, output_file_path)
+ print('Copy and replace file: {}'.format(output_file_path))
+ print(f'copy_count {copy_count}')
+
+
+if __name__ == '__main__':
+ run_compress()
diff --git a/tools/data/msrvtt/compress_msrvtt.sh b/tools/data/msrvtt/compress_msrvtt.sh
new file mode 100644
index 0000000000..18822ce312
--- /dev/null
+++ b/tools/data/msrvtt/compress_msrvtt.sh
@@ -0,0 +1,10 @@
+#!/usr/bin/env bash
+
+FPS=2
+SIZE=224
+DATA_DIR="../../../data/msrvtt/videos"
+OUT_DIR="../../../data/msrvtt/videos_2fps_224"
+
+python compress.py \
+ --input_root=${DATA_DIR} --output_root=${OUT_DIR} \
+ --fps=${FPS} --size=${SIZE} --file_type=video --num_workers 24
diff --git a/tools/data/msrvtt/download_msrvtt.sh b/tools/data/msrvtt/download_msrvtt.sh
new file mode 100644
index 0000000000..6ae40d942d
--- /dev/null
+++ b/tools/data/msrvtt/download_msrvtt.sh
@@ -0,0 +1,22 @@
+#!/usr/bin/env bash
+
+DATA_DIR="../../../data/msrvtt"
+mkdir -p ${DATA_DIR}
+
+if [ -f "MSRVTT.zip" ]; then
+ echo "MSRVTT.zip exists, skip downloading!"
+else
+ echo "Downloading MSRVTT.zip."
+ wget https://www.robots.ox.ac.uk/~maxbain/frozen-in-time/data/MSRVTT.zip
+fi
+
+echo "Processing videos started."
+unzip -q MSRVTT.zip -d ${DATA_DIR}
+mkdir -p "${DATA_DIR}/videos/" && find "${DATA_DIR}/MSRVTT/videos/all" -name "video*.mp4" -exec mv {} "${DATA_DIR}/videos/" \;
+echo "Processing videos completed."
+
+rm -rf "${DATA_DIR}/MSRVTT"
+rm -rf "${DATA_DIR}/msrvtt_data"
+rm msrvtt_data.zip
+rm MSRVTT.zip
+echo "The preparation of the msrvtt dataset has been successfully completed."
diff --git a/tools/data/skeleton/README.md b/tools/data/skeleton/README.md
index 2f55a2021e..b836db17da 100644
--- a/tools/data/skeleton/README.md
+++ b/tools/data/skeleton/README.md
@@ -32,7 +32,7 @@ We provide links to the pre-processed skeleton annotations, you can directly dow
- Diving48 \[2D Skeleton\]: https://download.openmmlab.com/mmaction/v1.0/skeleton/data/diving48_2d.pkl
- Kinetics400 \[2D Skeleton\]: https://download.openmmlab.com/mmaction/v1.0/skeleton/data/k400_2d.pkl (Table of contents only, no skeleton annotations)
-For Kinetics400, since the skeleton annotations are large, we do not provide the direct download links on aliyun. Please use the following link to download the `kpfiles` and extract it under `$MMACTION2/data/k400` for Kinetics400 training & testing: https://mycuhk-my.sharepoint.com/:u:/g/personal/1155136485_link_cuhk_edu_hk/EeyDCVskqLtClMVVwqD53acBF2FEwkctp3vtRbkLfnKSTw?e=B3SZlM
+For Kinetics400, since the skeleton annotations are large, we do not provide the direct download links on aliyun. Please use the following link to download the `k400_kpfiles_2d.zip` and extract it under `$MMACTION2/data/skeleton/kpfiles` for Kinetics400 training & testing: https://openxlab.org.cn/datasets/OpenMMLab/Kinetics400-skeleton
If you want to generate 2D skeleton annotations of specified video, please install mmdetection and mmpose first, then use the following script to extract skeleton annotations of NTURGB+D video:
diff --git a/tools/data/skeleton/README_zh-CN.md b/tools/data/skeleton/README_zh-CN.md
index 2cd354a1d5..c2e01a6311 100644
--- a/tools/data/skeleton/README_zh-CN.md
+++ b/tools/data/skeleton/README_zh-CN.md
@@ -44,7 +44,7 @@ bash download_annotations.sh ${DATASET}
- Diving48 \[2D Skeleton\]: https://download.openmmlab.com/mmaction/v1.0/skeleton/data/diving48_2d.pkl
- Kinetics400 \[2D Skeleton\]: https://download.openmmlab.com/mmaction/v1.0/skeleton/data/k400_2d.pkl (ๅชๅ
ๅซๆฐๆฎๅ่กจ๏ผๆฒกๆๅงฟๆๆ ๆณจๆไปถ)
-็ฑไบ Kinetics400 ๆฐๆฎ้ๅงฟๆๆ ๆณจๆไปถ่ฟๅคง๏ผๆไปฌไธๆไพ้ฟ้ไบ็ไธ่ฝฝ้พๆฅ๏ผ่ฏทไฝฟ็จๆญค[้พๆฅ](https://mycuhk-my.sharepoint.com/:u:/g/personal/1155136485_link_cuhk_edu_hk/EeyDCVskqLtClMVVwqD53acBF2FEwkctp3vtRbkLfnKSTw?e=B3SZlM)ไธ่ฝฝ `kpfiles`๏ผ่งฃๅๅฐ `$MMACTION2/data/k400` ็ฎๅฝไธ๏ผ็จไบ Kinetics400 ็่ฎญ็ปๅๆต่ฏใ
+็ฑไบ Kinetics400 ๆฐๆฎ้ๅงฟๆๆ ๆณจๆไปถ่ฟๅคง๏ผๆไปฌไธๆไพ้ฟ้ไบ็ไธ่ฝฝ้พๆฅ๏ผ่ฏทไฝฟ็จๆญค[้พๆฅ](https://openxlab.org.cn/datasets/OpenMMLab/Kinetics400-skeleton)ไธ่ฝฝ `k400_kpfiles_2d.zip`๏ผ่งฃๅๅฐ `$MMACTION2/data/skeleton/kpfiles` ็ฎๅฝไธ๏ผ็จไบ Kinetics400 ็่ฎญ็ปๅๆต่ฏใ
่ฅๆณ็ๆๅไธช่ง้ข็ 2D ๅงฟๆๆ ๆณจๆไปถ๏ผ็จๆทๅจๅฎ่ฃ
mmdetection ๅ mmpose ไนๅ๏ผๅฏไฝฟ็จไปฅไธ่ๆฌ่ฟ่ก NTURGB+D ่ง้ข็ๅงฟๆๆๅ๏ผ
diff --git a/tools/data/video_retrieval/README.md b/tools/data/video_retrieval/README.md
index 77f05ddcf7..99a7398c25 100644
--- a/tools/data/video_retrieval/README.md
+++ b/tools/data/video_retrieval/README.md
@@ -14,6 +14,16 @@
}
```
+```BibTeX
+@inproceedings{chen2011collecting,
+ title={Collecting highly parallel data for paraphrase evaluation},
+ author={Chen, David and Dolan, William B},
+ booktitle={ACL},
+ pages={190--200},
+ year={2011}
+}
+```
+
Before we start, please make sure that the directory is located at `$MMACTION2/tools/data/video_retrieval/`.
## Preparing MSRVTT dataset
@@ -43,3 +53,31 @@ mmaction2
โ โ โโโ ...
โ โ โโโ video9999.mp4
```
+
+## Preparing MSVD dataset
+
+For basic dataset information, you can refer to the MSVD dataset [website](https://www.cs.utexas.edu/users/ml/clamp/videoDescription/). Run the following command to prepare the MSVD dataset:
+
+```shell
+bash prepare_msvd.sh
+```
+
+After preparation, the folder structure will look like:
+
+```
+mmaction2
+โโโ mmaction
+โโโ tools
+โโโ configs
+โโโ data
+โ โโโ video_retrieval
+โ โ โโโ msrvd
+โ โ โโโ train.json
+โ โ โโโ test.json
+โ โ โโโ val.json
+โ โ โโโโ videos
+โ โ โโโ xxx.avi
+โ โ โโโ xxx.avi
+โ โ โโโ ...
+โ โ โโโ xxx.avi
+```
diff --git a/tools/data/video_retrieval/README_zh-CN.md b/tools/data/video_retrieval/README_zh-CN.md
index a4cd194f58..1814ff36e2 100644
--- a/tools/data/video_retrieval/README_zh-CN.md
+++ b/tools/data/video_retrieval/README_zh-CN.md
@@ -14,6 +14,16 @@
}
```
+```BibTeX
+@inproceedings{chen2011collecting,
+ title={Collecting highly parallel data for paraphrase evaluation},
+ author={Chen, David and Dolan, William B},
+ booktitle={ACL},
+ pages={190--200},
+ year={2011}
+}
+```
+
ๅจๆฐๆฎ้ๅๅคๅ๏ผ่ฏท็กฎไฟๅฝไปค่กๅฝๅ่ทฏๅพไธบ `$MMACTION2/tools/data/video_retrieval/`ใ
## ๅๅค MSRVTT ๆฐๆฎ้
@@ -24,7 +34,7 @@
bash prepare_msrvtt.sh
```
-ๅฎๅบไธ่ฟฐๅๅคๆญฅ้ชคๅ๏ผๆไปถ็ฎๅฝๅฆไธ๏ผ
+ๅฎๆไธ่ฟฐๅๅคๆญฅ้ชคๅ๏ผๆไปถ็ฎๅฝๅฆไธ๏ผ
```
mmaction2
@@ -43,3 +53,31 @@ mmaction2
โ โ โโโ ...
โ โ โโโ video9999.mp4
```
+
+## ๅๅค MSVD ๆฐๆฎ้
+
+็จๆทๅฏๅ่่ฏฅๆฐๆฎ้็[ๅฎ็ฝ](https://www.cs.utexas.edu/users/ml/clamp/videoDescription/)๏ผไปฅ่ทๅๆฐๆฎ้็ธๅ
ณ็ๅบๆฌไฟกๆฏใ่ฟ่กไธ้ข็ๅฝไปคๅๅค MSVD ๆฐๆฎ้๏ผ
+
+```shell
+bash prepare_msvd.sh
+```
+
+ๅฎๅบไธ่ฟฐๅๅคๆญฅ้ชคๅ๏ผๆไปถ็ฎๅฝๅฆไธ๏ผ
+
+```
+mmaction2
+โโโ mmaction
+โโโ tools
+โโโ configs
+โโโ data
+โ โโโ video_retrieval
+โ โ โโโ msvd
+โ โ โโโ train.json
+โ โ โโโ text.json
+โ โ โโโ val.json
+โ โ โโโโ videos
+โ โ โโโ xxx.avi
+โ โ โโโ xxx.avi
+โ โ โโโ ...
+โ โ โโโ xxx.avi
+```
diff --git a/tools/data/video_retrieval/prepare_msvd.py b/tools/data/video_retrieval/prepare_msvd.py
new file mode 100644
index 0000000000..b8cc4377cf
--- /dev/null
+++ b/tools/data/video_retrieval/prepare_msvd.py
@@ -0,0 +1,55 @@
+# Copyright (c) OpenMMLab. All rights reserved.
+import json
+import os.path as osp
+import pickle
+
+DATA_DIR = '../../../data/video_retrieval/msvd'
+SUFFIX = '.avi'
+
+data_path = osp.join(DATA_DIR, 'msvd_data/raw-captions.pkl')
+train_txt_path = osp.join(DATA_DIR, 'msvd_data/train_list.txt')
+test_txt_path = osp.join(DATA_DIR, 'msvd_data/test_list.txt')
+val_txt_path = osp.join(DATA_DIR, 'msvd_data/val_list.txt')
+train_json_path = osp.join(DATA_DIR, 'train.json')
+test_json_path = osp.join(DATA_DIR, 'test.json')
+val_json_path = osp.join(DATA_DIR, 'val.json')
+
+with open(data_path, 'rb') as F:
+ data = pickle.load(F)
+
+video_dict = {}
+for one_data in data:
+ caption = data[one_data]
+ if one_data not in video_dict:
+ video_dict[one_data] = []
+ for cap in caption:
+ video_dict[one_data].append(' '.join(cap))
+
+with open(train_txt_path, 'r') as f:
+ train_avi = f.readlines()
+
+train_avi_list = {}
+for video in train_avi:
+ train_avi_list[video.strip() + SUFFIX] = video_dict[video.strip()]
+
+with open(train_json_path, 'w') as f:
+ json.dump(train_avi_list, f)
+
+with open(test_txt_path, 'r') as f:
+ test_avi = f.readlines()
+
+test_avi_list = {}
+for video in test_avi:
+ test_avi_list[video.strip() + SUFFIX] = video_dict[video.strip()]
+with open(test_json_path, 'w') as f:
+ json.dump(test_avi_list, f)
+
+with open(val_txt_path, 'r') as f:
+ val_avi = f.readlines()
+
+val_avi_list = {}
+for video in val_avi:
+ val_avi_list[video.strip() + SUFFIX] = video_dict[video.strip()]
+
+with open(val_json_path, 'w') as f:
+ json.dump(val_avi_list, f)
diff --git a/tools/data/video_retrieval/prepare_msvd.sh b/tools/data/video_retrieval/prepare_msvd.sh
new file mode 100644
index 0000000000..5f804fe8bf
--- /dev/null
+++ b/tools/data/video_retrieval/prepare_msvd.sh
@@ -0,0 +1,35 @@
+#!/usr/bin/env bash
+
+DATA_DIR="../../../data/video_retrieval/msvd"
+mkdir -p ${DATA_DIR}
+
+
+if [ -f "msvd_data.zip" ]; then
+ echo "msvd_data.zip exists, skip downloading!"
+else
+ echo "Downloading msvd_data.zip."
+ wget https://github.com/ArrowLuo/CLIP4Clip/releases/download/v0.0/msvd_data.zip
+fi
+
+echo "Processing annotations started."
+unzip -q msvd_data.zip -d ${DATA_DIR}
+python prepare_msvd.py
+echo "Processing annotations completed."
+
+if [ -f "YouTubeClips.tar" ]; then
+ echo "YouTubeClips.tar exists, skip downloading!"
+else
+ echo "Downloading YouTubeClips.tar."
+ wget https://www.cs.utexas.edu/users/ml/clamp/videoDescription/YouTubeClips.tar
+fi
+
+echo "Processing videos started."
+tar -xf YouTubeClips.tar -C ${DATA_DIR}
+mkdir -p "${DATA_DIR}/videos/" && find "${DATA_DIR}/YouTubeClips" -name "*.avi" -exec mv {} "${DATA_DIR}/videos/" \;
+echo "Processing videos completed."
+
+rm -rf "${DATA_DIR}/YouTubeClips"
+rm -rf "${DATA_DIR}/msvd_data"
+rm msvd_data.zip
+rm YouTubeClips.tar
+echo "The preparation of the msvd dataset has been successfully completed."
diff --git a/tools/deployment/export_onnx_gcn.py b/tools/deployment/export_onnx_gcn.py
index a4fd237a59..b9cb8423a6 100644
--- a/tools/deployment/export_onnx_gcn.py
+++ b/tools/deployment/export_onnx_gcn.py
@@ -122,7 +122,7 @@ def main():
base_output = base_model(
input_tensor.unsqueeze(0), data_samples=[data_sample],
mode='predict')[0]
- base_output = base_output.pred_scores.item.detach().cpu().numpy()
+ base_output = base_output.pred_score.detach().cpu().numpy()
model = GCNNet(base_model).to(args.device)
model.eval()
diff --git a/tools/deployment/export_onnx_posec3d.py b/tools/deployment/export_onnx_posec3d.py
index 014096b48e..f8950dd8c8 100644
--- a/tools/deployment/export_onnx_posec3d.py
+++ b/tools/deployment/export_onnx_posec3d.py
@@ -118,7 +118,7 @@ def main():
base_output = base_model(
input_tensor.unsqueeze(0), data_samples=[data_sample],
mode='predict')[0]
- base_output = base_output.pred_scores.item.detach().cpu().numpy()
+ base_output = base_output.pred_score.detach().cpu().numpy()
model = GCNNet(base_model).to(args.device)
model.eval()
diff --git a/tools/test.py b/tools/test.py
index 4f310fa9e0..e1e62e16f7 100644
--- a/tools/test.py
+++ b/tools/test.py
@@ -6,6 +6,8 @@
from mmengine.config import Config, DictAction
from mmengine.runner import Runner
+from mmaction.registry import RUNNERS
+
def parse_args():
parser = argparse.ArgumentParser(
@@ -108,7 +110,13 @@ def main():
cfg.load_from = args.checkpoint
# build the runner from config
- runner = Runner.from_cfg(cfg)
+ if 'runner_type' not in cfg:
+ # build the default runner
+ runner = Runner.from_cfg(cfg)
+ else:
+ # build customized runner from the registry
+ # if 'runner_type' is set in the cfg
+ runner = RUNNERS.build(cfg)
# start testing
runner.test()
diff --git a/tools/train.py b/tools/train.py
index 74980a99e0..c7f4892332 100644
--- a/tools/train.py
+++ b/tools/train.py
@@ -6,6 +6,8 @@
from mmengine.config import Config, DictAction
from mmengine.runner import Runner
+from mmaction.registry import RUNNERS
+
def parse_args():
parser = argparse.ArgumentParser(description='Train a action recognizer')
@@ -125,7 +127,13 @@ def main():
cfg = merge_args(cfg, args)
# build the runner from config
- runner = Runner.from_cfg(cfg)
+ if 'runner_type' not in cfg:
+ # build the default runner
+ runner = Runner.from_cfg(cfg)
+ else:
+ # build customized runner from the registry
+ # if 'runner_type' is set in the cfg
+ runner = RUNNERS.build(cfg)
# start training
runner.train()